What Does Ml Mean
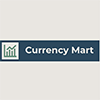
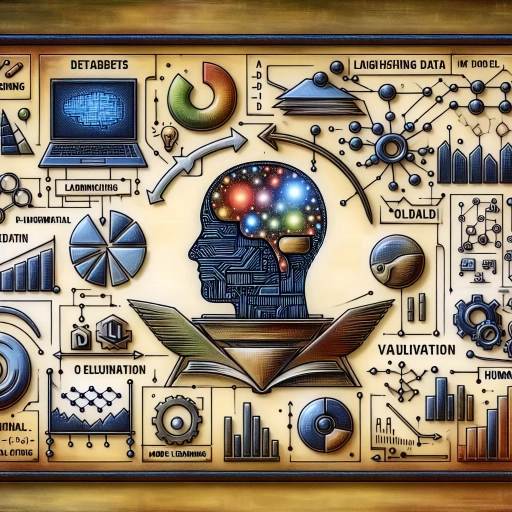
In the rapidly evolving landscape of technology, Machine Learning (ML) has emerged as a pivotal force, transforming how we interact with data and make decisions. At its core, ML is a subset of artificial intelligence that enables systems to learn from data without being explicitly programmed. This powerful technology has far-reaching implications across various sectors, from healthcare and finance to transportation and entertainment. To fully grasp the significance of ML, it is essential to delve into its fundamental principles, explore its diverse applications, and consider the future trends and challenges it presents. Understanding the basics of ML is crucial for appreciating its potential. This involves recognizing how algorithms are trained on datasets to predict outcomes or classify data points. By mastering these foundational concepts, one can better appreciate the myriad ways in which ML is applied in real-world scenarios. From predictive analytics in business to personalized recommendations in e-commerce, ML's applications are vast and varied. However, as ML continues to advance, it also faces significant challenges and raises important questions about ethics, privacy, and societal impact. In this article, we will first explore **Understanding the Basics of ML**, providing a comprehensive overview of the underlying mechanisms that drive this technology. We will then examine **Applications and Use Cases of ML**, highlighting how it is being utilized across different industries. Finally, we will discuss **Future Trends and Challenges in ML**, considering the potential developments and obstacles that lie ahead. By navigating these key aspects, readers will gain a holistic understanding of what ML means and its profound impact on our world. Let us begin by **Understanding the Basics of ML**.
Understanding the Basics of ML
In the rapidly evolving landscape of technology, Machine Learning (ML) has emerged as a cornerstone of innovation, transforming industries from healthcare to finance and beyond. To fully grasp the potential and applications of ML, it is essential to delve into its foundational elements. This article aims to provide a comprehensive overview of the basics of ML, starting with its **Definition and Origins**, which will explore the fundamental principles and historical roots that have shaped this field. We will then delve into **Key Concepts and Terminology**, breaking down the essential vocabulary and ideas that underpin ML, making it accessible to both beginners and seasoned practitioners. Finally, we will examine the **Historical Development** of ML, tracing its evolution from early theoretical frameworks to the sophisticated algorithms and models that drive modern applications. By understanding these core aspects, readers will gain a solid foundation in ML, enabling them to navigate its complexities and appreciate its transformative power. This journey through the basics of ML will equip you with the knowledge necessary to engage with this dynamic field and unlock its full potential. **Understanding the Basics of ML** is crucial for anyone looking to harness the power of artificial intelligence in today's digital age.
