What Is A Responding Variable
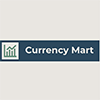
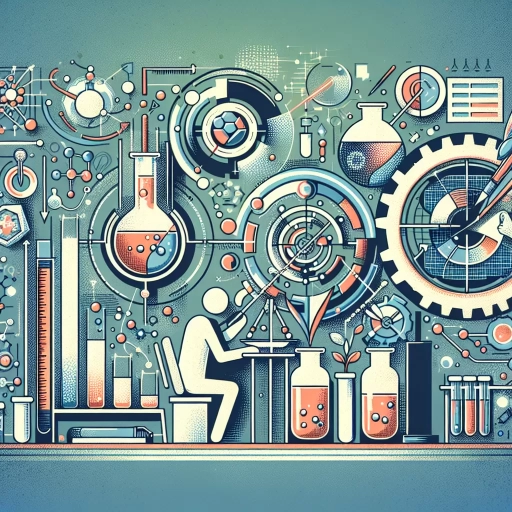
In the realm of scientific research and data analysis, understanding the nuances of variables is crucial for drawing accurate conclusions and making informed decisions. Among these variables, the responding variable stands out as a pivotal element that helps researchers gauge the impact of various factors on a specific outcome. This article delves into the concept of a responding variable, providing a comprehensive overview that will guide readers through its fundamental aspects. We will begin by **Understanding the Concept of a Responding Variable**, where we explore its definition and role within experimental designs. Next, we will discuss **Identifying and Measuring Responding Variables**, highlighting the methods and tools used to pinpoint and quantify these variables effectively. Finally, we will examine **Importance and Applications of Responding Variables**, revealing how these variables are essential in diverse fields such as medicine, psychology, and environmental science. By grasping these key points, readers will gain a deeper appreciation for the significance of responding variables in research. Let us start by **Understanding the Concept of a Responding Variable**.
Understanding the Concept of a Responding Variable
In the realm of scientific research and experimental design, understanding the nuances of variables is crucial for drawing accurate conclusions and making informed decisions. At the heart of this understanding lies the concept of the responding variable, a fundamental element that plays a pivotal role in how experiments are structured and interpreted. This article delves into the essence of responding variables, beginning with a clear **Definition and Context** to establish a solid foundation. It then explores the **Role in Experimental Design**, highlighting how responding variables influence the methodology and outcomes of studies. Additionally, it clarifies the **Difference from Independent Variables**, ensuring readers can distinguish between these often-confused terms. By grasping these key aspects, researchers and learners alike can enhance their comprehension of experimental processes, ultimately leading to a deeper **Understanding of the Concept of a Responding Variable**.
Definition and Context
In the context of scientific research and data analysis, understanding the concept of a responding variable is crucial for drawing meaningful conclusions. A responding variable, also known as the dependent variable or outcome variable, is a measurable attribute that is being observed or measured in response to changes made to another variable, known as the independent or predictor variable. This distinction is fundamental because it allows researchers to isolate cause-and-effect relationships and understand how different factors influence outcomes. To define it more precisely, a responding variable is the variable whose value is being measured or observed in an experiment or study. For instance, in a study examining the impact of exercise on blood pressure, blood pressure would be the responding variable because it is the outcome being measured in response to the independent variable (exercise). The context in which this variable operates is critical; it must be clearly defined and measured to ensure that any observed changes can be attributed to the manipulation of the independent variable rather than extraneous factors. The context also involves considering other variables that might influence the responding variable, known as confounding variables. These need to be controlled or accounted for to ensure that the results are reliable and valid. For example, in the exercise and blood pressure study, factors such as diet, age, and pre-existing health conditions could affect blood pressure and must be considered when interpreting the results. Understanding the responding variable within its context enables researchers to design studies that are robust and capable of providing clear insights into cause-and-effect relationships. It also helps in generalizing findings to broader populations and applying them in practical settings. For instance, if a study finds a significant relationship between exercise and reduced blood pressure, healthcare providers can use this information to recommend exercise as a treatment strategy for patients with hypertension. In summary, the responding variable is a cornerstone of scientific inquiry, allowing researchers to quantify and analyze the effects of various interventions or changes. By carefully defining and controlling for this variable within its context, researchers can build a strong foundation for understanding complex phenomena and making informed decisions based on empirical evidence. This understanding is essential not only for advancing knowledge but also for translating research findings into practical applications that benefit society.
