What Is Mu In Statistics
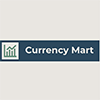
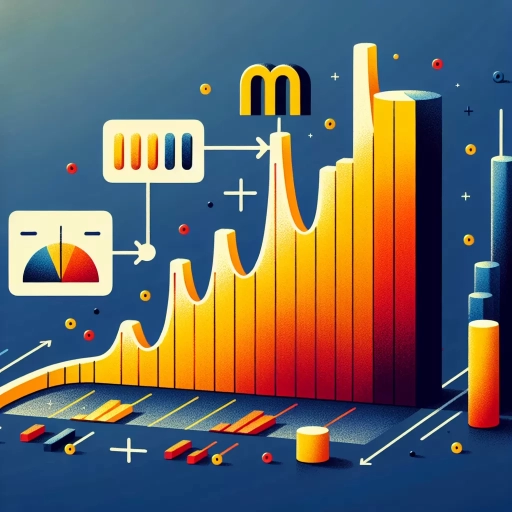
In the realm of statistics, the concept of "mu" (μ) plays a pivotal role in understanding and analyzing data. Mu, or the population mean, is a fundamental parameter that represents the average value of a population. This article delves into the significance of mu, exploring its conceptual underpinnings, practical applications, and real-world implications. We begin by **Understanding the Concept of Mu in Statistics**, where we will dissect the definition and importance of mu in statistical analysis. Next, we will examine **Applications of Mu in Statistical Analysis**, highlighting how mu is used in various statistical methods and models. Finally, we will discuss **Practical Implications and Real-World Examples of Mu**, illustrating how this concept influences decision-making across diverse fields. By grasping the essence of mu, readers will gain a deeper insight into statistical analysis and its far-reaching applications. Let us start by understanding the foundational concept of mu in statistics.
Understanding the Concept of Mu in Statistics
Understanding the concept of mu in statistics is crucial for any data analyst or researcher. Mu, often denoted as μ, represents the population mean in statistical analysis. To fully grasp this concept, it is essential to delve into three key areas: **Definition and Notation**, **Historical Context and Development**, and **Mathematical Representation**. Firstly, understanding the **Definition and Notation** of mu is fundamental. This involves recognizing how mu is used to represent the average value of a population, distinguishing it from sample means. Secondly, exploring the **Historical Context and Development** of mu provides insight into its origins and evolution within statistical theory. This historical perspective can enhance appreciation for the role mu plays in modern statistical practices. Lastly, examining the **Mathematical Representation** of mu reveals how it is calculated and applied in various statistical formulas and models. By starting with a clear **Definition and Notation**, we can establish a solid foundation for understanding mu's significance in statistical analysis. This foundational knowledge will then be built upon by exploring its historical context and mathematical underpinnings, ensuring a comprehensive understanding of this critical statistical concept. Therefore, let us begin by defining and notating mu, the cornerstone of population mean representation in statistics.
Definition and Notation
In the context of statistics, the concept of "mu" (μ) is fundamentally tied to the definition and notation of population parameters. **Mu** represents the population mean, which is a central tendency measure of the entire population from which a sample is drawn. This notation is crucial because it distinguishes the population mean from the sample mean, denoted by **x̄** (x-bar). Understanding this distinction is vital for statistical analysis, as it allows researchers to make inferences about the larger population based on sample data. The notation **μ** is derived from the Greek alphabet and is universally recognized in statistical literature. It signifies that we are referring to a parameter of the entire population rather than a statistic derived from a sample. For instance, if we are studying the average height of all adults in a country, **μ** would represent this true average height for the entire population. In contrast, **x̄** would represent the average height calculated from a random sample of adults. The definition of **μ** as the population mean involves summing all values in the population and dividing by the total number of observations. Mathematically, this can be expressed as: \[ \mu = \frac{\sum_{i=1}^{N} X_i}{N} \] where \( X_i \) represents each individual data point in the population and \( N \) is the total number of data points. This precise notation helps in avoiding confusion between population parameters and sample statistics, which is essential for accurate statistical inference. For example, when conducting hypothesis testing or constructing confidence intervals, knowing whether you are dealing with a population parameter (**μ**) or a sample statistic (**x̄**) is critical for interpreting results correctly. In summary, **mu** (μ) is a fundamental notation in statistics that denotes the population mean, distinguishing it from sample means and other statistics. Its accurate use ensures clarity and precision in statistical analysis, enabling researchers to draw reliable conclusions about populations based on sample data. This understanding is pivotal for applying statistical methods effectively and interpreting results meaningfully.
