What Is T5
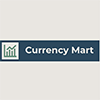
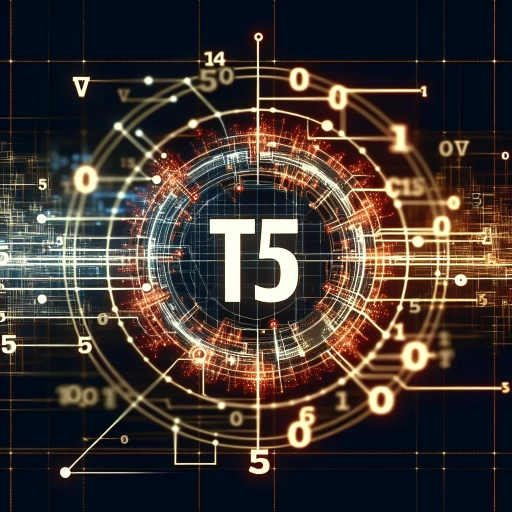
In the realm of artificial intelligence, particularly within the domain of natural language processing, the T5 (Text-to-Text Transfer Transformer) model has emerged as a groundbreaking innovation. Developed by Google, T5 represents a significant leap forward in machine learning capabilities, offering a unified approach to various NLP tasks. This article delves into the intricacies of T5, providing a comprehensive overview that spans its fundamental principles, technical mechanics, and real-world applications. We begin by **Understanding the Basics of T5**, where we explore the model's architecture and the underlying concepts that make it so powerful. From there, we dive deeper into **How T5 Works: Technical Insights**, examining the intricate details of its operation and what sets it apart from other models. Finally, we discuss **Applications and Impact of T5**, highlighting its practical uses and the profound impact it has on industries ranging from customer service to content generation. By the end of this journey, you will have a thorough grasp of what T5 is and why it stands at the forefront of AI research. Let's start by **Understanding the Basics of T5**.
Understanding the Basics of T5
In the realm of natural language processing, the T5 (Text-to-Text Transfer Transformer) model has emerged as a groundbreaking innovation, revolutionizing how we approach text generation and understanding. To fully grasp the capabilities and significance of T5, it is essential to delve into its core aspects. This article will provide a comprehensive overview by exploring three critical dimensions: **Definition and Purpose**, which will clarify what T5 is and its intended applications; **Key Components and Architecture**, detailing the structural elements that make T5 so effective; and **Historical Context and Development**, tracing the evolution of this model within the broader landscape of AI research. By understanding these foundational elements, readers will gain a deeper insight into the mechanics and impact of T5, ultimately enhancing their comprehension of this powerful tool. This journey through the basics of T5 will equip you with the knowledge needed to appreciate its potential and integrate it into various applications, making it an indispensable resource for anyone looking to leverage this technology. Understanding the Basics of T5 is crucial for harnessing its full potential, and this article is your gateway to that understanding.
Definition and Purpose
**Understanding the Basics of T5: Definition and Purpose** T5, or Text-to-Text Transfer Transformer, is a revolutionary AI model developed by Google Research that redefines the paradigm of natural language processing (NLP). At its core, T5 is a unified framework that treats every NLP task as a text-to-text problem, simplifying the complexity of diverse tasks into a single, coherent model architecture. This innovative approach allows T5 to excel in various NLP tasks such as translation, summarization, question answering, and text classification, among others. The purpose of T5 is multifaceted. Primarily, it aims to streamline the process of training and fine-tuning NLP models by providing a consistent interface for all tasks. This consistency enables researchers and developers to focus on improving the model's performance without the need to adapt to different task-specific architectures. By converting all tasks into text-to-text format, T5 leverages the power of large-scale pre-training, allowing it to learn robust representations of language that can be easily adapted to new tasks with minimal additional training. Moreover, T5's unified framework promotes efficiency and scalability. It reduces the overhead associated with maintaining multiple task-specific models, making it more feasible for deployment in real-world applications. This efficiency is particularly beneficial in scenarios where computational resources are limited or where rapid prototyping and iteration are crucial. Additionally, T5's ability to handle a wide range of tasks under a single architecture facilitates better knowledge sharing across different NLP domains, leading to improved overall performance. Another significant aspect of T5 is its flexibility. The model can be fine-tuned for specific tasks using task-specific prefixes, which guide the model on how to process the input text. This flexibility allows T5 to be tailored for various applications, from generating creative content to assisting in critical tasks like medical diagnosis or legal document analysis. The model's adaptability also makes it an attractive choice for researchers exploring new frontiers in NLP, as it provides a robust foundation for experimenting with novel tasks and datasets. In summary, T5's definition as a unified text-to-text transformer model underscores its purpose: to simplify, streamline, and enhance the capabilities of NLP systems. By treating all NLP tasks uniformly and leveraging large-scale pre-training, T5 offers unparalleled efficiency, scalability, and flexibility. This makes it an indispensable tool for both researchers and practitioners seeking to push the boundaries of what is possible with natural language processing. As the field continues to evolve, T5 stands as a beacon of innovation, ready to support the next generation of NLP applications and advancements.
