What Is A Sampling Frame
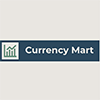
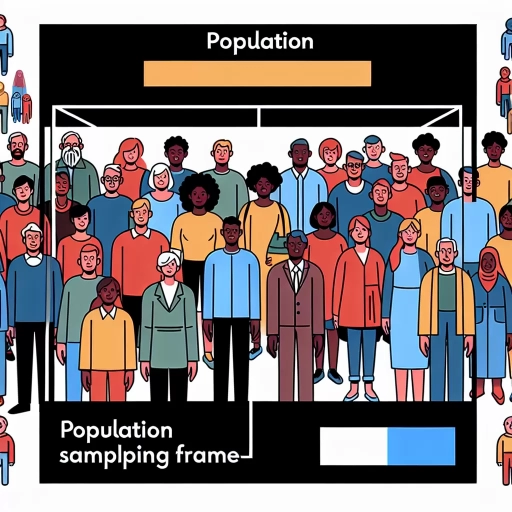
In the realm of statistical research and data analysis, the concept of a sampling frame is crucial for ensuring the accuracy and reliability of study results. A sampling frame serves as the foundation from which a sample is drawn, acting as a list or database of all potential participants or units that could be included in a study. Understanding what constitutes a sampling frame is essential for researchers to make informed decisions about their study design. This article delves into the definition and purpose of a sampling frame, exploring its role in research methodology. We will also examine the various types and characteristics of sampling frames, highlighting their unique features and applications. Additionally, we will discuss best practices for constructing and using sampling frames effectively. By grasping these fundamental aspects, researchers can enhance the validity and generalizability of their findings. Let us begin by defining and understanding the purpose of a sampling frame, which sets the stage for a comprehensive exploration of this critical research tool.
Definition and Purpose of a Sampling Frame
In the realm of statistical analysis and research, the concept of a sampling frame is pivotal for ensuring the accuracy and reliability of data. A sampling frame serves as the foundation from which a sample is drawn, and its definition and conceptual understanding are crucial for researchers. This article delves into the multifaceted role of a sampling frame, exploring its definition and conceptual underpinnings, its role in statistical analysis and research, and its importance in ensuring representative samples. By understanding what constitutes a sampling frame and how it is conceptually grounded, researchers can better appreciate its significance in statistical processes. Furthermore, recognizing the role of a sampling frame in statistical analysis highlights its practical applications and impact on research outcomes. Ultimately, the importance of a sampling frame in ensuring representative samples underscores its critical function in maintaining the integrity of research findings. To begin, let us first explore the definition and conceptual understanding of a sampling frame, laying the groundwork for a deeper analysis of its broader implications.
1. Definition and Conceptual Understanding
A sampling frame is a foundational concept in statistical research, serving as the blueprint from which a sample is drawn to represent a larger population. It is essentially a list or database of all the units from which the sample will be selected. The definition and conceptual understanding of a sampling frame are crucial because they determine the accuracy and reliability of the research findings. To grasp this concept fully, it's important to differentiate between the population and the sampling frame. The population refers to the entire group of individuals or units that the researcher aims to study, while the sampling frame is the accessible list of these units from which the sample will be chosen. For instance, if a researcher wants to study the opinions of all college students in a country, the population would be all college students, but the sampling frame might be a list of students registered in universities that are easily accessible for data collection. The quality of the sampling frame directly impacts the validity of the research. An ideal sampling frame should be comprehensive, meaning it includes all members of the population of interest, and it should be free from errors or biases. However, in practice, creating a perfect sampling frame can be challenging due to limitations such as incomplete records or difficulties in accessing certain segments of the population. For example, if a researcher relies on voter registration lists as a sampling frame for studying public opinion, they may inadvertently exclude non-registered voters who are still part of the population. Understanding these nuances is vital because they influence how representative the sample will be of the broader population. A well-defined sampling frame ensures that every member of the population has an equal chance of being selected, which is a key principle in achieving randomness and minimizing bias. This randomness helps in generalizing the findings from the sample to the entire population with greater confidence. Moreover, the conceptual understanding of a sampling frame extends beyond just its definition; it involves recognizing its role in research design. It guides the selection process and ensures that the sample is not only representative but also sufficient in size to provide reliable estimates. For instance, in market research, companies might use customer databases as their sampling frame to gather feedback on new products. Here, ensuring that the database is up-to-date and inclusive is critical for obtaining meaningful insights. In summary, the definition and conceptual understanding of a sampling frame are pivotal in research methodology. It acts as a bridge between the theoretical population and the practical sample, ensuring that research findings are robust and applicable to real-world scenarios. By meticulously defining and refining the sampling frame, researchers can enhance the credibility and applicability of their studies, ultimately contributing to more informed decision-making across various fields.
