What Is The Manipulated Variable
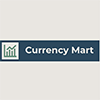
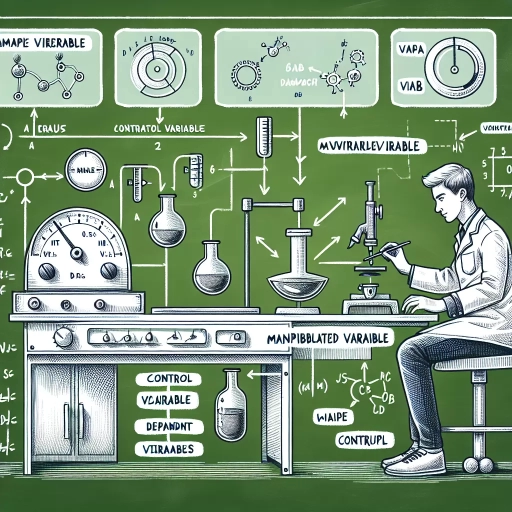
In the realm of scientific research and experimentation, understanding the manipulated variable is crucial for drawing meaningful conclusions and ensuring the validity of results. The manipulated variable, also known as the independent variable, is the factor that researchers intentionally change to observe its effect on the outcome or dependent variable. This concept is foundational in experimental design, allowing scientists to isolate cause-and-effect relationships. In this article, we will delve into the concept of the manipulated variable, exploring its definition and significance. We will also discuss how to identify the manipulated variable in research studies, highlighting its critical role in maintaining experimental integrity. Additionally, we will examine the practical applications of the manipulated variable across various fields, illustrating its impact on real-world problem-solving. By grasping these aspects, researchers can design more effective experiments and interpret data with greater precision. Let's begin by understanding the concept of the manipulated variable in depth.
Understanding the Concept of Manipulated Variable
Understanding the concept of a manipulated variable is crucial in the realm of scientific research and experimental design. This key component plays a pivotal role in determining the outcomes of experiments and is often misunderstood or conflated with other variables. To grasp its significance, it is essential to delve into its definition and context, explore its role in experimental design, and distinguish it from independent and dependent variables. By defining what a manipulated variable is and understanding its historical and practical context, researchers can better appreciate its importance. The role of the manipulated variable in experimental design highlights how it influences the experiment's structure and outcomes. Furthermore, distinguishing it from independent and dependent variables clarifies its unique function within the experimental framework. Starting with a clear definition and context will provide a solid foundation for understanding this critical concept. --- **Definition and Context**
Definition and Context
In the context of scientific experimentation and research, the concept of a manipulated variable is crucial for understanding cause-and-effect relationships. A manipulated variable, also known as an independent variable, is a factor that the researcher intentionally changes or controls to observe its effect on the outcome. This variable is "manipulated" because the experimenter actively alters it to see how these changes influence the dependent variable, which is the outcome being measured. For instance, in a study examining the impact of different light intensities on plant growth, the light intensity would be the manipulated variable. The researcher would systematically vary the light intensity (e.g., low, medium, high) while keeping other factors constant to observe how each level affects plant growth. This controlled manipulation allows scientists to isolate the effect of the variable in question and draw conclusions about its influence on the outcome. The definition and context of a manipulated variable are essential because they form the backbone of experimental design. By identifying and manipulating specific variables, researchers can establish causality rather than mere correlation. This distinction is vital in fields such as psychology, biology, physics, and social sciences where understanding cause-and-effect relationships is paramount for advancing knowledge and making informed decisions. In practical terms, identifying a manipulated variable involves careful consideration of what factors can be feasibly altered and measured within an experiment. It also requires ensuring that other variables (confounding variables) are controlled or randomized to prevent them from influencing the outcome. For example, in a study on the effect of exercise on blood pressure, the type and duration of exercise would be manipulated variables, while factors like diet and age might need to be controlled to avoid confounding effects. Understanding the concept of a manipulated variable is fundamental for designing robust experiments that yield reliable data. It enables researchers to test hypotheses systematically and make evidence-based conclusions about how changes in one variable affect another. This understanding is not only limited to academic research but also extends to real-world applications such as product development, policy-making, and healthcare interventions where identifying causal relationships is critical for decision-making. In summary, the manipulated variable is a cornerstone of experimental methodology that allows researchers to explore causal relationships by systematically altering one factor while controlling others. Its precise definition and context are essential for conducting rigorous scientific investigations that contribute to our understanding of various phenomena across different disciplines.