Role in Experimental Design
In the realm of experimental design, the role of a responding variable is pivotal in determining the outcomes and validity of the experiment. A responding variable, also known as the dependent variable, is the outcome or result that changes in response to the independent variable (the factor being manipulated by the experimenter). Understanding this concept is crucial because it allows researchers to measure the effect of their interventions accurately. When designing an experiment, identifying and defining the responding variable is a critical step. This involves clearly specifying what will be measured and how it will be quantified. For instance, in a study examining the impact of different fertilizers on plant growth, the responding variable could be the height of the plants or their biomass. The precision in defining this variable ensures that data collection is focused and relevant, thereby enhancing the reliability of the findings. Moreover, the responding variable must be measurable and quantifiable to allow for statistical analysis. This could involve using tools such as scales, spectrophotometers, or other specialized equipment depending on the nature of the variable. Ensuring that the measurement process is consistent and free from bias is essential for maintaining the integrity of the experiment. The selection of an appropriate responding variable also influences the experimental design's sensitivity and specificity. A well-chosen responding variable can capture subtle changes in response to the independent variable, providing nuanced insights into the underlying mechanisms. Conversely, a poorly chosen variable might fail to detect significant effects or introduce unnecessary variability, leading to inconclusive results. Additionally, the responding variable plays a key role in hypothesis testing. By comparing the values of the responding variable across different treatment groups, researchers can determine whether there are statistically significant differences that support or reject their hypotheses. This process relies heavily on the accuracy and precision of the measurements obtained for the responding variable. In summary, the responding variable is the cornerstone of experimental design, serving as the metric through which researchers evaluate the impact of their manipulations. Its careful selection, precise measurement, and appropriate analysis are essential for drawing valid conclusions and advancing scientific knowledge. By understanding and effectively utilizing the responding variable, researchers can ensure that their experiments yield meaningful and reliable results.
Difference from Independent Variables
When delving into the realm of scientific research and experimentation, understanding the distinction between independent variables and responding variables is crucial. The independent variable, often referred to as the predictor or explanatory variable, is the factor that researchers manipulate or change to observe its effect on the outcome. In contrast, the responding variable, also known as the dependent variable or outcome variable, is the result or outcome being measured in response to changes made to the independent variable. To illustrate this difference, consider a simple experiment where a researcher aims to determine how different levels of sunlight exposure affect plant growth. Here, the amount of sunlight (e.g., low, medium, high) would be the independent variable because it is the factor being intentionally varied by the researcher. On the other hand, plant growth (measured in terms of height or biomass) would be the responding variable because it is the outcome being observed and measured in response to varying levels of sunlight exposure. Understanding this distinction is vital for several reasons. Firstly, it allows researchers to establish cause-and-effect relationships between variables. By controlling for other factors and isolating the independent variable, researchers can confidently attribute changes in the responding variable to the manipulation of the independent variable. Secondly, this clarity helps in designing experiments that are both efficient and effective. For instance, knowing which variable to manipulate and which to measure ensures that data collection efforts are focused and meaningful. Moreover, recognizing the difference between independent and responding variables aids in interpreting results accurately. Misidentifying these variables can lead to flawed conclusions and potentially misleading interpretations of data. For example, if a study incorrectly identifies plant growth as an independent variable and sunlight exposure as a responding variable, it would suggest that plant growth causes changes in sunlight levels—a clearly nonsensical conclusion. In summary, the distinction between independent and responding variables forms the backbone of experimental design and data analysis. By clearly defining these roles within an experiment, researchers can ensure that their studies are well-structured, their findings are reliable, and their conclusions are valid. This foundational understanding is essential for anyone seeking to grasp the concept of a responding variable and its role in scientific inquiry.
