What Is Generative Ai Vs Ai
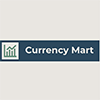
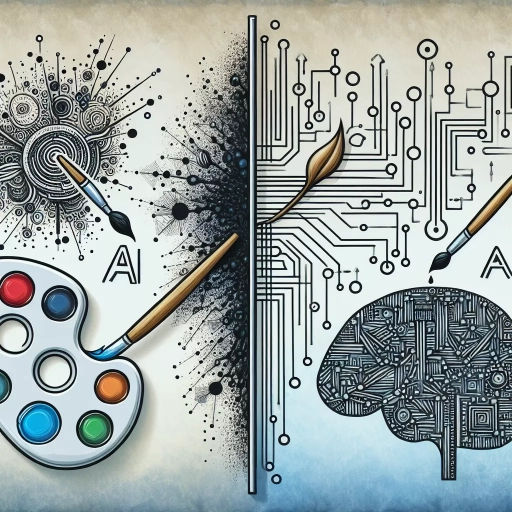
In the rapidly evolving landscape of artificial intelligence, two distinct branches have emerged: generative AI and traditional AI. While both types of AI are transformative in their own right, they serve different purposes and operate on different principles. Generative AI, with its ability to create new content such as images, text, and music, has captured the imagination of many due to its innovative applications. On the other hand, traditional AI focuses on solving specific problems through data analysis and decision-making processes. To fully appreciate the potential and limitations of these technologies, it is crucial to delve into their underlying mechanisms. This article will explore the intricacies of both generative AI and traditional AI, starting with an in-depth look at **Understanding Generative AI**, followed by **Understanding Traditional AI**, and culminating in **Comparing Generative AI and Traditional AI**. By examining these aspects, readers will gain a comprehensive understanding of how these technologies are reshaping various industries and our daily lives. Let us begin by **Understanding Generative AI**.
Understanding Generative AI
Understanding Generative AI is a multifaceted topic that delves into the heart of artificial intelligence's creative capabilities. At its core, generative AI involves algorithms that can generate new, synthetic data that resembles existing data. This field is underpinned by several key concepts and principles, which form the foundation of its functionality. To grasp the full scope of generative AI, it is essential to explore its definition and core principles, which will be discussed in detail in the following section. Beyond the foundational aspects, generative AI encompasses a variety of models, each with its unique strengths and applications. These models range from Generative Adversarial Networks (GANs) to Variational Autoencoders (VAEs), and understanding their differences is crucial for leveraging their potential effectively. The impact of generative AI extends far beyond the realm of technology itself, influencing various industries such as healthcare, finance, and entertainment. From generating realistic medical images for training purposes to creating personalized financial models, the applications are diverse and transformative. By delving into these three critical areas—definition and core principles, types of generative models, and applications in various industries—we can gain a comprehensive understanding of how generative AI works and its profound implications for our future. Let us begin by examining the definition and core principles that underpin this innovative technology.
Definition and Core Principles
**Understanding Generative AI: Definition and Core Principles** Generative AI, a subset of artificial intelligence, is defined by its ability to create new content that resembles existing data. This innovative technology leverages complex algorithms and machine learning models to generate outputs such as images, videos, music, text, and even entire datasets. At its core, generative AI operates on the principle of learning patterns and structures from large datasets and then using this knowledge to produce novel, coherent, and often indistinguishable content from real-world examples. The foundation of generative AI lies in deep learning techniques, particularly those involving neural networks. Two key architectures that underpin generative AI are Generative Adversarial Networks (GANs) and Variational Autoencoders (VAEs). GANs consist of two neural networks: a generator that creates new data samples and a discriminator that evaluates these samples against real data. Through an adversarial process, the generator improves its output quality until it can deceive the discriminator into believing the generated content is real. VAEs, on the other hand, use an encoder-decoder structure to learn a probabilistic representation of the input data, allowing for the generation of new samples by sampling from this learned distribution. Another crucial principle of generative AI is the concept of latent space representation. This involves mapping high-dimensional input data into a lower-dimensional latent space where meaningful patterns and relationships are preserved. By navigating this latent space, generative models can produce diverse outputs that capture the essence of the original dataset without simply replicating existing samples. Ethical considerations also play a significant role in the development and deployment of generative AI. As these models become increasingly sophisticated, they raise concerns about authenticity, privacy, and potential misuse. For instance, deepfakes—AI-generated videos or images that are nearly indistinguishable from real ones—pose significant ethical challenges regarding misinformation and identity theft. Therefore, researchers and developers must adhere to strict guidelines and regulations to ensure that generative AI is used responsibly. In summary, generative AI is built on advanced machine learning techniques that enable the creation of new content based on learned patterns from existing data. Its core principles include the use of GANs and VAEs, latent space representation, and a strong emphasis on ethical considerations. Understanding these principles is essential for harnessing the full potential of generative AI while mitigating its risks. As this technology continues to evolve, it holds promise for transformative applications across various fields such as art, entertainment, healthcare, and beyond.