Role in Experimental Design
In the context of experimental design, the role of the manipulated variable is pivotal in determining the causal relationship between variables. The manipulated variable, also known as the independent variable, is the factor that the researcher intentionally changes or controls to observe its effect on the outcome. This deliberate alteration allows scientists to isolate and measure the impact of this variable on the dependent variable, which is the outcome being measured. For instance, in a study examining the effect of different fertilizer types on plant growth, the type of fertilizer would be the manipulated variable. By systematically varying the type of fertilizer and keeping other factors constant, researchers can determine whether and how different fertilizers influence plant growth. The precision in manipulating this variable ensures that any observed changes in the dependent variable can be attributed to the manipulated variable rather than other extraneous factors. This control is crucial for establishing cause-and-effect relationships and for drawing valid conclusions about the phenomenon under study. Additionally, the manipulation of variables helps in minimizing confounding variables—factors that could also affect the outcome but are not of interest in the study. By controlling these extraneous variables through techniques such as randomization and matching, researchers can ensure that any observed effects are due solely to the manipulated variable. Moreover, the role of the manipulated variable extends beyond mere observation; it also enables researchers to test hypotheses and theories. For example, if a hypothesis suggests that a new teaching method will improve student performance, the new teaching method would be the manipulated variable. By comparing student performance under this new method against a traditional method (the control group), researchers can validate or refute the hypothesis. This systematic approach not only enhances the reliability of findings but also contributes to the advancement of knowledge in various fields by providing empirical evidence for theoretical constructs. In summary, the manipulated variable plays a central role in experimental design by allowing researchers to test hypotheses, establish cause-and-effect relationships, and control for extraneous factors. Its careful manipulation is essential for conducting rigorous and meaningful experiments that contribute significantly to scientific understanding and practical applications.
Distinguishing from Independent and Dependent Variables
In the realm of scientific research and experimentation, understanding the distinction between independent and dependent variables is crucial for designing and interpreting studies effectively. The **independent variable**, often referred to as the manipulated variable, is the factor that the researcher intentionally changes or controls to observe its effect on the outcome. This variable is typically the cause or predictor in a study. For instance, in an experiment to determine how different levels of fertilizer affect plant growth, the amount of fertilizer applied would be the independent variable. On the other hand, the **dependent variable** is the outcome or response being measured. It is the variable that changes in response to the independent variable. Continuing with the fertilizer example, plant growth (measured in terms of height, weight, or biomass) would be the dependent variable. The researcher manipulates the independent variable (fertilizer levels) to observe its impact on the dependent variable (plant growth). Distinguishing between these variables is essential because it allows researchers to establish cause-and-effect relationships. By controlling for other factors and isolating the independent variable, researchers can determine whether changes in the dependent variable are due to the manipulation of the independent variable rather than other extraneous factors. This clarity is vital for drawing valid conclusions and ensuring that the results are reliable and generalizable. Moreover, understanding these variables helps in avoiding confounding variables—factors other than the independent variable that could influence the dependent variable. For example, if an experiment on plant growth does not control for sunlight exposure, differences in plant growth could be attributed to sunlight rather than fertilizer levels. By identifying and controlling for such confounding variables, researchers can ensure that their findings accurately reflect the relationship between the independent and dependent variables. In summary, the independent variable is the factor manipulated by the researcher to observe its effect, while the dependent variable is the outcome being measured. This distinction is fundamental to experimental design and analysis, enabling researchers to establish clear cause-and-effect relationships and draw meaningful conclusions from their data. Understanding this concept is essential for anyone seeking to grasp what a manipulated variable is and how it functions within scientific inquiry.
