What Is Kd
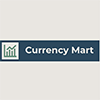
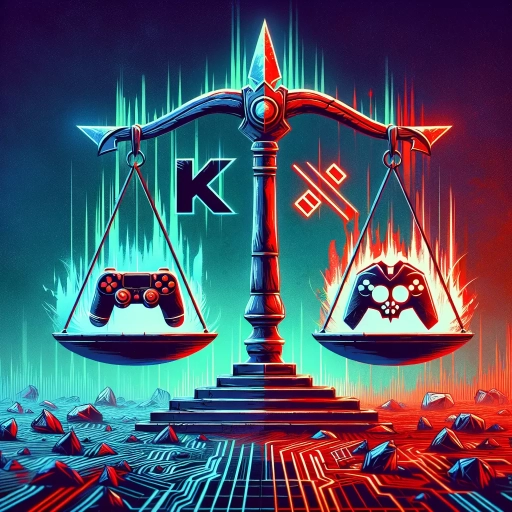
In the realm of data science and machine learning, the concept of Kd (K-dimensional) trees has emerged as a powerful tool for efficient data organization and retrieval. Kd trees are data structures that partition the space in a way that allows for fast search, nearest neighbor search, and range search operations. This article delves into the multifaceted world of Kd trees, exploring their fundamental principles, practical applications, and the benefits they offer along with the challenges they present. We will begin by **Understanding the Basics of Kd**, where we will dissect the structure and construction of Kd trees to grasp how they operate. Following this foundational knowledge, we will examine **Applications and Uses of Kd**, highlighting how these data structures are utilized in various fields such as computer graphics, geographic information systems, and machine learning algorithms. Finally, we will discuss **Benefits, Challenges, and Future Prospects**, analyzing the advantages of using Kd trees while also addressing the complexities and potential future developments in this area. By understanding these aspects, readers will gain a comprehensive insight into the significance and utility of Kd trees in modern data processing. Let's start by **Understanding the Basics of Kd**.
Understanding the Basics of Kd
Understanding the basics of Kd, or "kill-to-death ratio," is a fundamental aspect for anyone interested in competitive gaming, particularly in first-person shooter (FPS) games. This metric is crucial for evaluating player performance and team strategy. To delve into the world of Kd, it is essential to explore three key areas: the definition and origin of Kd, its key components and structure, and its historical context and evolution. The definition and origin of Kd provide a foundational understanding of how this ratio came to be and why it is so important. By examining its roots, we can see how Kd has become a standard measure of skill in gaming communities. Key components and structure are vital for comprehending how Kd is calculated and what factors influence it. This includes understanding the role of kills, deaths, and other metrics that contribute to a player's overall Kd ratio. Historical context and evolution reveal how Kd has adapted over time, reflecting changes in game mechanics, player strategies, and community standards. This perspective helps in appreciating the dynamic nature of Kd and its continued relevance in modern gaming. By exploring these facets, we gain a holistic view of what Kd represents and its significance in the gaming world. Let's begin by examining the definition and origin of Kd to understand its foundational importance.
