What Is A T5
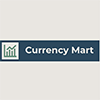
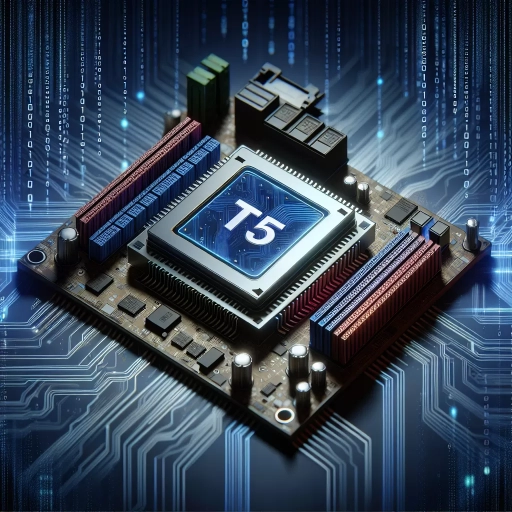
In the realm of artificial intelligence, particularly within natural language processing, the term "T5" has emerged as a significant milestone. T5, or Text-to-Text Transfer Transformer, represents a groundbreaking model that has revolutionized how machines understand and generate human language. This innovative technology is not just a tool but a comprehensive framework that encompasses various facets of AI research. To fully grasp the implications of T5, it is essential to delve into its core components, technical intricacies, and real-world applications. This article will provide an in-depth exploration of T5, starting with an **Introduction to T5**, where we will define what T5 is and its foundational principles. We will then dive into the **Technical Details of T5**, examining the architectural nuances and computational aspects that make this model so powerful. Finally, we will discuss the **Applications and Impact of T5**, highlighting how this technology is transforming industries and enhancing our daily interactions with AI systems. By understanding these three key aspects, readers will gain a comprehensive insight into the world of T5 and its profound influence on modern technology. Let us begin by exploring what T5 is in our next section, **Introduction to T5**.
Introduction to T5
In the realm of natural language processing, few models have garnered as much attention and acclaim as the T5 (Text-to-Text Transfer Transformer). This revolutionary model has redefined the landscape of AI-driven text generation and understanding, offering unparalleled versatility and performance. To fully appreciate the significance of T5, it is essential to delve into its core components. First, understanding the **Definition and Purpose** of T5 provides insight into its design philosophy and intended applications. Next, exploring the **Historical Context and Development** sheds light on the evolutionary journey that led to its creation, highlighting key milestones and innovations. Finally, examining the **Key Features and Innovations** reveals the technological advancements that set T5 apart from its predecessors. By grasping these fundamental aspects, readers can gain a comprehensive understanding of what makes T5 a groundbreaking achievement in AI research. This article aims to provide an in-depth **Introduction to T5**, guiding readers through these critical dimensions to appreciate the full scope of this transformative technology.