Identifying the Manipulated Variable in Research
Identifying the manipulated variable in research is a crucial step in understanding the design and outcomes of experiments. This process involves recognizing intentional changes made by the researcher, analyzing experimental controls, and understanding cause-and-effect relationships. By recognizing intentional changes, researchers can pinpoint the specific factor they are altering to observe its impact. Analyzing experimental controls helps ensure that other variables are held constant, allowing for a clear assessment of the manipulated variable's effect. Understanding cause-and-effect relationships is essential to interpreting the results accurately and drawing valid conclusions. These elements collectively form the foundation of rigorous scientific inquiry, enabling researchers to isolate and study the manipulated variable with precision. To delve deeper into this critical aspect, let's first explore the importance of recognizing intentional changes in experimental design.
Recognizing Intentional Changes
Recognizing intentional changes is a crucial aspect of identifying the manipulated variable in research. The manipulated variable, also known as the independent variable, is the factor that researchers deliberately alter to observe its effect on the outcome or dependent variable. To recognize these intentional changes, researchers must meticulously design their experiments to ensure that only the manipulated variable is altered while all other factors remain constant. This control allows for the isolation of the effect of the manipulated variable, enabling clear and reliable conclusions. In experimental design, recognizing intentional changes involves several key steps. First, researchers must clearly define the research question and hypothesis, which guides the identification of the manipulated variable. For instance, if a study aims to investigate how different levels of fertilizer affect plant growth, the amount of fertilizer would be the manipulated variable. Next, researchers must ensure that all other variables that could influence the outcome are controlled or held constant. This might involve using identical soil types, maintaining uniform lighting conditions, and ensuring consistent watering schedules for all experimental groups. Another critical aspect is randomization, where participants or subjects are randomly assigned to different treatment groups. This helps to distribute any confounding variables evenly across groups, reducing bias and ensuring that any observed effects are due to the manipulated variable rather than other factors. Additionally, researchers often use placebos or control groups to provide a baseline against which the effects of the manipulated variable can be compared. Effective recognition of intentional changes also requires precise measurement and data collection. Researchers must use reliable and validated instruments to measure both the manipulated variable and the dependent variable. For example, in a study examining the impact of exercise on blood pressure, accurate measurements of blood pressure before and after exercise would be essential. Finally, statistical analysis plays a vital role in confirming whether the observed changes are statistically significant and can be attributed to the manipulated variable. Techniques such as ANOVA (Analysis of Variance) or t-tests help determine if there are significant differences between treatment groups, thereby validating the effect of the manipulated variable. By meticulously recognizing and controlling for intentional changes, researchers can confidently identify the manipulated variable and draw meaningful conclusions about its impact on the outcome. This rigorous approach ensures that research findings are reliable, generalizable, and contribute valuable insights to the field of study.
Analyzing Experimental Controls
Analyzing experimental controls is a crucial step in identifying the manipulated variable in research. Experimental controls are the measures taken to ensure that the experiment is conducted in a way that isolates the effect of the manipulated variable, thereby allowing researchers to draw valid conclusions. These controls help minimize confounding variables, which are factors other than the manipulated variable that could influence the outcome of the experiment. To analyze experimental controls effectively, researchers must first identify potential confounding variables. This involves understanding the research question and the experimental design. For instance, in a study examining the effect of light on plant growth, factors such as soil quality, water availability, and temperature could act as confounding variables if not controlled. Researchers then implement control measures to hold these variables constant across all experimental groups. This might involve using identical soil types, maintaining consistent watering schedules, and ensuring uniform temperature conditions. Another key aspect of analyzing experimental controls is the use of control groups. A control group is a group in the experiment that does not receive the manipulated variable but is otherwise treated identically to the experimental group. By comparing the outcomes between the control and experimental groups, researchers can determine whether any observed effects are due to the manipulated variable rather than other factors. Randomization is also an essential control mechanism. Randomizing the assignment of participants or subjects to different groups helps distribute confounding variables evenly across groups, reducing bias and ensuring that any differences observed are more likely due to the manipulated variable. Additionally, researchers often use blinding techniques to further control for bias. Single-blinding involves keeping participants unaware of whether they are in the control or experimental group, while double-blinding extends this to keep researchers unaware as well. This helps prevent any unconscious biases that could influence the results. In summary, analyzing experimental controls involves identifying and managing confounding variables through careful design, implementing control groups, randomizing group assignments, and using blinding techniques. By doing so, researchers can confidently attribute observed effects to the manipulated variable, thereby enhancing the validity and reliability of their findings. This meticulous approach is essential for identifying the manipulated variable accurately and ensuring that research conclusions are based on robust evidence.
