What Is X Bar In Statistics
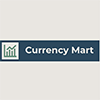
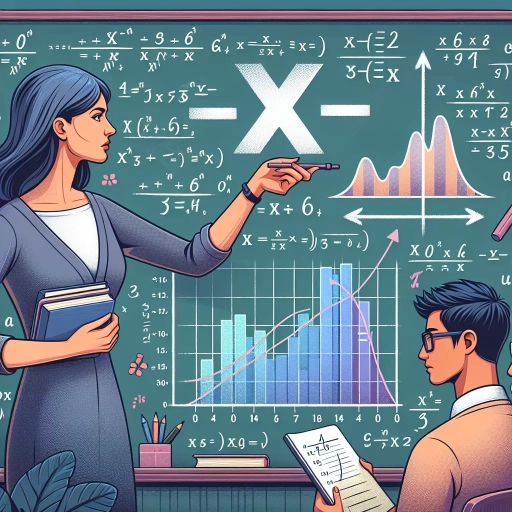
In the realm of statistics, the concept of "X bar" (often denoted as \(\bar{X}\)) is a fundamental tool for understanding and analyzing data. This statistical measure, known as the sample mean, plays a crucial role in summarizing and interpreting datasets. The article delves into the multifaceted nature of X bar, exploring its definition and concept, its various applications and uses, and the methods for calculating and interpreting it. By understanding what X bar represents, researchers and analysts can gain insights into the central tendency of a dataset. The applications of X bar extend across multiple fields, including quality control, hypothesis testing, and data visualization. Finally, knowing how to calculate and interpret X bar is essential for making informed decisions based on statistical data. This article begins by defining and conceptualizing X bar, laying the groundwork for a comprehensive understanding of this vital statistical concept.
Definition and Concept of X Bar
The concept of X Bar, or the sample mean, is a fundamental element in statistics that provides a snapshot of the central tendency of a dataset. Understanding X Bar involves grasping its statistical representation, the process of calculating it, and how it differs from the population mean. This article delves into these critical aspects to offer a comprehensive view of X Bar. First, we will explore the **Statistical Representation** of X Bar, which involves understanding how it is mathematically defined and visually represented. Next, we will discuss the **Sample Mean Calculation**, detailing the step-by-step process of deriving X Bar from a set of data points. Finally, we will examine the **Difference from Population Mean**, highlighting how X Bar contrasts with the mean of the entire population and why this distinction is crucial in statistical analysis. By understanding these facets, readers will gain a deeper insight into the significance and application of X Bar in statistical studies. Let's begin by examining the statistical representation of X Bar.
Statistical Representation
Statistical representation is a fundamental concept in statistics that involves summarizing and interpreting data to understand patterns, trends, and relationships. It is crucial for making informed decisions based on data analysis. In the context of the definition and concept of \( \bar{X} \) (X bar), statistical representation plays a pivotal role. \( \bar{X} \), or the sample mean, is a statistical measure that represents the average value of a sample of data. It is calculated by summing all the values in the sample and then dividing by the number of observations. This measure is essential because it provides a central tendency of the data, giving a snapshot of what the typical value might be. Effective statistical representation involves several key elements. First, it requires accurate data collection to ensure that the sample is representative of the population. This involves careful sampling techniques to avoid bias and ensure that the sample is large enough to be reliable. Once data is collected, it must be summarized using appropriate statistical measures such as mean, median, mode, and standard deviation. These measures help in understanding the distribution of data and identifying any outliers or anomalies. Visual representation is another critical aspect of statistical representation. Tools like histograms, box plots, scatter plots, and bar charts help in visualizing the data, making it easier to interpret and communicate findings. For instance, a histogram can show the distribution of values around the mean (\( \bar{X} \)), while a box plot can highlight skewness and outliers. Moreover, statistical representation involves interpreting the results in context. This includes understanding the limitations of the sample and the potential sources of error or bias. It also involves comparing the sample mean (\( \bar{X} \)) with other statistical measures or benchmarks to draw meaningful conclusions. In practice, statistical representation is used across various fields such as business, healthcare, social sciences, and engineering. For example, in business, statistical representation helps in understanding customer behavior by analyzing sales data and customer feedback. In healthcare, it aids in evaluating treatment outcomes by comparing sample means of different treatment groups. In conclusion, statistical representation is a vital tool for understanding and communicating data insights effectively. The concept of \( \bar{X} \) (X bar) is central to this process as it provides a concise summary of the sample data. By combining accurate data collection, appropriate statistical measures, visual representation, and contextual interpretation, statistical representation enables informed decision-making across diverse domains.
