What The Sigma
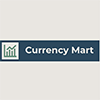
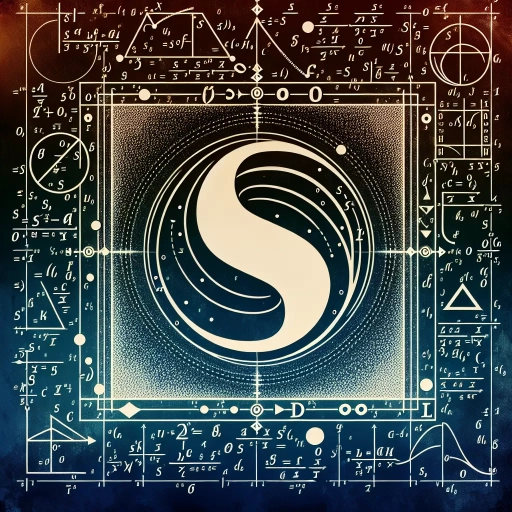
In the realm of statistics and data analysis, the term "sigma" holds significant importance, yet it remains a concept often shrouded in mystery for those outside the field. Sigma, denoted by the Greek letter σ, is a fundamental measure that underpins various statistical and analytical processes. This article aims to demystify sigma by delving into its core concept, its pivotal role in statistics and data analysis, and its practical applications across diverse fields. We will begin by **Understanding the Concept of Sigma**, where we will explore its definition, calculation, and significance in statistical contexts. This foundational understanding will then be built upon in **The Role of Sigma in Statistics and Data Analysis**, where we will examine how sigma influences statistical inference, hypothesis testing, and data interpretation. Finally, we will discuss **Practical Uses of Sigma in Various Fields**, highlighting its impact on decision-making in industries such as finance, healthcare, and engineering. By the end of this journey, readers will have a comprehensive grasp of sigma's multifaceted role and its indispensable value in modern data-driven environments. Let us start by **Understanding the Concept of Sigma**.
Understanding the Concept of Sigma
In the realm of statistics and quality control, the concept of sigma has emerged as a cornerstone for measuring precision and reliability. Understanding the concept of sigma is crucial for anyone seeking to grasp the intricacies of data analysis, process improvement, and performance metrics. This article delves into the multifaceted nature of sigma, exploring its historical context and origins, which trace back to the early 20th century and the pioneering work of Walter Shewhart. It also delves into the mathematical definition and formula behind sigma, providing a clear understanding of how it is calculated and interpreted. Furthermore, we examine real-world applications of sigma, highlighting its significance in industries such as manufacturing, healthcare, and finance. By navigating these three key aspects—historical context, mathematical definition, and real-world applications—this article aims to provide a comprehensive overview that transitions seamlessly into understanding the concept of sigma. Whether you are a seasoned professional or an inquisitive learner, this exploration will equip you with the knowledge necessary to appreciate the profound impact of sigma in various fields. Understanding the concept of sigma is not just about numbers; it's about enhancing quality, driving innovation, and achieving excellence.
Historical Context and Origins
The concept of "sigma" has its roots in various historical and cultural contexts, each contributing to the multifaceted understanding of this term. Historically, the term "sigma" originates from ancient Greek, where it was the 18th letter of the Greek alphabet. In mathematics and statistics, sigma (σ) is used to denote standard deviation, a measure of the amount of variation or dispersion of a set of values. This statistical context is crucial for understanding data distribution and variability. In social dynamics, the term "sigma" has been adopted by some modern theorists to describe individuals who operate outside the traditional hierarchical structures of society. This usage is often associated with the "alpha," "beta," and "omega" labels that categorize people based on their perceived social status and dominance. The sigma male, in particular, is seen as an outlier who rejects conventional social hierarchies and operates independently, neither seeking nor needing validation from others. Philosophically, the idea of being a sigma can be linked to existentialist thought, where individuals are seen as free agents responsible for their own choices and actions. This perspective aligns with the concept of the "sigma" as someone who defies categorization and instead forges their own path in life. Culturally, the sigma archetype can be observed in various forms of literature and media. For instance, characters like the Lone Ranger or the protagonist in Albert Camus's "The Stranger" embody qualities of independence and nonconformity that are central to the sigma persona. Understanding these diverse origins and contexts is essential for grasping the full scope of what it means to be a sigma. It is not merely a label but a complex interplay of social, philosophical, and cultural elements that define an individual's stance within society. By recognizing these historical and conceptual underpinnings, one can better appreciate the nuances of the sigma concept and its relevance in contemporary discourse. This multifaceted approach allows for a deeper exploration into how sigmas navigate and influence their environments, challenging traditional norms while forging unique paths that reflect their autonomy and self-determination.
