What Does Rng Mean
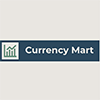
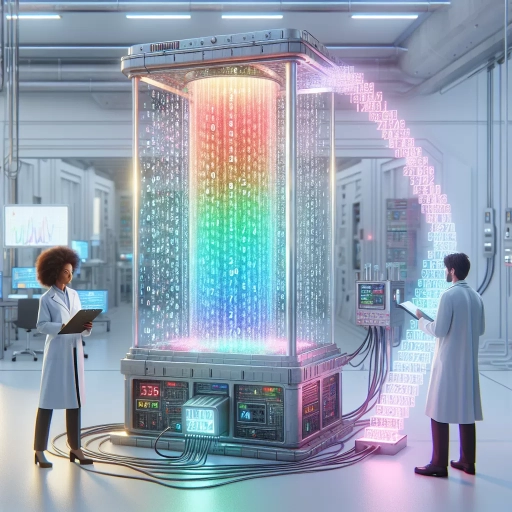
Random Number Generation (RNG) is a fundamental concept that permeates various aspects of modern life, from technology and gaming to scientific research and financial systems. At its core, RNG involves the creation of sequences of numbers that appear to be randomly distributed, lacking any predictable pattern. This article delves into the multifaceted world of RNG, starting with **Understanding the Basics of RNG**, where we explore the principles behind generating truly random numbers and the distinctions between true randomness and pseudo-randomness. We then examine **Applications of RNG in Various Fields**, highlighting how RNG is crucial in fields such as cryptography, simulations, and entertainment. Finally, we discuss the **Impact and Significance of RNG**, revealing its profound influence on data security, statistical analysis, and decision-making processes. By grasping these elements, readers will gain a comprehensive understanding of RNG's importance and its far-reaching implications. Let's begin by understanding the basics of RNG.
Understanding the Basics of RNG
Understanding the basics of Random Number Generators (RNGs) is crucial in various fields, from gaming and simulations to scientific research and cryptography. At its core, an RNG is a system that produces a sequence of numbers that lack any predictable pattern. This article delves into three key aspects of RNGs: their definition and origin, common usage in different contexts, and their key characteristics and features. First, we explore the definition and origin of RNGs, tracing back their historical development and how they have evolved over time. This foundational knowledge is essential for grasping the underlying principles that make RNGs effective. Next, we examine the common usage of RNGs across different domains. From generating random outcomes in video games to simulating real-world phenomena in scientific research, RNGs play a pivotal role in ensuring unpredictability and fairness. Finally, we discuss the key characteristics and features of RNGs, including their types (true vs. pseudo), algorithms, and the importance of entropy in ensuring randomness. By understanding these aspects, readers can appreciate the complexity and utility of RNGs in modern applications. To begin our journey into the world of RNGs, let's start with their definition and origin, laying the groundwork for a deeper exploration of this fascinating technology.
Definition and Origin
**Understanding the Basics of RNG: Definition and Origin** Random Number Generation (RNG) is a fundamental concept in various fields, including computer science, statistics, and gaming. At its core, RNG refers to the process of generating a sequence of numbers that lack any predictable pattern or sequence. These numbers appear to be randomly distributed and are often used to simulate real-world randomness in simulations, modeling, and decision-making processes. The origin of RNG can be traced back to the early 20th century when scientists and mathematicians began exploring ways to generate truly random numbers. One of the pioneers in this field was John von Neumann, who in the 1940s developed the first algorithm for generating pseudo-random numbers using a linear congruential generator. This method involved a recursive formula that produced a sequence of numbers that mimicked randomness but were actually deterministic. In the digital age, RNG has become crucial for numerous applications. In computing, RNGs are used in algorithms for tasks such as data encryption, statistical sampling, and Monte Carlo simulations. In gaming, RNG is essential for creating unpredictable outcomes in games of chance, ensuring fairness and excitement. For instance, in video games, RNG determines the drop rates of items, the outcomes of battles, and other dynamic elements that enhance player engagement. The distinction between true randomness and pseudo-randomness is important. True randomness involves generating numbers from inherently random physical phenomena like radioactive decay or thermal noise. Pseudo-random numbers, on the other hand, are generated by algorithms that produce sequences that appear random but are actually deterministic and can be reproduced given the same initial conditions. Modern RNG techniques include hardware-based solutions that leverage physical phenomena to generate truly random numbers. These methods are particularly important in high-stakes applications such as cryptography and scientific research where predictability could compromise security or accuracy. In summary, RNG is a vital tool across various disciplines, originating from early mathematical algorithms to modern hardware-based solutions. Understanding RNG is essential for appreciating how randomness is simulated and utilized in today's technology-driven world. Whether it's ensuring secure data transmission or creating immersive gaming experiences, RNG plays a pivotal role in making these processes possible and reliable.