Types of Generative Models
Generative models are a cornerstone of generative AI, enabling the creation of new, synthetic data that resembles existing data. These models can be broadly categorized into several types, each with its unique strengths and applications. **Generative Adversarial Networks (GANs)** are perhaps the most well-known type, consisting of two neural networks: a generator that produces synthetic data and a discriminator that evaluates the generated data's authenticity. This adversarial process refines the generator's output, leading to highly realistic results, particularly in image and video generation. **Variational Autoencoders (VAEs)** take a different approach by learning to compress and reconstruct data. They consist of an encoder that maps input data to a probabilistic latent space and a decoder that reconstructs the original data from this latent representation. VAEs are particularly useful for tasks like image compression and generation, as they provide a structured way to sample new data points. **Normalizing Flows** are another class of generative models that transform simple distributions into complex ones through a series of invertible transformations. This method allows for efficient sampling and density estimation, making it valuable for tasks requiring precise control over the generated data. **Transformers**, originally designed for natural language processing, have also been adapted for generative tasks. Models like **BERT** and **GPT** use transformer architectures to generate coherent text by predicting the next token in a sequence. These models have revolutionized text generation, enabling applications such as chatbots and content creation. **Diffusion Models** are a more recent innovation, which iteratively refine the input data by adding noise and then learning to reverse this process. This approach has shown remarkable results in image synthesis and denoising, offering a flexible framework for generating diverse and high-quality outputs. Each type of generative model has its own set of advantages and challenges. For instance, GANs can produce highly realistic images but often suffer from mode collapse and instability during training. VAEs offer more interpretable latent spaces but may lack the realism of GAN-generated data. Understanding these differences is crucial for selecting the appropriate model for specific applications, whether it be generating realistic images, synthesizing music, or creating engaging text content. In summary, the diversity of generative models allows researchers and practitioners to tackle a wide range of creative and analytical tasks. By leveraging the strengths of each model type—whether it's the realism of GANs, the interpretability of VAEs, or the versatility of transformers—generative AI can produce innovative solutions across various domains, from art and entertainment to science and technology. This versatility underscores the potential of generative AI to transform industries and push the boundaries of what is possible with artificial intelligence.
