What Is Quota Sampling
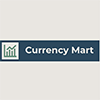
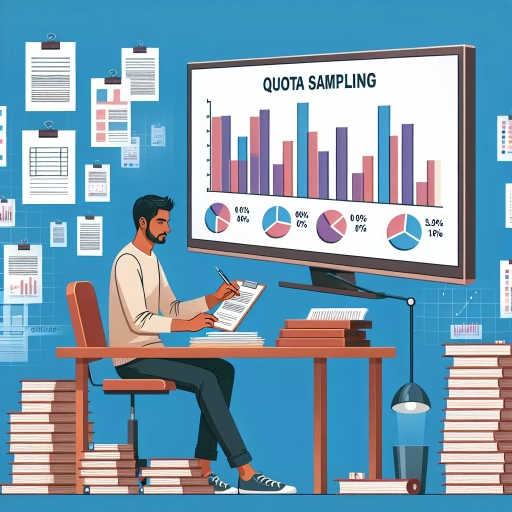
Quota sampling is a widely used method in research and market studies, designed to ensure that the sample population reflects the characteristics of the larger population. This technique is particularly valuable for gathering data that is representative and reliable, making it a cornerstone in various fields such as sociology, marketing, and public health. In this article, we will delve into the intricacies of quota sampling, starting with a comprehensive understanding of what it entails. We will explore how quota sampling works, including the steps involved in selecting participants and ensuring that the sample aligns with predefined criteria. Additionally, we will examine the advantages and limitations of this method, highlighting its strengths and potential drawbacks. By the end of this discussion, readers will have a clear grasp of the role and significance of quota sampling in research. Let us begin by **Understanding Quota Sampling**.
Understanding Quota Sampling
Understanding quota sampling is a crucial aspect of research methodology, particularly in social sciences and market research. This sampling technique involves selecting participants based on predefined criteria to ensure that the sample reflects the characteristics of the population being studied. To delve into the intricacies of quota sampling, it is essential to explore three key areas: its definition and purpose, its key characteristics, and how it differs from other sampling methods. Firstly, understanding the **definition and purpose** of quota sampling provides a foundational grasp of why this method is chosen over others. Quota sampling is designed to ensure that the sample includes a representative number of individuals from different subgroups within the population, thereby enhancing the validity of the research findings. Secondly, examining the **key characteristics** of quota sampling reveals how it is implemented in practice. This includes the selection process, the importance of quotas, and the potential biases that can arise if not managed properly. Lastly, comparing quota sampling with **other sampling methods** highlights its unique advantages and limitations. By understanding these differences, researchers can make informed decisions about which sampling technique best suits their research objectives. In this article, we will begin by exploring the **definition and purpose** of quota sampling, laying the groundwork for a comprehensive understanding of this valuable research tool.
Definition and Purpose
**Understanding Quota Sampling: Definition and Purpose** Quota sampling is a non-probability sampling technique that involves selecting participants based on predefined criteria to ensure that the sample reflects the characteristics of the population. The **definition** of quota sampling hinges on its methodological approach, where researchers identify key demographic or other relevant variables (such as age, gender, income level, or occupation) and then recruit participants until each predefined quota for these variables is filled. This method contrasts with probability sampling techniques, where every member of the population has an equal chance of being selected. The **purpose** of quota sampling is multifaceted. Primarily, it aims to ensure that the sample is representative of the broader population in terms of its key characteristics. By doing so, it helps researchers to draw more accurate and generalizable conclusions about the population being studied. Quota sampling is particularly useful when time and resources are limited, as it allows for quicker data collection compared to probability sampling methods. Additionally, it can be employed in situations where a random sample is not feasible due to constraints such as access to certain groups or limited budget. Another significant purpose of quota sampling is its ability to capture diverse perspectives within a population. By ensuring that all relevant subgroups are represented in the sample, researchers can gain a more comprehensive understanding of how different segments of the population think, behave, or respond to certain stimuli. This is crucial in fields like market research, social sciences, and public health, where understanding the nuances of different demographic groups can inform policy decisions or marketing strategies. Moreover, quota sampling offers flexibility in data collection. Researchers can use various methods to recruit participants, such as online surveys, interviews, or focus groups, making it adaptable to different research contexts. However, it is important to note that while quota sampling provides a structured approach to ensuring representativeness, it does not guarantee that the sample will be free from biases. Therefore, careful consideration must be given to the selection process to minimize potential biases and ensure that the quotas accurately reflect the population's characteristics. In summary, quota sampling is a valuable tool for researchers seeking to gather representative data efficiently. Its definition revolves around the deliberate selection of participants based on predefined criteria, and its purpose is to ensure representativeness, capture diverse perspectives, and facilitate flexible data collection. While it has its limitations, quota sampling remains an essential technique in many fields of research, enabling researchers to make informed decisions and draw meaningful conclusions about the populations they study.