Definition and Origins
**Understanding the Basics of ML: Definition and Origins** Machine Learning (ML), a subset of Artificial Intelligence (AI), is defined as the scientific study of algorithms and statistical models that enable computers to perform tasks without explicit instructions. At its core, ML involves training machines to learn from data, identify patterns, and make predictions or decisions based on that data. This concept has its roots in the mid-20th century when computer scientists began exploring ways to create machines that could learn and improve their performance over time. The term "Machine Learning" was first coined by Arthur Samuel in 1959, an American computer scientist who developed one of the first computer games, a checkers program that could improve its play through experience. However, the foundational ideas date back to earlier work by Alan Turing, who in his 1950 paper "Computing Machinery and Intelligence," proposed a test to measure a machine's ability to exhibit intelligent behavior equivalent to, or indistinguishable from, that of a human. The origins of ML are deeply intertwined with the development of AI. In the 1950s and 1960s, researchers like Marvin Minsky and Seymour Papert explored neural networks, which are now a cornerstone of ML. Their work laid the groundwork for later advancements in deep learning techniques. The 1980s saw a resurgence in ML research with the introduction of decision trees and rule-based systems, which were more interpretable and easier to understand than earlier models. Throughout its evolution, ML has been influenced by various disciplines including statistics, computer science, and cognitive psychology. Today, ML is a ubiquitous technology used in a wide range of applications from image recognition and natural language processing to predictive analytics and autonomous vehicles. The ability of ML algorithms to learn from large datasets has revolutionized industries such as healthcare, finance, and marketing by enabling personalized recommendations, fraud detection, and disease diagnosis. In essence, understanding the basics of ML involves grasping how these algorithms learn from data and how they can be applied to solve complex problems. As technology continues to advance, the importance of ML in driving innovation and improving efficiency across various sectors will only continue to grow. By delving into its definition and origins, we gain a deeper appreciation for the historical context and scientific rigor that underpin this powerful technology.
Key Concepts and Terminology
Understanding the basics of Machine Learning (ML) requires a solid grasp of key concepts and terminology. At its core, ML is a subset of Artificial Intelligence (AI) that enables systems to learn from data without being explicitly programmed. **Supervised Learning**, **Unsupervised Learning**, and **Reinforcement Learning** are the primary types of ML. In **Supervised Learning**, the algorithm is trained on labeled data to predict outcomes for new, unseen data. For instance, a model might learn to classify images as either cats or dogs based on a dataset of labeled images. **Unsupervised Learning** involves training on unlabeled data to discover patterns or groupings within the data, such as clustering customers based on their buying behavior. **Reinforcement Learning** focuses on training agents to make decisions in complex environments by receiving rewards or penalties for their actions, akin to how a child learns to play a game through trial and error. **Features** and **Labels** are crucial terms in ML. **Features** refer to the input variables or characteristics of the data that the model uses to make predictions, while **Labels** are the target outputs or responses that the model aims to predict. For example, in predicting house prices, features might include square footage, number of bedrooms, and location, while the label would be the price of the house. **Overfitting** and **Underfitting** are common challenges in ML. **Overfitting** occurs when a model is too complex and learns the noise in the training data, resulting in poor performance on new data. Conversely, **Underfitting** happens when a model is too simple and fails to capture the underlying patterns in the data. Techniques like **Regularization**, **Cross-Validation**, and **Feature Engineering** help mitigate these issues. **Bias-Variance Tradeoff** is another important concept, where **Bias** refers to the error introduced by simplifying a model too much, and **Variance** refers to the error from overfitting. A good model balances these two to achieve optimal performance. **Neural Networks**, inspired by the human brain's structure, are powerful tools in ML. They consist of layers of interconnected nodes (neurons) that process inputs through complex transformations. **Deep Learning**, a subset of ML, involves neural networks with multiple layers, enabling the learning of abstract representations from raw data. Understanding these key concepts and terminology is essential for diving deeper into the world of Machine Learning. By grasping these fundamentals, you can better appreciate how ML models are built, trained, and optimized to solve real-world problems effectively. This foundational knowledge also helps in identifying the appropriate ML approach for different tasks and datasets, ensuring that you can leverage the full potential of Machine Learning in your projects.