Definition and Purpose
**Definition and Purpose** The T5, or Text-to-Text Transfer Transformer, is a cutting-edge AI model designed by Google Research that revolutionizes the way we approach natural language processing tasks. At its core, the T5 model is a transformer-based architecture that treats every NLP task as a text-to-text problem, simplifying the complexity of various tasks into a unified framework. This innovative approach allows for greater flexibility and efficiency in handling diverse NLP challenges, from translation and summarization to question answering and text generation. The primary purpose of the T5 model is to streamline the process of training and fine-tuning AI systems for multiple NLP tasks. By converting all tasks into a text-to-text format, T5 eliminates the need for task-specific architectures, thereby reducing the overhead associated with developing and maintaining separate models for different applications. This unification enables researchers and developers to leverage a single, robust model that can be easily adapted to various scenarios, making it an invaluable tool for both academic research and practical applications. One of the key strengths of the T5 model lies in its ability to generalize across tasks. By training on a vast corpus of text data, T5 develops a deep understanding of linguistic structures and semantic relationships, which it can then apply to a wide range of NLP tasks. This generalizability not only enhances performance but also facilitates transfer learning, where knowledge gained from one task can be seamlessly transferred to another, thereby accelerating the development process. Moreover, the T5 model's architecture is highly scalable, allowing it to be fine-tuned for specific domains or tasks with relative ease. This scalability is crucial in real-world applications where models need to be tailored to meet the unique requirements of different industries or use cases. For instance, in healthcare, a T5 model can be fine-tuned to generate medical summaries or answer clinical questions with high accuracy, while in customer service, it can be adapted to handle customer inquiries and provide personalized responses. In summary, the T5 model's definition as a text-to-text transformer and its purpose of unifying NLP tasks into a single framework make it a powerful tool for advancing the field of natural language processing. Its ability to generalize across tasks, scalability, and ease of adaptation position it as a cornerstone for future AI research and applications, offering unparalleled potential for enhancing human-machine interaction and automating complex linguistic tasks. As we delve deeper into the capabilities and applications of the T5 model, it becomes clear that this technology is poised to significantly impact how we interact with and utilize AI in various aspects of our lives.
Historical Context and Development
The development of T5, or Text-to-Text Transfer Transformer, is deeply rooted in the historical context of natural language processing (NLP) and machine learning advancements. The journey began with the early days of NLP, where rule-based systems and statistical models were the norm. However, these approaches were limited by their inability to capture the nuances and complexities of human language. The advent of deep learning techniques in the late 2000s marked a significant shift, with neural networks demonstrating unprecedented capabilities in processing and understanding natural language. One pivotal moment was the introduction of the Transformer architecture in 2017 by Vaswani et al., which revolutionized NLP by leveraging self-attention mechanisms to handle sequential data more effectively than traditional recurrent neural networks (RNNs). This innovation paved the way for subsequent models like BERT (Bidirectional Encoder Representations from Transformers), introduced by Google in 2018, which further enhanced language understanding through pre-training on large-scale text datasets. Building on these foundations, the T5 model was introduced in 2020 by Colin Raffel et al. from Google Research. T5 unified various NLP tasks under a single framework by converting all text-based tasks into a text-to-text format. This uniformity allowed for more efficient training and better generalization across different tasks such as translation, summarization, and question answering. The model's architecture leveraged an encoder-decoder structure similar to the Transformer but with significant improvements in pre-training objectives and fine-tuning strategies. T5's historical context is also influenced by the availability of large-scale computing resources and extensive datasets like Common Crawl and Wikipedia. These resources enabled researchers to train models on vast amounts of data, leading to substantial improvements in performance. Additionally, advancements in optimization techniques and parallel computing facilitated faster training times and better scalability. The impact of T5 extends beyond its technical contributions; it has democratized access to advanced NLP capabilities by providing pre-trained models that can be fine-tuned for specific tasks with minimal computational resources. This has opened up new avenues for research and practical applications across various industries, from customer service chatbots to content generation tools. In summary, T5's development is a culmination of decades of research in NLP and machine learning, building upon groundbreaking architectures like the Transformer and BERT. Its innovative approach to unifying NLP tasks under a single text-to-text framework has set a new standard for versatility and performance in the field. As NLP continues to evolve, T5 stands as a testament to the power of collaborative research and technological advancements in driving forward our ability to understand and interact with human language.