Key Characteristics
**Key Characteristics** Quota sampling, a non-probability sampling method, is distinguished by several key characteristics that set it apart from other sampling techniques. One of the primary characteristics is the use of predefined criteria to select participants. Researchers identify specific demographic or socioeconomic groups that are relevant to the study and then recruit participants until each group's quota is filled. This approach ensures that the sample reflects the diversity of the population in terms of these predefined criteria, such as age, gender, income level, or occupation. Another crucial characteristic of quota sampling is its lack of randomness. Unlike probability sampling methods where every member of the population has an equal chance of being selected, quota sampling relies on the judgment of the researcher to choose participants. This can introduce bias if the researcher's selection is not carefully managed. However, it also allows for more control over the sample composition, which can be advantageous in certain contexts. The flexibility of quota sampling is another significant characteristic. It can be adapted to various research settings and is particularly useful when time and resources are limited. For instance, in market research, quota sampling can quickly provide insights into consumer behavior across different demographic segments without the need for extensive random sampling. Additionally, quota sampling often involves a multi-stage process. Initially, researchers define the quotas based on known population characteristics. Then, they may use various recruitment methods such as interviews, surveys, or focus groups to fill these quotas. This multi-stage approach helps ensure that the sample is representative of the broader population in terms of the specified criteria. Moreover, quota sampling can be more cost-effective compared to probability sampling methods. Since it does not require the exhaustive listing of the entire population or the use of complex randomization techniques, it can be executed with fewer resources. This makes it an attractive option for researchers working with limited budgets. Despite these advantages, quota sampling also has some limitations. The lack of randomness means that it may not be possible to generalize findings to the broader population with the same level of confidence as with probability sampling methods. Furthermore, the quality of the sample depends heavily on the researcher's ability to accurately identify and recruit participants who meet the quota criteria. In summary, quota sampling is characterized by its use of predefined criteria, non-random selection process, flexibility, multi-stage approach, cost-effectiveness, and potential limitations. These characteristics make it a valuable tool for researchers seeking to understand specific segments of a population quickly and efficiently while ensuring that their sample reflects key demographic or socioeconomic attributes. Understanding these characteristics is essential for effectively employing quota sampling in various research contexts and interpreting the results accurately.