Key Components and Architecture
Understanding the basics of T5, a text-to-text transformer model developed by Google, requires a deep dive into its key components and architecture. At its core, T5 leverages the transformer architecture, which is renowned for its ability to handle sequential data efficiently. The model is built around the encoder-decoder framework, where the encoder processes input text and the decoder generates output text. This setup allows T5 to perform a wide range of natural language processing tasks, from translation and summarization to question answering and text generation. A crucial component of T5 is its use of a shared vocabulary and model weights across both the encoder and decoder. This shared architecture simplifies training and improves performance by allowing the model to learn a unified representation of language that can be applied across various tasks. The model also employs a technique called "text-to-text" pre-training, where all tasks are framed as text generation problems. This approach enables T5 to generalize well across different tasks without requiring task-specific fine-tuning. The architecture of T5 includes multiple layers of self-attention mechanisms, which allow the model to weigh the importance of different input elements relative to each other. This self-attention mechanism is pivotal in capturing long-range dependencies within the input text, a feature that is particularly beneficial for tasks that require understanding complex contexts. Additionally, T5 uses positional embeddings to preserve the order of input tokens, ensuring that the model can effectively process sequential data. Another key aspect of T5's architecture is its use of multi-task learning during pre-training. By training on a diverse set of tasks simultaneously, T5 develops a robust understanding of language that can be adapted to various downstream tasks. This multi-task approach helps in reducing overfitting and improves the model's ability to generalize to unseen data. In summary, the architecture of T5 is designed to maximize flexibility and performance across a broad spectrum of NLP tasks. By leveraging a shared encoder-decoder framework, text-to-text pre-training, self-attention mechanisms, and multi-task learning, T5 sets a new standard for transformer-based models in natural language processing. Understanding these components is essential for appreciating how T5 achieves its impressive results and for effectively utilizing it in real-world applications.
Historical Context and Development
The development of T5, or Text-to-Text Transfer Transformer, is deeply rooted in the historical context of natural language processing (NLP) and the evolution of transformer models. The journey began with the introduction of recurrent neural networks (RNNs) and long short-term memory (LSTM) networks, which were pivotal in early NLP tasks but faced limitations such as sequential processing and difficulty in capturing long-range dependencies. The breakthrough came with the advent of transformer models, first introduced in the paper "Attention Is All You Need" by Vaswani et al. in 2017. This architecture revolutionized NLP by leveraging self-attention mechanisms to process input sequences in parallel, significantly improving performance on tasks like machine translation. Building on this foundation, subsequent models such as BERT (Bidirectional Encoder Representations from Transformers) and its variants further enhanced the state-of-the-art by incorporating pre-training techniques. BERT's success in understanding context through masked language modeling paved the way for more sophisticated models. The next significant leap was the introduction of T5, developed by the Google Brain team and presented in the paper "Exploring the Limits of Transfer Learning with a Unified Text-to-Text Transformer" by Raffel et al. in 2020. T5 unified various NLP tasks under a single text-to-text framework, treating every problem as a sequence-to-sequence task. This approach simplified the training process and allowed for more efficient transfer learning across different tasks. T5's historical context is also marked by advancements in computational power and large-scale datasets. The availability of massive datasets like Common Crawl and Wikipedia enabled large-scale pre-training, which was crucial for T5's performance. Additionally, improvements in hardware and distributed training techniques facilitated the training of these massive models. The development of T5 reflects a broader trend in AI research towards more generalizable and versatile models that can adapt to a wide range of tasks without extensive task-specific fine-tuning. This shift underscores the ongoing quest for models that can learn, generalize, and apply knowledge across diverse linguistic contexts, making T5 a significant milestone in the journey towards more intelligent and flexible NLP systems. Understanding T5 within this historical context provides a deeper appreciation for its innovative design and its potential to drive future advancements in NLP.