Key Features and Innovations
The T5, or Transformer 5, represents a significant leap in natural language processing (NLP) technology, introducing several key features and innovations that set it apart from its predecessors. At its core, the T5 is built on the transformer architecture, but it incorporates a unified text-to-text framework that simplifies the way tasks are approached. This framework treats every NLP task as a text-to-text problem, whether it's translation, summarization, or even answering questions. This unification allows for greater flexibility and ease of training, as the model can be fine-tuned for various tasks without needing task-specific architectures. One of the most notable innovations of the T5 is its use of a pre-training objective known as "masked span prediction." Unlike traditional BERT models that use masked token prediction, where only individual tokens are masked and predicted, the T5 masks entire spans of text. This approach helps the model better understand context and relationships between different parts of the text, leading to improved performance across a wide range of NLP tasks. Another key feature is the extensive use of large-scale datasets for pre-training. The T5 was trained on a massive corpus of text data, including but not limited to the Common Crawl dataset and Wikipedia. This extensive pre-training enables the model to capture a broad range of linguistic patterns and nuances, making it highly effective in understanding and generating human-like text. The T5 also benefits from advancements in computational efficiency and scalability. By leveraging distributed training methods and large-scale computing resources, researchers were able to train models with billions of parameters. This scale allows the T5 to capture complex patterns in language that smaller models might miss, resulting in superior performance on benchmarks such as GLUE and SuperGLUE. Furthermore, the T5's architecture is highly modular and adaptable. It supports a variety of model sizes, from smaller versions like T5-Small to larger ones like T5-3B (3 billion parameters), allowing users to choose the right balance between computational resources and performance needs. This modularity makes it accessible for both research and practical applications. In summary, the T5 stands out due to its unified text-to-text framework, innovative pre-training objectives like masked span prediction, extensive large-scale pre-training datasets, computational efficiency improvements, and modular architecture. These features collectively contribute to its superior performance across various NLP tasks, making it a powerful tool for advancing the field of natural language processing.
Technical Details of T5
The T5 (Text-to-Text Transfer Transformer) model, developed by Google, represents a significant advancement in the field of natural language processing (NLP). This innovative architecture leverages a unified text-to-text framework, enabling it to tackle a wide range of NLP tasks with remarkable efficiency and accuracy. To fully appreciate the capabilities of T5, it is essential to delve into its technical details. This article will explore three critical aspects of the T5 model: **Architecture and Model Design**, which examines the structural components and design principles that underpin its performance; **Training Process and Data Requirements**, which discusses the methodologies and datasets used to train the model; and **Performance Metrics and Evaluation**, which assesses how the model's effectiveness is measured and evaluated. By understanding these technical facets, readers will gain a comprehensive insight into what makes T5 a powerful tool in modern NLP. Transitioning seamlessly from these foundational elements, we will introduce the core concepts of T5, providing a thorough understanding of its operational mechanics and applications. **Introduction to T5** will follow, offering a detailed overview of how this model integrates these technical details to achieve state-of-the-art results in various NLP tasks.
Architecture and Model Design
In the realm of architecture and model design, the T5 (Transformer 5) model, a cutting-edge language model developed by Google, offers a unique intersection of technical innovation and creative application. When delving into the technical details of T5, it is crucial to understand how its architectural design influences its performance and versatility. At its core, T5 is built on the transformer architecture, which has revolutionized natural language processing by leveraging self-attention mechanisms to process input sequences in parallel. This allows for more efficient and scalable processing compared to traditional recurrent neural networks. The model's design is centered around a unified framework where all NLP tasks are treated as text-to-text problems, simplifying the training process and enabling the model to generalize across various tasks. This unified approach is facilitated by the use of a text encoder and a text decoder, similar to those found in machine translation models. The encoder processes the input text, while the decoder generates the output text based on the encoded representation. This architecture not only streamlines the training process but also enhances the model's ability to adapt to diverse tasks such as translation, summarization, and question answering. One of the key technical details of T5 is its use of pre-training objectives that are specifically designed to align with downstream tasks. For instance, the model is pre-trained on a mixture of unsupervised and supervised tasks, which helps in developing a robust understanding of language structures and semantics. This pre-training phase is critical as it equips the model with a broad knowledge base that can be fine-tuned for specific applications, thereby improving its performance on a wide range of NLP tasks. Moreover, the scalability of T5's architecture is another significant aspect. The model can be scaled up or down depending on the computational resources available, making it accessible for both research and practical applications. The smaller variants of T5, such as T5-Small and T5-Base, are particularly useful for deployment in resource-constrained environments, while larger variants like T5-Large and T5-3B offer state-of-the-art performance on complex tasks. In summary, the architectural design of T5, with its unified text-to-text framework and scalable transformer architecture, makes it a powerful tool for various NLP tasks. Its ability to generalize across different tasks and adapt to specific applications through fine-tuning underscores its versatility and effectiveness. As a result, T5 stands as a testament to the innovative potential at the intersection of architecture and model design in the field of artificial intelligence.