Definition and Origin
**Definition and Origin** Understanding the concept of "KD" begins with a clear definition and an exploration of its origins. KD, or "Kill Death" ratio, is a statistical measure used primarily in multiplayer video games, particularly in first-person shooter (FPS) and battle royale genres. It quantifies a player's performance by comparing the number of kills they achieve to the number of deaths they experience. The ratio is calculated by dividing the total number of kills by the total number of deaths, resulting in a numerical value that reflects a player's efficiency and skill level. The origin of KD ratios can be traced back to the early days of competitive gaming, where players sought ways to evaluate and compare their performance. As online gaming communities grew, especially with the rise of popular FPS titles like "Quake" and "Counter-Strike" in the late 1990s and early 2000s, players began tracking their in-game statistics to gauge improvement and competitiveness. This practice was further solidified with the advent of modern battle royale games such as "PlayerUnknown's Battlegrounds" (PUBG) and "Fortnite," which introduced large-scale multiplayer environments where individual performance metrics became crucial for team success. The significance of KD ratios extends beyond mere statistical analysis; it also serves as a benchmark for player improvement. A high KD ratio indicates that a player is not only skilled at eliminating opponents but also adept at avoiding death, suggesting strong situational awareness, tactical prowess, and possibly superior reflexes. Conversely, a low KD ratio may indicate areas for improvement such as better map knowledge, improved aim, or enhanced decision-making under pressure. Moreover, KD ratios have become integral to esports culture, where professional players are often judged on these metrics during tournaments and competitive matches. Coaches and analysts use KD ratios to assess team composition, identify strengths and weaknesses, and develop strategies that maximize their team's effectiveness. In this context, understanding one's own KD ratio is essential for self-improvement and contributing positively to team dynamics. In summary, the concept of KD ratios is deeply rooted in competitive gaming history and continues to play a vital role in evaluating player performance across various genres. By grasping what KD means and how it originated, gamers can better appreciate its importance in their own gameplay development and within the broader esports ecosystem. This foundational understanding sets the stage for more advanced discussions on how to improve one's KD ratio through practice, strategy, and continuous learning.
Key Components and Structure
When delving into the basics of Kd (Kd being a term that could refer to various concepts depending on the context, such as kinetic diameter in physics or Kd in biochemistry), it is crucial to understand its key components and structure. In the context of biochemistry, Kd stands for the dissociation constant, a measure of the binding affinity between a ligand and a protein. Here, the structure involves several critical elements: 1. **Ligand-Protein Interaction**: The core component is the interaction between the ligand (a molecule that binds to a protein) and the protein itself. This interaction is reversible and can be described by the law of mass action. 2. **Equilibrium Constant**: The dissociation constant (Kd) is an equilibrium constant that quantifies the strength of this interaction. A lower Kd value indicates higher affinity, meaning the ligand binds more tightly to the protein. 3. **Concentration of Ligand and Protein**: The Kd is defined as the concentration of ligand at which the binding sites on the protein are half-occupied. This concentration is a key structural element in understanding how tightly a ligand binds. 4. **Binding Sites**: Proteins often have specific binding sites where ligands can attach. The structure of these binding sites, including their shape, charge, and hydrophobicity, plays a significant role in determining the Kd. 5. **Thermodynamic Parameters**: The binding process is influenced by thermodynamic parameters such as enthalpy (H), entropy (S), and Gibbs free energy (ΔG). These parameters provide insights into the energetics of the binding process and are integral to understanding the structural aspects of Kd. 6. **Kinetics vs. Thermodynamics**: While Kd is a thermodynamic parameter, understanding the kinetics of binding (on-rate and off-rate) can also provide valuable information about how quickly a ligand binds to or dissociates from a protein. 7. **Experimental Methods**: Various experimental methods such as surface plasmon resonance (SPR), isothermal titration calorimetry (ITC), and fluorescence spectroscopy are used to determine Kd values. Each method has its own structural requirements and limitations. In summary, understanding the key components and structure of Kd involves recognizing the ligand-protein interaction, equilibrium constant, concentration dynamics, binding site specifics, thermodynamic influences, kinetic considerations, and experimental methodologies. These elements collectively provide a comprehensive framework for grasping how Kd reflects the binding affinity in biochemical contexts. By appreciating these structural aspects, researchers can better interpret and utilize Kd values in their studies, enhancing our understanding of biological processes at the molecular level.