Historical Development
The historical development of Machine Learning (ML) is a rich and evolving narrative that spans several decades, intertwining advancements in computer science, statistics, and artificial intelligence. The journey began in the mid-20th century when Alan Turing proposed the Turing Test in 1950, a benchmark for measuring a machine's ability to exhibit intelligent behavior equivalent to, or indistinguishable from, that of a human. This foundational idea sparked significant interest in creating machines that could learn and adapt. In the 1950s and 1960s, pioneers like Marvin Minsky and Seymour Papert explored neural networks, laying the groundwork for modern deep learning techniques. However, their work also highlighted the limitations of early neural networks, leading to a period known as the "AI winter" in the 1970s and 1980s, where funding and interest waned due to the lack of tangible progress. The resurgence of ML in the late 1980s and 1990s was driven by the development of decision trees, support vector machines, and other algorithms that could handle complex data sets more effectively. This period also saw the rise of Bayesian networks and probabilistic graphical models, which provided robust frameworks for reasoning under uncertainty. The 21st century marked a new era for ML with the advent of big data and powerful computing resources. The availability of vast amounts of data and advancements in hardware enabled the training of deep neural networks, leading to breakthroughs in image recognition, natural language processing, and speech recognition. Techniques such as convolutional neural networks (CNNs) and recurrent neural networks (RNNs) became staples in the field. Key milestones include the ImageNet Large Scale Visual Recognition Challenge (ILSVRC) in 2012, where AlexNet, a deep CNN, significantly outperformed other models, and the AlphaGo victory over a human world champion in Go in 2016, demonstrating the power of reinforcement learning. These achievements have propelled ML into mainstream applications across industries, from healthcare and finance to autonomous vehicles and customer service. Today, ML continues to evolve with ongoing research in areas like transfer learning, explainability, and ethical considerations. The integration of ML with other technologies such as the Internet of Things (IoT), blockchain, and edge computing is further expanding its potential. Understanding this historical context is crucial for appreciating the current state and future directions of ML, as it underscores the cumulative nature of scientific progress and the continuous innovation that drives this field forward.
Applications and Use Cases of ML
Machine learning (ML) has revolutionized various sectors by enabling systems to learn from data and make informed decisions without explicit programming. This transformative technology is now integral to numerous applications, each leveraging its unique capabilities to drive innovation and efficiency. In the industrial and commercial sphere, ML enhances operational processes, predicts market trends, and optimizes resource allocation. Within healthcare and medical research, ML aids in diagnosing diseases, personalizing treatment plans, and accelerating the discovery of new therapies. Additionally, in consumer technology and everyday life, ML powers intelligent assistants, recommends personalized content, and improves user experiences across multiple platforms. As we delve into these diverse use cases, it becomes clear that understanding the basics of ML is crucial for appreciating its full potential. By exploring these applications in depth, we can better grasp how ML is reshaping industries and our daily lives, making it essential to transition into understanding the foundational principles that underpin this powerful technology.
Industrial and Commercial Applications
Machine learning (ML) has revolutionized various industrial and commercial sectors by enhancing efficiency, accuracy, and decision-making processes. In the manufacturing industry, ML algorithms are used for predictive maintenance, where sensors and IoT devices monitor equipment health in real-time, predicting potential failures and scheduling maintenance before downtime occurs. This approach significantly reduces operational costs and improves overall plant reliability. Additionally, quality control is optimized through ML-driven inspection systems that can detect anomalies and defects more accurately than human inspectors, ensuring higher product quality. In the retail sector, ML powers personalized customer experiences through recommendation engines that analyze consumer behavior and preferences to suggest relevant products. This not only increases customer satisfaction but also boosts sales by encouraging purchases based on tailored suggestions. Inventory management is another area where ML excels; by analyzing historical sales data and seasonal trends, retailers can optimize stock levels, reduce overstocking, and prevent stockouts. The financial industry leverages ML for risk assessment and fraud detection. Advanced algorithms analyze vast amounts of transaction data to identify patterns indicative of fraudulent activity, enabling swift intervention and minimizing financial losses. Credit scoring models also benefit from ML, providing more accurate assessments of creditworthiness by considering a broader range of factors than traditional methods. Healthcare is another domain where ML has made significant strides. Diagnostic accuracy is improved through ML algorithms that analyze medical images such as X-rays and MRIs to detect conditions like cancer earlier and more reliably than human radiologists. Personalized medicine is also becoming more prevalent with ML, as it helps tailor treatment plans to individual patient profiles based on genetic data and medical histories. In logistics and supply chain management, ML optimizes route planning for delivery vehicles, reducing fuel consumption and lowering emissions while ensuring faster delivery times. Demand forecasting models predict future demand with high accuracy, allowing companies to adjust production levels accordingly and avoid supply chain disruptions. Furthermore, in the energy sector, ML is used to predict energy consumption patterns and optimize energy distribution networks. Smart grids equipped with ML capabilities can manage energy flow more efficiently, reducing waste and ensuring a stable supply of electricity. Overall, the integration of machine learning into industrial and commercial applications has transformed operational efficiency, customer engagement, and decision-making across various sectors. By automating complex tasks, enhancing predictive capabilities, and providing actionable insights from vast datasets, ML continues to drive innovation and growth in diverse industries.