Differences from Other Sampling Methods
When delving into the nuances of quota sampling, it is crucial to understand how it differs from other sampling methods. Unlike **random sampling**, where every member of the population has an equal chance of being selected, quota sampling involves selecting participants based on predefined criteria to ensure that the sample mirrors the population's demographic characteristics. This method contrasts with **stratified sampling**, which divides the population into distinct subgroups and then randomly samples from each subgroup. In quota sampling, the selection process is not random; instead, researchers actively seek out participants who fit specific quotas. Another key distinction lies between quota sampling and **convenience sampling**. While convenience sampling relies on easily accessible participants, quota sampling aims to represent the broader population by ensuring that certain demographic quotas are met. This makes quota sampling more robust in terms of representativeness compared to convenience sampling. **Systematic sampling** also differs significantly from quota sampling. Systematic sampling involves selecting every nth member from a list, ensuring a structured and systematic approach. In contrast, quota sampling does not follow a structured sequence but rather focuses on meeting specific demographic targets. Moreover, **cluster sampling** involves dividing the population into clusters and then randomly selecting some of these clusters for the sample. Quota sampling, on the other hand, does not involve clustering; it focuses on individual characteristics rather than geographical or other types of clusters. The flexibility of quota sampling sets it apart from **panel studies**, which involve tracking the same group of participants over time. Quota sampling allows for a fresh set of participants each time it is conducted, providing a snapshot of the current population rather than longitudinal data. In addition, while **snowball sampling** relies on existing study subjects to recruit future subjects, quota sampling does not depend on referrals but instead seeks out participants directly based on predefined criteria. Understanding these differences highlights the unique strengths and limitations of quota sampling. It offers a balanced representation of the population by ensuring that key demographic groups are included, making it particularly useful in market research and social sciences where representativeness is crucial. However, it lacks the randomness that other methods provide, which can introduce bias if not managed carefully. In summary, quota sampling stands out due to its deliberate selection process aimed at achieving demographic balance, distinguishing it from other methods that either rely on randomness or convenience. This approach makes it an invaluable tool for researchers seeking to capture a representative cross-section of the population without the constraints of random selection or the limitations of other non-random methods.
How Quota Sampling Works
Quota sampling is a methodological approach in research that ensures the sample reflects the demographic characteristics of the population being studied. This technique is particularly useful for gathering data that is representative and reliable. To understand how quota sampling works, it is essential to delve into three key components: the selection process, quota determination and allocation, and data collection techniques. The selection process involves identifying and recruiting participants who fit specific demographic criteria, such as age, gender, income level, or occupation. This step is crucial as it ensures that the sample mirrors the population's diversity. Quota determination and allocation involve setting the number of participants needed for each demographic group based on the population's proportions. This ensures that the sample is balanced and representative. Finally, data collection techniques are tailored to gather information from these selected participants efficiently. These techniques can include surveys, interviews, or observational studies. By understanding these elements, researchers can effectively implement quota sampling to achieve high-quality, informative, and engaging data. Let's start by examining the **Selection Process** in more detail.
Selection Process
In the context of quota sampling, the selection process is a critical component that ensures the sample accurately reflects the desired demographic characteristics of the population. This method involves identifying specific quotas or proportions of different subgroups within the population and then selecting participants to meet these quotas. Here’s how it works: First, researchers define the relevant demographic variables such as age, gender, income level, and geographic location. They then determine the desired proportions of each subgroup based on prior knowledge or data about the population. For instance, if a study aims to understand consumer preferences among different age groups, the quotas might be set to reflect the actual age distribution in the population. Once these quotas are established, researchers proceed to recruit participants. This can be done through various methods including surveys, interviews, or online questionnaires. The key is to ensure that each participant fits into one of the predefined subgroups until all quotas are filled. For example, if a quota for 18-24-year-olds is set at 20%, researchers will continue recruiting individuals within this age range until they reach the specified number. The selection process in quota sampling is often non-random; instead of using random sampling techniques, researchers may use convenience sampling or judgment sampling to select participants who meet the quota criteria. This approach allows for more control over the sample composition but can introduce biases if not managed carefully. To mitigate this risk, researchers should strive for diversity within each subgroup and avoid relying solely on easily accessible populations. Another important aspect of the selection process is ensuring that participants are representative within their respective subgroups. While quota sampling guarantees that the overall sample reflects the desired demographic mix, it does not guarantee representativeness within each subgroup. Therefore, researchers must take additional steps to validate that their sample is not skewed by other factors such as socioeconomic status or education level. In summary, the selection process in quota sampling involves meticulous planning and execution to ensure that the sample aligns with predefined demographic quotas. By carefully defining these quotas and systematically recruiting participants to meet them, researchers can create a sample that mirrors key characteristics of the population under study. However, it is crucial to be aware of potential biases and take measures to ensure representativeness within each subgroup to enhance the validity and reliability of the findings. This balanced approach makes quota sampling a valuable tool for market research, social sciences, and other fields where understanding diverse perspectives is essential.