Historical Context and Evolution
Understanding the basics of Kd, or kill-death ratio, requires a deep dive into its historical context and evolution. The concept of Kd has its roots in early competitive gaming, particularly in first-person shooter (FPS) games like "Doom" and "Quake," which emerged in the 1990s. During this era, players began to track their performance metrics to gauge skill levels and bragging rights. As online gaming communities grew, so did the importance of quantifying player performance. The early 2000s saw the rise of more sophisticated multiplayer games such as "Counter-Strike" and "Halo," where team-based strategies became crucial. Here, the Kd ratio evolved from a simple metric to a more nuanced indicator of individual contribution within a team dynamic. Players with high Kd ratios were often seen as valuable assets, capable of turning the tide of matches in their favor. The advent of esports in the mid-2000s further solidified the significance of Kd. Professional players and teams began to analyze these metrics rigorously, using them to identify strengths, weaknesses, and areas for improvement. This period also saw the development of more advanced statistical tools and software that could track and analyze player performance in real-time. As gaming technology advanced and new genres emerged, such as battle royale games like "Fortnite" and "PlayerUnknown's Battlegrounds" (PUBG), the Kd ratio adapted to accommodate different gameplay styles. For instance, in battle royale games, survival time and placement became additional factors to consider alongside traditional kill and death counts. Today, the Kd ratio remains a cornerstone metric in competitive gaming but has evolved to include more holistic assessments of player performance. Modern analytics incorporate additional metrics such as assists, objective captures, and overall game impact. This evolution reflects a broader understanding that individual skill is just one aspect of a player's value; teamwork, strategy, and situational awareness are equally important. Moreover, with the proliferation of streaming platforms like Twitch and YouTube, the visibility of professional gamers has increased dramatically. Fans can now watch live gameplay and see real-time statistics displayed on screen, making the Kd ratio a focal point of discussion among viewers. This increased transparency has led to a more informed community where players can learn from each other's strengths and weaknesses. In summary, the historical context and evolution of Kd illustrate how this metric has transformed from a simple measure of individual skill to a multifaceted tool for evaluating player performance within complex team environments. As gaming continues to evolve with new technologies and genres emerging, it is likely that the Kd ratio will continue to adapt, reflecting the ever-changing landscape of competitive gaming. Understanding this evolution is crucial for appreciating the nuances of Kd and its role in modern gaming culture.
Applications and Uses of Kd
The applications and uses of Kd, a versatile and highly sought-after material, span a wide range of industries and aspects of life. From its industrial and commercial uses, where it plays a crucial role in manufacturing and production processes, to its scientific and research applications, where it aids in groundbreaking discoveries and innovations, Kd's utility is multifaceted. Additionally, its presence in everyday life and consumer products underscores its importance in our daily routines. This article will delve into these three key areas, exploring how Kd contributes to industrial efficiency, scientific advancements, and consumer convenience. We will begin by examining the industrial and commercial uses of Kd, highlighting its role in enhancing productivity and driving economic growth. --- **Industrial and Commercial Uses**
Industrial and Commercial Uses
Industrial and commercial uses of KD (Kiln-Dried) wood are multifaceted and critical, reflecting its superior quality and reliability. In the construction industry, KD wood is a preferred choice for building frames, flooring, and roofing due to its stability and resistance to warping. The drying process ensures that the wood has a uniform moisture content, which minimizes shrinkage and expansion, thereby enhancing structural integrity. This makes it ideal for applications where precision and durability are paramount, such as in the fabrication of furniture, cabinetry, and architectural woodwork. In manufacturing, KD wood is used extensively in the production of engineered wood products like plywood, medium-density fiberboard (MDF), and oriented strand board (OSB). These products benefit from the consistent moisture levels of KD wood, which improve their performance and longevity. Additionally, KD wood is favored in the automotive industry for making vehicle parts and components due to its dimensional stability and reduced risk of cracking or splitting. The commercial sector also leverages KD wood for various applications. Retailers and wholesalers prefer KD lumber for shelving and storage solutions because it resists moisture-related damage, ensuring that the structures remain sturdy over time. In the hospitality industry, KD wood is often used in hotel furniture and decor due to its aesthetic appeal and durability. Furthermore, KD wood is a popular choice for packaging materials, particularly for shipping sensitive goods that require protection from moisture. Another significant use of KD wood is in the field of renewable energy. It is increasingly being utilized in the construction of wind turbines and other renewable energy infrastructure due to its strength, stability, and resistance to environmental factors. The precision engineering required in these applications makes KD wood an indispensable material. Moreover, KD wood plays a crucial role in the agricultural sector. It is used in the construction of barns, silos, and other farm structures where exposure to moisture is common. The durability of KD wood ensures that these structures can withstand harsh weather conditions and maintain their integrity over years. In summary, the industrial and commercial uses of KD wood are diverse and vital. Its superior quality, stability, and resistance to moisture make it an essential material across various sectors, from construction and manufacturing to retail and renewable energy. The consistent performance of KD wood under different conditions underscores its importance as a reliable choice for a wide range of applications.
