What Is Experimental Probability
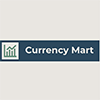
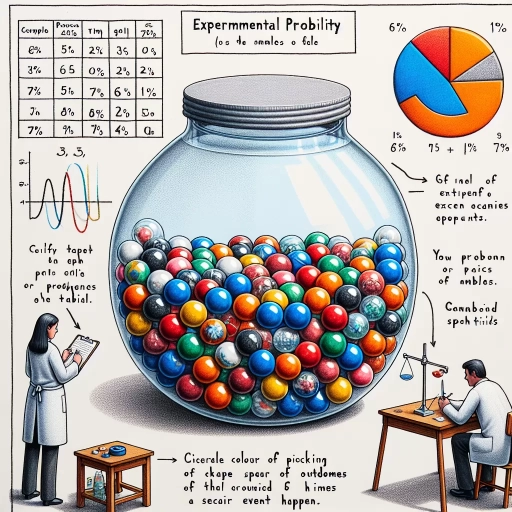
Experimental probability is a fundamental concept in statistics and probability theory, offering a practical approach to understanding the likelihood of events occurring. Unlike theoretical probability, which relies on mathematical models and assumptions, experimental probability is derived from actual observations and experiments. This method involves conducting repeated trials of an event to determine the frequency at which it occurs, providing a more empirical and often more reliable measure of probability. In this article, we will delve into the core aspects of experimental probability, starting with **Understanding Experimental Probability**, where we explore the definition and principles behind this concept. We will then move on to **Calculating Experimental Probability**, where we discuss the methods and formulas used to compute probabilities based on experimental data. Finally, we will examine **Examples and Case Studies** to illustrate how experimental probability is applied in real-world scenarios. By the end of this article, you will have a comprehensive understanding of how experimental probability works and its significance in various fields. Let's begin by understanding the foundational aspects of experimental probability.
Understanding Experimental Probability
Understanding experimental probability is a crucial concept in statistics and probability theory, offering insights into the likelihood of events based on actual outcomes. This article delves into three key aspects: the definition and concept of experimental probability, its distinction from theoretical probability, and its real-world applications. Experimental probability is derived from empirical data, reflecting the frequency of an event occurring in repeated trials. Unlike theoretical probability, which is based on mathematical models and assumptions, experimental probability provides a practical, data-driven approach. This distinction is vital for understanding the reliability and applicability of probability measures in various contexts. Real-world applications of experimental probability are diverse, ranging from quality control in manufacturing to risk assessment in finance. By exploring these facets, readers will gain a comprehensive understanding of how experimental probability operates and its significance in everyday decision-making. Let's begin by examining the definition and concept of experimental probability, which forms the foundation of this discussion.
Definition and Concept
**Definition and Concept** Experimental probability is a fundamental concept in statistics that quantifies the likelihood of an event occurring based on repeated trials or experiments. Unlike theoretical probability, which relies on mathematical models and assumptions, experimental probability is derived from actual observations and data. It is defined as the ratio of the number of times an event occurs to the total number of trials conducted. For instance, if you flip a coin 100 times and observe heads 55 times, the experimental probability of getting heads is 55/100 or 0.55. This approach allows for a more practical understanding of probability by reflecting real-world outcomes rather than theoretical expectations. In essence, experimental probability captures the empirical nature of chance events, making it a crucial tool in fields such as science, engineering, and social sciences where experiments are common. It helps researchers and analysts to understand patterns and trends that may not be immediately apparent through theoretical calculations alone. By conducting multiple trials and analyzing the results, one can refine their understanding of how likely an event is to occur in real-world scenarios. This method also highlights the importance of sample size; larger samples generally provide more reliable estimates of experimental probability. Moreover, experimental probability can be used to validate or challenge theoretical probabilities. For example, if the theoretical probability of rolling a six on a fair die is 1/6 (approximately 0.17), repeated experiments can confirm whether this holds true in practice. If the experimental probability significantly deviates from the theoretical value, it may indicate biases or flaws in the experiment setup. Understanding experimental probability is essential for making informed decisions based on empirical evidence rather than assumptions. It fosters a deeper appreciation for the role of chance and variability in real-world phenomena, enabling better prediction and risk assessment in various domains. By combining theoretical frameworks with empirical data, researchers can develop more robust models that accurately reflect real-world probabilities, thereby enhancing the reliability and applicability of their findings. In summary, experimental probability offers a practical and data-driven approach to understanding chance events, complementing theoretical probability and providing valuable insights into the nature of randomness and uncertainty.