Identifying and Measuring Responding Variables
In the realm of research and data analysis, understanding and accurately measuring responding variables is crucial for drawing meaningful conclusions and making informed decisions. Responding variables, which are outcomes or results that change in response to changes in other variables, form the backbone of many studies across various disciplines. To effectively identify and measure these variables, it is essential to grasp the different types that exist, each with its unique characteristics and implications. This article will delve into the **Types of Responding Variables**, exploring their distinct features and how they are utilized in different contexts. Additionally, we will discuss **Methods for Measurement**, highlighting the various techniques and approaches used to quantify and analyze these variables. Finally, we will examine **Tools and Instruments Used**, detailing the specific tools and instruments that researchers employ to collect and analyze data on responding variables. By understanding these key aspects, readers will gain a comprehensive insight into the concept of responding variables, enabling them to apply this knowledge effectively in their own research endeavors. This foundational understanding is pivotal for **Understanding the Concept of a Responding Variable**.
Types of Responding Variables
When delving into the realm of research and experimentation, understanding the types of responding variables is crucial for accurate data collection and analysis. Responding variables, also known as dependent variables, are the outcomes or results that researchers measure in response to changes made to the independent variable. There are several key types of responding variables that researchers encounter, each with its own characteristics and implications for study design. **Quantitative Responding Variables** are numerical in nature and can be measured on a continuous scale. Examples include height, weight, blood pressure, and scores on a standardized test. These variables allow for precise measurements and statistical analysis, enabling researchers to draw robust conclusions about the relationships between variables. For instance, in a study examining the effect of exercise on blood pressure, the responding variable would be the measured blood pressure readings before and after the exercise intervention. **Qualitative Responding Variables**, on the other hand, are non-numerical and represent categories or attributes. These variables are often used in social sciences and include factors such as gender, ethnicity, or satisfaction levels (e.g., satisfied, neutral, dissatisfied). Qualitative responding variables require different analytical approaches compared to quantitative ones, often involving frequency distributions or chi-square tests to identify patterns and associations. **Ordinal Responding Variables** fall somewhere in between quantitative and qualitative variables. They represent categories that have a natural order or ranking but do not have equal intervals between them. Examples include educational levels (high school, bachelor's degree, master's degree) or pain levels (mild, moderate, severe). These variables are useful when the data does not meet the criteria for quantitative analysis but still conveys meaningful order. **Nominal Responding Variables** are purely categorical without any inherent order. They are used to label or classify data into distinct groups without any quantitative value. Examples include brand preference or type of medication used. Nominal variables are often analyzed using frequency counts and cross-tabulations to understand distributions and relationships. Understanding these types of responding variables is essential for designing effective studies and interpreting results accurately. By identifying the appropriate type of responding variable, researchers can select the most suitable statistical methods and ensure that their findings are reliable and meaningful. This precision in measurement and analysis enhances the validity of research conclusions, allowing for better decision-making and policy development across various fields. In summary, recognizing the different types of responding variables is a foundational step in conducting rigorous research that yields actionable insights.