Sample Mean Calculation
The calculation of the sample mean, denoted as \(\bar{X}\) (X bar), is a fundamental concept in statistics that provides an estimate of the population mean. To calculate \(\bar{X}\), you start by summing all the values in your sample dataset. This sum is then divided by the number of observations in the sample, denoted as \(n\). Mathematically, this can be expressed as \(\bar{X} = \frac{\sum_{i=1}^{n} X_i}{n}\), where \(X_i\) represents each individual data point and \(n\) is the total number of data points. For instance, if you have a sample of exam scores: 80, 70, 90, 85, and 75, you would first sum these scores: \(80 + 70 + 90 + 85 + 75 = 400\). Then, you divide this sum by the number of scores, which is 5: \(\frac{400}{5} = 80\). Thus, the sample mean \(\bar{X}\) for this dataset is 80. The sample mean serves as a central tendency measure, providing a single value that best represents the entire dataset. It is crucial for making inferences about the population from which the sample was drawn. For example, if you are analyzing student performance based on a random sample of exam scores, the sample mean can give you an idea of the average performance level of the entire student population. Moreover, understanding how to calculate \(\bar{X}\) is essential for various statistical analyses such as hypothesis testing and confidence intervals. In hypothesis testing, the sample mean is often compared to a known population mean or another sample mean to determine if there are significant differences. In confidence intervals, the sample mean is used to construct an interval that likely contains the true population mean. In practice, calculating the sample mean involves careful attention to detail to ensure accuracy. This includes ensuring that all data points are correctly recorded and included in the summation process. Additionally, it is important to recognize that while the sample mean provides valuable insights into central tendency, it does not capture variability or dispersion within the dataset. In summary, calculating the sample mean involves summing all data points and dividing by the number of observations. This straightforward yet powerful statistical tool is indispensable for understanding and analyzing data, making it a cornerstone of statistical inference and decision-making processes.
Difference from Population Mean
The concept of the difference from the population mean is a crucial aspect in understanding the definition and concept of \( \bar{X} \) (X bar) in statistics. \( \bar{X} \) represents the sample mean, which is the average value of a sample drawn from a larger population. The difference from the population mean, denoted as \( \mu - \bar{X} \), highlights how far the sample mean deviates from the true population mean. This deviation is significant because it helps statisticians assess whether the sample is representative of the population. When calculating \( \bar{X} \), one must consider that samples are subject to variability due to random sampling error. The Central Limit Theorem (CLT) states that as sample size increases, the distribution of sample means will approximate a normal distribution, with the mean of these sample means being equal to the population mean (\( \mu \)). However, for any given sample, there will be some degree of deviation from \( \mu \). Understanding this deviation is essential for statistical inference. For instance, in hypothesis testing, the difference between \( \bar{X} \) and \( \mu \) is used to determine if there is sufficient evidence to reject the null hypothesis that the sample mean is equal to the population mean. Similarly, in confidence intervals, this deviation helps in constructing intervals that are likely to contain the true population mean. Moreover, the standard error of the mean (SEM), which is calculated as \( \sigma / \sqrt{n} \) where \( \sigma \) is the population standard deviation and \( n \) is the sample size, provides a measure of how much variability one can expect in sample means. A smaller SEM indicates that sample means are likely to be closer to the population mean, reducing the difference from \( \mu \). In practical terms, if a researcher finds that their sample mean is significantly different from the known or hypothesized population mean, it may indicate issues such as sampling bias or measurement errors. Conversely, if the sample mean closely approximates the population mean, it suggests that the sample is representative and reliable for making inferences about the larger population. In summary, understanding the difference from the population mean is vital for interpreting and using \( \bar{X} \) effectively in statistical analysis. It allows researchers to evaluate sample representativeness, perform hypothesis tests accurately, and construct reliable confidence intervals—all of which are foundational components of statistical inference. By recognizing and quantifying this deviation, statisticians can make more informed decisions based on their data.
Applications and Uses of X Bar
The X Bar, a statistical tool used to monitor and control processes, has diverse and significant applications across various fields. In manufacturing, it plays a crucial role in **Quality Control**, ensuring that products meet specified standards. By analyzing the mean of sample data, manufacturers can quickly identify deviations and take corrective actions to maintain consistent quality. Beyond manufacturing, the X Bar is integral to **Statistical Process Control (SPC)**, where it helps in detecting shifts in the process mean, thereby enabling real-time adjustments to prevent defects. Additionally, in **Data Analysis in Research Studies**, the X Bar aids researchers in understanding the central tendency of their data, facilitating more accurate conclusions. These applications underscore the versatility and importance of the X Bar in maintaining high standards and driving efficiency. Transitioning to its role in **Quality Control in Manufacturing**, we will delve into how this tool is essential for ensuring product reliability and consistency.