Distinction from Theoretical Probability
Understanding the distinction between theoretical probability and experimental probability is crucial for grasping the fundamental concepts of probability theory. Theoretical probability, often referred to as classical probability, is based on the number of favorable outcomes divided by the total number of possible outcomes in a given scenario. This approach assumes that all outcomes are equally likely and can be calculated using the formula \( P(A) = \frac{\text{Number of favorable outcomes}}{\text{Total number of possible outcomes}} \). For instance, in a fair six-sided die, the theoretical probability of rolling a 4 is \( \frac{1}{6} \) because there is one favorable outcome (rolling a 4) out of six possible outcomes. In contrast, experimental probability is derived from actual observations and experiments. It is calculated by dividing the number of times an event occurs by the total number of trials conducted. This method is particularly useful when the outcomes are not equally likely or when the theoretical probabilities are unknown. For example, if you roll a die 100 times and observe that the number 4 appears 17 times, the experimental probability of rolling a 4 would be \( \frac{17}{100} \) or 0.17. Experimental probability provides a real-world approximation that can be refined with more trials, ideally converging towards the theoretical probability as the number of trials increases. The distinction between these two types of probabilities highlights their different applications and limitations. Theoretical probability is ideal for situations where all outcomes are known and equally likely, offering a precise calculation based on mathematical principles. However, it may not accurately reflect real-world scenarios where outcomes might be influenced by various factors such as bias or external conditions. Experimental probability, on the other hand, offers a practical approach to understanding probabilities in real-world contexts but requires multiple trials to achieve reliable results. In summary, while theoretical probability provides a theoretical framework based on equal likelihood of outcomes, experimental probability offers a pragmatic approach grounded in empirical data. Understanding this distinction is essential for applying probability concepts effectively in various fields such as statistics, engineering, and decision-making processes. By recognizing the strengths and limitations of each type of probability, one can better navigate complex problems involving chance and uncertainty.
Real-World Applications
Understanding experimental probability is crucial for its numerous real-world applications, which span across various fields. In **medicine**, experimental probability helps in clinical trials to determine the efficacy and safety of new drugs. For instance, if a trial involves administering a new medication to a group of patients and observing the outcomes, the experimental probability of success can be calculated by dividing the number of successful outcomes by the total number of patients treated. This data is vital for regulatory approvals and informed decision-making. In **engineering**, experimental probability is used in reliability testing to predict the lifespan and failure rates of components. Manufacturers conduct experiments where components are subjected to stress tests, and the probability of failure is calculated based on the observed data. This information is essential for designing more reliable systems and scheduling maintenance. In **finance**, experimental probability plays a key role in risk assessment. Financial analysts use historical data to calculate the probability of certain market events occurring, such as stock price fluctuations or default rates on loans. These probabilities help in portfolio management and risk mitigation strategies. In **environmental science**, experimental probability aids in predicting weather patterns and natural disasters. By analyzing historical weather data, meteorologists can estimate the likelihood of events like hurricanes or droughts, enabling better preparedness and response planning. In **quality control**, manufacturing companies use experimental probability to ensure product quality. By conducting random sampling and testing products, they can determine the probability of defects, which helps in setting quality standards and improving production processes. Moreover, in **sports analytics**, teams use experimental probability to predict game outcomes and player performance. For example, analyzing past game data can help estimate the probability of a team winning or a player scoring a certain number of points. In **transportation**, experimental probability is applied in traffic management and accident risk assessment. By studying traffic patterns and accident data, urban planners can optimize traffic flow and reduce accident risks. Lastly, in **education**, understanding experimental probability helps students develop critical thinking skills through hands-on experiments. Conducting simple experiments like coin tosses or dice rolls allows students to grasp the concept of probability in a practical manner. These diverse applications highlight the importance of experimental probability in making informed decisions across various sectors, demonstrating its relevance and utility in real-world scenarios.