Quota Determination and Allocation
Quota determination and allocation are critical components of the quota sampling method, ensuring that the sample accurately reflects the demographic characteristics of the population being studied. This process begins with quota determination, where researchers identify the key demographic variables that are crucial for the study, such as age, gender, income level, and geographic location. These variables are selected based on their relevance to the research objectives and the need to ensure that the sample is representative of the broader population. Once the relevant demographic variables are identified, researchers proceed to set quotas for each category. For instance, if a study aims to understand consumer behavior among different age groups, quotas might be set to ensure that the sample includes a specific number of participants from each age group (e.g., 18-24, 25-34, 35-44). These quotas are typically determined based on the known proportions of these groups within the population, ensuring that the sample mirrors the population's demographic structure. The allocation phase involves selecting participants to fill these quotas. Researchers may use various methods to recruit participants, such as surveys, interviews, or online questionnaires. Unlike random sampling methods where every member of the population has an equal chance of being selected, quota sampling relies on non-probability sampling techniques. This means that researchers actively seek out individuals who fit into specific demographic categories until all quotas are filled. To maintain the integrity of the sample, it is essential to ensure that participants are selected in a way that minimizes bias. For example, researchers might use stratified sampling within each quota group to capture a diverse range of opinions and experiences. Additionally, they may employ techniques such as snowball sampling or convenience sampling to reach hard-to-reach populations while still adhering to the predefined quotas. The advantages of quota determination and allocation include cost-effectiveness and efficiency. By targeting specific demographic groups directly, researchers can gather data more quickly and with fewer resources compared to random sampling methods. However, this approach also has its limitations; it does not provide the same level of statistical reliability as probability sampling methods because it does not guarantee that every member of the population has an equal chance of being selected. Despite these limitations, quota sampling remains a valuable tool in many fields such as market research, social sciences, and public health studies where rapid data collection and cost constraints are significant factors. By carefully determining and allocating quotas based on relevant demographic variables, researchers can construct a sample that is representative enough to provide meaningful insights into the population under study. This approach allows for timely and targeted data collection while ensuring that key demographic groups are adequately represented in the sample. Ultimately, effective quota determination and allocation are pivotal in ensuring that quota sampling achieves its intended purpose of providing a reliable snapshot of the population's characteristics and behaviors.
Data Collection Techniques
Data collection techniques are the backbone of any research or survey methodology, including quota sampling. These techniques involve systematic methods to gather data that accurately represent the population being studied. There are several key data collection techniques that researchers employ, each with its own strengths and limitations. **Surveys and Questionnaires** are among the most common methods, allowing researchers to collect self-reported data directly from participants. These can be administered online, via phone, or in person, making them versatile and cost-effective. However, they rely on participants' honesty and may suffer from biases such as social desirability bias. **Interviews**, both structured and unstructured, offer deeper insights into participants' thoughts and behaviors. Structured interviews follow a set of predefined questions, while unstructured interviews allow for more open-ended discussions. This method is particularly useful for qualitative research but can be time-consuming and may introduce interviewer bias. **Observational Studies** involve observing participants in their natural environments or under controlled conditions. This method can provide rich, contextual data but requires careful planning to avoid observer bias and ensure ethical considerations are met. **Secondary Data Collection** involves using existing data from sources such as databases, literature reviews, or previous studies. This approach is efficient and cost-effective but may lack the specificity needed for certain research questions. **Experiments** are used to establish cause-and-effect relationships by manipulating variables and measuring outcomes. While experiments offer high internal validity, they can be expensive and may not generalize well to real-world settings. In the context of quota sampling, these data collection techniques are crucial for ensuring that the sample reflects the desired demographic characteristics of the population. For instance, if a researcher is using quota sampling to study consumer behavior among different age groups, they might use surveys or interviews to collect data from participants who fit into predefined age categories. By combining these techniques with quota sampling, researchers can ensure that their sample is representative and that the data collected are reliable and meaningful. Understanding these various data collection techniques is essential for implementing quota sampling effectively. It allows researchers to select the most appropriate method based on their research objectives, resources, and population characteristics, thereby enhancing the validity and reliability of their findings. By leveraging these methods judiciously, researchers can gather high-quality data that accurately represent the population of interest, making quota sampling a powerful tool in social sciences and market research.
