What Is Asr
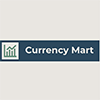
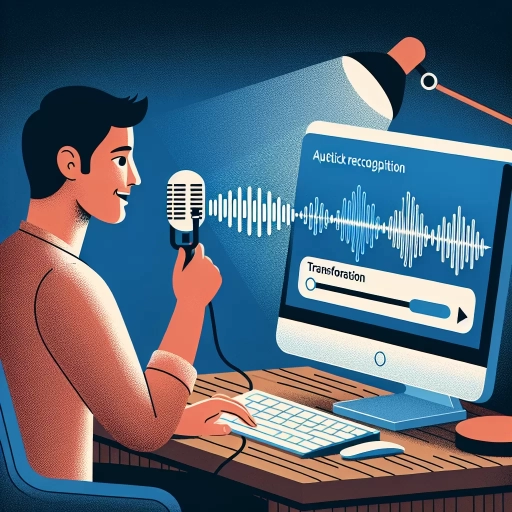
In the rapidly evolving landscape of technology, Automatic Speech Recognition (ASR) has emerged as a transformative force, revolutionizing how we interact with machines and access information. ASR, the technology that enables computers to understand and interpret human speech, is no longer a futuristic concept but a ubiquitous tool in our daily lives. This article delves into the intricacies of ASR, starting with **Understanding ASR: Definition and Basics**, where we explore the fundamental principles and definitions that underpin this technology. We will then dive into **How ASR Works: Technical Insights**, uncovering the complex algorithms and technical processes that make speech recognition possible. Finally, we will examine **Applications and Impact of ASR**, highlighting its diverse uses and the significant impact it has on various industries and aspects of society. By understanding these core aspects, readers will gain a comprehensive insight into the world of ASR and its profound implications. Let us begin by laying the groundwork with **Understanding ASR: Definition and Basics**.
Understanding ASR: Definition and Basics
Understanding Automatic Speech Recognition (ASR) is a multifaceted topic that encompasses various aspects, from its historical development to the key components and types of technologies involved. At its core, ASR is a technology that enables machines to interpret and transcribe spoken language into text. To fully grasp the intricacies of ASR, it is essential to delve into its historical development, which spans several decades and has seen significant advancements driven by innovations in signal processing, machine learning, and computational power. This journey highlights the evolution from early rule-based systems to modern deep learning models. Additionally, understanding the key components of ASR systems, such as acoustic models, language models, and decoding algorithms, provides insight into how these technologies work together to achieve accurate speech recognition. Finally, exploring the different types of ASR technologies, including hybrid models and end-to-end neural networks, reveals the diverse approaches being used to improve performance and adaptability. By examining these facets, we can appreciate the complexity and sophistication of ASR. Let us begin by tracing the historical development of ASR, which lays the foundation for understanding its current state and future potential.
Historical Development of ASR
The historical development of Automatic Speech Recognition (ASR) is a narrative of continuous innovation and technological advancement, spanning several decades. The journey began in the 1950s with the first attempts at speech recognition, led by researchers like Alan Newell and his team at the RAND Corporation, who developed a system capable of recognizing digits spoken by a single speaker. This early work laid the foundation for future research. In the 1960s and 1970s, ASR technology evolved with the introduction of dynamic time warping (DTW) algorithms, which allowed for more robust recognition by aligning spoken words with pre-recorded templates. This period also saw the development of the first commercial ASR systems, such as IBM's Shoebox machine, which could recognize a limited set of words. The 1980s marked a significant leap forward with the advent of Hidden Markov Models (HMMs), which revolutionized ASR by modeling speech as a sequence of states. This approach enabled more accurate and flexible recognition systems. The introduction of Gaussian Mixture Models (GMMs) further enhanced performance by better capturing the variability in speech. The 1990s and early 2000s witnessed the rise of large vocabulary continuous speech recognition (LVCSR) systems, capable of recognizing thousands of words in continuous speech. This was facilitated by advancements in machine learning, particularly with the use of neural networks. The development of deep learning techniques, such as Recurrent Neural Networks (RNNs) and Long Short-Term Memory (LSTM) networks, significantly improved ASR accuracy. In recent years, the integration of deep learning architectures like Convolutional Neural Networks (CNNs) and Transformers has further elevated ASR performance. These models leverage large datasets and computational power to achieve state-of-the-art results. The widespread adoption of cloud computing and the availability of open-source frameworks like Kaldi and TensorFlow have democratized access to ASR technology, enabling its integration into various applications such as virtual assistants, voice-controlled devices, and transcription services. Throughout its development, ASR has been driven by interdisciplinary research involving linguistics, signal processing, and machine learning. Today, ASR is a cornerstone of modern technology, transforming how humans interact with machines and facilitating numerous applications across industries. Despite its advancements, ongoing research continues to address challenges such as handling noisy environments, recognizing diverse accents, and improving real-time processing capabilities. The historical development of ASR underscores the relentless pursuit of innovation in speech technology, paving the way for even more sophisticated and ubiquitous applications in the future.