Common Usage in Different Contexts
Random Number Generators (RNGs) are ubiquitous in various contexts, each leveraging their unique capabilities to serve distinct purposes. In **gaming**, RNGs are crucial for creating unpredictable outcomes, such as loot drops, enemy spawn locations, and dice rolls, enhancing player engagement and realism. For instance, in role-playing games (RPGs), RNGs determine the success of attacks, skill checks, and item drops, making each playthrough unique. In **scientific research**, RNGs are essential for simulations and statistical analysis. They help in generating random samples for experiments, ensuring unbiased data collection and accurate results. For example, in clinical trials, RNGs are used to randomize participants into different groups to eliminate selection bias. In **finance**, RNGs play a significant role in risk management and modeling. They are used to simulate market conditions, predict stock prices, and assess portfolio risks through Monte Carlo simulations. This helps financial analysts make informed decisions based on probabilistic outcomes. In **cryptography**, RNGs are vital for generating secure keys and nonces (numbers used once). High-quality RNGs ensure that cryptographic keys are unpredictable and resistant to attacks, safeguarding data integrity and confidentiality. In **education**, RNGs can be used to create interactive learning tools such as quizzes and games that offer randomized questions or scenarios, keeping students engaged and challenged. In **entertainment**, RNGs are used in lotteries and other games of chance to ensure fairness and unpredictability. For example, lottery draws rely on RNGs to select winning numbers randomly. In **engineering**, RNGs are employed in quality control processes to simulate real-world conditions and test the reliability of systems. This helps engineers identify potential flaws and optimize system performance. Overall, RNGs are versatile tools that bring randomness and unpredictability into various fields, enhancing functionality, fairness, and accuracy across different contexts. Understanding how RNGs work is fundamental to appreciating their widespread applications and importance in modern technology and science.
Key Characteristics and Features
When delving into the basics of Random Number Generators (RNGs), it is crucial to understand their key characteristics and features. At its core, an RNG is a system designed to produce a sequence of numbers that appear to be randomly distributed and lack any predictable pattern. Here are the primary characteristics and features that define RNGs: 1. **Unpredictability**: The most fundamental feature of an RNG is its ability to generate numbers that are unpredictable. This means that each number produced should be independent of the previous one, making it impossible to forecast the next number in the sequence. 2. **Uniform Distribution**: High-quality RNGs aim to produce numbers that are uniformly distributed across a specified range. This ensures that every possible number has an equal chance of being generated, which is essential for applications requiring fairness and randomness. 3. **Periodicity**: RNGs can be either truly random or pseudo-random. Truly random RNGs, often referred to as Hardware Random Number Generators (HRNGs), draw randomness from physical phenomena like thermal noise or radioactive decay. Pseudo-random RNGs, on the other hand, use algorithms to generate sequences that appear random but eventually repeat due to their deterministic nature. 4. **Seed Value**: Pseudo-random RNGs rely on a seed value to initiate the generation process. The quality of the seed directly impacts the randomness of the generated sequence. A good seed should be unique and unpredictable to ensure the sequence does not repeat too quickly. 5. **Speed and Efficiency**: The speed at which an RNG can generate numbers is critical for many applications, especially in real-time systems or simulations. Efficient algorithms and hardware implementations are necessary to meet performance requirements without compromising randomness. 6. **Security**: For applications requiring high security, such as cryptography, RNGs must be resistant to attacks that could predict or manipulate the generated numbers. Secure RNGs often combine multiple sources of randomness and employ advanced algorithms to ensure robustness. 7. **Entropy**: Entropy measures the amount of uncertainty or randomness in a sequence. High-entropy RNGs are more desirable as they provide better randomness and are less predictable. Entropy is particularly important in cryptographic applications where security depends on the unpredictability of keys and nonces. 8. **Regulatory Compliance**: In certain fields like gaming and finance, RNGs must comply with regulatory standards to ensure fairness and transparency. These standards often specify minimum requirements for randomness, uniformity, and unpredictability. Understanding these characteristics and features is essential for selecting and implementing the right RNG for specific use cases, whether it be in gaming, simulations, statistical analysis, or secure data transmission. By recognizing the strengths and limitations of different types of RNGs, users can ensure that their applications meet the necessary standards for randomness and reliability.