How T5 Works: Technical Insights
The T5 (Text-to-Text Transfer Transformer) model, developed by Google, represents a significant advancement in natural language processing (NLP) by unifying various NLP tasks under a single text-to-text framework. This innovative approach simplifies the complexity of handling diverse tasks such as translation, summarization, and question answering, making it a powerful tool for AI researchers and practitioners. To fully appreciate the capabilities of T5, it is essential to delve into its technical insights. This article will explore three key aspects of T5: the **Text-to-Text Framework**, which explains how T5 integrates multiple NLP tasks into a cohesive system; the **Training Process and Data Requirements**, detailing the extensive datasets and computational resources needed to train the model; and **Model Variants and Customization**, highlighting the flexibility and adaptability of T5 for specific applications. By understanding these components, readers will gain a comprehensive grasp of how T5 works, setting the stage for a deeper exploration of its underlying mechanics and potential applications. Transitioning seamlessly into **Understanding the Basics of T5**, this introduction lays the groundwork for a thorough examination of what makes T5 a groundbreaking model in the field of NLP.
Text-to-Text Framework
The Text-to-Text Framework, a cornerstone of the T5 (Text-to-Text Transfer Transformer) model, revolutionizes how we approach natural language processing tasks by unifying them under a single, versatile framework. This innovative approach simplifies the complexity of various NLP tasks by converting them into text-to-text transformations, thereby leveraging the power of a single model to handle diverse tasks such as translation, summarization, question answering, and even text classification. At its core, the Text-to-Text Framework operates by framing every task as a sequence-to-sequence problem, where input text is transformed into output text through a series of learned transformations. This framework's elegance lies in its ability to abstract away task-specific nuances, allowing the model to focus on learning generalizable patterns and relationships within language. For instance, translating English to Spanish or summarizing a long document can be treated as analogous tasks within this framework, where the input text is processed and transformed into the desired output text. This uniformity enables T5 to be pre-trained on a vast array of tasks simultaneously, enhancing its ability to generalize across different domains and tasks. The technical insights behind this framework are rooted in the transformer architecture, which relies on self-attention mechanisms to capture contextual dependencies within input sequences. By pre-training T5 on a large corpus of text data with diverse tasks, the model develops a robust understanding of linguistic structures and semantic relationships. This pre-training phase is crucial as it equips the model with a broad knowledge base that can be fine-tuned for specific tasks, leading to state-of-the-art performance in many NLP benchmarks. Moreover, the Text-to-Text Framework facilitates easier model development and deployment. Developers no longer need to design and train separate models for each NLP task; instead, they can leverage a single, pre-trained T5 model and fine-tune it for their specific needs. This not only reduces the computational resources required but also accelerates the development cycle, making it more feasible to integrate advanced NLP capabilities into various applications. In summary, the Text-to-Text Framework is a powerful paradigm that simplifies and unifies NLP tasks, enabling models like T5 to achieve remarkable versatility and performance. By converting all tasks into text-to-text transformations, this framework leverages the strengths of transformer architectures to deliver robust, generalizable models that can be easily adapted for a wide range of applications. This approach has significantly advanced the field of NLP, making it more accessible and efficient for both researchers and practitioners.