Key Components of ASR Systems
**Key Components of ASR Systems** Automatic Speech Recognition (ASR) systems are complex technologies that rely on several key components to accurately transcribe spoken language into text. At the core of any ASR system are **Speech Signal Processing**, **Feature Extraction**, **Acoustic Modeling**, **Language Modeling**, and **Decoding**. 1. **Speech Signal Processing**: This initial step involves capturing and preprocessing the audio signal to enhance its quality. Techniques such as noise reduction, echo cancellation, and normalization are applied to ensure the signal is clear and consistent. 2. **Feature Extraction**: Following preprocessing, the audio signal is converted into a set of features that can be analyzed by the system. Common features include Mel-Frequency Cepstral Coefficients (MFCCs) and spectrograms, which capture the spectral characteristics of speech. 3. **Acoustic Modeling**: This component involves training machine learning models to recognize patterns in speech sounds. Acoustic models, typically deep neural networks, learn to map the extracted features to phonemes or sub-phonemes, which are the basic units of sound in spoken language. 4. **Language Modeling**: While acoustic models focus on the sound patterns, language models predict the likelihood of a sequence of words occurring in a language. These models, often based on statistical methods or recurrent neural networks (RNNs), help the ASR system choose the most plausible transcription based on linguistic context. 5. **Decoding**: The final step integrates the outputs from both acoustic and language models to generate the most likely transcription. Decoding algorithms, such as beam search or dynamic programming, balance the probabilities from both models to produce an accurate text representation of the spoken input. Additionally, **Adaptation Techniques** play a crucial role in improving ASR performance. These techniques allow the system to adapt to different speakers, accents, and environments, enhancing its robustness and accuracy. **Speaker Adaptation** adjusts the acoustic model to better match the characteristics of a specific speaker, while **Environment Adaptation** compensates for variations in background noise and recording conditions. In summary, the synergy between these components—speech signal processing, feature extraction, acoustic modeling, language modeling, decoding, and adaptation techniques—enables ASR systems to achieve high levels of accuracy and reliability in transcribing spoken language into text. Understanding these key components is essential for appreciating the complexity and sophistication of ASR technology.
Types of ASR Technologies
**Types of ASR Technologies** Automatic Speech Recognition (ASR) technologies have evolved significantly, offering various approaches to transcribe spoken language into text. These technologies can be broadly categorized based on their underlying methodologies and applications. 1. **Rule-Based Systems**: These early ASR systems relied on predefined rules and phonetic transcriptions. They were limited by their inability to adapt to new speakers or environments, making them less accurate compared to modern methods. 2. **Statistical Models**: This category includes Hidden Markov Models (HMMs) and Gaussian Mixture Models (GMMs). HMMs model the temporal structure of speech, while GMMs represent the distribution of acoustic features. These models were widely used but have been largely superseded by more advanced techniques. 3. **Deep Learning Models**: The advent of deep learning has revolutionized ASR. Techniques such as Convolutional Neural Networks (CNNs), Recurrent Neural Networks (RNNs), and Long Short-Term Memory (LSTM) networks have significantly improved accuracy. These models can learn complex patterns in speech data, making them highly effective for real-world applications. 4. **End-to-End Models**: These models integrate the entire ASR pipeline into a single neural network, eliminating the need for separate components like acoustic modeling and language modeling. Examples include Connectionist Temporal Classification (CTC) and sequence-to-sequence models with attention mechanisms. End-to-end models have shown remarkable performance and are increasingly popular. 5. **Hybrid Models**: Some ASR systems combine different approaches to leverage their strengths. For instance, a hybrid model might use a deep learning-based acoustic model with a traditional HMM for decoding. This combination can offer robustness and flexibility. 6. **Cloud-Based ASR**: Cloud-based solutions utilize remote servers to process speech data, offering advantages such as scalability, continuous updates, and access to powerful computational resources. Companies like Google, Amazon, and Microsoft provide cloud-based ASR services that are widely used in various applications. 7. **On-Device ASR**: In contrast to cloud-based solutions, on-device ASR processes speech locally on the user's device. This approach is beneficial for real-time applications requiring low latency and privacy-sensitive scenarios where data cannot be sent to the cloud. 8. **Specialized ASR**: There are also specialized ASR technologies tailored for specific use cases, such as medical transcription, legal transcription, or voice assistants in smart home devices. These systems are often trained on domain-specific data to achieve higher accuracy within their respective fields. Each type of ASR technology has its own set of advantages and challenges, making them suitable for different applications and environments. Understanding these types is crucial for selecting the most appropriate technology for a given task, ensuring optimal performance and user experience.