Training Process and Data Requirements
The training process of T5, a text-to-text transformer model, is a complex and data-intensive endeavor that requires meticulous attention to detail. At its core, T5 is trained using a masked language modeling objective, where some of the input tokens are randomly replaced with a special token, and the model predicts these missing tokens. This approach allows T5 to learn a wide range of tasks by framing them as text-to-text problems, thereby leveraging the power of self-supervised learning. To initiate the training process, large-scale datasets are essential. T5 relies on vast amounts of text data to learn patterns, relationships, and context within language. The model is typically trained on a combination of datasets such as Common Crawl, Wikipedia, and BookCorpus, among others. These datasets provide a diverse range of texts that help in generalizing the model's understanding across different domains and styles. The data requirements for training T5 are substantial. The model is often trained on hundreds of gigabytes of text data, which necessitates significant computational resources. High-performance GPUs or TPUs are typically used to accelerate the training process, which can still take several weeks or even months to complete. Additionally, careful preprocessing of the data is crucial; this includes tokenization, normalization, and possibly filtering out low-quality or irrelevant content. During training, hyperparameters such as batch size, learning rate, and number of epochs need to be finely tuned to optimize performance. The model's architecture also plays a critical role; T5's design includes an encoder-decoder structure with multiple layers of self-attention mechanisms, which allows it to capture long-range dependencies in text effectively. Moreover, the training process involves various techniques to prevent overfitting and ensure robustness. These include dropout regularization, weight decay, and early stopping based on validation performance metrics. Monitoring the model's performance on a validation set during training helps in identifying when the model has reached its optimal point and prevents further overfitting. In summary, the training process of T5 is a sophisticated process that demands extensive computational resources and high-quality datasets. By leveraging large-scale text data and carefully tuning hyperparameters and architectural components, T5 can achieve state-of-the-art performance across a wide array of natural language processing tasks. This comprehensive approach underscores the technical details that make T5 a powerful tool in modern NLP applications.
Performance Metrics and Evaluation
When delving into the technical details of T5, a crucial aspect to consider is the role of performance metrics and evaluation. These metrics are essential for assessing the effectiveness and efficiency of T5, a text-to-text transformer model developed by Google. Performance metrics serve as benchmarks that help in understanding how well the model performs on various tasks, from translation and summarization to question answering and text classification. **Accuracy and F1 Score** are among the primary metrics used to evaluate T5's performance. Accuracy measures the proportion of correct predictions out of total predictions, providing a straightforward indication of the model's reliability. The F1 score, on the other hand, offers a balanced measure of precision and recall, which is particularly useful in tasks where class imbalance might be an issue. For instance, in text classification tasks, an F1 score helps in evaluating both the model's ability to correctly identify positive instances (precision) and its ability to identify all relevant instances (recall). **Perplexity** is another key metric, especially in generative tasks such as language translation or text generation. Perplexity measures how well the model predicts a sample of text; lower perplexity indicates better performance. This metric is crucial for understanding the model's ability to generate coherent and contextually appropriate text. **BLEU Score** is commonly used for evaluating machine translation tasks. It measures the similarity between the generated translation and one or more reference translations, providing insights into the model's ability to produce translations that are both grammatically correct and semantically accurate. **ROUGE Score** is often employed for evaluating summarization tasks. It assesses the overlap between the generated summary and reference summaries, helping to gauge the model's capability to capture essential information from the original text. In addition to these quantitative metrics, qualitative evaluations such as human judgment and user feedback are also vital. These evaluations can provide deeper insights into aspects like readability, coherence, and overall user satisfaction with the generated text. The choice of performance metrics depends on the specific task at hand and the desired outcomes. For example, in question answering tasks, metrics like **Exact Match** and **F1 Score** are used to evaluate how accurately the model can extract relevant information from a given context. By leveraging these performance metrics and evaluation methods, developers can fine-tune T5 to achieve optimal results across various applications. Continuous monitoring and improvement based on these metrics ensure that T5 remains a robust and reliable tool for natural language processing tasks. This comprehensive approach to evaluation not only enhances the model's performance but also fosters trust among users by providing transparent and measurable outcomes.