Quality Control in Manufacturing
Quality control in manufacturing is a critical process that ensures products meet specified standards and requirements, thereby enhancing customer satisfaction and reducing the risk of defects. It involves a systematic approach to monitoring and controlling the production process to prevent defects and maintain consistent quality. One of the key tools in quality control is the X-bar chart, which is a type of control chart used to monitor the mean of a process. In the context of manufacturing, X-bar charts are particularly useful for tracking the average performance of a process over time. Here’s how it works: samples are taken at regular intervals from the production line, and the mean of each sample is calculated. These sample means are then plotted on an X-bar chart, which has upper and lower control limits. If the sample means fall within these limits, it indicates that the process is in control and operating within acceptable parameters. However, if any sample mean falls outside these limits, it signals that the process may be out of control, prompting immediate investigation and corrective action. The applications and uses of X-bar charts in manufacturing are multifaceted. For instance, they help in identifying trends or shifts in the process mean, allowing for timely interventions to prevent quality issues. This proactive approach minimizes waste, reduces rework, and lowers overall production costs. Additionally, X-bar charts facilitate continuous improvement by providing insights into process stability and variability. By analyzing these charts, manufacturers can identify opportunities for process optimization and implement changes that lead to improved product quality and increased efficiency. Moreover, X-bar charts are often used in conjunction with other statistical process control (SPC) tools, such as R-charts, which monitor process variability. Together, these tools provide a comprehensive view of the production process, enabling manufacturers to maintain tight control over both the mean and variability of their processes. This integrated approach ensures that products consistently meet quality standards, enhancing brand reputation and customer trust. In summary, quality control in manufacturing relies heavily on tools like X-bar charts to ensure that products are produced to high standards. By monitoring process means and identifying any deviations from expected values, manufacturers can take swift action to correct issues before they escalate. This not only improves product quality but also contributes to operational efficiency and cost savings, making X-bar charts an indispensable part of modern manufacturing practices.
Statistical Process Control (SPC)
Statistical Process Control (SPC) is a methodology that employs statistical techniques to monitor and control processes, ensuring that they operate within predetermined limits. It is a crucial tool in quality control, helping organizations maintain consistency and reduce variability in their processes. SPC involves the use of control charts, such as the X-bar chart, to track the mean of a process over time. The X-bar chart, specifically, plots the average values of samples taken from the process at regular intervals. This allows for the detection of any deviations from the expected mean, enabling prompt corrective actions to be taken. In applications, SPC is widely used across various industries including manufacturing, healthcare, and service sectors. For instance, in manufacturing, SPC helps in maintaining product quality by monitoring key parameters such as dimensions, weight, and performance metrics. In healthcare, it can be used to track patient outcomes and ensure that medical procedures are performed consistently within safe limits. The use of SPC also extends to service industries where it can help in managing customer service quality and operational efficiency. The X-bar chart is particularly useful because it provides a visual representation of the process mean over time, making it easier to identify trends or shifts that may indicate a problem. For example, if the X-bar chart shows a consistent upward trend, it might suggest that the process is drifting away from its target value, necessitating an adjustment. Similarly, if there are sudden spikes or dips in the chart, it could indicate special causes of variation that need immediate attention. Moreover, SPC integrates well with other quality management tools like Six Sigma and Total Quality Management (TQM). By combining these methodologies with SPC techniques, organizations can achieve higher levels of process control and improvement. For instance, Six Sigma projects often rely on SPC to monitor and control processes after improvements have been implemented. In summary, Statistical Process Control is an essential methodology for ensuring process stability and quality. The X-bar chart is a key component of SPC, providing valuable insights into process behavior over time. Its applications span multiple industries and sectors, making it an indispensable tool for maintaining high standards of quality and efficiency. By leveraging SPC and tools like the X-bar chart, organizations can enhance their operational performance and customer satisfaction significantly.