How ASR Works: Technical Insights
Automatic Speech Recognition (ASR) is a sophisticated technology that converts spoken language into text, leveraging a combination of advanced technical insights. At its core, ASR relies on three key components: Speech Signal Processing, Machine Learning Algorithms, and Acoustic and Language Models. Speech Signal Processing is the initial step where raw audio data is cleaned, filtered, and transformed into a format that can be analyzed. This process sets the stage for the application of Machine Learning Algorithms, which are trained to recognize patterns in speech and translate them into meaningful text. Additionally, Acoustic and Language Models play a crucial role in refining the accuracy of ASR by understanding the nuances of human speech and the context in which words are used. By integrating these elements, ASR systems can achieve high levels of precision and usability. To delve deeper into the intricacies of ASR, we begin with the foundational aspect of Speech Signal Processing, which lays the groundwork for the entire recognition process.
Speech Signal Processing
Speech Signal Processing is a critical component in the functioning of Automatic Speech Recognition (ASR) systems. It involves the analysis, manipulation, and enhancement of speech signals to improve their quality and extract meaningful information. Here’s how it works: 1. **Signal Acquisition**: The process begins with capturing the speech signal through a microphone or other recording device. This raw signal is then digitized, converting it into a digital format that can be processed by computers. 2. **Preprocessing**: The digitized signal undergoes preprocessing to remove noise and other unwanted components. Techniques such as noise reduction, echo cancellation, and normalization are applied to enhance the signal-to-noise ratio (SNR) and ensure consistency across different recording conditions. 3. **Feature Extraction**: This step involves extracting relevant features from the preprocessed signal that are useful for speech recognition. Common features include Mel-Frequency Cepstral Coefficients (MFCCs), which capture the spectral characteristics of speech, and other acoustic features like pitch and energy. 4. **Model Training**: These extracted features are then used to train machine learning models, typically Hidden Markov Models (HMMs) or more recently, deep neural networks (DNNs). These models learn to map acoustic features to phonemes or words based on large datasets of labeled speech. 5. **Decoding**: During the recognition phase, the trained model processes the input speech signal in real-time. It uses the extracted features to predict the most likely sequence of words or phonemes that correspond to the spoken input. This prediction is often done using algorithms like Viterbi decoding for HMMs or beam search for DNNs. 6. **Post-processing**: Finally, post-processing techniques are applied to refine the recognized text. This may include spell correction, grammar checking, and contextual understanding to improve the accuracy and coherence of the output. In summary, Speech Signal Processing is essential for ASR as it transforms raw audio into a format that can be understood by machines, enabling accurate speech recognition and transcription. By carefully managing each stage from acquisition to post-processing, ASR systems can achieve high levels of accuracy and reliability in various applications such as voice assistants, transcription services, and voice-controlled interfaces.
Machine Learning Algorithms in ASR
Machine learning algorithms are the backbone of Automatic Speech Recognition (ASR) systems, enabling them to accurately transcribe spoken language into text. These algorithms leverage vast amounts of data to learn patterns and relationships within speech, allowing ASR systems to improve over time. Here’s a detailed look at how these algorithms work: 1. **Data Collection and Preprocessing**: The process begins with collecting large datasets of speech recordings paired with their corresponding transcripts. These datasets are then preprocessed to enhance audio quality, normalize volumes, and remove noise. 2. **Feature Extraction**: Machine learning models require numerical representations of speech data. Techniques such as Mel-Frequency Cepstral Coefficients (MFCCs) or spectrograms are used to extract features from audio signals that capture the essence of speech patterns. 3. **Model Training**: The extracted features are fed into machine learning models for training. Common models include Hidden Markov Models (HMMs), Deep Neural Networks (DNNs), and more recently, Recurrent Neural Networks (RNNs) and their variants like Long Short-Term Memory (LSTM) networks. These models learn to map acoustic features to phonetic units or words. 4. **Acoustic Modeling**: Acoustic models predict the probability of observing a sequence of acoustic features given a sequence of phonemes or words. DNNs have significantly improved the accuracy of ASR by learning complex representations of speech. 5. **Language Modeling**: Language models predict the likelihood of a word sequence in a language, helping to disambiguate words with similar pronunciations. N-gram models and more advanced models like Recurrent Neural Network Language Models (RNNLMs) are used. 6. **Decoding**: During the recognition phase, the ASR system uses both acoustic and language models to decode the input speech into text. Beam search algorithms are often employed to find the most likely word sequence given the input audio. 7. **Post-processing**: Finally, post-processing techniques such as spell correction and fluency evaluation can be applied to refine the output transcript. In summary, machine learning algorithms in ASR transform raw audio data into meaningful text through a series of sophisticated steps involving data preprocessing, feature extraction, model training, and decoding. These algorithms continuously evolve with advancements in deep learning, leading to more accurate and robust ASR systems.