Applications in Various Industries
Generative AI, with its ability to create new content based on existing data, has revolutionized various industries by offering innovative solutions and enhancing operational efficiency. In the **healthcare sector**, generative AI is used to generate synthetic medical images for training models, reducing the need for real patient data and protecting privacy. It also aids in drug discovery by generating molecular structures that could have therapeutic properties, accelerating the development of new medicines. Additionally, generative models can predict patient outcomes and personalize treatment plans, leading to more effective care. In **finance**, generative AI helps in fraud detection by creating synthetic transaction data that mimics real-world scenarios, allowing systems to better identify anomalies. It also generates realistic financial scenarios for risk analysis and portfolio optimization, enabling more informed investment decisions. The technology is further used in customer service chatbots, creating personalized responses that simulate human-like interactions, thereby enhancing customer experience. The **entertainment industry** has seen a significant impact from generative AI. For instance, it is used in video game development to create dynamic environments and non-player characters (NPCs) that behave realistically. Music and video production also benefit from generative AI, which can generate original compositions or complete unfinished works based on the style of known artists. This technology has opened up new avenues for creativity and collaboration between humans and machines. In **manufacturing**, generative AI optimizes production processes by generating designs for products and components that meet specific criteria such as strength, weight, and cost. It also predicts maintenance needs through predictive analytics, reducing downtime and improving overall efficiency. The automotive industry, in particular, uses generative AI to design new vehicle models and simulate various driving conditions to test safety features. **Education** is another sector where generative AI makes a significant impact. It helps in creating personalized learning materials tailored to individual students' needs and learning styles. Generative models can also generate practice problems and assessments, freeing up instructors to focus on more critical aspects of teaching. In **marketing**, generative AI enhances customer engagement by creating personalized content such as emails, ads, and social media posts. It analyzes consumer behavior data to predict preferences and generate targeted campaigns that are more likely to resonate with the audience. Lastly, in **architecture and urban planning**, generative AI aids in designing buildings and urban spaces by generating multiple design options based on parameters like sustainability, aesthetics, and functionality. This allows architects to explore a wide range of possibilities efficiently. Overall, the applications of generative AI across these industries highlight its potential to drive innovation, improve efficiency, and enhance decision-making processes. As the technology continues to evolve, its impact is likely to be felt even more profoundly across various sectors.
Understanding Traditional AI
Understanding Traditional AI is a multifaceted topic that encompasses a rich history, sophisticated algorithms, and diverse applications. At its core, Traditional AI refers to the early approaches and methodologies developed in the field of artificial intelligence, which laid the groundwork for modern AI technologies. To fully grasp Traditional AI, it is essential to delve into its **Definition and Historical Context**, exploring how the concept of AI evolved over time and the key milestones that shaped its development. Additionally, examining **Key Algorithms and Techniques** such as rule-based systems, decision trees, and early machine learning models provides insight into the computational methods that underpin Traditional AI. Finally, understanding **Common Use Cases and Limitations** highlights where these traditional methods have been successfully applied and where they fall short, offering a balanced view of their utility and constraints. By exploring these aspects, we can gain a comprehensive understanding of Traditional AI and its enduring impact on the field. Let us begin by examining the **Definition and Historical Context** of Traditional AI.
Definition and Historical Context
**Definition and Historical Context** Understanding traditional AI begins with a clear definition and an appreciation of its historical context. Traditional AI, often referred to as "narrow" or "weak" AI, is designed to perform a specific task with precision and speed, leveraging algorithms and data to achieve this goal. Unlike the more recent advancements in generative AI, traditional AI systems are not capable of general intelligence or creativity; instead, they excel in well-defined domains such as image recognition, natural language processing, and decision-making within constrained parameters. Historically, the concept of AI dates back to the mid-20th century when computer scientists like Alan Turing, Marvin Minsky, and John McCarthy began exploring the possibility of creating machines that could think. The Dartmouth Summer Research Project on Artificial Intelligence in 1956 is often cited as the birthplace of AI as a field of research. Early efforts focused on developing rule-based systems and symbolic reasoning, leading to the development of expert systems in the 1970s and 1980s. These systems mimicked human decision-making processes by using predefined rules to solve problems within specific domains. The 1990s saw a resurgence in AI research with the advent of machine learning, particularly supervised learning techniques. This shift was driven by advances in computational power and the availability of large datasets. Machine learning algorithms enabled computers to learn from data without being explicitly programmed, leading to breakthroughs in areas such as speech recognition, text classification, and game playing. The success of IBM's Deep Blue in defeating chess champion Garry Kasparov in 1997 marked a significant milestone, demonstrating AI's capability to outperform humans in complex tasks. In the 21st century, traditional AI has continued to evolve with deep learning techniques becoming increasingly prevalent. Deep learning models, which use neural networks with multiple layers, have achieved state-of-the-art performance in various tasks such as image classification, object detection, and natural language processing. These models are trained on vast amounts of data and can learn complex patterns that were previously difficult for machines to discern. Despite its impressive capabilities, traditional AI operates within predefined boundaries and lacks the ability to generalize across different tasks or exhibit creativity. It is this limitation that distinguishes it from generative AI, which aims to create new content or solutions rather than simply processing existing information. Understanding the historical development and current state of traditional AI provides a foundational context for appreciating the innovations and challenges associated with generative AI. By recognizing how traditional AI has evolved over time, we can better grasp the transformative potential of generative AI and its implications for future technological advancements.