Historical Context and Development
The concept of "mu" in statistics, often denoted as the population mean, has its roots in the historical development of statistical theory and practice. The term "mu" itself is derived from the Greek alphabet and was popularized by statisticians in the late 19th and early 20th centuries. One of the key figures in this historical context is Karl Pearson, a British mathematician and statistician who laid foundational work in modern statistics. Pearson's contributions included the development of the method of moments and the chi-square test, both of which rely on understanding population parameters like mu. In the early days of statistics, researchers were primarily concerned with understanding large datasets and making inferences about entire populations based on sample data. The work of Sir Francis Galton and Ronald Fisher further solidified the importance of mu as a central tendency measure. Fisher's introduction of the concept of a "population" versus a "sample" helped clarify how mu represents an average value for an entire population, as opposed to the sample mean (often denoted as "x-bar"), which is an estimate derived from a subset of that population. The development of statistical inference, particularly hypothesis testing and confidence intervals, also hinges on understanding mu. For instance, when conducting a t-test or z-test, researchers compare their sample mean to the known or hypothesized population mean (mu) to determine if there is a significant difference. This process relies on assumptions about the distribution of data and the accuracy with which sample means approximate the true population mean. In practical applications, knowing mu is crucial for making informed decisions across various fields such as medicine, economics, and social sciences. For example, in clinical trials, understanding the population mean response to a new drug (mu) helps researchers assess its efficacy compared to existing treatments. Similarly, in economics, knowing the average income or expenditure of a population (mu) aids policymakers in designing effective fiscal policies. The historical context and development of mu highlight its significance as a fundamental concept in statistical analysis. From its origins in early statistical theory to its widespread use today, mu remains an essential tool for understanding and interpreting data at both theoretical and practical levels. This understanding is pivotal for advancing knowledge across diverse disciplines and ensuring that statistical methods are applied accurately and meaningfully.
Mathematical Representation
Mathematical representation is a fundamental aspect of understanding statistical concepts, including the notion of "mu" (μ) in statistics. Mu, or the population mean, is a central parameter that describes the average value of a population. Mathematically, it is represented as μ = (Σx_i) / N, where x_i denotes each individual data point and N is the total number of data points in the population. This formula encapsulates the essence of mu by averaging all values to find the central tendency. To delve deeper, consider that statistical analysis often involves working with samples rather than entire populations due to practical constraints. However, understanding mu is crucial because it serves as a benchmark against which sample means can be compared. The mathematical representation of mu allows statisticians to model real-world phenomena using probability distributions such as the normal distribution, where mu is a key parameter alongside sigma (σ), the standard deviation. In practice, mathematical representation of statistical concepts like mu enables precise calculations and predictions. For instance, in hypothesis testing, mu is used to formulate null and alternative hypotheses. The null hypothesis typically states that there is no significant difference between the sample mean and the known population mean (H0: μ = μ0), while the alternative hypothesis posits that there is a difference (H1: μ ≠ μ0). These hypotheses are then tested using statistical tests like t-tests or z-tests, which rely heavily on mathematical representations involving mu. Moreover, mathematical representation facilitates the use of advanced statistical techniques such as regression analysis and time series analysis. In regression models, for example, mu represents the expected value of the dependent variable when all independent variables are set to zero. This baseline expectation is critical for interpreting model coefficients and making predictions. The precision offered by mathematical representation also extends to data visualization. Graphical tools like histograms and box plots can be used to visually represent data distributions around their means (mu). These visual aids help in identifying patterns and outliers, further enhancing our understanding of how closely sample data aligns with population parameters. In summary, the mathematical representation of mu in statistics provides a robust framework for understanding population means and their role in statistical inference. By leveraging these representations, statisticians can conduct rigorous analyses, make informed decisions, and communicate complex ideas clearly. This foundational concept underscores the importance of mathematical rigor in statistical practice and research.
Applications of Mu in Statistical Analysis
In the realm of statistical analysis, the concept of "mu" (μ), or the population mean, plays a pivotal role in various applications. This article delves into three critical areas where mu is essential: Population Mean Estimation, Hypothesis Testing and Confidence Intervals, and Regression Analysis and Modeling. Understanding the population mean is fundamental because it serves as a benchmark for describing the central tendency of a dataset. In Population Mean Estimation, mu is the target parameter that statisticians aim to estimate using sample data, ensuring accurate representation of the population. Hypothesis Testing and Confidence Intervals rely on mu to make inferences about the population, allowing researchers to draw conclusions with a specified level of confidence. Additionally, Regression Analysis and Modeling use mu to establish relationships between variables, predicting outcomes based on the mean behavior of the data. By exploring these applications, this article provides a comprehensive overview of how mu underpins statistical analysis, beginning with the foundational concept of Population Mean Estimation.