Applications of RNG in Various Fields
Random Number Generators (RNGs) have become indispensable tools across a wide range of fields, each leveraging their unique capabilities to drive innovation and efficiency. In the realm of **Gaming and Entertainment**, RNGs are crucial for creating unpredictable and engaging experiences, from simulating dice rolls in board games to generating random events in video games. This application ensures that players encounter diverse scenarios, enhancing replay value and overall enjoyment. Beyond entertainment, **RNGs in Science and Research** facilitate simulations and modeling, allowing scientists to study complex systems and predict outcomes with greater accuracy. They are also vital in statistical analysis, helping researchers to randomize samples and minimize bias. In **Technology and Computing**, RNGs play a critical role in cryptography, ensuring secure data transmission by generating unguessable keys. Additionally, they are used in optimization algorithms and machine learning models to introduce randomness, which can lead to better solutions and more robust models. Understanding the diverse applications of RNGs highlights their versatility and importance across various disciplines, starting with their significant impact in the dynamic world of gaming and entertainment.
RNG in Gaming and Entertainment
Random Number Generation (RNG) plays a pivotal role in the gaming and entertainment industries, enhancing user experience and ensuring fairness. In video games, RNG is used to create dynamic and unpredictable environments, making each playthrough unique. For instance, in role-playing games (RPGs), RNG determines the drop rates of items, the outcomes of battles, and the behaviors of non-player characters (NPCs), adding a layer of realism and replayability. In multiplayer games like online poker or card games, RNG ensures that card shuffles and deals are random, preventing any form of predictability or cheating. This randomness also extends to procedural generation in games like Minecraft or No Man's Sky, where entire worlds are generated on the fly using complex algorithms that rely on RNG to create diverse landscapes and resources. In the realm of entertainment beyond gaming, RNG is crucial in simulations and interactive experiences. For example, in virtual reality (VR) and augmented reality (AR) experiences, RNG can be used to generate realistic scenarios or unexpected events that keep users engaged. In film and television production, RNG can be employed in special effects to create realistic simulations of natural phenomena such as weather patterns or crowd behavior. Additionally, RNG is integral in the development of artificial intelligence (AI) characters in movies and video games, allowing them to make unpredictable decisions that mimic human behavior. The use of RNG also extends to live events and performances. In music festivals or concerts, RNG can be used to generate unique light shows or visual effects that are synchronized with the music but vary each time they are displayed. Similarly, in theater productions, RNG can be used to introduce random elements into the script or stage design, making each performance distinct. Moreover, RNG has significant implications for game development and testing. It allows developers to simulate a wide range of scenarios and edge cases without manually creating each one, thereby ensuring that their games are robust and bug-free. This also helps in balancing game mechanics by testing various combinations of variables to find the optimal settings. In summary, RNG is a fundamental component of modern gaming and entertainment, providing the necessary randomness to create engaging, dynamic, and realistic experiences. Its applications span from enhancing gameplay and simulations to ensuring fairness and realism in various forms of entertainment. As technology continues to evolve, the role of RNG will only become more pronounced, driving innovation and creativity in these industries.
RNG in Science and Research
Random Number Generators (RNGs) play a crucial role in various scientific and research fields due to their ability to introduce randomness, which is essential for simulating real-world phenomena, testing hypotheses, and ensuring the integrity of experiments. In **statistical analysis**, RNGs are used to generate random samples for statistical inference, allowing researchers to draw conclusions about larger populations from smaller, representative subsets. This is particularly important in fields like **epidemiology**, where random sampling helps in understanding disease prevalence and distribution. In **computational simulations**, RNGs are vital for modeling complex systems such as weather patterns, financial markets, and molecular interactions. These simulations rely on random inputs to mimic the inherent uncertainties and variability present in real-world systems, enabling scientists to predict outcomes and understand system behaviors under different conditions. For instance, in **climate modeling**, RNGs help simulate the stochastic nature of atmospheric and oceanic processes, which are crucial for predicting future climate scenarios. RNGs also find significant application in **experimental design**, particularly in clinical trials and psychological studies. Randomization ensures that participants are assigned to treatment or control groups without bias, which is essential for establishing cause-and-effect relationships and validating the efficacy of treatments. This methodological rigor helps in minimizing confounding variables and enhancing the reliability of research findings. Moreover, RNGs are indispensable in **machine learning** and **artificial intelligence**. They are used in algorithms such as Monte Carlo methods and stochastic gradient descent to introduce randomness during training processes, which helps in avoiding local minima and improving model generalizability. This randomization is critical for developing robust models that can handle diverse datasets and real-world variability. In **physics and engineering**, RNGs are employed in Monte Carlo simulations to solve problems that are analytically intractable. For example, in **nuclear physics**, RNGs simulate the behavior of subatomic particles, while in **materials science**, they help model the properties of materials under various conditions. These simulations allow researchers to predict material strengths, failure rates, and other critical properties without the need for extensive physical testing. Additionally, RNGs are used in **data encryption** and **cryptography** within scientific research to ensure data security. Secure RNGs generate truly random numbers that are used as keys for encrypting sensitive data, protecting it from unauthorized access. This is particularly important in fields like **genomics** and **medical research**, where sensitive patient information must be safeguarded. In summary, RNGs are a fundamental tool across various scientific disciplines, enabling researchers to simulate real-world randomness, ensure experimental integrity, and develop robust models. Their applications span from statistical analysis and computational simulations to experimental design, machine learning, and data security, making them an indispensable component of modern scientific research.