Acoustic and Language Models
In the realm of Automatic Speech Recognition (ASR), two critical components are the Acoustic Model and the Language Model. The **Acoustic Model** is responsible for mapping audio signals to phonemes or sub-phonetic units. This model is typically trained using machine learning algorithms, such as Hidden Markov Models (HMMs) or more recently, Deep Neural Networks (DNNs). The acoustic model learns to recognize patterns in speech sounds by analyzing the acoustic features extracted from audio data. These features often include Mel-Frequency Cepstral Coefficients (MFCCs) or spectrograms, which capture the spectral characteristics of speech. By training on large datasets, the acoustic model becomes adept at distinguishing between different phonemes, even in the presence of noise or variations in speaker voices. The **Language Model**, on the other hand, predicts the likelihood of a sequence of words in a given language. It is essential for disambiguating words that sound similar but have different meanings and for ensuring that the recognized speech adheres to grammatical and semantic rules. Language models can be statistical, such as n-gram models, or more advanced neural network-based models like Recurrent Neural Networks (RNNs) and Transformers. These models are trained on vast amounts of text data to learn the probabilities of word sequences, enabling them to suggest the most likely word or phrase given the context. In ASR systems, the language model works in tandem with the acoustic model to refine the transcription output by favoring word sequences that are more probable in the language. Together, these models form a powerful synergy that enhances the accuracy and robustness of ASR systems. During speech recognition, the acoustic model generates a set of possible phonetic transcriptions, which are then evaluated by the language model to determine the most plausible sequence of words. This integrated approach ensures that ASR systems can handle complex speech patterns, dialects, and even out-of-vocabulary words effectively. The continuous advancements in both acoustic and language modeling have significantly improved the performance of ASR systems, making them indispensable in applications ranging from virtual assistants and voice-controlled devices to transcription services and accessibility tools.
Applications and Impact of ASR
The advent of Automatic Speech Recognition (ASR) technology has revolutionized various aspects of modern life, transforming the way we interact with devices and access information. This article delves into the multifaceted applications and profound impact of ASR, exploring its real-world use cases, economic and social implications, and future trends and innovations. From voice assistants in smart homes to transcription services in healthcare and education, ASR has become an indispensable tool in everyday life. The economic and social implications of ASR are equally significant, as it enhances productivity, improves accessibility, and opens up new job opportunities. Looking ahead, advancements in machine learning and artificial intelligence are expected to further refine ASR capabilities, leading to even more innovative applications. To understand the full scope of ASR's influence, we begin by examining its real-world use cases, where the technology is already making a tangible difference in various industries and daily routines.
Real-World Use Cases for ASR
Automatic Speech Recognition (ASR) has revolutionized various industries with its real-world applications, significantly enhancing efficiency, accessibility, and user experience. In **customer service**, ASR powers voice assistants and chatbots, enabling 24/7 support without human intervention. For instance, companies like Amazon and Google use ASR to handle customer inquiries through their virtual assistants, providing instant responses and reducing wait times. In **healthcare**, ASR facilitates the transcription of medical dictations, allowing doctors to focus more on patient care rather than paperwork. This technology also aids in creating detailed patient records quickly and accurately, improving data integrity and reducing the risk of errors. Additionally, ASR helps in remote health monitoring by enabling patients to communicate their symptoms and medical history more easily. The **education sector** benefits from ASR through tools that help students with disabilities. For example, speech-to-text software assists students with dyslexia or other learning disabilities by converting spoken words into written text, making it easier for them to complete assignments and participate in class discussions. In **transportation**, ASR is integrated into vehicle systems to enhance driver safety and convenience. Voice-controlled navigation systems, such as those found in cars equipped with Apple CarPlay or Android Auto, allow drivers to set destinations, make calls, and send messages without taking their hands off the wheel. The **entertainment industry** also leverages ASR to improve user interaction with smart TVs and streaming services. Voice commands enable users to search for content, change channels, and adjust settings seamlessly, enhancing the overall viewing experience. Furthermore, **law enforcement** and **legal services** use ASR for transcribing interviews, interrogations, and court proceedings. This helps in creating accurate records quickly, which can be crucial for legal cases. In **retail**, ASR is used in voice-activated shopping assistants that help customers find products, check prices, and complete purchases using voice commands alone. This technology also aids in inventory management by allowing warehouse staff to update stock levels and track items using voice inputs. Lastly, **smart home devices** rely heavily on ASR to control lighting, temperature, security systems, and other appliances with voice commands. This not only adds convenience but also makes these systems more accessible for people with mobility issues. Overall, the widespread adoption of ASR across these sectors underscores its transformative potential in enhancing productivity, accessibility, and user satisfaction.