Mathematical Definition and Formula
In the realm of mathematics, definitions and formulas serve as the foundational pillars upon which various concepts are built. When delving into the concept of sigma, it is crucial to understand its mathematical underpinnings. Sigma, often represented by the Greek letter Σ, is a symbol that denotes summation in mathematics. The **summation formula** is defined as: \[ \sum_{i=1}^{n} a_i = a_1 + a_2 + \cdots + a_n \] This formula indicates that the sum of a sequence of numbers \(a_i\) from \(i=1\) to \(n\) is calculated by adding each term in the sequence. For instance, if we have a series of numbers \(1, 2, 3, 4, 5\), the summation would be: \[ \sum_{i=1}^{5} i = 1 + 2 + 3 + 4 + 5 = 15 \] Understanding this basic definition is essential because it forms the basis for more complex mathematical operations and theories. In statistics, sigma is also used to represent standard deviation, which measures the amount of variation or dispersion from the average. The **standard deviation formula** for a population is given by: \[ \sigma = \sqrt{\frac{\sum_{i=1}^{n} (x_i - \mu)^2}{n}} \] where \(x_i\) are individual data points, \(\mu\) is the mean, and \(n\) is the number of data points. This formula highlights how sigma encapsulates the spread of data around its mean value. Moreover, in calculus, sigma notation is used to express definite integrals as sums. The **Riemann sum**, which approximates the area under a curve, can be written using sigma notation: \[ \sum_{i=1}^{n} f(x_i) \Delta x \] where \(f(x_i)\) is the function value at each interval and \(\Delta x\) is the width of each interval. The versatility of sigma in mathematics underscores its importance across various disciplines. From basic arithmetic to advanced statistical analysis and calculus, understanding the mathematical definition and formula behind sigma provides a robust foundation for further exploration and application of these concepts. By grasping these fundamental principles, one can better appreciate how sigma contributes to our understanding of data, functions, and mathematical structures in general. This foundational knowledge not only enhances problem-solving skills but also fosters deeper insights into the intricate world of mathematics.
Real-World Applications
Understanding the concept of sigma, a statistical measure of standard deviation, opens up a wide array of real-world applications that are both practical and impactful. In finance, sigma is crucial for risk management and portfolio optimization. For instance, the volatility of stocks and bonds can be measured using standard deviation, helping investors gauge potential risks and returns. This information is vital for creating diversified portfolios that balance risk and reward. In healthcare, sigma is used in quality control to ensure consistent patient care. The Six Sigma methodology, which aims to reduce defects to near zero, has been adopted by many hospitals to improve patient outcomes and streamline processes. This approach involves rigorous data analysis to identify and eliminate variations in care, leading to higher quality services. In manufacturing, sigma plays a key role in quality assurance. Companies like General Electric and Motorola have successfully implemented Six Sigma programs to enhance product reliability and reduce production costs. By minimizing defects and variability in production processes, these companies can maintain high standards of quality while improving efficiency. In environmental science, sigma helps in understanding climate variability and predicting weather patterns. Climate models use standard deviation to quantify the uncertainty associated with temperature and precipitation forecasts, enabling better decision-making for policymakers and researchers. In sports analytics, sigma is used to evaluate player performance and team strategies. Advanced metrics such as sabermetrics in baseball rely on statistical analysis, including standard deviation, to assess player consistency and predict future performance. This data-driven approach has revolutionized how teams evaluate talent and make strategic decisions. Additionally, in social sciences, sigma aids in understanding population trends and behaviors. Demographers use standard deviation to analyze demographic data, such as population growth rates and income distributions, which helps in formulating public policies that address societal needs effectively. Moreover, in technology and software development, sigma is essential for testing and quality assurance. Software companies use statistical methods to identify bugs and ensure that their products meet high standards of reliability before release. This rigorous testing process involves analyzing data to detect anomalies and variations that could impact user experience. Overall, the concept of sigma is not just a theoretical construct but a powerful tool with diverse applications across various fields, enhancing decision-making, improving quality, and driving innovation. By understanding sigma, professionals can leverage data more effectively to achieve better outcomes in their respective domains.