Healthcare and Medical Research
In the realm of healthcare and medical research, machine learning (ML) has emerged as a transformative force, revolutionizing the way data is analyzed, insights are derived, and patient care is delivered. By leveraging ML algorithms, healthcare professionals can sift through vast amounts of complex data—ranging from electronic health records (EHRs) to genomic sequences and medical imaging—to uncover patterns and correlations that might elude human analysis. For instance, ML can be used to predict patient outcomes by analyzing historical data, enabling early intervention and personalized treatment plans. In medical research, ML accelerates the discovery of new treatments by identifying potential drug targets and predicting the efficacy of therapeutic interventions. Image recognition algorithms, powered by deep learning techniques, enhance diagnostic accuracy in radiology and pathology, allowing for quicker and more precise diagnoses of conditions such as cancer and cardiovascular diseases. Additionally, natural language processing (NLP) can extract valuable information from clinical notes and medical literature, facilitating evidence-based medicine and continuous learning. The integration of ML with wearable devices and IoT sensors also enables real-time monitoring of patient health, providing timely alerts for critical conditions and improving preventive care. Furthermore, ML-driven analytics help in optimizing hospital operations, streamlining resource allocation, and reducing healthcare costs without compromising quality. Overall, the applications of ML in healthcare and medical research are vast and multifaceted, promising to enhance patient care, accelerate scientific breakthroughs, and redefine the future of medicine. By harnessing the power of ML, we can move closer to achieving precision medicine, improving patient outcomes, and advancing our understanding of human health and disease.
Consumer Technology and Everyday Life
Consumer technology has revolutionized everyday life, seamlessly integrating into our daily routines and transforming the way we interact, work, and entertain ourselves. At the heart of this transformation is Machine Learning (ML), a subset of Artificial Intelligence (AI) that enables devices and systems to learn from data and improve their performance over time. In the realm of consumer technology, ML is ubiquitous, powering a wide array of applications that enhance user experience and efficiency. For instance, virtual assistants like Siri, Alexa, and Google Assistant leverage ML to understand voice commands, learn user preferences, and provide personalized recommendations. These assistants can manage schedules, control smart home devices, play music, and even assist with cooking recipes by analyzing vast amounts of data to predict and adapt to user behavior. Smartphones, another cornerstone of modern life, use ML for facial recognition in biometric security systems like Face ID and Google Face Unlock. Additionally, ML-driven algorithms optimize battery life by predicting usage patterns and adjusting power consumption accordingly. In the entertainment sector, streaming services such as Netflix and Spotify employ ML to recommend content based on viewing and listening history. These recommendations are not just random suggestions but are tailored to individual tastes through complex algorithms that analyze user behavior and preferences. Gaming consoles also benefit from ML, enhancing gameplay with adaptive difficulty levels and personalized game recommendations. Health and fitness tracking devices like smartwatches and fitness bands use ML to monitor vital signs such as heart rate and sleep patterns. These devices can detect anomalies in health data and alert users or healthcare providers if necessary. Furthermore, ML is integral in smart home automation systems where devices like thermostats (e.g., Nest) learn occupants' schedules and preferences to optimize heating and cooling settings for energy efficiency. The integration of ML into consumer technology has also significantly impacted retail and commerce. Online shopping platforms utilize ML for product recommendations, fraud detection, and personalized marketing campaigns. For example, Amazon's recommendation engine suggests products based on past purchases and browsing history, enhancing the shopping experience and driving sales. In summary, Machine Learning has become an indispensable component of consumer technology, enhancing various aspects of everyday life from home automation to entertainment and health monitoring. Its ability to learn from data and adapt to user behavior makes it a powerful tool for creating more intuitive, efficient, and personalized experiences. As ML continues to evolve, we can expect even more innovative applications that further integrate technology into our daily lives.