Population Mean Estimation
Population mean estimation is a fundamental concept in statistical analysis, particularly when dealing with the parameter μ (mu), which represents the true mean of a population. In practice, it is often impractical or impossible to measure every individual in a population, so statisticians rely on sample data to estimate μ. This process involves selecting a random sample from the population and calculating the sample mean, denoted as \(\bar{x}\). The sample mean serves as an unbiased estimator of the population mean, meaning that over many samples, the average of these sample means will converge to the true population mean. The accuracy of this estimation depends on several factors, including the sample size and the variability within the sample. Larger samples generally provide more precise estimates because they tend to capture more of the population's characteristics. The standard error of the mean (SEM), calculated as \(SEM = \frac{s}{\sqrt{n}}\), where \(s\) is the sample standard deviation and \(n\) is the sample size, quantifies this precision. A smaller SEM indicates a more reliable estimate. In addition to sample size, the distribution of the data also plays a crucial role. For normally distributed data, the Central Limit Theorem (CLT) states that the distribution of sample means will be approximately normal, even if the underlying population distribution is not normal, provided the sample size is sufficiently large. This allows for the use of statistical inference techniques such as confidence intervals and hypothesis testing to make inferences about μ. Confidence intervals are particularly useful for population mean estimation. For example, a 95% confidence interval for μ might be constructed using the formula \(\bar{x} \pm 1.96 \times SEM\), assuming normality and large enough sample sizes. This interval provides a range within which we expect the true population mean to lie with 95% confidence. Hypothesis testing is another application where estimating μ is crucial. For instance, in a t-test or z-test, the null hypothesis often involves a specific value for μ (e.g., \(H_0: \mu = 0\)). By comparing the sample mean to this hypothesized value and considering the standard error, one can determine whether there is sufficient evidence to reject the null hypothesis. In real-world applications, accurate estimation of μ can have significant implications. For instance, in quality control, manufacturers need to ensure that their products meet certain standards; estimating the mean quality metric (e.g., weight or performance) helps in making informed decisions about production processes. Similarly, in healthcare research, estimating the mean response to a new treatment can help in evaluating its efficacy. In conclusion, population mean estimation using μ is a cornerstone of statistical analysis. By understanding how to accurately estimate μ from sample data and considering factors such as sample size and data distribution, researchers and practitioners can make informed decisions across various fields. The applications of these estimations are diverse and critical for advancing knowledge and improving processes in many areas of study.
Hypothesis Testing and Confidence Intervals
Hypothesis testing and confidence intervals are fundamental concepts in statistical analysis, particularly when dealing with the population mean, denoted by μ (mu). **Hypothesis Testing** involves making an educated guess about a population parameter and then testing this guess using sample data. The process begins with formulating a null hypothesis (H0) and an alternative hypothesis (H1). For instance, if we want to determine whether the average height of a population has changed, our null hypothesis might be H0: μ = 175 cm (the known average height), while the alternative hypothesis could be H1: μ ≠ 175 cm. We then collect sample data, calculate a test statistic, and compare it to a critical value or use a p-value to decide whether to reject the null hypothesis. This method helps in making informed decisions about population parameters based on sample data. **Confidence Intervals**, on the other hand, provide a range of values within which the true population parameter is likely to lie. A confidence interval for the population mean (μ) is constructed using sample data and is expressed as an interval with a lower and upper bound. For example, if we are estimating the average height of a population and our sample data yields a mean of 172 cm with a standard deviation of 5 cm, we might construct a 95% confidence interval that ranges from 170 cm to 174 cm. This interval suggests that we are 95% confident that the true population mean lies between these values. Confidence intervals offer a more nuanced understanding of the population parameter by providing a range rather than a single point estimate. Both hypothesis testing and confidence intervals rely heavily on the concept of μ (mu), as they aim to make inferences about this population parameter. In hypothesis testing, μ is the value against which our sample mean is compared, while in confidence intervals, μ is the central value around which our interval is constructed. These methods are crucial in various fields such as medicine, social sciences, and business, where understanding population characteristics is essential for decision-making and policy formulation. By applying these statistical tools effectively, researchers can draw reliable conclusions about population means and other parameters, thereby enhancing the accuracy and reliability of their findings.