Key Algorithms and Techniques
Understanding traditional AI is deeply rooted in the mastery of key algorithms and techniques that have been the backbone of artificial intelligence for decades. At the heart of traditional AI lies a suite of algorithms designed to solve complex problems through logical reasoning, learning, and optimization. One of the foundational techniques is **Rule-Based Systems**, which rely on predefined rules to make decisions. These systems are particularly useful in expert systems where human knowledge is codified into rules that the system can execute. Another critical component is **Decision Trees**, which provide a structured approach to decision-making by breaking down complex problems into simpler, more manageable parts. Decision trees are widely used in classification tasks and are a precursor to more advanced machine learning models. **Neural Networks**, inspired by the human brain's structure, are another cornerstone of traditional AI. These networks consist of layers of interconnected nodes (neurons) that process inputs and produce outputs, enabling tasks such as pattern recognition and prediction. **Genetic Algorithms** draw inspiration from natural selection and genetics, using principles like mutation, crossover, and selection to search for optimal solutions in large solution spaces. This evolutionary approach is particularly effective in solving optimization problems where traditional methods may fail. **Support Vector Machines (SVMs)** are powerful tools for classification and regression tasks, leveraging the concept of hyperplanes to find the best boundary between different classes. SVMs are known for their robustness and ability to handle high-dimensional data. **K-Means Clustering** is a popular unsupervised learning algorithm used for grouping similar data points into clusters based on their features. This technique is essential in exploratory data analysis and customer segmentation. **Gradient Descent**, a fundamental optimization algorithm, is used to minimize the cost function in many machine learning models. It iteratively adjusts parameters to find the optimal solution by moving in the direction of the negative gradient. **Dynamic Programming** solves complex problems by breaking them down into simpler subproblems, storing the solutions to these subproblems to avoid redundant computation. This technique is crucial in solving problems like the shortest path in a graph or the longest common subsequence. **Heuristics** and **Metaheuristics** play a significant role in traditional AI by providing approximate solutions to problems that are too complex to solve exactly within a reasonable time frame. Heuristics use domain-specific knowledge to guide the search towards promising areas, while metaheuristics employ higher-level strategies to explore the solution space efficiently. These algorithms and techniques collectively form the bedrock upon which traditional AI stands. They have been refined over years of research and application, enabling AI systems to perform a wide range of tasks from simple automation to sophisticated decision-making. Understanding these foundational elements is essential for appreciating the evolution of AI and the emergence of more advanced forms like generative AI. By mastering these key algorithms and techniques, developers can build robust, efficient, and intelligent systems that solve real-world problems effectively.
Common Use Cases and Limitations
Understanding traditional AI involves delving into its common use cases and limitations, which are crucial for appreciating the advancements and challenges in the field. Traditional AI, often referred to as narrow or weak AI, is designed to perform specific tasks with high efficiency. One of the most prevalent use cases is **predictive analytics**, where AI algorithms analyze historical data to forecast future trends and outcomes. For instance, in finance, AI models predict stock prices, credit scores, and risk assessments, enabling more informed decision-making. Another significant application is **natural language processing (NLP)**, which powers chatbots, sentiment analysis tools, and language translation software. These NLP applications enhance customer service, improve text analysis, and facilitate cross-lingual communication. **Image recognition** is another key area where traditional AI excels. Computer vision algorithms are used in self-driving cars to detect objects, in medical imaging to diagnose diseases, and in surveillance systems to identify individuals. Additionally, **recommendation systems** are widely used in e-commerce platforms to suggest products based on user behavior and preferences, thereby enhancing user experience and driving sales. However, traditional AI also has several limitations. One major constraint is its **narrow scope**; these systems are designed to perform a single task and lack the ability to generalize across different domains. For example, a chess-playing AI cannot suddenly start recognizing images or translating languages without significant retraining. Another limitation is **data dependency**; traditional AI models require large amounts of high-quality data to learn and perform effectively. This can be a challenge in domains where data is scarce or noisy. Moreover, traditional AI often struggles with **explainability**; many models operate as black boxes, making it difficult to understand the reasoning behind their decisions. This lack of transparency can be problematic in critical applications such as healthcare and finance, where understanding the decision-making process is essential for trust and accountability. Finally, traditional AI is vulnerable to **adversarial attacks**, which are designed to mislead the model into making incorrect predictions. These attacks highlight the need for robust security measures to ensure the reliability of AI systems in real-world scenarios. In summary, while traditional AI has numerous practical applications across various industries, its limitations underscore the need for ongoing research and development. As we transition towards more advanced forms of AI like generative AI, understanding these limitations will be crucial for leveraging the strengths of each type of AI effectively. By recognizing both the capabilities and constraints of traditional AI, we can better navigate the evolving landscape of artificial intelligence and harness its full potential.