Future Trends and Challenges in ML
As we navigate the complexities of the 21st century, Machine Learning (ML) stands at the forefront of technological innovation, transforming industries and redefining the boundaries of what is possible. The future of ML is marked by several key trends and challenges that will shape its trajectory. On one hand, **Advancements in Deep Learning** are pushing the limits of artificial intelligence, enabling more sophisticated models that can tackle complex tasks with unprecedented accuracy. However, these advancements also raise critical **Ethical Considerations and Bias**, highlighting the need for careful oversight to ensure fairness and transparency in AI systems. Additionally, the **Integration with Other Technologies**, such as IoT, blockchain, and edge computing, promises to unlock new applications and efficiencies but also introduces new challenges in terms of interoperability and security. Understanding these trends is crucial for grasping the full potential of ML and its role in shaping our future. To fully appreciate these developments, it is essential to start by **Understanding the Basics of ML**, laying the groundwork for a deeper exploration of these emerging trends and challenges.
Advancements in Deep Learning
**Advancements in Deep Learning** Deep learning, a subset of machine learning (ML), has witnessed unprecedented advancements in recent years, transforming the landscape of artificial intelligence. At its core, deep learning leverages neural networks with multiple layers to learn complex patterns in data, mimicking the human brain's ability to process information. One of the most significant breakthroughs has been the development of convolutional neural networks (CNNs) for image recognition and natural language processing (NLP) models like transformers for text analysis. These models have achieved state-of-the-art performance in various tasks such as object detection, sentiment analysis, and machine translation. The advent of large-scale datasets and powerful computing resources, including graphics processing units (GPUs) and tensor processing units (TPUs), has been instrumental in driving these advancements. Techniques like transfer learning and fine-tuning pre-trained models have made it possible to adapt deep learning models to new tasks with minimal additional training data. Furthermore, advancements in reinforcement learning have enabled agents to learn from interactions with their environment, leading to significant improvements in areas such as game playing and autonomous driving. Another critical area of progress is explainability and interpretability in deep learning. As these models become increasingly complex, there is a growing need to understand how they make decisions. Techniques such as saliency maps and SHAP values are being developed to provide insights into the decision-making processes of deep neural networks. Additionally, there has been a surge in research on adversarial robustness, aiming to make deep learning models more resilient to attacks designed to mislead them. The integration of deep learning with other fields like healthcare and finance has also seen substantial growth. In healthcare, deep learning is being used for disease diagnosis from medical images and predicting patient outcomes. In finance, it is applied for risk assessment and fraud detection. However, these advancements come with challenges such as ethical considerations, data privacy concerns, and the need for more robust and transparent models. As we look to the future, the integration of deep learning with other AI disciplines like symbolic reasoning and edge AI is expected to further enhance its capabilities. The rise of edge AI will enable real-time processing on devices with limited computational resources, while symbolic reasoning will add a layer of interpretability and logic to deep learning models. Despite these promising trends, addressing issues such as bias in training data and ensuring ethical use will remain critical challenges in the field of deep learning. Overall, the rapid evolution of deep learning is poised to continue revolutionizing various industries and aspects of our lives, but it must be accompanied by careful consideration of its ethical and societal implications.
