What Is A Test Statistic
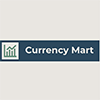
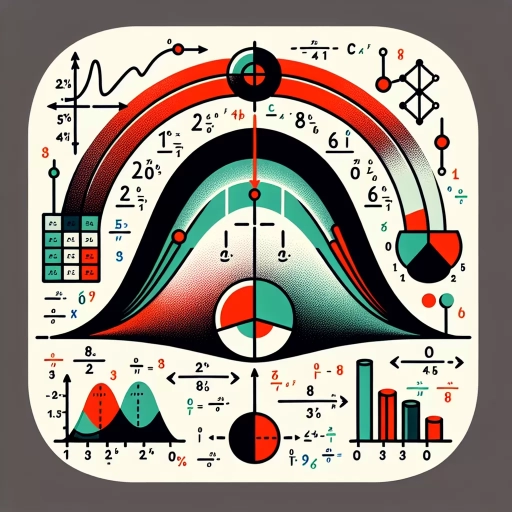
In the realm of statistical analysis, the concept of a test statistic is a cornerstone that enables researchers and analysts to make informed decisions about hypotheses and data. A test statistic is a numerical value that summarizes the data in relation to a hypothesis, providing a basis for determining whether the observed data are consistent with the null hypothesis or if they suggest an alternative explanation. This article delves into the intricacies of test statistics, starting with **Understanding the Concept of a Test Statistic**, where we explore the fundamental principles and definitions that underpin this statistical tool. We will then proceed to **How Test Statistics Are Calculated**, detailing the mathematical processes involved in deriving these values from sample data. Finally, we will examine **Applications and Examples of Test Statistics**, highlighting real-world scenarios where test statistics play a crucial role in decision-making. By grasping these aspects, readers will gain a comprehensive understanding of how test statistics are used to draw meaningful conclusions from data. Let us begin by understanding the concept of a test statistic.
Understanding the Concept of a Test Statistic
Understanding the concept of a test statistic is a cornerstone in statistical analysis, enabling researchers and analysts to make informed decisions based on data. This fundamental concept is multifaceted, encompassing several key aspects that are crucial for its effective application. First, it is essential to grasp the definition and purpose of a test statistic, which serves as the foundation for interpreting statistical results. This understanding helps in determining whether observed data align with or deviate from a hypothesized value. Second, recognizing the various types of test statistics is vital, as different scenarios call for different statistical measures. For instance, t-statistics are used for comparing means, while chi-square statistics are employed for categorical data. Lastly, the importance of test statistics in statistical analysis cannot be overstated; they provide a quantitative measure to evaluate hypotheses, thereby guiding decision-making processes in fields ranging from medicine to finance. By delving into these aspects, one can appreciate the robustness and utility of test statistics. Let us begin by exploring the definition and purpose of test statistics, which sets the stage for a deeper understanding of this critical statistical tool.
Definition and Purpose
In the realm of statistical analysis, the concept of a test statistic is pivotal for making informed decisions about hypotheses. **Definition and Purpose** are fundamental aspects that underpin the understanding of test statistics. A test statistic is a numerical value calculated from sample data that is used to determine whether there is sufficient evidence to reject the null hypothesis in favor of an alternative hypothesis. Essentially, it quantifies the difference between the observed data and what would be expected under the null hypothesis, allowing researchers to gauge the likelihood of observing such data if the null hypothesis were true. The purpose of a test statistic is multifaceted. Primarily, it serves as a bridge between empirical data and theoretical hypotheses, enabling researchers to evaluate the validity of their hypotheses objectively. By comparing the calculated test statistic to a critical value or by determining its p-value, researchers can assess the probability of obtaining the observed results (or more extreme) assuming that the null hypothesis is correct. This process helps in making decisions about whether to reject or fail to reject the null hypothesis, thereby guiding conclusions about population parameters based on sample data. Moreover, test statistics are designed to be robust and reliable indicators of statistical significance. They account for various factors such as sample size, variability, and distribution type, ensuring that conclusions drawn are not merely due to chance but reflect genuine patterns or differences in the data. For instance, in hypothesis testing involving means, the t-statistic or z-statistic might be used; for proportions, chi-square statistics could be employed; and for correlations, t-statistics or F-statistics might be relevant. The versatility of test statistics also lies in their ability to accommodate different types of data and research questions. Whether it's comparing means between groups (e.g., t-test), assessing associations between variables (e.g., correlation coefficient), or evaluating goodness-of-fit (e.g., chi-square test), each type of test statistic is tailored to address specific research objectives. This adaptability ensures that researchers can apply appropriate statistical tools to their data regardless of the complexity or nature of their inquiry. In summary, understanding the definition and purpose of a test statistic is crucial for conducting rigorous statistical analysis. It empowers researchers with a systematic approach to hypothesis testing, allowing them to draw meaningful conclusions from empirical evidence while minimizing errors due to chance or sampling variability. By leveraging test statistics effectively, researchers can enhance the reliability and validity of their findings, contributing significantly to scientific knowledge and decision-making processes across various disciplines.