The Role of Sigma in Statistics and Data Analysis
In the realm of statistics and data analysis, the concept of sigma (σ) plays a pivotal role in understanding and interpreting data. Sigma, often referred to as the standard deviation, is a measure of the amount of variation or dispersion in a set of values. This fundamental concept is crucial for several key statistical processes. First, it is integral to calculating **Standard Deviation and Variance**, which help in quantifying the spread of data points from their mean value. Second, sigma is essential in constructing **Confidence Intervals and Hypothesis Testing**, enabling researchers to make informed decisions about populations based on sample data. Third, it is vital in **Regression Analysis and Correlation**, where it aids in assessing the strength and direction of relationships between variables. Understanding these applications of sigma is essential for any data analyst or statistician, as it provides a robust framework for interpreting and analyzing data. By delving into these aspects, we can gain a deeper appreciation for the significance of sigma in statistical analysis, ultimately leading to a comprehensive understanding of the concept itself. Therefore, this article aims to explore the multifaceted role of sigma in statistics, transitioning seamlessly into **Understanding the Concept of Sigma**.
Standard Deviation and Variance
Standard Deviation and Variance are fundamental concepts in statistics and data analysis, playing a crucial role in understanding the dispersion of data points within a dataset. **Standard Deviation**, often denoted by the Greek letter sigma (σ), measures the amount of variation or dispersion from the average value of a set of data. It quantifies how much each data point deviates from the mean, providing insight into the spread or volatility of the dataset. A low standard deviation indicates that the data points are close to the mean, while a high standard deviation suggests that the data points are spread out over a wider range. **Variance**, on the other hand, is the average of the squared differences from the mean. It is calculated as the square of the standard deviation (σ²) and provides another way to measure dispersion. Variance is particularly useful because it allows for easier mathematical manipulation and is essential in various statistical analyses such as regression and hypothesis testing. The relationship between variance and standard deviation is symbiotic; knowing one allows you to easily calculate the other. In practical terms, these metrics are indispensable in various fields. For instance, in finance, standard deviation is used to assess the risk associated with an investment by measuring its volatility. In quality control, manufacturers use these metrics to ensure that products meet certain standards by monitoring the variability in production processes. In research, scientists rely on standard deviation and variance to understand the reliability of their findings and to make informed decisions about sample sizes. The role of sigma (standard deviation) in statistics extends beyond mere measurement; it is a cornerstone for many statistical tests and models. For example, the normal distribution (Gaussian distribution) is characterized by its mean and standard deviation, making sigma a key parameter in understanding probabilities and confidence intervals. Additionally, sigma is integral in hypothesis testing where it helps determine whether observed differences are statistically significant. In summary, Standard Deviation and Variance are essential tools for understanding data dispersion. They provide critical insights into how data points are spread out around the mean, enabling better decision-making across various disciplines. The significance of sigma in these contexts underscores its importance as a fundamental parameter in statistical analysis and data interpretation. By grasping these concepts, analysts can better navigate complex datasets, identify trends, and make more accurate predictions. This foundational understanding of sigma's role sets the stage for more advanced statistical techniques and ensures robust data analysis practices.