Regression Analysis and Modeling
Regression analysis and modeling are fundamental tools in statistical analysis, enabling researchers to understand the relationships between variables and make predictions. At its core, regression analysis involves fitting a model to data to describe the relationship between a dependent variable (the outcome) and one or more independent variables (predictors). This method is crucial for identifying how changes in the independent variables affect the dependent variable, thereby allowing for the estimation of outcomes based on known inputs. In practical applications, regression models are widely used across various fields. For instance, in economics, regression can be used to predict economic indicators such as GDP based on factors like inflation rates, unemployment levels, and consumer spending. In healthcare, regression models help in understanding the impact of different treatments on patient outcomes by controlling for confounding variables. In marketing, regression analysis aids in forecasting sales based on advertising expenditures, pricing strategies, and seasonal trends. The process of building a regression model typically involves several steps: data collection, model specification, estimation of parameters, and validation. The most common type of regression is linear regression, which assumes a linear relationship between the dependent and independent variables. However, other types such as logistic regression (for binary outcomes), polynomial regression (for non-linear relationships), and multiple regression (involving more than one independent variable) are also frequently employed. The coefficient of determination (R-squared) is a key metric in evaluating the goodness of fit for a regression model, indicating the proportion of variance in the dependent variable that is predictable from the independent variable(s). Additionally, statistical tests like the F-test and t-tests are used to assess the significance of the model and its coefficients. Advanced techniques such as generalized linear models (GLMs) and generalized additive models (GAMs) extend traditional linear regression by accommodating different types of response variables and non-linear relationships. Machine learning algorithms like ridge regression, lasso regression, and elastic net regression are also used to handle high-dimensional data and prevent overfitting. In the context of applications of μ (population mean) in statistical analysis, regression models often rely on estimates of μ to construct confidence intervals for regression coefficients or to test hypotheses about population parameters. For example, when conducting hypothesis tests about the slope of a regression line, estimates of μ are used to determine whether there is a significant relationship between variables. Overall, regression analysis and modeling provide powerful tools for understanding complex relationships and making informed decisions across diverse fields. By leveraging these methods, researchers can uncover insights that would otherwise remain hidden, thereby enhancing our understanding of the world around us.
Practical Implications and Real-World Examples of Mu
The practical implications of Mu, a versatile and powerful tool, are far-reaching and multifaceted, impacting various fields in significant ways. In the realm of **Medical Research and Clinical Trials**, Mu enables the development of more accurate and efficient diagnostic tools, streamlining the process of clinical trials and enhancing patient outcomes. In **Business and Economic Studies**, Mu facilitates advanced data analytics, helping companies make informed decisions and optimize their operations. Additionally, in **Social Sciences and Public Policy**, Mu aids in the analysis of complex societal trends, informing policy decisions that can lead to better governance and community welfare. These diverse applications underscore the transformative potential of Mu across different disciplines. As we delve deeper into these practical implications, it becomes clear that Mu's impact is particularly pronounced in **Medical Research and Clinical Trials**, where its precision and speed can significantly advance our understanding of diseases and treatments.
Medical Research and Clinical Trials
Medical research and clinical trials are pivotal components in the advancement of healthcare, offering a structured approach to evaluating the safety and efficacy of new treatments, medications, and medical devices. These trials are meticulously designed to ensure that any new intervention is rigorously tested before it is introduced into clinical practice. The process begins with preclinical studies, where researchers conduct laboratory and animal tests to assess the potential benefits and risks of a new treatment. If these initial studies show promise, the intervention proceeds to clinical trials, which are divided into four phases. **Phase 1** focuses on safety, involving a small group of healthy volunteers or patients to determine the maximum tolerated dose and identify side effects. **Phase 2** evaluates the efficacy and side effects of the treatment in a larger group of patients, typically those with the condition the treatment aims to address. **Phase 3** involves a much larger population to confirm efficacy, monitor side effects, compare the new treatment to commonly used treatments, and collect information that will allow the new treatment to be used safely. Finally, **Phase 4** post-marketing surveillance trials monitor the long-term effects of the treatment in the general population. The practical implications of these trials are profound. For instance, clinical trials have led to the development of vaccines that have nearly eradicated diseases such as polio and measles. They have also enabled the creation of targeted therapies for cancer, significantly improving survival rates for many types of tumors. Moreover, trials have facilitated the approval of medications like antiretrovirals for HIV, transforming a once-fatal disease into a manageable chronic condition. Real-world examples include the COVID-19 vaccine trials conducted during the pandemic. These trials were expedited due to the urgent need for a vaccine, yet they still adhered to rigorous scientific standards. The results were remarkable: multiple vaccines were developed and approved within a year, saving countless lives worldwide. Another example is the development of immunotherapies for melanoma, which have dramatically improved outcomes for patients with this previously aggressive form of cancer. In addition to advancing medical treatments, clinical trials also contribute significantly to our understanding of disease mechanisms and patient outcomes. They provide valuable data that can be analyzed using statistical methods, such as those involving the concept of "mu" (population mean) in statistics. By comparing treatment outcomes across different populations, researchers can identify trends and patterns that might not be apparent otherwise. This statistical analysis is crucial for making informed decisions about healthcare policies and treatment guidelines. In summary, medical research and clinical trials are essential for advancing healthcare by ensuring that new treatments are safe and effective. Their practical implications are evident in the development of life-saving vaccines and medications, and their real-world examples underscore the critical role they play in improving patient outcomes and advancing our understanding of disease.