Comparing Generative AI and Traditional AI
The advent of generative AI has marked a significant shift in the landscape of artificial intelligence, prompting a critical comparison with traditional AI. This article delves into the distinctions and potential synergies between these two AI paradigms, exploring three key aspects: differences in functionality and purpose, performance metrics and evaluation criteria, and future implications and potential overlaps. Generative AI, characterized by its ability to create new content such as text, images, and music, stands in stark contrast to traditional AI, which is often focused on solving specific problems through rule-based systems or machine learning algorithms. Understanding these differences is crucial for evaluating their respective strengths and weaknesses. The performance metrics used to assess these AI types also vary, reflecting their unique objectives and operational contexts. Finally, considering the future implications and potential overlaps between generative and traditional AI can provide insights into how these technologies might converge or diverge in the coming years. By examining these facets, we can better appreciate the diverse roles that different AI approaches play in modern technology. Let us begin by exploring the differences in functionality and purpose that underpin these distinct AI methodologies.
Differences in Functionality and Purpose
When comparing generative AI and traditional AI, one of the most significant distinctions lies in their functionality and purpose. Traditional AI, often referred to as discriminative AI, is designed to recognize patterns and make predictions based on existing data. It excels in tasks such as image classification, natural language processing for sentiment analysis, and predictive analytics. For instance, traditional AI can be used to identify spam emails, classify medical images, or forecast sales trends. The primary goal of traditional AI is to optimize performance on a specific task by learning from labeled data and minimizing errors. In contrast, generative AI is focused on creating new content that resembles existing data. This type of AI leverages models like Generative Adversarial Networks (GANs) and Variational Autoencoders (VAEs) to generate novel outputs such as images, videos, music, or text. The purpose of generative AI is not just to recognize patterns but to produce original content that could pass as real. For example, generative AI can create realistic portraits of people who do not exist, generate coherent text based on a prompt, or even compose music in the style of a particular artist. This capability opens up new avenues for creative applications, data augmentation, and even synthetic data generation for training other AI models. Another key difference is the level of creativity and autonomy involved. Traditional AI operates within well-defined boundaries and follows strict rules to achieve its objectives. It is highly efficient but lacks the ability to innovate beyond its training data. On the other hand, generative AI introduces an element of randomness and exploration, allowing it to produce unique outcomes that may not have been seen before. This makes generative AI particularly useful in fields like art, design, and research where innovation is crucial. However, this increased creativity comes with its own set of challenges. Generative models often require vast amounts of data and computational resources to train effectively. Additionally, ensuring the quality and relevance of generated content can be more complex compared to traditional AI tasks. There is also a growing concern about the ethical implications of generative AI, such as the potential for deepfakes or misinformation. In summary, while traditional AI excels at recognizing patterns and making predictions within predefined constraints, generative AI is adept at creating new content that mirrors real-world data. The functionality and purpose of these two types of AI are distinct yet complementary, each offering unique advantages depending on the application. Understanding these differences is essential for leveraging the full potential of AI technologies in various fields.