Types of Test Statistics
When delving into the concept of test statistics, it is crucial to understand the various types that are employed in statistical analysis. Test statistics are numerical values calculated from sample data to determine whether there is sufficient evidence to reject a null hypothesis. There are several key types of test statistics, each tailored to specific scenarios and data characteristics. 1. **Z-Statistic**: This is one of the most common test statistics, particularly useful when the population standard deviation is known. The Z-statistic measures how many standard deviations an element is from the mean. It is widely used in hypothesis testing for large samples and is a cornerstone in many statistical analyses. 2. **T-Statistic**: Unlike the Z-statistic, the T-statistic is used when the population standard deviation is unknown. It is more robust and suitable for smaller sample sizes. The T-statistic is essential in t-tests, which are fundamental in comparing means between groups or against a known population mean. 3. **Chi-Square Statistic**: This statistic is used for categorical data and is particularly useful in tests of independence and goodness-of-fit. The Chi-square statistic measures the difference between observed frequencies and expected frequencies under a null hypothesis, making it invaluable in assessing whether there is a significant association between variables. 4. **F-Statistic**: The F-statistic is primarily used in analysis of variance (ANOVA) and regression analysis. It compares the variance between groups to the variance within groups, helping to determine if there are significant differences among group means or if a regression model is a good fit for the data. 5. **Wilcoxon Rank-Sum Statistic**: This non-parametric test statistic is used when the data do not meet the assumptions required for parametric tests like the T-test. It compares two independent samples to assess if one is consistently larger than the other, making it a versatile tool for ordinal data or when normality cannot be assumed. 6. **Kruskal-Wallis H Statistic**: Another non-parametric test, the Kruskal-Wallis H statistic extends the Wilcoxon rank-sum test to compare more than two independent samples. It is used to determine if there are significant differences among three or more groups, providing a robust alternative to ANOVA when assumptions are violated. Understanding these different types of test statistics is essential for selecting the appropriate statistical test for a given research question or data set. Each type has its own strengths and limitations, and choosing the right one ensures that the analysis is valid and interpretable. By recognizing which test statistic to use in various scenarios, researchers can accurately evaluate hypotheses and draw meaningful conclusions from their data. This knowledge not only enhances the rigor of statistical analyses but also aids in communicating findings effectively to both technical and non-technical audiences.