Confidence Intervals and Hypothesis Testing
In the realm of statistical analysis, Confidence Intervals and Hypothesis Testing are two fundamental concepts that play crucial roles in understanding and interpreting data, particularly when considering the role of sigma (standard deviation) in these processes. **Confidence Intervals** provide a range of values within which a population parameter is likely to lie, based on a sample of data. For instance, if we are estimating the average height of a population, a 95% confidence interval might suggest that the true average height lies between 170 and 180 cm. The width of this interval is influenced by sigma; a smaller standard deviation (sigma) results in a narrower interval, indicating more precise estimation. This precision is vital because it allows researchers to make more accurate inferences about the population from their sample data. **Hypothesis Testing**, on the other hand, involves making decisions about a population parameter based on sample data. Here, sigma is essential for calculating test statistics and determining the significance of results. For example, in a t-test or z-test, the standard deviation of the sample (or population if known) is used to standardize the test statistic. This standardization helps in comparing the observed difference or relationship against what would be expected by chance alone. A smaller sigma generally leads to more powerful tests because it reduces the variability in the sample, making it easier to detect statistically significant differences or relationships. The interplay between Confidence Intervals and Hypothesis Testing is also noteworthy. While Confidence Intervals provide an estimate with a margin of error, Hypothesis Testing offers a binary decision (reject or fail to reject the null hypothesis). Both methods rely on sigma to quantify uncertainty and variability in data. In practice, researchers often use these methods in tandem; for instance, if a hypothesis test indicates a significant difference between two groups, a confidence interval can provide insight into the magnitude of this difference. Understanding sigma's role in these statistical tools is paramount because it directly affects the reliability and precision of conclusions drawn from data analysis. A well-estimated sigma ensures that confidence intervals are appropriately wide or narrow and that hypothesis tests have adequate power to detect true effects. Misestimation of sigma can lead to misleading results—either overly broad intervals that offer little practical insight or tests that fail to detect significant effects due to excessive variability. In summary, Confidence Intervals and Hypothesis Testing are indispensable in statistical analysis, and their effectiveness is heavily dependent on accurate estimation of sigma. By grasping how sigma influences these methods, researchers can make more informed decisions and draw more reliable conclusions from their data, ultimately enhancing the quality and validity of their findings. This underscores the critical role of sigma in ensuring robust and meaningful statistical inference.
Regression Analysis and Correlation
Regression analysis and correlation are fundamental concepts in statistics and data analysis, playing a crucial role in understanding the relationships between variables. **Regression analysis** is a statistical method used to establish a relationship between two or more variables, typically to predict the value of one variable based on the values of others. This technique helps in modeling the relationship between a dependent variable (the outcome) and one or more independent variables (the predictors). For instance, in a simple linear regression, the relationship is modeled using a straight line that best fits the data points, allowing for the prediction of the dependent variable's value given the independent variable's value. Multiple regression extends this concept by incorporating multiple independent variables to improve the accuracy of predictions. **Correlation**, on the other hand, measures the strength and direction of the linear relationship between two variables. It is quantified using the correlation coefficient, which ranges from -1 to 1. A correlation coefficient close to 1 indicates a strong positive linear relationship, while a coefficient close to -1 indicates a strong negative linear relationship. A coefficient near 0 suggests no linear relationship. Correlation does not imply causation; it merely indicates that as one variable changes, the other variable tends to change in a predictable manner. Understanding correlation is essential because it helps in identifying potential predictors for regression models and in assessing the reliability of these models. The interplay between regression and correlation is significant. In regression analysis, correlation is often used as a preliminary step to identify which variables are likely to be good predictors. For example, if two variables have a high correlation coefficient, it suggests that they are strongly related, making one a good candidate for inclusion in a regression model. Conversely, if the correlation is weak, it may indicate that the variable is not a useful predictor. In the context of **The Role of Sigma in Statistics and Data Analysis**, sigma (σ) represents the standard deviation of a population or sample. In regression and correlation analysis, sigma is crucial for understanding the variability of the data and the reliability of the models. For instance, the standard error of the regression coefficient is calculated using sigma, which helps in determining the precision of the estimated relationship. Additionally, sigma is used in hypothesis testing related to regression and correlation, such as testing whether a correlation coefficient is significantly different from zero. In summary, regression analysis and correlation are powerful tools for uncovering relationships within data sets. By understanding how these techniques interact with measures of variability like sigma, analysts can build more accurate and reliable models, ultimately enhancing their ability to make informed decisions based on data. This integration of concepts underscores the importance of a comprehensive approach to statistical analysis, where each component—regression, correlation, and measures like sigma—contributes to a deeper understanding of the data's underlying structure.