Understanding Cause-and-Effect Relationships
Understanding cause-and-effect relationships is a cornerstone of scientific inquiry, particularly when identifying the manipulated variable in research. At its core, a cause-and-effect relationship involves an independent variable (the cause) that influences a dependent variable (the effect). In experimental research, the manipulated variable is the independent variable that researchers intentionally change to observe its impact on the dependent variable. This distinction is crucial because it allows researchers to isolate and measure the specific effect of the manipulated variable, thereby establishing a clear cause-and-effect relationship. To illustrate this concept, consider a study examining the impact of exercise on blood pressure. Here, exercise is the manipulated variable (independent variable) because it is the factor being altered by the researcher. Blood pressure, in turn, is the dependent variable (effect) because it is the outcome being measured in response to changes in exercise. By systematically varying the amount of exercise and controlling for other potential influences, researchers can determine whether there is a direct cause-and-effect relationship between exercise and blood pressure. The key to establishing a robust cause-and-effect relationship lies in ensuring that the manipulation of the independent variable is the sole factor influencing the dependent variable. This involves careful control over extraneous variables that could confound the results. For instance, in the exercise and blood pressure study, factors such as diet, age, and pre-existing health conditions must be controlled or accounted for to ensure that any observed changes in blood pressure are indeed due to the exercise intervention. Moreover, understanding cause-and-effect relationships requires an appreciation for temporal precedence (the cause precedes the effect), covariation (the cause and effect vary together), and non-spuriousness (the relationship is not due to a third variable). These criteria help researchers distinguish between correlation and causation, ensuring that observed effects are not merely coincidental but rather the direct result of manipulating the independent variable. In summary, identifying the manipulated variable in research is essential for understanding cause-and-effect relationships. By carefully controlling for extraneous variables and ensuring that the manipulated variable precedes and covaries with the dependent variable, researchers can establish clear and reliable cause-and-effect relationships. This rigorous approach not only enhances the validity of research findings but also provides a solid foundation for making informed decisions and predictions in various fields of study.
Practical Applications of the Manipulated Variable
The concept of the manipulated variable is a cornerstone in various fields, offering a robust framework for understanding cause-and-effect relationships. In scientific experiments, manipulating variables allows researchers to isolate and study the impact of specific factors on outcomes, thereby enhancing the validity and reliability of their findings. In business and marketing strategies, identifying and manipulating key variables can optimize product development, pricing, and advertising efforts, leading to improved market performance. Additionally, in educational research and teaching methods, manipulating variables helps educators design more effective learning environments and instructional techniques. By examining these practical applications, we can gain a deeper understanding of how the manipulated variable contributes to scientific discovery, business success, and educational excellence. This article will delve into these areas, starting with an exploration of how scientific experiments and studies leverage the manipulated variable to advance our knowledge.
Scientific Experiments and Studies
Scientific experiments and studies are the cornerstone of advancing our understanding of the world around us. These structured investigations involve the manipulation of variables to observe and measure their effects, providing valuable insights into cause-and-effect relationships. In the context of practical applications, the manipulated variable is a crucial element that allows researchers to isolate and study specific factors. For instance, in a study on plant growth, the manipulated variable might be the amount of fertilizer applied, while other factors such as light exposure and water intake are controlled. By systematically varying the amount of fertilizer and observing the resulting differences in plant growth, scientists can determine whether fertilizer has a significant impact. This approach not only helps in understanding biological processes but also has practical applications in agriculture, where optimizing fertilizer use can enhance crop yields and reduce environmental impact. Similarly, in medical research, manipulating variables such as dosage levels of a new drug can help determine its efficacy and safety, leading to better treatment options for patients. The precision and control inherent in scientific experiments ensure that findings are reliable and can be replicated, making them essential for developing evidence-based policies and practices across various fields. By meticulously designing and conducting experiments, scientists can uncover underlying mechanisms and translate these discoveries into tangible benefits, such as improved healthcare outcomes, more efficient technologies, and sustainable environmental practices. Ultimately, the careful manipulation of variables in scientific experiments is a key driver of innovation and progress, enabling us to address complex challenges and improve the quality of life for individuals and communities worldwide.