Data Analysis in Research Studies
Data analysis is a crucial component of research studies, serving as the backbone for extracting meaningful insights from raw data. In the context of applications and uses of X-bar, which represents the mean of a sample, data analysis plays a pivotal role in statistical process control and quality assurance. Here’s how it integrates: 1. **Statistical Process Control (SPC):** X-bar charts are used to monitor the mean of a process over time. By analyzing these charts, researchers can identify trends, shifts, and anomalies in the process, ensuring that it remains within specified limits. This is particularly important in manufacturing and production environments where maintaining consistent quality is paramount. 2. **Quality Assurance:** In quality assurance, data analysis involving X-bar helps in determining whether a process is stable and capable of producing output within desired specifications. By analyzing the mean of samples over time, researchers can detect any deviations from the expected mean, allowing for timely interventions to correct the process. 3. **Hypothesis Testing:** Data analysis involving X-bar is also integral to hypothesis testing. For instance, when comparing the mean of a sample to a known population mean or comparing means between different samples, X-bar provides a reliable metric for making these comparisons. This is essential in various fields such as medicine, social sciences, and engineering where understanding differences or similarities between groups is critical. 4. **Regression Analysis:** While X-bar itself is not directly used in regression analysis, understanding the mean of independent variables can help in interpreting regression coefficients. Data analysis here involves examining how changes in these means affect the dependent variable, thereby providing deeper insights into relationships between variables. 5. **Descriptive Statistics:** At its core, data analysis involves descriptive statistics where X-bar is a fundamental measure. It helps in summarizing large datasets into a single value that represents the central tendency of the data. This simplification enables researchers to quickly grasp the essence of their data and make informed decisions based on these summaries. 6. **Comparative Studies:** In comparative studies, X-bar is used to compare the means of different groups or samples. For example, in clinical trials, comparing the mean outcomes of treatment groups versus control groups helps in determining the efficacy of treatments. Similarly, in business research, comparing the mean sales figures of different regions or product lines can inform strategic decisions. 7. **Time Series Analysis:** When analyzing time series data, X-bar can be used to smooth out short-term fluctuations and highlight long-term trends. This is particularly useful in fields like economics and finance where understanding long-term patterns is crucial for forecasting and policy-making. In summary, data analysis involving X-bar is indispensable in research studies across various disciplines. It facilitates statistical process control, quality assurance, hypothesis testing, regression analysis, descriptive statistics, comparative studies, and time series analysis. By leveraging these applications, researchers can derive actionable insights from their data, ensuring that their findings are reliable and meaningful.
Calculating and Interpreting X Bar
Calculating and interpreting the X-bar, or mean, is a crucial step in statistical process control and quality assurance. This metric provides insights into the central tendency of a dataset, helping to monitor and improve processes. To fully understand and apply X-bar effectively, it is essential to delve into three key areas: **Formulas and Mathematical Steps**, **Graphical Representation (Control Charts)**, and **Common Errors and Considerations**. Firstly, mastering the **Formulas and Mathematical Steps** involved in calculating X-bar is fundamental. This includes understanding the arithmetic mean formula and how to apply it to sample data. Secondly, visualizing these calculations through **Graphical Representation (Control Charts)** allows for a clearer understanding of process stability and variability over time. Lastly, being aware of **Common Errors and Considerations** ensures that the results are accurate and reliable, avoiding pitfalls that could mislead decision-making. By exploring these aspects, one can ensure that the X-bar is calculated correctly and interpreted meaningfully. Let's begin by examining the **Formulas and Mathematical Steps** that underpin this critical statistical measure.
Formulas and Mathematical Steps
When calculating and interpreting \( \bar{X} \) (X bar) in statistics, understanding the underlying formulas and mathematical steps is crucial. \( \bar{X} \) represents the sample mean, which is a central tendency measure that helps summarize a dataset. The formula for calculating \( \bar{X} \) is straightforward: \( \bar{X} = \frac{\sum_{i=1}^{n} X_i}{n} \), where \( X_i \) are the individual data points and \( n \) is the total number of observations in the sample. To apply this formula, start by summing all the data points: \( \sum_{i=1}^{n} X_i \). This involves adding each value in your dataset together. For example, if your dataset is {2, 4, 6, 8}, the sum would be \( 2 + 4 + 6 + 8 = 20 \). Next, divide this sum by the number of observations, which in this case is 4. Therefore, \( \bar{X} = \frac{20}{4} = 5 \). Interpreting \( \bar{X} \) involves understanding its significance within the context of your data. The sample mean provides a snapshot of the average value of your dataset and can be used to make inferences about the population mean. For instance, if you are analyzing exam scores and find that \( \bar{X} = 75 \), this suggests that, on average, students scored 75 on the exam. Moreover, \( \bar{X} \) is sensitive to extreme values (outliers), so it's important to check for skewness in your data distribution. If your dataset contains outliers, the median might be a more robust measure of central tendency. In statistical analysis, \( \bar{X} \) is often used in conjunction with other measures like standard deviation (\( s \)) to understand variability. The standard error of the mean (SEM), calculated as \( SEM = \frac{s}{\sqrt{n}} \), provides insight into how representative \( \bar{X} \) is of the population mean. In summary, calculating \( \bar{X} \) involves summing all data points and dividing by the number of observations. Interpreting \( \bar{X} \) requires understanding its role as an average value and considering its limitations, especially in the presence of outliers. By following these mathematical steps and considering contextual factors, you can effectively use \( \bar{X} \) to analyze and interpret your data accurately.