Business and Economic Studies
In the realm of Business and Economic Studies, understanding statistical concepts such as "mu" (population mean) is crucial for making informed decisions and analyzing market trends. Mu represents the average value of a population, which can be a key metric in various business applications. For instance, in market research, knowing the population mean of customer spending habits can help companies set realistic sales targets and tailor their marketing strategies. In finance, mu is essential for calculating expected returns on investments, allowing portfolio managers to assess risk and optimize asset allocation. Practical implications of mu can be seen in quality control processes. Manufacturers use the population mean to ensure that their products meet quality standards. For example, a car manufacturer might use mu to monitor the average fuel efficiency of their vehicles, ensuring that they meet regulatory requirements and customer expectations. In human resources, understanding the population mean of employee performance metrics can help organizations identify areas for improvement and develop targeted training programs. Real-world examples abound. Walmart, for instance, uses statistical analysis to determine the average sales per store (mu) to allocate resources effectively and predict future sales trends. Similarly, tech giants like Google analyze user behavior data to find the population mean of user engagement metrics, which helps them refine their algorithms and improve user experience. Moreover, in economic studies, mu is vital for policymakers who need to understand the average income levels or unemployment rates within a population. This data helps in formulating policies that address economic disparities and stimulate growth. For example, central banks use population means to set interest rates that balance economic growth with inflation control. In summary, the concept of mu in statistics has profound practical implications across various sectors of business and economics. By understanding and applying this concept, businesses can enhance their decision-making processes, optimize operations, and drive growth, while policymakers can create more effective and targeted economic policies. This underscores the importance of statistical literacy in both academic and professional settings.
Social Sciences and Public Policy
In the realm of Social Sciences and Public Policy, understanding statistical concepts like "mu" (the population mean) is crucial for informed decision-making and policy formulation. Mu serves as a foundational element in statistical analysis, allowing researchers to make inferences about entire populations based on sample data. Here’s how it practically impacts social sciences and public policy: 1. **Policy Evaluation**: When evaluating the effectiveness of social programs, policymakers rely on statistical measures to determine if interventions have a significant impact. For instance, if a new educational initiative aims to improve average test scores, mu helps in establishing a baseline against which the program's success can be measured. By comparing the sample mean of test scores before and after the intervention, policymakers can determine if there has been a statistically significant improvement. 2. **Resource Allocation**: In public health, understanding the population mean (mu) of health metrics such as life expectancy, disease prevalence, or vaccination rates is essential for resource allocation. For example, if a public health campaign aims to increase vaccination rates, knowing the population mean vaccination rate (mu) allows health officials to target areas where rates are below average, thereby optimizing resource use. 3. **Economic Policy**: In economics, mu is used to analyze trends in economic indicators such as unemployment rates, GDP growth, and inflation. Policymakers use these statistics to formulate fiscal and monetary policies. For instance, if the sample mean unemployment rate indicates a higher-than-average rate in a particular region, policymakers might implement job creation programs or economic stimulus packages tailored to that area. 4. **Social Justice**: In addressing social inequalities, researchers often use statistical analysis to identify disparities in outcomes such as income levels, educational attainment, or access to healthcare. By comparing sample means against the population mean (mu), researchers can highlight areas where certain groups are disadvantaged and advocate for targeted policies to address these inequalities. 5. **Data-Driven Governance**: The use of mu in statistical analysis promotes data-driven governance by providing a robust framework for decision-making. It helps policymakers move beyond anecdotal evidence and instead rely on empirical data to formulate policies that are likely to have a positive impact on the broader population. In summary, understanding and applying the concept of mu in statistical analysis is vital for creating effective public policies that are grounded in empirical evidence. By leveraging this statistical tool, policymakers can make more informed decisions that address real-world problems and improve societal outcomes across various domains of social sciences and public policy.