Training Process and Data Requirements
The training process of T5, or Text-to-Text Transfer Transformer, is a complex and data-intensive endeavor that underscores the model's versatility and effectiveness. At its core, T5 is trained using a paradigm known as "text-to-text" tasks, where the model learns to convert input text into output text through a variety of tasks such as translation, summarization, and question answering. This approach allows T5 to generalize across multiple NLP tasks, making it highly adaptable. ### Training Process 1. **Pre-training**: The initial phase involves pre-training the model on a massive corpus of text data. This corpus is typically sourced from diverse domains to ensure the model's exposure to a wide range of linguistic structures and styles. During pre-training, T5 is tasked with predicting missing spans of text within the input sequence, a process known as "masked language modeling." This task helps the model develop a deep understanding of language semantics and syntax. 2. **Fine-tuning**: After pre-training, the model is fine-tuned on specific downstream tasks. This involves adjusting the model's parameters to optimize performance on particular NLP tasks such as sentiment analysis, machine translation, or text classification. Fine-tuning allows T5 to specialize in tasks that require nuanced understanding and context-specific knowledge. 3. **Multi-task Learning**: A key feature of T5's training process is its use of multi-task learning. By training on multiple tasks simultaneously, the model can leverage shared knowledge across different tasks, enhancing its overall performance and robustness. This approach also helps in reducing overfitting by preventing the model from becoming overly specialized to any single task. ### Data Requirements 1. **Scale and Diversity**: The success of T5 heavily depends on the scale and diversity of the training data. The model requires vast amounts of text data to learn generalizable representations of language. Diverse datasets ensure that the model is exposed to various writing styles, genres, and linguistic patterns, which are crucial for its ability to generalize well across different tasks. 2. **Quality and Preprocessing**: High-quality data is essential for effective training. This includes preprocessing steps such as tokenization, normalization, and handling out-of-vocabulary words. Ensuring that the data is free from noise and biases is also critical to prevent the model from learning undesirable patterns. 3. **Task-Specific Data**: For fine-tuning, task-specific datasets are necessary. These datasets should be carefully curated to reflect the nuances and requirements of each specific NLP task. For instance, a sentiment analysis task would require labeled data indicating positive, negative, or neutral sentiments. In summary, the training process of T5 involves a combination of pre-training on large-scale text data and fine-tuning on task-specific datasets. The model's ability to handle diverse tasks through multi-task learning and its reliance on high-quality, diverse data make it a powerful tool in the realm of natural language processing. Understanding these aspects provides valuable insights into how T5 works and why it has become a benchmark in NLP research and applications.
Model Variants and Customization
In the realm of the T5 (Text-to-Text Transfer Transformer) model, one of the key strengths lies in its versatility and adaptability, which is largely facilitated by the concept of model variants and customization. The T5 architecture, developed by Google, is inherently designed to be flexible and modular, allowing researchers and practitioners to tailor the model to specific tasks and datasets. This flexibility is crucial because different tasks often require different configurations to achieve optimal performance. **Model Variants:** T5 comes in various sizes, ranging from the small T5 model with approximately 60 million parameters to the large T5 model with around 770 million parameters. Each variant is pre-trained on a massive corpus of text data, but they differ in their capacity to capture complex patterns and nuances. For instance, smaller models are more efficient and suitable for deployment on edge devices or in resource-constrained environments, while larger models offer superior performance on more complex tasks but require more computational resources. **Customization:** Beyond the pre-defined model sizes, T5 allows for extensive customization. One of the primary ways to customize T5 is through fine-tuning, where the pre-trained model is further trained on a specific dataset to adapt to the nuances of that particular task. This process can significantly enhance the model's performance on tasks such as sentiment analysis, question answering, or text summarization. Additionally, users can modify the model's architecture by adjusting hyperparameters like the number of layers, attention heads, or embedding dimensions to better suit their specific needs. **Task-Specific Adaptations:** Another layer of customization involves task-specific adaptations. For example, in tasks that require generating long-form text, such as article writing or story generation, users might adjust the model's decoding strategy or use techniques like beam search to improve coherence and quality. In contrast, for tasks that involve short-form responses, such as chatbots or customer service bots, the model can be optimized for quicker response times and more concise outputs. **Domain Adaptation:** Domain adaptation is another critical aspect of T5 customization. By fine-tuning the model on domain-specific data, users can adapt it to perform well in specialized domains such as legal documents, medical texts, or technical manuals. This domain-specific fine-tuning helps the model learn domain-specific terminology and concepts, leading to more accurate and relevant outputs. In summary, the ability to create and customize different variants of the T5 model is a powerful feature that makes it highly versatile and applicable across a wide range of natural language processing tasks. Whether it's through selecting an appropriate model size, fine-tuning on specific datasets, or making architectural adjustments, T5's customization options enable users to optimize performance for their unique needs, making it a valuable tool in the NLP toolkit.