Applications and Impact of T5
The Transformer-based model T5, short for "Text-to-Text Transfer Transformer," has revolutionized the field of natural language processing (NLP) with its versatility and performance. Developed by Google, T5 has shown remarkable capabilities in handling a wide range of NLP tasks, from text classification and machine translation to question answering and text generation. This article delves into the applications and impact of T5, exploring its efficacy in various **Natural Language Processing Tasks**, its integration into **Real-World Use Cases and Industries**, and the **Future Potential and Research Directions** that this model opens up. By understanding how T5 excels in these areas, we can appreciate its transformative power in enhancing AI-driven solutions. As we navigate through these key aspects, we will also introduce the foundational concepts of T5, setting the stage for a comprehensive understanding of this groundbreaking technology. Let's begin with an **Introduction to T5** to grasp the fundamental principles behind its success.
Natural Language Processing Tasks
Natural Language Processing (NLP) tasks form the backbone of various applications that leverage the transformative power of language understanding and generation. These tasks are pivotal in enabling machines to comprehend, interpret, and generate human language, thereby bridging the gap between human communication and machine intelligence. One of the most versatile and impactful models in this domain is T5 (Text-to-Text Transfer Transformer), which has revolutionized how NLP tasks are approached. At its core, NLP encompasses a wide range of tasks such as **text classification**, where models categorize text into predefined categories; **sentiment analysis**, which involves determining the emotional tone or sentiment behind a piece of text; **named entity recognition**, where specific entities like names, locations, and organizations are identified; and **machine translation**, which translates text from one language to another. T5 excels in these areas by treating all NLP tasks as text-to-text problems, allowing it to generalize across different tasks with remarkable efficiency. T5's versatility is further highlighted in its ability to perform **question answering**, where it can extract relevant information from a given context to answer specific questions, and **summarization**, where it condenses lengthy documents into concise summaries. Additionally, T5's prowess in **language generation** enables it to produce coherent and contextually relevant text, making it an invaluable tool for applications such as chatbots and content creation. The impact of T5 extends beyond these individual tasks. Its ability to be fine-tuned for specific domains has led to significant advancements in fields like **healthcare**, where it can help analyze medical texts and generate summaries of patient records, and **education**, where it can assist in grading assignments and providing feedback. In **customer service**, T5-powered chatbots can handle a wide range of queries with increased accuracy and empathy, enhancing user experience. Moreover, T5's robustness and adaptability make it a crucial component in **research and development**. By facilitating the automation of data preprocessing and analysis, T5 accelerates the research cycle, allowing scientists and researchers to focus on higher-level insights and innovations. This has far-reaching implications for fields such as **social media monitoring**, where T5 can help analyze public sentiment and detect trends, and **content moderation**, where it can identify and flag inappropriate content. In summary, the applications and impact of T5 are profound and multifaceted. By unifying various NLP tasks under a single framework, T5 has not only improved the performance of these tasks but also opened up new avenues for innovation across diverse industries. Its ability to generalize and adapt makes it an indispensable tool in the ever-evolving landscape of natural language processing.