RNG in Technology and Computing
Random Number Generation (RNG) plays a pivotal role in various technological and computing applications, underpinning the randomness and unpredictability that are crucial for numerous processes. In computing, RNG is essential for simulations, modeling, and statistical analysis. For instance, Monte Carlo simulations rely on RNG to generate random samples that help in estimating outcomes and behaviors in complex systems. This is particularly important in fields like finance, where risk analysis and portfolio optimization depend on the ability to simulate diverse scenarios accurately. In cybersecurity, RNG is vital for generating secure keys and nonces (numbers used once) that are indispensable for encryption algorithms. Secure random number generators ensure that cryptographic keys are unpredictable, thereby enhancing the security of data transmission and storage. Without robust RNG, cryptographic systems would be vulnerable to attacks, compromising the integrity and confidentiality of sensitive information. Gaming also heavily relies on RNG to create dynamic and unpredictable environments. Random number generators are used to determine outcomes such as character stats, loot drops, and enemy behaviors, ensuring that each player's experience is unique and engaging. This randomness adds a layer of realism and replayability to games, making them more enjoyable and challenging. Moreover, RNG has significant applications in scientific research. In fields like quantum mechanics and particle physics, random number generators are used to simulate the inherent randomness of quantum phenomena. This helps scientists understand and predict the behavior of particles at the subatomic level, which is crucial for advancing our understanding of the universe. In addition, RNG is used in machine learning and artificial intelligence to introduce randomness into algorithms, which can help avoid overfitting and improve model generalization. Random initialization of weights in neural networks and random sampling of data during training are common practices that leverage RNG to enhance model performance. Lastly, RNG finds its way into everyday applications such as lotteries and online gambling, where fairness and unpredictability are paramount. Ensuring that outcomes are truly random is essential for maintaining trust and compliance with regulatory standards. In summary, RNG is a fundamental component across various technological and computing domains, providing the necessary randomness for simulations, security, gaming, scientific research, machine learning, and other critical applications. Its importance cannot be overstated, as it underpins many of the technologies that we rely on daily.
Impact and Significance of RNG
The impact and significance of Random Number Generators (RNGs) are multifaceted and far-reaching, influencing various aspects of modern life. RNGs play a crucial role in ensuring randomization and fairness, which is essential in fields such as gaming, statistical analysis, and cryptography. However, their implementation is not without challenges and limitations, including the difficulty in achieving true randomness and the potential for bias. Despite these hurdles, ongoing research and innovations are paving the way for future developments that will enhance the capabilities and reliability of RNGs. This article delves into these key areas, starting with the critical role RNGs play in randomization and fairness, where their ability to generate unpredictable sequences ensures equitable outcomes in diverse applications.
Role in Randomization and Fairness
Randomization plays a crucial role in ensuring fairness across various domains, from scientific research and clinical trials to gaming and decision-making processes. At its core, randomization involves the use of random number generators (RNGs) to introduce unpredictability and eliminate bias. In scientific research, randomization helps in distributing participants into different groups (such as treatment and control groups) in a way that minimizes confounding variables, thereby enhancing the reliability and generalizability of the results. This method ensures that any observed differences between groups can be attributed to the intervention being tested rather than pre-existing differences. In clinical trials, randomization is essential for maintaining ethical standards and ensuring that the outcomes are not influenced by external factors. It helps in balancing known and unknown confounders, which is critical for drawing valid conclusions about the efficacy and safety of new treatments. For instance, in a randomized controlled trial (RCT), participants are randomly assigned to either receive the new treatment or a placebo, which helps in isolating the effect of the treatment itself. In the realm of gaming, RNGs are used to create unpredictable outcomes, such as the roll of dice or the draw of cards, ensuring that each player has an equal chance of winning. This fairness is paramount for maintaining player trust and engagement. For example, in online multiplayer games, RNGs can be used to determine spawn points, item drops, or other critical elements that affect gameplay balance. Moreover, randomization is vital in decision-making processes where impartiality is required. For instance, in legal contexts, RNGs can be used to select jurors randomly from a pool of eligible citizens, ensuring that the jury composition is unbiased and representative of the community. Similarly, in educational settings, randomization can be used to assign students to different classrooms or groups, helping to distribute abilities and backgrounds evenly. The impact of RNGs on fairness is significant because they provide a mechanism for eliminating human bias and ensuring that outcomes are based on chance rather than preconceived notions or external influences. This not only enhances the credibility of results but also fosters trust among participants. In summary, the role of randomization in ensuring fairness is indispensable across multiple fields, making RNGs a cornerstone of ethical and reliable practices. By leveraging RNGs, we can create more equitable environments where outcomes are determined by chance rather than by human prejudices or biases.