Calculating Experimental Probability
Calculating experimental probability is a fundamental concept in statistics that helps us understand the likelihood of an event occurring based on actual data. This process is crucial in various fields, including science, engineering, and social sciences, where accurate predictions are essential. To delve into the intricacies of experimental probability, it is important to follow a systematic approach. Our article will guide you through a **Step-by-Step Process** to ensure you grasp the methodology accurately. Additionally, we will explore the **Use of Data and Statistics** to illustrate how real-world data can be utilized to calculate experimental probability. It is also vital to be aware of **Common Mistakes to Avoid**, as these can significantly impact the accuracy of your results. By understanding these key aspects, you will be well-equipped to calculate experimental probability with confidence. Let's begin by breaking down the **Step-by-Step Process** involved in this calculation.
Step-by-Step Process
When calculating experimental probability, it is crucial to follow a systematic step-by-step process to ensure accuracy and clarity. Here’s a detailed breakdown of the steps involved: 1. **Define the Experiment**: Clearly outline the experiment or event you are analyzing. This could be anything from flipping a coin to rolling a die or drawing cards from a deck. 2. **Identify the Outcomes**: List all possible outcomes of the experiment. For example, if you are flipping a coin, the outcomes are heads and tails. 3. **Conduct the Experiment**: Perform the experiment multiple times to gather data. The more trials you conduct, the more reliable your results will be. 4. **Record the Results**: Keep a record of each outcome for each trial. This can be done using a table or any other method that helps you track the data efficiently. 5. **Count the Number of Trials**: Note the total number of trials conducted during the experiment. 6. **Count the Number of Successful Outcomes**: Identify and count how many times the specific outcome you are interested in occurred. 7. **Calculate the Experimental Probability**: Use the formula for experimental probability: \( \text{Experimental Probability} = \frac{\text{Number of Successful Outcomes}}{\text{Total Number of Trials}} \). 8. **Interpret the Results**: Analyze and interpret your findings. Compare your experimental probability with theoretical probabilities if applicable to see how close they are. 9. **Repeat and Validate**: If possible, repeat the experiment several times to validate your results and ensure consistency. By following these steps meticulously, you can accurately calculate experimental probability and gain valuable insights into the likelihood of different outcomes in various scenarios. This method not only helps in understanding real-world phenomena but also provides a practical approach to probability theory.
Use of Data and Statistics
In the realm of experimental probability, the use of data and statistics is paramount for deriving accurate and reliable outcomes. Experimental probability, which is determined through repeated trials or experiments, heavily relies on the collection, analysis, and interpretation of data. Here, statistics play a crucial role in quantifying the likelihood of an event occurring based on empirical evidence. When conducting experiments to calculate experimental probability, it is essential to gather a sufficient amount of data to ensure that the results are representative and reliable. This involves setting up controlled conditions, executing multiple trials, and meticulously recording the outcomes. For instance, if you are calculating the probability of flipping a coin landing on heads, you would conduct numerous coin flips and record each result. The more trials you perform, the closer your experimental probability will be to the theoretical probability. Data analysis is the next critical step. By tallying the number of successful outcomes (e.g., heads) and dividing it by the total number of trials, you obtain the experimental probability. This calculation provides a numerical value that represents how likely an event is to occur based on your experiment. For example, if out of 100 coin flips, 55 result in heads, the experimental probability of flipping a head would be 0.55 or 55%. Statistics also help in assessing the reliability of these findings. Measures such as mean, median, mode, and standard deviation can provide insights into the distribution of your data. Additionally, statistical tools like confidence intervals can be used to estimate how close your experimental probability is to the true population parameter. Moreover, data visualization techniques such as histograms or bar charts can help in understanding patterns within your data set. These visual aids make it easier to identify any biases or anomalies that might affect your calculations. In conclusion, the use of data and statistics is indispensable for calculating experimental probability accurately. By collecting robust data sets, analyzing them meticulously, and employing statistical methods to interpret results, researchers can derive reliable probabilities that reflect real-world phenomena. This approach not only enhances the validity of experimental findings but also provides a solid foundation for making informed decisions in various fields ranging from science and engineering to economics and social sciences.