Ethical Considerations and Bias
As we delve into the future trends and challenges in Machine Learning (ML), it is imperative to address the ethical considerations and biases that underpin this rapidly evolving field. Ethical considerations in ML are multifaceted, encompassing issues such as data privacy, fairness, transparency, and accountability. One of the most pressing concerns is bias, which can manifest in various forms—algorithmic bias, data bias, and societal bias. Algorithmic bias arises when ML models are designed or trained in ways that inadvertently or intentionally discriminate against certain groups. For instance, facial recognition systems have been shown to perform less accurately on individuals with darker skin tones due to the lack of diverse training data. Data bias occurs when the datasets used to train models are skewed or incomplete, reflecting historical prejudices or societal inequalities. This can lead to models that perpetuate existing disparities, such as in hiring practices or loan approvals. Transparency and explainability are crucial in mitigating these biases. Techniques like model interpretability and feature attribution help in understanding how ML models arrive at their decisions, enabling the identification and rectification of biased outcomes. However, achieving full transparency is challenging due to the complexity of deep learning models and the proprietary nature of many algorithms. Accountability is another key ethical consideration; developers and deployers of ML systems must be held responsible for ensuring that their models do not harm individuals or communities. Moreover, ethical ML practices require a holistic approach that involves diverse stakeholders, including ethicists, policymakers, and end-users. This collaborative effort can help in developing guidelines and regulations that ensure ML systems are fair, equitable, and beneficial to society as a whole. For example, initiatives like the Fairness, Accountability, and Transparency (FAT) framework aim to provide a structured approach to evaluating and improving the ethical standards of ML models. Looking ahead, future trends in ML will likely involve more stringent ethical standards and regulatory frameworks. There will be an increased focus on developing explainable AI (XAI) and fair AI (FAI) methodologies to combat bias and ensure that ML systems are transparent and accountable. Additionally, advancements in areas like federated learning and differential privacy will help protect sensitive data while still allowing for the development of robust ML models. As ML continues to permeate various aspects of life, addressing ethical considerations and biases will be essential for harnessing its full potential while safeguarding societal values and individual rights. By prioritizing ethical ML practices, we can ensure that these powerful technologies serve humanity's best interests and contribute positively to our collective future.
Integration with Other Technologies
Integration with other technologies is a pivotal aspect of the future trends and challenges in Machine Learning (ML). As ML continues to evolve, its seamless integration with various technological domains will be crucial for unlocking its full potential. One key area of integration is with the Internet of Things (IoT), where ML algorithms can analyze vast amounts of data generated by IoT devices to enhance decision-making, improve efficiency, and enable predictive maintenance. For instance, in industrial settings, ML integrated with IoT sensors can predict equipment failures, reducing downtime and increasing overall productivity. Another significant integration is with cloud computing, which provides the scalable infrastructure needed to handle the computational demands of ML models. Cloud platforms offer robust services for data storage, processing, and deployment of ML models, making it easier for businesses to adopt and scale their ML initiatives. Additionally, the integration of ML with edge computing allows for real-time processing at the edge of the network, reducing latency and enhancing performance in applications such as autonomous vehicles and smart homes. The convergence of ML with blockchain technology also holds promise, particularly in ensuring data integrity and security. Blockchain's decentralized nature can provide a secure environment for data sharing and model training, addressing some of the privacy concerns associated with traditional centralized ML approaches. Furthermore, integrating ML with augmented reality (AR) and virtual reality (VR) can revolutionize industries like healthcare, education, and entertainment by creating immersive experiences that are personalized through ML-driven insights. Moreover, the integration of ML with natural language processing (NLP) has already led to significant advancements in chatbots, voice assistants, and text analysis tools. This synergy enables more sophisticated human-machine interactions, enhancing customer service and content generation capabilities. However, these integrations also present challenges such as ensuring data quality, managing complexity, and addressing ethical considerations like bias and transparency. In the realm of cybersecurity, integrating ML with traditional security measures can help detect and mitigate threats more effectively. ML algorithms can analyze network traffic patterns to identify anomalies that may indicate cyber attacks, thereby improving incident response times. However, this integration also raises concerns about adversarial attacks designed to deceive ML models, highlighting the need for robust security protocols. In conclusion, the integration of ML with other technologies is not only a trend but a necessity for driving innovation and solving complex problems across various sectors. While these integrations offer immense opportunities for growth and improvement, they also introduce new challenges that must be addressed through careful planning, ethical considerations, and continuous innovation. As ML continues to evolve, its ability to seamlessly integrate with other technologies will be a key determinant of its success in shaping the future.