Methods for Measurement
When it comes to identifying and measuring responding variables, the choice of method is crucial for the accuracy and reliability of the data collected. There are several robust methods that researchers and analysts employ to measure these variables, each tailored to specific contexts and objectives. **Surveys and Questionnaires** are among the most common tools, allowing for the collection of self-reported data. These can be administered online, via mail, or in person, and they offer a flexible way to gather information on attitudes, behaviors, and experiences. However, they are subject to biases such as social desirability bias and recall bias. **Observational Studies** provide an alternative by allowing researchers to collect data through direct observation of behaviors or phenomena. This method can be further divided into structured and unstructured observations. Structured observations involve predefined criteria and protocols to ensure consistency, while unstructured observations offer more flexibility but may lack standardization. **Experiments** are another powerful method, particularly useful for establishing causality between variables. By manipulating independent variables and controlling for extraneous factors, experiments can provide clear insights into how responding variables change in response to different conditions. **Physiological Measures** are increasingly used in various fields, including psychology and healthcare. Techniques such as electroencephalography (EEG), heart rate monitoring, and skin conductance tests offer objective measures of physiological responses that can complement or validate self-reported data. **Interviews**, both structured and semi-structured, provide rich qualitative data that can offer deep insights into respondents' thoughts and experiences. These are particularly useful when exploring complex or nuanced topics where quantitative measures might fall short. **Secondary Data Analysis** involves using existing datasets to measure responding variables. This approach is cost-effective and can provide large sample sizes, but it requires careful consideration of the original data collection methods to ensure relevance and reliability. **Sensors and Wearable Devices** are becoming more prevalent, enabling continuous monitoring of physical activities, environmental exposures, and other health-related metrics. These devices offer high-resolution data that can capture subtle changes over time. In conclusion, the selection of measurement methods for responding variables depends on the research question, the nature of the variable being measured, and the resources available. By choosing the appropriate method or combination of methods, researchers can ensure that their data is accurate, reliable, and meaningful, thereby enhancing the validity of their findings. Each method has its strengths and limitations, and understanding these is essential for making informed decisions in the process of identifying and measuring responding variables.
Tools and Instruments Used
When identifying and measuring responding variables, the choice of tools and instruments is crucial for ensuring accuracy, reliability, and validity. Responding variables, which are outcomes or dependent variables that change in response to an independent variable, require precise measurement to draw meaningful conclusions. Here, a variety of tools and instruments come into play. **Quantitative Instruments:** For numerical data, tools such as surveys with Likert scales, questionnaires, and rating scales are commonly used. These instruments allow for the collection of data that can be statistically analyzed to identify trends and patterns. For example, in a study examining the impact of a new teaching method on student performance, a standardized test could serve as a quantitative instrument to measure the responding variable of student achievement. **Qualitative Instruments:** When qualitative data is needed, tools like interviews, focus groups, and observational studies are employed. These methods provide rich, detailed insights into respondents' experiences and perceptions. For instance, in a study on patient satisfaction with healthcare services, semi-structured interviews could be used to gather qualitative data on the responding variable of patient satisfaction. **Technological Tools:** Modern technology has introduced a range of innovative instruments for data collection. Mobile apps, online platforms, and wearable devices can collect real-time data on various responding variables such as physical activity levels, heart rate, or cognitive function. These tools offer the advantage of continuous monitoring and can provide large datasets for analysis. **Psychometric Instruments:** In fields like psychology and education, psychometric instruments such as personality tests (e.g., Myers-Briggs Type Indicator) and cognitive assessments (e.g., IQ tests) are used to measure complex responding variables like personality traits or cognitive abilities. These instruments are designed to be reliable and valid measures of the constructs they assess. **Physical Measurement Tools:** In scientific research, particularly in fields like physics, biology, and engineering, precise physical measurement tools are essential. Instruments such as thermometers, spectrometers, and microscopes allow researchers to accurately measure responding variables related to physical phenomena. For example, in a study on the effects of temperature on chemical reactions, a thermometer would be used to measure the responding variable of reaction rate. **Software and Analytical Tools:** Once data is collected, software tools such as SPSS, R, or Excel are used for data analysis. These tools enable researchers to perform statistical tests, visualize data, and draw conclusions about the relationships between variables. Additionally, specialized software for qualitative data analysis like NVivo or Atlas.ti helps in coding and analyzing textual data from interviews or focus groups. In summary, the selection of appropriate tools and instruments is pivotal in accurately identifying and measuring responding variables. By choosing the right tools—whether quantitative, qualitative, technological, psychometric, physical measurement tools, or analytical software—researchers can ensure that their data is reliable and valid, leading to robust conclusions and meaningful insights. This careful consideration of tools and instruments underscores the importance of methodological rigor in scientific research.