Importance in Statistical Analysis
Statistical analysis is a cornerstone of modern research and decision-making, and its importance cannot be overstated. At the heart of this discipline lies the concept of the test statistic, a numerical value that encapsulates the essence of data and guides us toward meaningful conclusions. Understanding the significance of statistical analysis begins with recognizing its role in validating hypotheses and making informed decisions. In any field, whether it be medicine, economics, or social sciences, statistical analysis provides a systematic approach to extracting insights from data. It allows researchers to move beyond anecdotal evidence and intuition by quantifying trends, patterns, and relationships within datasets. The test statistic, in particular, serves as a critical tool for hypothesis testing—a fundamental process in scientific inquiry. By calculating a test statistic, researchers can determine whether observed differences or associations are likely due to chance or if they reflect a genuine effect. This distinction is crucial because it helps in avoiding false positives and false negatives, thereby ensuring that conclusions are grounded in empirical evidence. Moreover, statistical analysis enhances the reliability and generalizability of findings. By applying statistical methods, researchers can account for variability and uncertainty inherent in data, leading to more robust and replicable results. This is particularly important in fields like medicine, where the accuracy of clinical trials can have life-or-death implications. For instance, a pharmaceutical company might use statistical analysis to determine whether a new drug is effective in treating a disease. The test statistic would help them assess whether the observed improvement in patient outcomes is statistically significant, thereby informing regulatory approvals and clinical practice guidelines. Beyond hypothesis testing, statistical analysis also facilitates exploratory data analysis (EDA) and predictive modeling. EDA involves using various statistical techniques to uncover hidden patterns and relationships within datasets, which can lead to new research questions and hypotheses. Predictive models, on the other hand, leverage statistical algorithms to forecast future outcomes based on historical data. These models are essential in fields such as finance, where they help in risk assessment and portfolio management. In addition to its practical applications, statistical analysis contributes significantly to the advancement of knowledge across disciplines. It enables researchers to synthesize large volumes of data into coherent narratives, thereby contributing to our understanding of complex phenomena. For example, in climate science, statistical analysis of temperature records and other environmental data helps scientists understand global warming trends and predict future climate changes. Finally, the importance of statistical analysis extends beyond academic and professional circles; it also has a profound impact on public policy and decision-making. Policymakers rely heavily on statistical data to inform their decisions on issues ranging from healthcare policy to economic stimulus packages. Accurate statistical analysis ensures that these decisions are based on evidence rather than speculation, leading to more effective and efficient governance. In conclusion, statistical analysis is indispensable in today's data-driven world. The test statistic, as a key component of this analysis, plays a vital role in hypothesis testing, ensuring that conclusions drawn from data are reliable and meaningful. By leveraging statistical methods, researchers and policymakers can make informed decisions, advance scientific knowledge, and drive progress across various fields. Understanding the concept of a test statistic is thus essential for anyone seeking to navigate the complexities of data-driven decision-making effectively.
How Test Statistics Are Calculated
Understanding how test statistics are calculated is a cornerstone of statistical analysis, enabling researchers and analysts to draw meaningful conclusions from data. This process involves several key components that work together to provide a comprehensive picture. First, **Formulas and Equations** serve as the foundation, outlining the mathematical steps necessary to compute test statistics. These formulas vary depending on the type of test being conducted, such as t-tests, ANOVA, or chi-square tests. Next, **Common Statistical Tests** highlight the different types of analyses that can be performed, each with its own set of assumptions and applications. Finally, **Interpretation of Results** is crucial for understanding what the calculated test statistics signify in the context of the research question or hypothesis. By mastering these elements, one can ensure that statistical analyses are both accurate and insightful. To delve deeper into this subject, let's begin by examining the **Formulas and Equations** that underpin the calculation of test statistics.
Formulas and Equations
Formulas and equations are the backbone of statistical analysis, particularly when calculating test statistics. These mathematical expressions provide a structured way to quantify and analyze data, enabling researchers to draw meaningful conclusions. At the heart of test statistic calculation lies the ability to apply specific formulas that transform raw data into interpretable measures. For instance, the t-statistic, commonly used in t-tests, is calculated using the formula \( t = \frac{\bar{X} - \mu}{s / \sqrt{n}} \), where \( \bar{X} \) is the sample mean, \( \mu \) is the population mean, \( s \) is the sample standard deviation, and \( n \) is the sample size. This formula encapsulates the relationship between sample data and population parameters, allowing for hypotheses about population means to be tested. Similarly, the z-statistic, often employed in z-tests and confidence intervals, is computed using \( z = \frac{\bar{X} - \mu}{\sigma / \sqrt{n}} \), where \( \sigma \) represents the known population standard deviation. This equation highlights how standard deviation plays a crucial role in assessing variability and its impact on statistical inference. Another key formula is that of the chi-square statistic (\( \chi^2 \)), which is calculated as \( \chi^2 = \sum \frac{(O_i - E_i)^2}{E_i} \), where \( O_i \) and \( E_i \) are observed and expected frequencies, respectively. This formula is pivotal in goodness-of-fit tests and tests of independence. The F-statistic, used in analysis of variance (ANOVA), is derived from the ratio of between-group variance to within-group variance: \( F = \frac{MSB}{MSW} \), where MSB is the mean square between groups and MSW is the mean square within groups. This equation underscores the importance of variance components in comparing group means. Each of these formulas and equations serves as a tool for extracting insights from data, facilitating the process of hypothesis testing and statistical inference. Moreover, understanding these formulas requires a grasp of underlying statistical concepts such as sampling distributions, degrees of freedom, and levels of significance. For example, knowing that degrees of freedom affect the shape of t-distributions or that alpha levels determine critical values is essential for interpreting test statistics accurately. By applying these formulas correctly, researchers can ensure that their conclusions are grounded in robust statistical analysis. In summary, formulas and equations are indispensable in calculating test statistics. They provide a systematic approach to data analysis, enabling researchers to evaluate hypotheses rigorously. Mastering these mathematical expressions is crucial for anyone involved in statistical analysis, as they form the foundation upon which reliable conclusions are drawn. By leveraging these tools effectively, one can navigate the complexities of statistical inference with confidence and precision.