Scientific and Research Applications
Scientific and research applications of Kd (the distribution coefficient) are pivotal in various fields, offering a robust framework for understanding and predicting the behavior of substances in different environments. In environmental science, Kd is crucial for assessing the mobility and fate of contaminants in soil and groundwater. By determining how a contaminant partitions between the solid phase (soil or sediment) and the aqueous phase (water), researchers can predict its potential for leaching into groundwater or being transported through soil layers. This information is essential for risk assessment and remediation strategies, helping scientists and policymakers develop effective measures to mitigate pollution. In the field of pharmacology, Kd plays a significant role in drug development. It helps in understanding the distribution of drugs within the body, particularly how they partition between plasma and tissues. This knowledge is vital for predicting drug efficacy, toxicity, and pharmacokinetics. For instance, a drug with a high Kd value for a specific tissue may indicate higher tissue affinity, which could influence its therapeutic effect and side effects. In materials science, Kd is used to study the adsorption properties of materials, which is critical for developing advanced technologies such as water purification systems and catalysts. By understanding how substances interact with material surfaces, researchers can design more efficient systems for removing pollutants from water or enhancing chemical reactions. In geology, Kd is applied to study the geochemical behavior of elements in natural systems. It helps in understanding the cycling of nutrients and trace elements through ecosystems, which is important for environmental monitoring and management. For example, knowing the Kd values for heavy metals in soil can help in assessing their bioavailability and potential impact on plant growth. Moreover, in nuclear science, Kd values are used to predict the behavior of radionuclides in the environment. This is crucial for assessing the risks associated with nuclear waste disposal and developing strategies for safe storage and remediation of contaminated sites. Overall, the application of Kd in scientific research spans multiple disciplines, providing a fundamental tool for understanding complex interactions between substances and their environments. Its precise measurement and interpretation are essential for advancing our knowledge in various fields and addressing critical environmental, health, and technological challenges. By leveraging Kd, scientists can make more accurate predictions, develop more effective solutions, and contribute significantly to societal well-being.
Everyday Life and Consumer Products
In everyday life, consumer products play a pivotal role in enhancing convenience, comfort, and efficiency. From the moment we wake up to the time we go to bed, we interact with a myriad of products that are designed to make our lives easier. For instance, kitchen appliances like microwaves and refrigerators streamline food preparation and storage, while personal care items such as toothbrushes and shampoos maintain our hygiene. The integration of technology into these products has further elevated their utility; smart home devices like thermostats and lighting systems can be controlled remotely, optimizing energy consumption and creating a more comfortable living environment. Consumer electronics, such as smartphones and laptops, are indispensable tools for communication, entertainment, and productivity. These devices are equipped with advanced software and hardware that enable seamless connectivity and access to a vast array of information. Moreover, wearable technology like fitness trackers and smartwatches monitor our health metrics in real-time, providing valuable insights that help us make informed decisions about our well-being. The impact of consumer products extends beyond individual use; they also influence societal trends and behaviors. For example, the rise of eco-friendly products has led to increased awareness about sustainability, encouraging consumers to adopt more environmentally conscious lifestyles. Similarly, the proliferation of streaming services has revolutionized how we consume media, offering on-demand access to a vast library of content. In the context of applications and uses of KD (Knowledge Discovery), these everyday consumer products present numerous opportunities for data-driven insights. KD can be applied to analyze consumer behavior patterns, helping manufacturers understand market demands and preferences. By leveraging data from smart home devices, KD can optimize energy consumption models and predict maintenance needs. In the realm of personal care, KD can help develop personalized product recommendations based on user preferences and usage habits. Furthermore, KD can enhance the development process of consumer products by identifying trends in user feedback and reviews. This allows companies to refine their products continuously, ensuring they meet evolving consumer needs. The integration of KD into supply chain management can also improve inventory forecasting and logistics, reducing waste and ensuring that products are available when needed. In summary, everyday life is intricately woven with consumer products that not only enhance our daily routines but also offer a wealth of data for analysis. The applications and uses of KD in this domain are vast, ranging from optimizing product design to improving supply chain efficiency. By harnessing the power of KD, companies can create more intuitive, efficient, and sustainable consumer products that better serve the needs of modern society.