Importance and Applications of Responding Variables
In the realm of scientific inquiry and data analysis, understanding the concept of responding variables is crucial for drawing accurate conclusions and making informed decisions. Responding variables, often the focal point of research studies, are the outcomes or results that researchers aim to measure and analyze in response to changes in independent variables. The importance and applications of responding variables span multiple dimensions, each contributing significantly to the integrity and utility of research findings. This article delves into three key aspects: the **Impact on Research Outcomes**, where we explore how responding variables influence the validity and reliability of study results; **Real-World Applications Across Disciplines**, highlighting their relevance in fields such as medicine, psychology, and economics; and **Best Practices for Analysis and Interpretation**, providing insights into how to effectively analyze and interpret data derived from responding variables. By understanding these facets, researchers and practitioners can better grasp the significance of responding variables, ultimately enhancing their ability to design robust studies and derive meaningful insights. Transitioning seamlessly into the core discussion, this article aims to illuminate the multifaceted role of responding variables, leading to a deeper understanding of **Understanding the Concept of a Responding Variable**.
Impact on Research Outcomes
The impact of responding variables on research outcomes is profound and multifaceted, significantly influencing the validity, reliability, and overall quality of scientific studies. Responding variables, which are the outcomes or results measured in response to changes in independent variables, serve as the cornerstone of empirical research. Their accurate measurement and interpretation are crucial for drawing meaningful conclusions and making informed decisions. Firstly, responding variables determine the direction and magnitude of effects observed in a study. For instance, in clinical trials, the responding variable might be the reduction in symptoms or improvement in patient outcomes following a new treatment. If these variables are not precisely defined or measured, the true efficacy of the treatment could be misinterpreted, leading to incorrect conclusions about its effectiveness. Secondly, responding variables play a critical role in hypothesis testing and theory validation. By carefully selecting and measuring these variables, researchers can test hypotheses and validate theoretical frameworks. For example, in social sciences, responding variables such as attitudes or behaviors can help researchers understand how different interventions affect societal outcomes. Misalignment between the hypothesized effect and the actual responding variable can lead to flawed interpretations and undermine the validity of the research. Thirdly, responding variables are essential for generalizability and replicability. When responding variables are well-defined and consistently measured across studies, it enhances the ability to compare results and draw broader conclusions. This consistency is particularly important in fields like medicine and psychology where replication is key to establishing robust evidence-based practices. Moreover, the choice of responding variables can influence the ethical implications of research. For instance, in studies involving human subjects, ensuring that responding variables are ethically sound and do not cause harm is paramount. Ethical considerations must be integrated into the selection and measurement of these variables to protect participants and maintain public trust in scientific research. Finally, advancements in technology and methodology have expanded the scope of responding variables that can be measured. Modern tools such as wearable devices, genomic sequencing, and advanced statistical software enable researchers to capture complex and nuanced data that were previously unattainable. This has opened up new avenues for research in fields like personalized medicine and environmental science, where detailed responding variables can provide insights into intricate systems and processes. In summary, responding variables are the linchpin of scientific inquiry, directly impacting the accuracy, relevance, and ethical standing of research outcomes. Their careful selection, precise measurement, and thoughtful interpretation are essential for producing high-quality research that contributes meaningfully to our understanding of various phenomena across diverse disciplines.