Economic and Social Implications
The economic and social implications of Automatic Speech Recognition (ASR) are multifaceted and profound, influencing various sectors and aspects of daily life. Economically, ASR technology has the potential to significantly enhance operational efficiency and reduce costs in industries such as customer service, healthcare, and finance. For instance, automated call centers can handle a higher volume of customer inquiries without the need for human operators, leading to cost savings and improved response times. In healthcare, ASR can streamline clinical documentation processes, allowing medical professionals to focus more on patient care rather than administrative tasks. This not only improves the quality of care but also reduces the financial burden associated with manual data entry. From a social perspective, ASR has the power to bridge communication gaps and enhance inclusivity. For individuals with disabilities, such as those who are deaf or hard of hearing, ASR can provide real-time transcription services that facilitate better communication in educational settings, workplaces, and social interactions. Additionally, ASR can support language learning by offering instant feedback on pronunciation and grammar, making language acquisition more accessible and effective. The technology also plays a crucial role in emergency services by enabling quick and accurate transcription of emergency calls, which can be critical in life-threatening situations. Moreover, ASR is transforming the way we interact with technology in our daily lives. Smart home devices and virtual assistants like Alexa and Google Assistant rely on ASR to understand voice commands, making it easier for people to control their living environments without manual intervention. This convenience extends to various applications such as voice-controlled navigation systems in vehicles and hands-free operation of smartphones. However, the widespread adoption of ASR also raises important ethical considerations. Privacy concerns are paramount as ASR systems often require access to sensitive audio data. Ensuring that these systems are secure and compliant with data protection regulations is essential to maintain public trust. Furthermore, there is a risk of job displacement in sectors where automation replaces human roles, necessitating policies that support workforce retraining and adaptation. In conclusion, the economic and social implications of ASR are far-reaching and impactful. While it offers numerous benefits in terms of efficiency, cost savings, and inclusivity, it also presents challenges related to privacy and job displacement. As ASR continues to evolve and become more integrated into our lives, it is crucial to address these issues proactively to maximize its positive impact while mitigating its negative consequences.
Future Trends and Innovations
As we delve into the future trends and innovations in Automatic Speech Recognition (ASR), it becomes evident that this technology is poised for significant advancements. One of the most promising areas is the integration of deep learning techniques, particularly those involving neural networks. These models, such as Recurrent Neural Networks (RNNs) and Transformers, are expected to enhance accuracy and robustness in speech recognition, especially in noisy environments. Another trend is the development of edge AI, where ASR capabilities are being moved from cloud-based services to local devices, reducing latency and improving real-time processing. Personalization is also a key trend, with ASR systems becoming more adept at recognizing individual voices and adapting to unique speech patterns. This personalization will be crucial for applications like smart home devices, virtual assistants, and healthcare diagnostics. Furthermore, advancements in multi-language support will expand ASR's global reach, enabling more inclusive communication across diverse linguistic communities. The rise of voice biometrics will play a significant role in security and authentication processes, leveraging ASR to verify identities through voice patterns. Additionally, the integration of ASR with other technologies like computer vision and natural language processing (NLP) will create more sophisticated human-machine interfaces, enhancing user experiences in various sectors such as customer service, education, and entertainment. In terms of impact, these innovations will have far-reaching consequences. For instance, improved ASR will revolutionize accessibility for people with disabilities by providing more accurate and reliable speech-to-text systems. In healthcare, ASR can streamline clinical documentation processes, allowing healthcare professionals to focus more on patient care. In education, personalized learning experiences can be tailored using ASR to analyze student responses and provide real-time feedback. Moreover, the applications of ASR will extend into new domains such as autonomous vehicles, where voice commands can enhance safety and convenience. The retail sector will also benefit from enhanced customer service through voice-activated interfaces in stores and online platforms. Overall, the future of ASR is marked by exciting innovations that promise to transform how we interact with technology and each other.