Benefits, Challenges, and Future Prospects
The exploration of benefits, challenges, and future prospects in any field is a multifaceted endeavor that requires a comprehensive understanding of its various dimensions. This article delves into the intricacies of these aspects, providing a balanced view that highlights both the advantages and the obstacles. We begin by examining the **Advantages and Benefits in Various Fields**, where we explore how these innovations have positively impacted different sectors, from healthcare and education to technology and environmental sustainability. Following this, we address the **Challenges and Limitations** that hinder progress, including financial constraints, ethical concerns, and operational complexities. Finally, we look towards the **Future Developments and Potential Innovations**, discussing emerging trends and breakthroughs that promise to overcome current barriers and unlock new possibilities. By understanding these three interrelated components, we can gain a deeper insight into the overall trajectory of this field and its potential to shape our future. Let us first delve into the numerous advantages and benefits that have been realized across various fields.
Advantages and Benefits in Various Fields
The advantages and benefits of Knowledge Discovery (KD) are multifaceted and far-reaching, impacting various fields in profound ways. In **business**, KD enables organizations to uncover hidden patterns and trends within their data, leading to better decision-making, enhanced customer segmentation, and improved operational efficiency. For instance, retail companies can use KD to analyze consumer behavior, predict sales trends, and optimize inventory management. This not only boosts revenue but also enhances customer satisfaction by offering personalized services. In **healthcare**, KD plays a crucial role in medical research and patient care. By analyzing large datasets, healthcare professionals can identify risk factors for diseases, develop more accurate diagnostic models, and tailor treatment plans to individual patients. For example, KD can help in the early detection of diseases like cancer by identifying subtle patterns in medical imaging data that might be missed by human eyes. This leads to better patient outcomes and more effective disease management. In **education**, KD can revolutionize the learning experience by providing insights into student performance and learning behaviors. Educational institutions can use KD to develop adaptive learning systems that adjust the curriculum based on individual student needs, thereby improving academic achievement and reducing dropout rates. Additionally, KD can help in identifying at-risk students early on, allowing for timely interventions. The **environmental sector** also benefits significantly from KD. By analyzing data from sensors and satellite imagery, researchers can monitor climate changes, track deforestation, and predict natural disasters such as hurricanes or wildfires. This information is crucial for developing effective conservation strategies and mitigating the impact of environmental disasters. In **finance**, KD helps in fraud detection and risk assessment. Financial institutions can use machine learning algorithms to identify suspicious transactions in real-time, reducing the risk of financial fraud. Moreover, KD can assist in credit scoring by analyzing a wide range of data points beyond traditional credit history, making lending processes more inclusive and accurate. Lastly, in **scientific research**, KD accelerates the discovery process by automating the analysis of large datasets. Scientists can use KD to identify new compounds with potential therapeutic benefits, understand complex biological systems, and explore vast astronomical datasets for signs of life beyond Earth. This not only speeds up the scientific discovery process but also opens up new avenues for research that were previously unexplored. Overall, the benefits of KD are vast and diverse, transforming how we approach challenges across multiple disciplines. As technology continues to evolve, the potential applications of KD will only expand further, driving innovation and progress in various fields.