Common Mistakes to Avoid
When calculating experimental probability, it is crucial to avoid several common mistakes that can lead to inaccurate results. One of the most prevalent errors is **miscounting trials**. Ensure that you accurately record the total number of trials conducted, as this forms the denominator in your probability calculation. Another mistake is **inconsistent definitions of success**; it is essential to clearly define what constitutes a successful outcome and maintain this definition throughout all trials to ensure consistency. **Ignoring the independence of events** can also skew results. Each trial should be independent, meaning the outcome of one trial does not affect the outcome of another. If trials are not independent, this can lead to biased probabilities. Additionally, **failing to account for all possible outcomes** can result in an incomplete picture of the experimental probability. Make sure to consider every possible outcome when calculating the probability. **Rounding errors** can accumulate and significantly impact your final probability value. It is advisable to keep as many decimal places as possible during intermediate calculations and only round at the final step if necessary. Furthermore, **not repeating experiments sufficiently** can lead to unreliable results due to small sample sizes. Increasing the number of trials generally improves the accuracy of experimental probability estimates. **Misinterpreting results** is another common pitfall. Experimental probability is an empirical measure and may not always align with theoretical probabilities due to random variation. It's important to understand that experimental probabilities are approximations and may vary from one set of trials to another. Lastly, **neglecting to check for biases** in your experimental setup can introduce systematic errors. Ensure that your method of conducting trials does not inherently favor certain outcomes over others. By avoiding these common mistakes, you can ensure that your calculations of experimental probability are reliable and accurate, providing a solid foundation for further analysis and decision-making.
Examples and Case Studies
When exploring the realm of examples and case studies, it is crucial to understand the diverse spectrum of experiments that underpin our understanding of data and outcomes. This article delves into three key areas: Simple Experiments, Complex Experiments, and Interpreting Results and Drawing Conclusions. Simple experiments, such as coin tosses and dice rolls, provide foundational insights into probability and statistical analysis. These straightforward scenarios help establish basic principles that can be scaled up to more intricate studies. On the other hand, complex experiments like medical trials and market research involve multifaceted variables and rigorous methodologies, offering deeper insights into real-world phenomena. Finally, interpreting results and drawing conclusions is essential for extracting meaningful information from any experiment. By examining these three aspects, we can gain a comprehensive understanding of how experiments are designed, executed, and analyzed. Let's begin by exploring the simplicity and clarity of simple experiments, such as coin tosses and dice rolls, which serve as the building blocks for more advanced research.
Simple Experiments (Coin Toss, Dice Roll)
In the realm of experimental probability, simple experiments such as coin tosses and dice rolls serve as foundational examples that illustrate key concepts. A coin toss, for instance, is a straightforward experiment where the outcome can be either heads or tails. When conducting multiple coin tosses, one can observe how the frequency of each outcome tends to stabilize around 50% over a large number of trials, aligning with the theoretical probability of 0.5 for each side. This phenomenon is a direct demonstration of the law of large numbers, which states that as the number of trials increases, the observed frequency of an event will converge to its theoretical probability. Similarly, rolling a fair six-sided die is another classic example. Each face of the die has an equal chance of landing face up, with a theoretical probability of 1/6 or approximately 0.167 for each number. By repeating the roll numerous times, one can calculate the experimental probability by dividing the number of times each number appears by the total number of rolls. Over many trials, these frequencies will approximate the theoretical probabilities, reinforcing the concept that experimental results converge to theoretical expectations as sample sizes grow. These simple experiments are not only educational tools but also practical illustrations of how probability works in real-world scenarios. They help in understanding that while individual outcomes are unpredictable, patterns emerge when considering large datasets. For example, in a classroom setting, students can conduct these experiments to see firsthand how theoretical probabilities are validated through repeated trials. This hands-on approach makes abstract concepts more tangible and engaging, fostering a deeper understanding of experimental probability. Moreover, these experiments highlight the importance of sample size and the role of randomness. Small sample sizes may yield results that deviate significantly from theoretical probabilities due to random fluctuations. However, as the sample size increases, these deviations diminish, and the observed frequencies align more closely with theoretical expectations. This principle is crucial in fields such as statistics, engineering, and social sciences where accurate predictions are based on large datasets. In conclusion, simple experiments like coin tosses and dice rolls are invaluable in illustrating the principles of experimental probability. They provide clear, accessible examples that demonstrate how theoretical probabilities are realized through repeated trials, making them essential case studies for understanding this fundamental concept in probability theory. By conducting these experiments, individuals can gain a practical grasp of how probability works, which is essential for making informed decisions in various fields where uncertainty is inherent.