Practical Uses of Sigma in Various Fields
In today's fast-paced and data-driven world, the concept of Sigma has emerged as a pivotal tool across various fields, enhancing efficiency, accuracy, and decision-making. Sigma, often associated with the Six Sigma methodology, is a measure of quality that signifies the number of standard deviations from the mean in a normal distribution. This statistical metric has far-reaching practical applications that transcend traditional manufacturing processes. For instance, in **Quality Control in Manufacturing**, Sigma helps companies achieve near-perfect production by minimizing defects and optimizing processes. In **Financial Risk Management**, it aids in assessing and mitigating risks by analyzing data variability and potential deviations from expected outcomes. Additionally, **Medical Research and Clinical Trials** benefit from Sigma by ensuring the reliability and precision of experimental results, thereby informing critical healthcare decisions. Understanding these diverse applications underscores the importance of grasping the fundamental concept of Sigma. As we delve into these specific areas, it becomes clear that mastering Sigma is essential for achieving excellence in multiple disciplines. Therefore, it is crucial to **Understand the Concept of Sigma** to fully appreciate its transformative potential.
Quality Control in Manufacturing
Quality control in manufacturing is a critical component that ensures the production of high-quality products, enhancing customer satisfaction and reducing the risk of defects. It involves a systematic approach to monitoring and controlling processes to meet specified standards. In the context of Six Sigma, a data-driven methodology, quality control is elevated to a precise science. Six Sigma employs statistical tools and techniques to identify and eliminate defects, aiming for near-perfect quality by achieving a defect rate of no more than 3.4 per million opportunities. The implementation of Six Sigma in manufacturing begins with defining key performance indicators (KPIs) and setting clear goals for quality improvement. The DMAIC (Define, Measure, Analyze, Improve, Control) framework is commonly used to structure these efforts. During the Define phase, projects are identified and objectives are set. The Measure phase involves collecting data to understand current processes, while the Analyze phase uses statistical methods to identify root causes of defects. In the Improve phase, solutions are developed and tested, and finally, in the Control phase, new processes are implemented and monitored to ensure sustainability. Quality control teams in manufacturing often include certified professionals such as Green Belts, Black Belts, and Master Black Belts who lead and support these initiatives. These professionals are trained in advanced statistical techniques like regression analysis, hypothesis testing, and design of experiments. They use tools such as control charts, Pareto charts, and fishbone diagrams to visualize data and identify areas for improvement. The practical application of Six Sigma in manufacturing has numerous benefits. It leads to significant reductions in defect rates, resulting in lower production costs and improved product reliability. Enhanced quality also boosts customer trust and loyalty, driving business growth. Additionally, Six Sigma fosters a culture of continuous improvement within organizations, encouraging employees to contribute to quality initiatives and fostering a collaborative environment. In various industries, from automotive to pharmaceuticals, Six Sigma has been instrumental in achieving high-quality standards. For instance, in the automotive sector, Six Sigma has helped manufacturers like General Motors and Ford reduce defect rates in their vehicles, enhancing safety and performance. Similarly, in the pharmaceutical industry, companies like Pfizer have used Six Sigma to ensure the quality of their products, adhering to stringent regulatory requirements. In summary, quality control in manufacturing is a vital process that leverages methodologies like Six Sigma to achieve exceptional product quality. By employing rigorous statistical analysis and structured frameworks, manufacturers can significantly improve their processes, reduce defects, and enhance customer satisfaction. This approach not only benefits the organization financially but also contributes to a culture of excellence and continuous improvement.