Performance Metrics and Evaluation Criteria
When comparing generative AI and traditional AI, a crucial aspect to consider is the performance metrics and evaluation criteria used to assess their effectiveness. Performance metrics serve as the benchmarks against which the success of AI models is measured, and they vary significantly between generative and traditional AI due to their distinct objectives. For **traditional AI**, which often focuses on tasks like classification, regression, and decision-making, common performance metrics include accuracy, precision, recall, F1-score, mean squared error (MSE), and mean absolute error (MAE). These metrics are quantifiable and straightforward, allowing for clear comparisons between different models. For instance, in a classification task, accuracy measures the proportion of correctly classified instances out of total instances. In predictive modeling, MSE and MAE evaluate how close the model's predictions are to the actual values. These metrics are well-established and widely understood, making it easier to evaluate and compare traditional AI models. In contrast, **generative AI**, which involves creating new content such as images, text, or music, requires more nuanced evaluation criteria. Here, metrics like perplexity, inception score, and Frechet inception distance (FID) are commonly used. Perplexity measures how well a model predicts a sample from a test set; lower perplexity indicates better performance. The inception score evaluates the quality and diversity of generated samples by comparing them against a pre-trained classifier. FID assesses the similarity between generated samples and real-world data by comparing their feature distributions. Additionally, human evaluation plays a significant role in assessing generative AI outputs because subjective qualities like coherence, creativity, and realism are crucial but harder to quantify. Another key difference lies in the evaluation process itself. Traditional AI models are often evaluated using standardized datasets and well-defined evaluation protocols, ensuring consistency and reproducibility. Generative AI models, however, may require more customized evaluation approaches due to the variability in output types and the subjective nature of quality assessment. This can involve user studies where human evaluators rate the generated content based on various criteria such as aesthetic appeal or functional utility. Moreover, generative AI models may also be evaluated based on their ability to generalize across different contexts or domains. Metrics such as domain adaptation accuracy or transfer learning performance can provide insights into how well these models adapt to new scenarios without extensive retraining. In summary, while traditional AI relies heavily on quantifiable metrics that measure predictive accuracy or classification performance, generative AI necessitates a broader range of evaluation criteria that include both quantitative and qualitative assessments. Understanding these differences in performance metrics and evaluation criteria is essential for comparing the strengths and weaknesses of generative AI versus traditional AI effectively. By recognizing these distinctions, researchers and practitioners can better align their evaluation methods with the specific goals and challenges of each AI paradigm.
Future Implications and Potential Overlaps
As we delve into the future implications of generative AI and traditional AI, it becomes increasingly evident that their paths are likely to intersect and influence each other in profound ways. Generative AI, with its ability to create new content such as images, text, and music, is poised to revolutionize industries like entertainment, education, and healthcare. For instance, generative models could produce personalized educational materials tailored to individual learning styles, or generate realistic patient data for medical research without compromising privacy. However, these advancements also raise ethical concerns regarding authenticity and accountability, particularly in domains like journalism and legal documentation. On the other hand, traditional AI excels in predictive analytics and decision-making processes, driving efficiencies in sectors such as finance, logistics, and manufacturing. Traditional AI systems can optimize supply chains, predict market trends, and automate repetitive tasks with high accuracy. Yet, their reliance on historical data limits their ability to innovate beyond established patterns. The potential overlaps between these two AI paradigms are vast and promising. For example, integrating generative models with traditional AI could enable the creation of more robust predictive systems. Generative AI could generate synthetic data to augment sparse datasets, thereby improving the accuracy of traditional AI models. Conversely, traditional AI's analytical capabilities could help refine and validate the outputs of generative models, ensuring they align with real-world constraints and objectives. Moreover, as AI continues to evolve, we may see the emergence of hybrid models that leverage both generative and traditional AI strengths. These hybrids could tackle complex problems that require both creativity and precision—such as developing new drug compounds or designing sustainable urban infrastructure. The synergy between these approaches will be crucial in addressing some of humanity's most pressing challenges while mitigating risks associated with unchecked technological advancement. In conclusion, the future of AI is likely to be characterized by a dynamic interplay between generative and traditional AI. As these technologies converge, they will not only enhance each other but also redefine the boundaries of what is possible in various fields. Understanding these implications and potential overlaps is essential for harnessing the full potential of AI while ensuring its development aligns with societal values and ethical standards. By fostering collaboration between these two AI paradigms, we can unlock new frontiers of innovation and progress.