Advantages and Limitations of Quota Sampling
Quota sampling is a widely used method in research studies, offering several advantages while also presenting some limitations. This article delves into the multifaceted nature of quota sampling, exploring its benefits, potential biases, and real-world applications. On one hand, quota sampling provides significant benefits in research studies by allowing researchers to ensure that their sample is representative of the population's key characteristics, thereby enhancing the generalizability of findings. However, it is crucial to acknowledge the potential biases and errors that can arise from this method, such as the risk of interviewer bias and the lack of randomness in selection. Additionally, understanding real-world applications and examples of quota sampling can provide valuable insights into its practical utility. By examining these aspects, researchers can make informed decisions about when and how to use quota sampling effectively. This article will first explore the benefits of quota sampling in research studies, highlighting its ability to streamline data collection and improve sample representativeness.
Benefits in Research Studies
In the context of research studies, particularly those employing quota sampling, the benefits are multifaceted and significant. Quota sampling, a method where participants are selected to ensure that the sample represents the major characteristics of the population, offers several advantages that enhance the quality and reliability of research findings. One of the primary benefits is **cost-effectiveness**; compared to other sampling methods like random sampling, quota sampling can be less expensive and time-consuming. This is because researchers do not need to exhaustively list every member of the population or use complex randomization techniques. Instead, they can focus on recruiting participants who fit specific demographic criteria, which can be done more efficiently. Another key benefit is **representativeness**. Quota sampling ensures that the sample includes a diverse range of participants that mirror the broader population in terms of key characteristics such as age, gender, income level, and education. This helps in generalizing the findings to the larger population with greater confidence. For instance, in market research, quota sampling can provide insights into consumer behavior across different demographic segments, allowing businesses to tailor their strategies more effectively. **Practicality** is another significant advantage. In many cases, especially when dealing with hard-to-reach populations or sensitive topics, quota sampling can be more feasible than other methods. It allows researchers to target specific groups without the need for extensive resources or complex logistics. This practicality makes it an attractive option for studies with limited budgets or tight timelines. Moreover, quota sampling can offer **faster data collection**. Since researchers know exactly which demographic groups they need to target, they can streamline their recruitment process. This efficiency is crucial in fast-paced research environments where timely data collection is paramount. Additionally, **improved data quality** can be achieved through quota sampling. By ensuring that the sample includes participants from diverse backgrounds, researchers can gather more comprehensive and nuanced data. This diversity helps in identifying patterns and trends that might be overlooked in less representative samples. However, it is important to note that while these benefits are substantial, they must be balanced against the limitations of quota sampling. For example, the lack of randomness in participant selection can introduce biases and reduce the generalizability of findings. Despite these limitations, the benefits of quota sampling make it a valuable tool in many research contexts, particularly when combined with other methodologies to enhance overall study validity. By leveraging these advantages, researchers can conduct high-quality studies that provide meaningful insights into various phenomena, ultimately contributing to a deeper understanding of the world around us.