Complex Experiments (Medical Trials, Market Research)
Complex experiments, such as medical trials and market research, are meticulously designed to gather data and draw meaningful conclusions. In medical trials, these experiments involve rigorous protocols to test the efficacy and safety of new treatments or drugs. For instance, randomized controlled trials (RCTs) are considered the gold standard because they randomly assign participants to either a treatment group or a control group, minimizing bias and ensuring that any observed effects can be attributed to the treatment itself. The process includes multiple phases, from initial small-scale studies to larger, more comprehensive trials that involve thousands of participants. This structured approach helps in identifying potential side effects, optimal dosages, and the overall effectiveness of the treatment. In market research, complex experiments are used to understand consumer behavior and preferences. These experiments often involve A/B testing, where two versions of a product or service are presented to different groups of consumers to see which one performs better. For example, a company might conduct an experiment to determine whether a new packaging design increases sales by randomly assigning different packaging versions to various retail locations. By analyzing the data from these experiments, researchers can identify trends, preferences, and potential barriers to consumer engagement, allowing businesses to make informed decisions about product development and marketing strategies. Both types of experiments rely heavily on statistical analysis to interpret results accurately. In medical trials, statistical methods are used to determine whether the observed outcomes are due to chance or the actual effect of the treatment. Similarly, in market research, statistical tools help in identifying significant differences between the experimental groups and in predicting future consumer behavior based on the data collected. Case studies provide valuable insights into how these complex experiments are conducted and their outcomes. For example, the development of vaccines during the COVID-19 pandemic involved extensive clinical trials that adhered to strict experimental protocols. These trials were crucial in ensuring the safety and efficacy of the vaccines before they were approved for widespread use. In market research, companies like Amazon and Google frequently conduct A/B testing to optimize their user interfaces and improve customer satisfaction. These experiments have led to significant improvements in user experience and have driven business growth by aligning products more closely with consumer needs. Overall, complex experiments in medical trials and market research are essential for making informed decisions based on empirical evidence. They require careful planning, execution, and analysis to ensure that the results are reliable and actionable. By understanding how these experiments are designed and conducted, we can appreciate the importance of experimental probability in real-world applications and how it contributes to advancements in healthcare and business.
Interpreting Results and Drawing Conclusions
When interpreting results and drawing conclusions in the context of experimental probability, it is crucial to approach the data with a systematic and analytical mindset. Experimental probability, which is derived from conducting repeated trials or experiments, provides empirical evidence that can be used to make informed decisions. Here are the key steps to follow: 1. **Data Collection and Organization**: Ensure that the data collected from the experiments is accurate, complete, and well-organized. This involves recording the outcomes of each trial meticulously. 2. **Frequency Analysis**: Calculate the frequency of each outcome and determine how often each event occurs. This can be presented in a table or graph for better visualization. 3. **Probability Calculation**: Use the formula for experimental probability: \( P(\text{event}) = \frac{\text{Number of times the event occurs}}{\text{Total number of trials}} \). This calculation helps in quantifying the likelihood of an event. 4. **Comparison with Theoretical Probability**: If applicable, compare the experimental probability with the theoretical probability to see if there are any discrepancies. This comparison can help in understanding whether the experimental results align with expected outcomes. 5. **Drawing Conclusions**: Based on the calculated probabilities, draw conclusions about the likelihood of future events. For instance, if an experiment shows that a certain event occurs 60% of the time over 100 trials, it can be concluded that this event has a high probability of occurring in future trials. 6. **Limitations and Sources of Error**: Consider any limitations or potential sources of error in the experiment. Factors such as sample size, experimental design, and external influences can affect the reliability of the results. 7. **Generalizability**: Assess whether the findings can be generalized to larger populations or different contexts. This involves considering whether the sample is representative and if the conditions under which the experiment was conducted are typical. 8. **Case Studies and Examples**: Use real-world examples and case studies to illustrate how experimental probability is applied in practice. For example, in quality control, manufacturers might conduct experiments to determine the probability of defects in their products. By analyzing these probabilities, they can make informed decisions about production processes and quality assurance measures. By following these steps, one can ensure that the interpretation of results is robust and that conclusions drawn are well-supported by empirical evidence. This systematic approach not only enhances the credibility of the findings but also facilitates better decision-making in various fields where probability plays a critical role.