Applications and Impact of T5
The T5 (Text-to-Text Transfer Transformer) model has revolutionized the field of natural language processing (NLP) with its versatile and powerful architecture. This transformative technology has far-reaching applications and significant impacts across various domains. In this article, we will delve into the multifaceted world of T5, exploring its capabilities and implications. First, we will examine how T5 excels in a wide range of **Natural Language Processing Tasks**, from text classification and machine translation to question answering and text generation. Next, we will highlight **Real-World Use Cases and Examples**, showcasing how T5 is being utilized in industries such as customer service, content creation, and data analysis. Finally, we will discuss the **Future Potential and Limitations** of T5, considering its potential for further innovation and the challenges it may face. By understanding these aspects, readers will gain a comprehensive insight into the applications and impact of T5, setting the stage for a deeper exploration of its underlying mechanics in **Understanding the Basics of T5**.
Natural Language Processing Tasks
Natural Language Processing (NLP) tasks are the backbone of modern AI applications, and the T5 model has significantly advanced these capabilities. NLP encompasses a wide range of tasks designed to enable computers to understand, interpret, and generate human language. One of the foundational tasks is **text classification**, where models like T5 are trained to categorize text into predefined categories such as spam vs. non-spam emails or positive vs. negative product reviews. Another crucial task is **sentiment analysis**, which involves determining the emotional tone or attitude conveyed by a piece of text, helping businesses gauge customer feedback and sentiment. **Machine translation** is another critical NLP task that T5 excels in, enabling the translation of text from one language to another with high accuracy. This has profound implications for global communication, facilitating cross-cultural interactions and breaking language barriers. **Named Entity Recognition (NER)** is another important task where models identify and classify named entities in unstructured text into predefined categories such as names, locations, and organizations. This is particularly useful in data extraction and information retrieval systems. T5 also performs exceptionally well in **question answering** tasks, where the model is given a passage and a question, and it must provide an accurate answer based on the information in the passage. This has significant applications in customer service chatbots and educational tools. Additionally, **text summarization** tasks involve condensing long documents into shorter summaries while retaining key information, which T5 handles with precision, making it invaluable for news aggregation services and research assistants. The impact of these NLP tasks extends beyond just technology; they have real-world applications that transform industries. For instance, in healthcare, NLP can be used to analyze patient records and medical literature to aid in diagnosis and treatment plans. In finance, NLP can help analyze market sentiment from news articles and social media posts to inform investment decisions. The versatility of T5 in handling various NLP tasks makes it a powerful tool for driving innovation across multiple sectors, enhancing efficiency, and improving decision-making processes. By leveraging T5's capabilities, developers can create more sophisticated AI systems that not only understand but also generate coherent and contextually relevant human language, opening up new avenues for automation and human-computer interaction.