Potential Biases and Errors
When discussing the advantages and limitations of quota sampling, it is crucial to address the potential biases and errors inherent in this method. Quota sampling, which involves selecting participants to match predetermined demographic characteristics of the population, can be prone to several biases that may compromise the accuracy and reliability of the results. One significant bias is **selection bias**, where the researcher's subjective judgment in choosing participants within each quota can lead to an unrepresentative sample. For instance, if researchers are more likely to select individuals who are easily accessible or cooperative, this could skew the sample towards those who are more sociable or available, rather than a true representation of the population. Another potential error is **non-response bias**, where certain groups within the quotas may be less likely to participate or respond to surveys. This can occur if certain demographics are harder to reach or less inclined to engage in research studies, leading to underrepresentation and potentially misleading conclusions. Additionally, **social desirability bias** can also affect quota sampling if participants provide answers they believe are socially acceptable rather than their true opinions, further distorting the data. Moreover, quota sampling lacks the randomization element present in probability sampling methods, which can lead to **sampling error**. Without random selection, it becomes challenging to generalize findings from the sample to the larger population with confidence. This limitation is exacerbated by the fact that quota sampling does not provide a clear estimate of sampling error, making it difficult to assess the reliability of the results. Furthermore, **interviewer bias** can also play a role if researchers or interviewers inadvertently influence participant responses through their interactions or the way questions are posed. This can result in biased data that reflects more about the interviewer's perceptions than the actual attitudes and behaviors of the population. To mitigate these biases and errors, researchers must employ rigorous methods to ensure that participants within each quota are selected systematically and without bias. This might involve using multiple recruitment strategies, ensuring interviewer training to minimize interviewer bias, and implementing data validation techniques to check for consistency and accuracy. Despite these challenges, when executed carefully and with awareness of these potential pitfalls, quota sampling can still provide valuable insights into specific populations and demographics. In conclusion, while quota sampling offers several advantages such as cost-effectiveness and ease of implementation, it is essential to be aware of and address the potential biases and errors that can arise. By understanding these limitations and taking steps to minimize them, researchers can enhance the validity and reliability of their findings, ultimately contributing to more accurate and informative research outcomes.
Real-World Applications and Examples
Quota sampling, a method where the sample is selected to match the population in terms of certain characteristics, has numerous real-world applications that highlight both its advantages and limitations. One of the most significant advantages of quota sampling is its ability to ensure representativeness in market research. For instance, in consumer goods marketing, companies often use quota sampling to gather insights from diverse demographic groups. A cosmetics company might use quota sampling to ensure that their survey includes equal numbers of men and women, different age groups, and various ethnic backgrounds, thereby providing a comprehensive understanding of consumer preferences. This approach helps in tailoring products and marketing strategies to meet the needs of a broad customer base. In social sciences, quota sampling is frequently employed in studies that aim to understand public opinion or behavior. For example, political polls often use quota sampling to ensure that the sample reflects the broader population's demographic makeup, such as age, gender, income level, and geographic location. This method allows researchers to make more accurate predictions about election outcomes or public sentiment on key issues. However, one of the limitations of quota sampling is the potential for interviewer bias. Interviewers may select participants who are more accessible or cooperative rather than strictly adhering to the quota criteria, which can skew the results. In healthcare research, quota sampling can be used to study disease prevalence and treatment outcomes across different demographic groups. For instance, a study on diabetes management might use quota sampling to ensure that the sample includes equal numbers of participants from urban and rural areas, as well as different socioeconomic statuses. This helps in identifying disparities in healthcare access and outcomes, which can inform policy decisions and resource allocation. However, another limitation is that quota sampling does not guarantee randomness within each stratum, which can lead to biased results if the selection process is not rigorously controlled. In educational research, quota sampling can help in understanding student performance and educational outcomes across various demographic segments. A study on academic achievement might use quota sampling to include students from different socio-economic backgrounds, ethnicities, and school types (public vs. private). This ensures that the findings are representative of the broader student population, enabling educators and policymakers to develop targeted interventions. Despite these advantages, quota sampling lacks the statistical rigor of probability sampling methods like random sampling, which can limit the generalizability of findings. Overall, while quota sampling offers a practical and efficient way to achieve representativeness in various fields, it is crucial to be aware of its limitations. By understanding these limitations—such as potential interviewer bias and lack of randomness within strata—researchers can take steps to mitigate them and ensure that their findings are as accurate and reliable as possible. Despite these challenges, the real-world applications of quota sampling underscore its value in providing valuable insights that can inform decision-making across diverse sectors.