Graphical Representation (Control Charts)
Graphical representation, particularly through control charts, is a crucial tool in statistical process control (SPC) that complements the calculation and interpretation of X-bar values. Control charts are visual representations that help monitor and control processes by tracking the performance of a process over time. When calculating X-bar, which represents the average of sample means, control charts provide a clear and intuitive way to visualize these averages and assess whether the process is in control. In an X-bar chart, each point represents the mean of a sample taken at regular intervals. The chart typically includes three key lines: the central line (CL), which is the grand mean or target value; the upper control limit (UCL); and the lower control limit (LCL). These limits are usually set at three standard deviations from the central line, ensuring that if the process is in control, nearly all sample means will fall within these limits. The graphical representation allows for quick identification of trends, shifts, or anomalies in the process. For instance, if sample means consistently fall above or below the central line, it may indicate a shift in the process mean. Similarly, points that fall outside the control limits suggest that the process is out of control, prompting further investigation to identify and correct any issues. Moreover, control charts can be used to detect patterns such as runs (a sequence of points above or below the central line), trends (a gradual increase or decrease in sample means), or cycles (recurring patterns). These patterns can signal underlying problems that need attention, such as changes in raw materials, equipment malfunctions, or operator errors. In addition to X-bar charts, other types of control charts like R-charts (range charts) and S-charts (standard deviation charts) are often used in conjunction to monitor process variability. Together, these charts provide a comprehensive view of both the mean and variability of the process, ensuring that it operates within specified limits. The use of control charts in conjunction with X-bar calculations enhances statistical process control by making it easier to interpret data and make informed decisions. By visually inspecting these charts, quality control teams can quickly identify when corrective actions are necessary, thereby maintaining high-quality output and reducing variability in the production process. This integrated approach ensures that processes remain stable and capable of producing consistent results, which is essential for maintaining quality standards in various industries.
Common Errors and Considerations
When calculating and interpreting the X-bar (mean) in statistics, it is crucial to be aware of common errors and considerations to ensure accurate and meaningful results. One of the most prevalent errors is **sampling bias**, where the sample does not represent the population adequately, leading to skewed or misleading averages. To mitigate this, it is essential to use random sampling methods and ensure that the sample size is sufficiently large. Another critical consideration is **data quality**. Errors in data collection, such as measurement errors or incorrect recording, can significantly impact the X-bar. Therefore, it is vital to validate data for accuracy and consistency before performing any calculations. Additionally, **outliers** can distort the mean if not handled properly. Identifying and addressing outliers through methods like Winsorization or robust statistical techniques can help maintain the integrity of the analysis. **Homogeneity of variance** is another important factor. If the data does not exhibit constant variance across different groups or conditions, it may be necessary to use transformations or alternative statistical methods that are more robust to non-constant variance. Furthermore, **normality assumptions** should be checked, especially when using parametric tests that rely on normal distribution. Non-normal data may require non-parametric tests or transformations to meet normality assumptions. In terms of interpretation, it is essential to consider the **context** in which the X-bar is being used. For instance, in quality control, X-bar charts are used to monitor process stability over time. Here, understanding the control limits and how they are calculated is crucial for interpreting whether the process is in control or if there are significant deviations. Moreover, **confidence intervals** should be constructed around the X-bar to provide a range within which the true population mean is likely to lie. This helps in understanding the precision of the estimate and making more informed decisions. Lastly, **comparative analysis** with other statistical measures like median and mode can offer a more comprehensive view of the data distribution, especially in cases where the data is skewed. By being mindful of these common errors and considerations, researchers and analysts can ensure that their calculations of X-bar are accurate, reliable, and interpretable, leading to better decision-making and more robust conclusions in various fields of study.