Financial Risk Management
Financial risk management is a critical component in the broader spectrum of risk management, particularly when discussing the practical uses of sigma in various fields. Sigma, or standard deviation, is a statistical measure that quantifies the amount of variation or dispersion of a set of data values. In financial risk management, sigma plays a pivotal role in assessing and mitigating potential risks associated with investments, portfolios, and financial instruments. For instance, in portfolio management, sigma is used to measure the volatility of asset returns. A higher sigma indicates greater volatility, suggesting that the asset's returns are more likely to deviate from the mean. This information is crucial for investors and portfolio managers who need to balance risk and return. By understanding the sigma of different assets, they can construct diversified portfolios that minimize risk while maximizing returns. Additionally, sigma is integral in calculating Value-at-Risk (VaR), a widely used metric to estimate the potential loss in portfolio value over a specific time horizon with a given probability. In derivatives trading, sigma is essential for pricing options and other financial derivatives. The Black-Scholes model, for example, relies heavily on sigma to determine the fair price of an option. Here, sigma represents the volatility of the underlying asset's price, which directly influences the option's premium. Accurate estimation of sigma is vital because it affects the perceived risk and hence the price of the derivative. Moreover, financial institutions use sigma to manage operational risk. By analyzing historical data on operational losses, firms can estimate the standard deviation of these losses and develop strategies to mitigate them. This approach helps in setting aside appropriate capital reserves and implementing risk-reducing measures. Regulatory bodies also leverage sigma in their oversight roles. For example, Basel II and III accords require banks to maintain sufficient capital buffers based on their risk-weighted assets, which are often calculated using sigma-based models. These regulations ensure that banks have adequate capital to absorb potential losses, thereby enhancing financial stability. In summary, sigma is a fundamental metric in financial risk management, enabling practitioners to quantify and manage various types of financial risks effectively. Its applications span portfolio optimization, derivatives pricing, operational risk management, and regulatory compliance. By understanding and applying sigma accurately, financial professionals can make informed decisions that balance risk and reward, ultimately contributing to the stability and efficiency of financial markets. This underscores the significance of sigma not just as a statistical tool but as a cornerstone of practical risk management strategies across diverse financial domains.
Medical Research and Clinical Trials
Medical research and clinical trials are pivotal in advancing healthcare, and they heavily rely on statistical analysis to ensure the validity and reliability of their findings. One of the key statistical concepts used in these fields is the sigma level, which measures the standard deviation from the mean in a normal distribution. In medical research, sigma levels are crucial for understanding the variability of data, such as patient outcomes, treatment efficacy, and side effects. For instance, in clinical trials, researchers often aim to achieve a high sigma level (e.g., six sigma) to minimize errors and ensure that the results are highly reliable. This involves rigorous data collection, precise measurement tools, and robust statistical analysis. The practical application of sigma in medical research can be seen in several areas. Firstly, it helps in the design of clinical trials by determining the appropriate sample size needed to detect statistically significant differences between treatment groups. This ensures that the trial is adequately powered to provide conclusive results. Secondly, sigma levels are used to monitor and control the quality of data during the trial, helping to identify any anomalies or outliers that could skew the results. For example, in pharmaceutical development, achieving a six sigma level in manufacturing processes ensures that the medication produced meets stringent quality standards, reducing the risk of adverse reactions. Moreover, sigma is essential in evaluating the effectiveness of new treatments. By analyzing data with high sigma levels, researchers can confidently determine whether a new drug or therapy is superior to existing treatments. This not only aids in regulatory approvals but also informs healthcare providers about the best treatment options for their patients. Additionally, sigma plays a role in personalized medicine by helping to identify genetic variations that may influence how individuals respond to different treatments. This precision medicine approach relies on high-quality data with minimal variability to tailor treatments to individual patient profiles. In summary, the application of sigma in medical research and clinical trials is fundamental for ensuring the accuracy, reliability, and generalizability of findings. By leveraging statistical methods that emphasize high sigma levels, researchers can enhance the quality of their studies, improve patient outcomes, and advance the field of medicine. This rigorous approach to data analysis underscores the importance of sigma in various fields beyond just manufacturing and quality control, highlighting its critical role in driving scientific progress and improving human health.