Challenges and Limitations of RNG
Despite the significant impact and importance of Random Number Generators (RNGs) in various fields such as cryptography, simulations, and gaming, they face several challenges and limitations. One of the primary challenges is ensuring true randomness. Pseudorandom number generators (PRNGs), which are commonly used due to their efficiency and predictability, can fall short in applications requiring high entropy. True randomness, often derived from physical phenomena like thermal noise or radioactive decay, is harder to achieve and more expensive to implement. This dichotomy between practicality and security can be a significant hurdle. Another limitation is the potential for bias and predictability. Even with advanced algorithms, PRNGs can exhibit patterns over time, making them vulnerable to attacks in cryptographic contexts. For instance, if an attacker can predict the sequence of numbers generated by an RNG, they could compromise secure communications or break through encryption. Ensuring that RNGs produce uniformly distributed numbers without any discernible pattern is a continuous challenge. Scalability is another issue. As the demand for high-quality random numbers increases, especially in fields like quantum computing and high-stakes simulations, existing RNGs may not be able to keep up. Generating large quantities of truly random numbers quickly enough to meet these demands while maintaining quality is a technical challenge. Additionally, regulatory and compliance issues can arise. In industries such as online gaming and financial transactions, RNGs must adhere to strict standards to ensure fairness and transparency. Meeting these regulatory requirements while also ensuring the RNGs are secure and efficient can be complex. Furthermore, environmental factors can affect the performance of RNGs. For example, hardware-based RNGs that rely on physical phenomena can be sensitive to temperature changes, electromagnetic interference, or other environmental conditions that might skew their output. Lastly, the cost and complexity of implementing high-quality RNGs can be prohibitive for some applications. Developing and maintaining robust RNG systems requires significant investment in both technology and expertise, which may not be feasible for all organizations. In summary, while RNGs are crucial in many areas, they are not without their challenges. Addressing these limitations is essential to ensure that RNGs continue to support the integrity and security of various applications across different industries.
Future Developments and Innovations
As we delve into the future developments and innovations surrounding Random Number Generators (RNGs), it becomes clear that these advancements will significantly impact various sectors. One of the most promising areas is in **quantum computing**, where RNGs play a crucial role in generating truly random numbers, essential for cryptographic security and simulations. Quantum RNGs, leveraging principles like photon arrival times or radioactive decay, offer unparalleled randomness and speed, enhancing data security and computational efficiency. In **gaming**, future RNG innovations will focus on creating more realistic and unpredictable outcomes, enhancing player engagement and fairness. Advanced algorithms and machine learning techniques will be integrated to detect and prevent cheating, ensuring a level playing field. This will be particularly important in online gaming, where trust in RNGs is paramount. The **financial sector** will also benefit from improved RNGs, especially in high-frequency trading and risk analysis. More sophisticated RNGs will help in simulating complex financial scenarios, allowing for better decision-making and risk management. Additionally, blockchain technology, which relies heavily on RNGs for secure transactions, will continue to evolve with more robust and decentralized random number generation methods. In **scientific research**, RNGs are vital for simulations and experiments. Future innovations will include the development of ultra-high-speed RNGs capable of generating billions of random numbers per second, facilitating breakthroughs in fields like climate modeling, drug discovery, and materials science. Moreover, **Internet of Things (IoT)** devices will increasingly rely on RNGs for secure communication and data encryption. As IoT expands, the demand for lightweight yet secure RNG algorithms will grow, driving innovation in this area. Lastly, **artificial intelligence** and **machine learning** models will incorporate advanced RNG techniques to improve model training and validation. This will lead to more accurate predictions and better generalization of AI models across various applications. In summary, future developments in RNG technology are poised to revolutionize multiple industries by providing enhanced security, efficiency, and realism. These innovations will be pivotal in driving forward technological advancements and ensuring the integrity of data-driven processes across diverse fields.