Common Statistical Tests
When delving into the realm of statistical analysis, understanding common statistical tests is crucial for making informed decisions and drawing meaningful conclusions from data. These tests serve as the backbone of hypothesis testing, allowing researchers to evaluate the significance of their findings and determine whether observed effects are due to chance or genuine patterns. Here, we explore some of the most frequently used statistical tests and their applications. **1. T-Test:** The t-test is a versatile tool for comparing means between two groups. It is particularly useful when sample sizes are small or when the population standard deviation is unknown. There are two main types: the independent samples t-test, which compares means between two distinct groups, and the paired samples t-test, which compares means before and after a treatment within the same group. **2. ANOVA (Analysis of Variance):** ANOVA extends the concept of the t-test by allowing comparisons among three or more groups. It helps determine if there is a significant difference between the means of these groups. ANOVA can be one-way (comparing means across different levels of a single factor) or two-way (examining the interaction between two factors). **3. Chi-Square Test:** The chi-square test is used for categorical data and assesses whether there is a significant association between two variables. It is commonly employed in cross-tabulations to determine if observed frequencies differ significantly from expected frequencies under a null hypothesis of no association. **4. Regression Analysis:** Regression analysis, including simple and multiple linear regression, aims to model the relationship between a dependent variable and one or more independent variables. It helps in predicting the value of the dependent variable based on the values of the independent variables and in understanding how changes in these variables affect the outcome. **5. Non-Parametric Tests:** Non-parametric tests are used when data do not meet the assumptions required for parametric tests, such as normality or equal variances. Examples include the Wilcoxon rank-sum test (a non-parametric alternative to the independent samples t-test) and the Kruskal-Wallis H-test (a non-parametric alternative to ANOVA). **6. Correlation Coefficient:** The correlation coefficient measures the strength and direction of a linear relationship between two continuous variables. Commonly used coefficients include Pearson's r for normally distributed data and Spearman's rho for non-normal data. Each of these statistical tests has its own set of assumptions and requirements, and choosing the right test depends on the nature of the data, the research question, and the level of measurement. By understanding these tests and their appropriate applications, researchers can ensure that their analyses are robust and their conclusions are reliable. This foundational knowledge is essential for calculating test statistics accurately, as it allows for the correct interpretation of results and informed decision-making in various fields such as medicine, social sciences, business, and more.