Challenges and Limitations
While the benefits of Knowledge Discovery (KD) are numerous, it is equally important to acknowledge and address the challenges and limitations that come with this powerful technology. One of the primary challenges is the sheer volume and complexity of data that KD processes. Big data, characterized by its high velocity, variety, and volume, can be overwhelming for traditional data processing tools, leading to issues such as data quality problems, noise, and inconsistencies. This complexity necessitates advanced algorithms and robust computational resources, which can be costly and may not always be accessible to smaller organizations. Another significant limitation is the issue of privacy and security. KD often involves analyzing sensitive and personal data, which raises ethical concerns about data protection and privacy breaches. Ensuring that data is anonymized and securely stored is crucial but can be a daunting task, especially in environments where data is shared across multiple stakeholders. Additionally, the risk of biased algorithms and discriminatory outcomes is a critical challenge. If the training data contains biases, these can be perpetuated and even amplified by KD processes, leading to unfair and unjust outcomes. Furthermore, KD requires specialized skills and expertise, which can be a barrier for many organizations. The process involves not only technical proficiency in areas like machine learning and data mining but also domain-specific knowledge to interpret the results accurately. This skill gap can hinder the widespread adoption of KD, particularly in sectors where such expertise is scarce. Moreover, the interpretability of KD models is another challenge. Many advanced algorithms used in KD are complex and difficult to interpret, making it challenging for stakeholders to understand how decisions are made. This lack of transparency can erode trust in the system and make it harder to identify and correct errors or biases. Lastly, the dynamic nature of data environments poses a continuous challenge. Data is constantly evolving, and KD models must be regularly updated to remain relevant. This requires ongoing investment in model maintenance and retraining, which can be resource-intensive. Despite these challenges, addressing them is crucial for maximizing the benefits of KD. By investing in robust data management systems, ensuring ethical data practices, developing more interpretable models, and fostering a skilled workforce, organizations can mitigate these limitations and fully leverage the potential of KD to drive innovation and improvement.
Future Developments and Potential Innovations
As we delve into the future developments and potential innovations in the realm of Knowledge Discovery (KD), it becomes evident that this field is poised for significant advancements. One of the most promising areas is the integration of Artificial Intelligence (AI) and Machine Learning (ML) technologies. These technologies will enable more sophisticated data analysis, allowing for deeper insights and more accurate predictions. For instance, AI-driven algorithms can automate the process of identifying patterns in large datasets, thereby speeding up the discovery process and reducing human error. Additionally, advancements in Natural Language Processing (NLP) will enhance the ability to extract meaningful information from unstructured data sources such as text documents and social media posts. Another key area of innovation is the development of Big Data analytics. As data volumes continue to grow exponentially, advanced tools and techniques are necessary to handle and analyze these vast datasets efficiently. Future developments in distributed computing frameworks like Apache Spark and Hadoop will facilitate faster processing times and improved scalability, enabling organizations to derive value from their data more quickly. Furthermore, the rise of Edge Computing will allow for real-time data processing at the source of generation, reducing latency and enhancing decision-making capabilities. The Internet of Things (IoT) also plays a crucial role in future KD innovations. With an increasing number of devices connected to the internet, IoT generates a massive amount of data that can be leveraged for knowledge discovery. Advanced IoT analytics platforms will help organizations extract valuable insights from sensor data, leading to improved operational efficiency and new business opportunities. For example, in healthcare, IoT devices can monitor patient health in real-time, enabling early detection of diseases and personalized treatment plans. Moreover, advancements in Quantum Computing hold immense potential for accelerating complex computations involved in KD. Quantum algorithms like Shor's algorithm and Grover's algorithm could significantly speed up certain types of data analysis tasks compared to classical computers. This could lead to breakthroughs in fields such as cryptography, optimization problems, and machine learning models. However, these innovations also come with challenges. Ensuring data privacy and security remains a top concern as more sensitive information is processed and analyzed. Developing robust ethical frameworks and regulatory standards will be essential to protect individual rights while fostering innovation. Additionally, addressing the skills gap in AI and ML will be critical; organizations must invest in training programs to equip their workforce with the necessary skills to leverage these technologies effectively. Despite these challenges, the future prospects for KD are highly promising. As these technologies mature and become more accessible, they will drive significant improvements across various industries such as healthcare, finance, and manufacturing. The ability to uncover hidden patterns and relationships within vast datasets will lead to better decision-making processes, improved operational efficiencies, and the creation of new products and services. Ultimately, the convergence of AI, ML, Big Data analytics, IoT, and Quantum Computing will transform the landscape of knowledge discovery, enabling organizations to derive unprecedented value from their data assets.