Real-World Applications Across Disciplines
Responding variables play a crucial role across various disciplines, demonstrating their importance and versatility in real-world applications. In **medicine**, responding variables are essential for clinical trials, where they measure the outcomes of treatments or interventions. For instance, in a study evaluating the efficacy of a new drug, the responding variable might be the reduction in blood pressure or the improvement in symptoms. This data helps researchers and healthcare professionals make informed decisions about treatment protocols. In **environmental science**, responding variables are used to monitor and assess the impact of human activities on ecosystems. For example, water quality studies might focus on variables such as pH levels, dissolved oxygen, and nutrient concentrations to gauge the health of aquatic environments. These measurements inform policies and practices aimed at preserving natural resources. In **economics**, responding variables help economists understand the effects of policy changes or economic interventions. For example, analyzing how changes in interest rates affect employment rates or GDP growth provides valuable insights into economic dynamics. This information is critical for policymakers to make data-driven decisions that promote economic stability and growth. In **psychology**, responding variables are used to measure the outcomes of therapeutic interventions or educational programs. For instance, a study on cognitive-behavioral therapy might use variables such as symptom severity or quality of life scores to evaluate treatment effectiveness. This helps psychologists refine their approaches and improve patient outcomes. In **engineering**, responding variables are crucial for optimizing system performance and safety. In the context of structural engineering, variables like stress levels and material deformation are monitored to ensure that buildings and bridges can withstand various loads. Similarly, in aerospace engineering, responding variables such as temperature and pressure are tracked to ensure the safe operation of aircraft and spacecraft. In **agriculture**, responding variables help farmers and researchers optimize crop yields and plant health. Variables such as soil moisture levels, nutrient uptake, and pest resistance are monitored to develop more efficient farming practices and improve crop resilience to environmental stressors. Across these disciplines, the accurate measurement and analysis of responding variables enable professionals to draw meaningful conclusions, make informed decisions, and drive innovation. By understanding how different factors influence these variables, researchers can develop more effective solutions to complex problems, ultimately enhancing our quality of life and advancing scientific knowledge. The importance of responding variables lies in their ability to provide quantifiable evidence that supports evidence-based practices, making them indispensable tools in a wide range of fields.
Best Practices for Analysis and Interpretation
When analyzing and interpreting responding variables, it is crucial to adhere to best practices that ensure accuracy, reliability, and meaningful insights. First, **clearly define the responding variable** at the outset of your study. This involves understanding its context, relevance to the research question, and how it will be measured. **Operationalize the variable** by specifying the methods and tools used for measurement to ensure consistency and reproducibility. For instance, if you are studying the impact of a new educational program on student performance, define what aspects of performance you will measure (e.g., grades, test scores) and how these measurements will be collected. **Data quality** is another critical aspect. Ensure that data collection instruments are validated and reliable. Conduct pilot studies or pre-tests to identify any potential biases or issues with the measurement tools. **Data cleaning** is essential; meticulously check for errors, outliers, and missing values, and apply appropriate statistical methods to handle these issues without compromising the integrity of the data. **Statistical analysis** should be tailored to the nature of the responding variable. For continuous variables, consider using parametric tests such as ANOVA or regression analysis, while categorical variables may require non-parametric tests like chi-square tests. Always **check assumptions** underlying statistical tests to ensure their validity. **Interpretation** of results must be grounded in the research context. Avoid over-interpreting findings; instead, focus on what the data actually reveal about the relationship between the responding variable and other variables in your study. **Visualize data** using plots and charts to facilitate understanding and communication of complex results. This not only aids in identifying patterns but also helps in presenting findings in a clear and compelling manner. Finally, **consider external validity** when interpreting results. Ask whether the findings can be generalized to other populations or contexts. Reflect on potential limitations of your study and discuss how these might impact the broader applicability of your conclusions. By following these best practices, researchers can ensure that their analysis and interpretation of responding variables are rigorous, insightful, and contribute meaningfully to the body of knowledge in their field. In the context of responding variables, these practices are particularly important because they directly influence the conclusions drawn about cause-and-effect relationships or correlations. By meticulously defining, measuring, analyzing, and interpreting responding variables, researchers can provide robust evidence that supports or refutes hypotheses, ultimately enhancing the importance and applications of their findings.