Real-World Use Cases and Industries
The T5 (Text-to-Text Transfer Transformer) model, with its versatile and powerful architecture, has found extensive applications across various industries, showcasing its real-world impact. In **natural language processing (NLP)**, T5 is used for tasks such as text summarization, machine translation, and question answering. For instance, in the **media and publishing industry**, T5 can automatically generate concise summaries of long articles, helping readers quickly grasp key points. Similarly, in **customer service**, T5 can be integrated into chatbots to provide accurate and contextually relevant responses to customer inquiries, enhancing user experience and reducing the workload on human support agents. In the **healthcare sector**, T5's ability to process and generate human-like text makes it invaluable for tasks like clinical note summarization and medical text classification. This helps healthcare professionals quickly review patient histories and make informed decisions. Additionally, in **education**, T5 can assist in generating personalized learning materials and adaptive assessments, tailoring educational content to individual students' needs. The **financial industry** also benefits from T5's capabilities, particularly in sentiment analysis and financial news summarization. By analyzing large volumes of financial news articles, T5 can help investors and analysts gauge market sentiment and make more informed investment decisions. Furthermore, in **marketing and advertising**, T5 can generate compelling product descriptions and personalized marketing content, driving engagement and conversion rates. In **software development**, T5 is used for code generation and documentation. It can help developers by generating boilerplate code or explaining complex code snippets, thereby speeding up the development process. The **legal sector** leverages T5 for contract analysis and document summarization, enabling legal professionals to efficiently review and understand large volumes of legal documents. Moreover, T5's impact extends to **environmental monitoring** where it can be used to analyze and summarize environmental reports, helping policymakers and researchers track changes in environmental conditions more effectively. In **government services**, T5 aids in automating the processing of public records and generating reports, improving transparency and efficiency. Overall, the T5 model's versatility and performance make it a valuable tool across diverse industries, enhancing productivity, accuracy, and decision-making capabilities. Its ability to handle a wide range of NLP tasks has made it an indispensable asset for organizations seeking to leverage AI for operational excellence.
Future Potential and Research Directions
The future potential of T5, a text-to-text transformer model, is vast and multifaceted, offering numerous avenues for research and development. As a foundational technology, T5 has already demonstrated its versatility in various applications such as machine translation, text summarization, and question answering. However, its true potential lies in its ability to be fine-tuned for specialized tasks, making it a powerful tool for domain-specific research. One of the most promising research directions involves exploring T5's capabilities in low-resource languages and domains. By leveraging transfer learning, researchers can adapt T5 to languages and tasks where large datasets are scarce, thereby bridging the gap in linguistic and cultural representation. This could significantly enhance global communication and access to information, particularly in underserved communities. Another area of interest is the integration of T5 with other AI technologies, such as multimodal models that combine text with images or audio. This fusion could lead to innovative applications like automated content creation for multimedia platforms, enhancing user engagement and content diversity. Additionally, incorporating T5 into decision-making systems could improve the accuracy and fairness of AI-driven decisions by providing more nuanced and contextually aware text analysis. The ethical implications of T5 also present a critical research direction. As AI models become more pervasive, understanding and mitigating biases within these systems is crucial. Researchers can use T5 to develop more transparent and explainable AI models, ensuring that the outputs are not only accurate but also fair and unbiased. This could involve developing new evaluation metrics and methodologies to assess the ethical performance of T5 and similar models. Furthermore, the scalability and efficiency of T5 are key areas for future research. As AI models grow in complexity, they require significant computational resources. Investigating ways to optimize T5 for deployment on edge devices or developing more efficient training algorithms could make these powerful models accessible to a broader range of users and applications. In conclusion, the future of T5 is marked by exciting possibilities and challenges. From expanding linguistic inclusivity to integrating with other AI technologies and addressing ethical concerns, the research directions are diverse and impactful. As researchers continue to explore and refine T5, its applications will likely extend beyond current imagination, driving innovation across various sectors and enhancing the lives of people worldwide.