Real-World Use Cases and Examples
The T5 (Text-to-Text Transfer Transformer) model, developed by Google, has revolutionized the field of natural language processing with its versatility and robust performance across a wide range of real-world use cases. One of the most compelling aspects of T5 is its ability to handle diverse tasks through a unified text-to-text framework, making it an invaluable tool for various applications. In **content generation**, T5 excels at producing coherent and contextually relevant text. For instance, it can be used in automated writing tools for generating articles, blog posts, or even entire books. Companies like Medium and WordPress have leveraged such models to assist writers in creating engaging content more efficiently. Additionally, T5 can be fine-tuned for specific domains such as technical writing or creative writing, enhancing the quality and relevance of the generated content. **Language translation** is another significant area where T5 demonstrates its prowess. By training on large multilingual datasets, T5 can translate text from one language to another with remarkable accuracy. This capability is crucial for global communication platforms like Google Translate or Microsoft Translator, enabling seamless interactions between people speaking different languages. T5 also finds extensive use in **customer service automation**. Chatbots powered by T5 can understand and respond to customer queries more effectively than traditional rule-based systems. For example, companies like Amazon and Apple use advanced language models to power their customer support chatbots, providing 24/7 assistance and improving customer satisfaction. Moreover, T5's **summarization capabilities** make it an essential tool for news aggregation services and research institutions. By condensing lengthy documents into concise summaries, T5 helps users quickly grasp the key points without having to read through entire articles or reports. This feature is particularly useful in industries where staying updated with the latest information is critical. In **education**, T5 can be employed to create personalized learning materials and adaptive assessments. Educational platforms can use T5 to generate customized lesson plans based on a student's learning style and pace, thereby enhancing the learning experience. Furthermore, T5's **question-answering abilities** make it a valuable asset for search engines and knowledge bases. By accurately identifying relevant information within vast amounts of data, T5 can provide precise answers to user queries, improving search engine results and user experience. Lastly, in **healthcare**, T5 can assist in clinical decision support systems by analyzing medical literature and patient records to provide healthcare professionals with timely insights and recommendations. This application has the potential to significantly improve patient outcomes by ensuring that healthcare providers have access to the most current and relevant medical information. In summary, the T5 model's adaptability and performance make it a cornerstone in various real-world applications, from content creation and language translation to customer service and healthcare. Its impact is felt across multiple industries, enhancing efficiency, accuracy, and user experience in ways that were previously unimaginable.
Future Potential and Limitations
The future potential of T5, a text-to-text transformer model developed by Google, is vast and promising, yet it also comes with several limitations that need to be addressed. On the potential side, T5's versatility in handling a wide range of natural language processing tasks makes it a powerful tool for various applications. For instance, its ability to perform tasks such as translation, summarization, and question answering with high accuracy opens up new avenues for enhancing user experience in search engines, chatbots, and content generation platforms. Additionally, T5's capability to be fine-tuned for specific domains can significantly improve the performance of specialized AI systems in fields like healthcare, finance, and education. This adaptability could lead to breakthroughs in personalized medicine through more accurate diagnosis and treatment recommendations, or in financial analysis by providing more precise market predictions. However, despite these promising prospects, there are several limitations that must be considered. One major limitation is the requirement for substantial computational resources and large datasets for training and fine-tuning T5 models. This can make it challenging for smaller organizations or individuals to leverage the full potential of T5 without significant investment in infrastructure. Another critical issue is the ethical concern surrounding bias in AI models; T5, like other large language models, can perpetuate existing biases present in the training data, leading to unfair outcomes and discrimination. Ensuring fairness and transparency in AI decision-making processes is crucial but remains a complex challenge. Moreover, the interpretability of T5 models is another area of concern. While they excel at generating coherent and contextually relevant text, understanding how they arrive at their conclusions can be difficult due to their complex architecture. This lack of interpretability can hinder trust in AI-driven decisions and limit their adoption in critical domains where accountability is paramount. In conclusion, while T5 holds immense potential for transforming various sectors through its advanced capabilities in natural language processing, it is essential to address its limitations proactively. By investing in more efficient training methods, ensuring ethical standards are met, and improving model interpretability, we can unlock the full potential of T5 and maximize its positive impact across diverse applications.