Interpretation of Results
When interpreting the results of test statistics, it is crucial to understand the context and implications of the calculated values. Test statistics are numerical measures that help determine whether observed data support or reject a hypothesis. The interpretation involves several key steps and considerations. First, **understand the null and alternative hypotheses**. The null hypothesis typically represents a statement of no effect or no difference, while the alternative hypothesis suggests an effect or difference. The test statistic is calculated to determine if there is sufficient evidence to reject the null hypothesis in favor of the alternative. Next, **consider the p-value**, which is a probability measure that indicates how likely it is to observe the test statistic (or a more extreme value) assuming the null hypothesis is true. A small p-value (usually less than 0.05) suggests that the observed data are unlikely under the null hypothesis, leading to its rejection. Conversely, a large p-value indicates that the data are consistent with the null hypothesis, and it should not be rejected. **Effect size** is another critical aspect to consider. While a statistically significant result (indicated by a low p-value) tells us that an effect is unlikely due to chance, it does not necessarily indicate the magnitude of the effect. Effect size measures, such as Cohen's d or odds ratios, provide insight into how practically significant the findings are. Additionally, **confidence intervals** can be used to interpret results. These intervals provide a range of plausible values for the population parameter being estimated. If the confidence interval does not include the null hypothesis value (e.g., zero for differences or one for ratios), it suggests statistical significance. It is also important to **consider the sample size** and **data quality**. Larger samples generally provide more reliable estimates and greater statistical power to detect effects. However, larger samples can also make trivial effects statistically significant but practically insignificant. Data quality issues such as missing values, outliers, or measurement errors can affect the validity of the results. **Contextual interpretation** is vital as well. Results should be interpreted within the framework of existing literature and theoretical expectations. For instance, if a study finds a statistically significant effect but it contradicts established theories or previous findings, further investigation may be necessary. Finally, **avoid common pitfalls** such as mistaking statistical significance for practical significance or ignoring potential biases and confounding variables. Proper interpretation requires a balanced view that considers both statistical and practical aspects of the results. In summary, interpreting test statistics involves understanding hypotheses, evaluating p-values and effect sizes, considering confidence intervals and sample characteristics, interpreting within context, and avoiding common pitfalls. By doing so, researchers can draw meaningful conclusions from their data and contribute valuable insights to their field of study.
Applications and Examples of Test Statistics
Test statistics are fundamental tools in statistical analysis, enabling researchers and practitioners to make informed decisions based on data. This article delves into the diverse applications and examples of test statistics, highlighting their significance in various contexts. We will explore real-world scenarios where test statistics are crucial for decision-making, examine case studies that illustrate their practical application, and discuss their essential role in research methodologies. By understanding how test statistics are applied in everyday situations, we can appreciate their impact on fields such as medicine, finance, and social sciences. For instance, in healthcare, test statistics help determine the efficacy of new treatments, while in finance, they aid in risk assessment and portfolio management. These practical uses underscore the importance of test statistics in driving evidence-based decisions. To begin, let's consider how test statistics play out in real-world scenarios, where their application can have direct and significant consequences.
Real-World Scenarios
In real-world scenarios, test statistics play a crucial role in making informed decisions across various fields. For instance, in medical research, test statistics are used to determine the efficacy of new treatments. Imagine a clinical trial comparing the outcomes of a new drug versus a placebo. Researchers calculate test statistics such as the t-statistic or z-score to assess whether the observed differences in patient outcomes are statistically significant, thereby guiding decisions on whether to approve the drug for market use. Similarly, in finance, test statistics help analysts evaluate investment strategies. For example, a portfolio manager might use statistical tests to compare the performance of different investment portfolios, ensuring that any observed differences are not due to chance but rather reflect genuine variations in strategy effectiveness. In quality control, manufacturing companies rely on test statistics to monitor product quality. By conducting hypothesis tests on sample data, manufacturers can determine if there are significant deviations from expected quality standards, allowing them to adjust production processes accordingly. In social sciences, researchers use test statistics to analyze survey data and understand public opinions or behaviors. For example, political analysts might employ statistical tests to gauge the significance of shifts in public opinion polls before an election, helping them predict voter behavior more accurately. Moreover, in environmental studies, scientists use test statistics to assess changes in ecological systems. For instance, they might conduct t-tests or ANOVA (Analysis of Variance) to determine if there is a statistically significant impact of pollution on wildlife populations or if climate change is affecting temperature patterns over time. These analyses are crucial for developing evidence-based policies aimed at mitigating environmental issues. In education, test statistics are essential for evaluating educational programs and assessing student performance. Educators can use statistical tests to compare the academic achievements of students who have undergone different teaching methods or curricula, helping them identify which approaches are most effective. Additionally, in marketing research, businesses utilize test statistics to measure the impact of advertising campaigns on consumer behavior. By analyzing data from controlled experiments or surveys using statistical tests like chi-square tests or regression analysis, marketers can determine whether their campaigns have had a significant effect on sales or brand awareness. These examples illustrate how test statistics are integral to decision-making processes across diverse sectors by providing a quantitative framework for evaluating hypotheses and making data-driven conclusions. By applying these statistical tools effectively, professionals can ensure that their decisions are grounded in empirical evidence rather than intuition alone. This not only enhances the reliability of their findings but also fosters a culture of data-driven decision-making that drives innovation and improvement in various fields.