Business and Marketing Strategies
In the realm of business and marketing, understanding and effectively leveraging the manipulated variable is crucial for driving strategic outcomes. The manipulated variable, often a controllable factor such as pricing, advertising spend, or product features, serves as a key lever that businesses can adjust to influence desired outcomes like sales, customer engagement, or market share. For instance, in a competitive market, a company might manipulate its pricing strategy to undercut competitors and capture a larger market share. This could involve conducting thorough market research to identify the optimal price point that balances revenue goals with customer affordability and perceived value. Marketing strategies also heavily rely on the manipulation of variables such as advertising channels and messaging. By adjusting the mix of digital and traditional advertising, businesses can target specific demographics more effectively. For example, a company aiming to reach a younger audience might allocate more resources to social media campaigns, while those targeting an older demographic might focus on print media or television ads. Additionally, the messaging itself can be manipulated to resonate better with the target audience; this could involve emphasizing different product benefits or using language that aligns with the values and preferences of the target market. Another critical aspect is the manipulation of product features and offerings. Companies can introduce new features or variants to cater to evolving consumer needs and preferences. This could involve gathering feedback through customer surveys or focus groups to identify areas for improvement or innovation. For instance, a tech company might introduce a new smartphone model with enhanced camera capabilities in response to consumer demand for better photography features. Moreover, businesses can manipulate their distribution channels to improve accessibility and convenience for customers. This might involve expanding into new markets through e-commerce platforms or partnering with retailers to increase physical presence. The manipulation of distribution channels can also be used to create strategic alliances that enhance brand visibility and reach. In practical applications, the manipulation of variables is often data-driven, relying on analytics and performance metrics to measure the effectiveness of different strategies. A/B testing, for example, allows businesses to compare the impact of different manipulated variables in real-time, enabling them to make informed decisions based on empirical evidence rather than intuition. This data-driven approach ensures that resources are allocated efficiently and that strategies are continuously optimized for maximum impact. Ultimately, the ability to identify, manipulate, and measure the impact of key variables is at the heart of successful business and marketing strategies. By understanding which levers to pull and how to pull them, businesses can navigate complex market landscapes with greater precision and achieve their strategic objectives more effectively. This nuanced approach not only enhances competitive advantage but also fosters a culture of continuous improvement and innovation within the organization.
Educational Research and Teaching Methods
Educational research plays a pivotal role in shaping teaching methods by providing empirical evidence and insights into what works best in the classroom. This field of study involves systematic investigations into various aspects of education, including learning outcomes, instructional strategies, and student engagement. By manipulating variables such as teaching techniques, curriculum design, and classroom environment, researchers can identify the most effective practices that enhance student learning. For instance, research on active learning has shown that engaging students in hands-on activities and discussions significantly improves their understanding and retention of material compared to traditional lecturing methods. Similarly, studies on personalized learning have highlighted the benefits of tailoring instruction to individual students' needs and abilities, leading to better academic performance and higher student satisfaction. The manipulation of variables such as teacher feedback, technology integration, and peer collaboration has also been extensively studied, providing teachers with evidence-based strategies to optimize their teaching practices. Moreover, educational research informs policy decisions and curriculum development. For example, research on early childhood education has led to the implementation of programs like Head Start in the United States, which aim to provide disadvantaged children with a strong educational foundation. At the higher education level, research on flipped classrooms and online learning has influenced the adoption of these models in universities worldwide. The practical applications of these findings are multifaceted. Teachers can use research-based methods to create more inclusive and engaging learning environments. For example, incorporating Universal Design for Learning (UDL) principles ensures that lessons are accessible and meaningful for all students, regardless of their abilities. Additionally, educational technology can be leveraged to enhance teaching methods; tools like learning management systems and educational apps can facilitate personalized learning experiences. In summary, educational research is crucial for developing and refining teaching methods. By manipulating variables and analyzing their impact on student learning, researchers provide educators with actionable insights that can be applied directly in the classroom. This continuous cycle of research and application ensures that teaching practices remain innovative, effective, and aligned with the evolving needs of students. As such, it is essential for educators to stay informed about the latest research findings to deliver high-quality education that prepares students for success in an ever-changing world.