Case Studies and Examples
In the realm of statistical analysis, case studies and examples serve as the backbone for illustrating the practical applications and interpretations of test statistics. These real-world scenarios not only validate theoretical concepts but also provide tangible insights into how statistical methods can be applied to solve real problems. For instance, in medical research, a case study might involve comparing the efficacy of two different treatments for a specific disease using a t-test or ANOVA. Here, the test statistic would help determine whether there is a significant difference in treatment outcomes, guiding healthcare professionals in making informed decisions. In business, case studies often focus on market trends and consumer behavior. For example, a company might conduct an A/B testing experiment to see which marketing strategy yields higher sales. The test statistic, such as the z-score or chi-square statistic, would indicate whether the observed differences between the two strategies are statistically significant or merely due to chance. This information is crucial for optimizing marketing budgets and strategies. Educational institutions also benefit from case studies involving test statistics. For example, researchers might use regression analysis to study the relationship between student performance and various factors such as socio-economic status, teacher experience, and school resources. The resulting test statistics would help identify which factors have a significant impact on student outcomes, enabling policymakers to allocate resources more effectively. Furthermore, environmental studies frequently employ test statistics to analyze data on pollution levels, climate change, and conservation efforts. A case study might involve comparing pollution levels in different regions using a non-parametric test like the Wilcoxon rank-sum test. The findings could inform policy decisions regarding environmental regulations and conservation strategies. In addition to these fields, case studies in psychology use test statistics to understand human behavior and mental health. For instance, a study on the effectiveness of cognitive-behavioral therapy (CBT) might use a paired t-test to compare pre- and post-treatment scores for patients. This would help clinicians evaluate the efficacy of CBT and make recommendations for treatment protocols. These diverse examples underscore the versatility and importance of test statistics in various domains. By analyzing real-world data through case studies, researchers and practitioners can draw meaningful conclusions that drive decision-making processes. Ultimately, these applications highlight how test statistics are not just abstract concepts but powerful tools for solving real-world problems and advancing knowledge across multiple disciplines.
Practical Uses in Research
In the realm of statistical analysis, test statistics play a pivotal role in various research fields, offering practical uses that are both diverse and indispensable. One of the primary applications of test statistics is in hypothesis testing, where they help researchers determine whether observed data support or reject a null hypothesis. For instance, in medical research, test statistics such as the t-statistic or z-score are used to evaluate the efficacy of new treatments. By comparing the mean outcomes of treatment and control groups, researchers can statistically determine if the observed differences are due to chance or if they are significant, thereby guiding clinical decisions. In social sciences, test statistics are crucial for understanding population trends and behaviors. For example, chi-square tests are often employed to analyze categorical data, helping researchers identify associations between variables such as gender and voting behavior or educational attainment and career choices. This information is vital for policymakers who need data-driven insights to formulate effective policies. In business and economics, test statistics are essential for making informed decisions. The F-statistic, for instance, is used in regression analysis to assess the overall significance of a model, helping analysts determine if the predictors are useful in explaining the dependent variable. This is particularly important in forecasting models where accurate predictions can significantly impact business strategies and investment decisions. Moreover, test statistics are integral in quality control processes within manufacturing industries. Here, statistical process control (SPC) uses test statistics like the p-value to monitor production processes and detect any deviations from expected standards. This ensures that products meet quality specifications, reducing the risk of defects and improving overall efficiency. Furthermore, in environmental studies, test statistics are used to analyze data from field observations and experiments. For example, ANOVA (Analysis of Variance) tests help researchers compare means across different groups, such as comparing the impact of different pollutants on plant growth. This helps in understanding the effects of environmental changes and informing conservation efforts. Additionally, in educational research, test statistics are employed to evaluate the effectiveness of teaching methods or curriculum changes. By using test statistics like the Cohen's d effect size, researchers can quantify the magnitude of differences between experimental and control groups, providing valuable feedback for educators and policymakers. In summary, test statistics are not just theoretical constructs but have numerous practical applications across various disciplines. They provide a robust framework for making data-driven decisions, ensuring that conclusions drawn from data are reliable and statistically valid. Whether it's in medicine, social sciences, business, manufacturing, environmental studies, or education, test statistics serve as a critical tool for researchers to interpret data accurately and meaningfully.