2. Role in Statistical Analysis and Research
In the realm of statistical analysis and research, the role of a sampling frame is pivotal. A sampling frame serves as the foundation from which a sample is drawn, ensuring that the selected participants accurately represent the broader population. This foundational element is crucial for several reasons. First, it helps in avoiding biases that could skew the results of the study. By defining a clear and comprehensive sampling frame, researchers can ensure that all members of the population have an equal chance of being selected, thereby enhancing the generalizability of the findings. For instance, in a study aimed at understanding consumer preferences, a well-defined sampling frame might include all registered voters in a particular region, ensuring that the sample reflects the diverse demographics and opinions of the entire population. Second, a sampling frame facilitates the calculation of sampling errors and confidence intervals. When researchers know the total number of elements in the sampling frame, they can estimate how representative their sample is likely to be. This statistical precision is essential for drawing reliable conclusions and making informed decisions based on the data. For example, in epidemiological studies, a sampling frame that includes all residents of a specific area allows researchers to accurately estimate disease prevalence rates and identify risk factors. Third, a sampling frame aids in the replication and validation of research findings. By clearly defining the population from which the sample is drawn, other researchers can replicate the study using the same criteria, thereby verifying or challenging the original results. This process of replication is a cornerstone of scientific research, as it helps to build a body of evidence that is robust and reliable. In fields such as psychology or sociology, where studies often involve complex variables and nuanced interpretations, a well-defined sampling frame ensures that subsequent studies can be compared and integrated into a cohesive understanding. Moreover, a sampling frame plays a critical role in ensuring ethical standards in research. By clearly outlining who is included and excluded from the study, researchers can avoid inadvertently marginalizing certain groups or introducing ethical dilemmas. For example, in studies involving vulnerable populations such as children or the elderly, a carefully defined sampling frame helps ensure that these groups are treated with respect and dignity while also protecting their rights. Finally, the role of a sampling frame extends beyond data collection to data analysis and interpretation. It provides a context within which the data can be understood and interpreted. For instance, in market research, knowing the specific demographics and characteristics of the sampling frame allows analysts to tailor their recommendations to the target audience more effectively. This contextual understanding is essential for translating raw data into actionable insights that can inform policy decisions or business strategies. In summary, the role of a sampling frame in statistical analysis and research is multifaceted and indispensable. It ensures representativeness, facilitates statistical precision, aids in replication and validation, upholds ethical standards, and provides context for data interpretation. By meticulously defining and utilizing a sampling frame, researchers can enhance the validity, reliability, and generalizability of their findings, ultimately contributing to more informed decision-making across various fields.
3. Importance in Ensuring Representative Samples
Ensuring representative samples is crucial in the context of a sampling frame, as it directly impacts the validity and reliability of research findings. A representative sample is one that accurately reflects the characteristics of the population from which it is drawn, thereby allowing researchers to generalize their results with confidence. Here are three key reasons why ensuring representative samples is essential: 1. **Accurate Generalization**: The primary goal of sampling is to make inferences about a larger population based on a smaller subset. If the sample is not representative, any conclusions drawn from it may not accurately reflect the population's characteristics. For instance, if a study on consumer behavior only includes participants from urban areas, it would be misleading to generalize those findings to rural populations. Ensuring representativeness helps researchers avoid such biases and ensures that their conclusions are applicable to the broader population. 2. **Reducing Sampling Bias**: Sampling bias occurs when the sample does not accurately represent the population due to systematic errors in the sampling process. This can happen if certain groups are overrepresented or underrepresented in the sample. For example, if a survey about health habits only includes individuals who are easily accessible through social media, it may miss out on older adults who are less likely to use these platforms. By ensuring representativeness, researchers can minimize such biases and increase the reliability of their data. 3. **Enhancing Statistical Power**: Representative samples also enhance the statistical power of a study. Statistical power refers to the ability of a test to detect an effect if there is one. A sample that is representative of the population provides a more robust dataset, allowing for more precise estimates and stronger statistical analyses. This is particularly important in fields like medicine and social sciences where small effects can have significant implications. By ensuring that samples are representative, researchers can increase their chances of detecting meaningful differences or relationships within the data. In summary, ensuring representative samples is vital for maintaining the integrity of research. It allows for accurate generalization, reduces sampling bias, and enhances statistical power. These factors collectively contribute to the reliability and validity of research findings, making them more trustworthy and applicable in real-world contexts. Therefore, careful attention must be given to ensuring that samples are as representative as possible when constructing a sampling frame.
Types and Characteristics of Sampling Frames
When discussing the types and characteristics of sampling frames, it is crucial to delve into the various methods and considerations that underpin effective sampling. A comprehensive understanding of sampling frames involves examining three key areas: population-based sampling frames, list-based sampling frames, and the potential errors and limitations associated with these approaches. Population-based sampling frames focus on selecting samples from the entire population, ensuring that every individual has an equal chance of being included. List-based sampling frames, on the other hand, rely on pre-existing lists or databases to select participants. However, both methods are not without their challenges; frame errors and limitations can significantly impact the accuracy and representativeness of the sample. By understanding these different types of sampling frames and their inherent issues, researchers can make informed decisions to ensure the validity and reliability of their studies. This article will explore each of these aspects in detail, starting with an in-depth look at population-based sampling frames.
1. Population-Based Sampling Frames
**Population-Based Sampling Frames** Population-based sampling frames are foundational in statistical research, ensuring that the sample accurately represents the entire population of interest. These frames are constructed by identifying and listing all members of the target population, which can be individuals, households, businesses, or any other relevant units. The key characteristic of population-based sampling frames is their comprehensiveness; they aim to include every element within the defined population boundaries. For instance, in a study on national health trends, a population-based sampling frame might consist of a list of all residents within a country, obtained from census data or national health registries. The construction of these frames involves meticulous data collection and validation to ensure accuracy and completeness. This process often relies on official records such as voter registration lists, tax records, or census data. In some cases, researchers may also use administrative databases like school enrollment records or employment registries to create a robust sampling frame. The advantages of population-based sampling frames include reduced bias and increased generalizability of findings to the broader population. However, challenges such as data privacy concerns, outdated records, and resource-intensive data collection processes can complicate their development. Despite these challenges, population-based sampling frames are crucial for conducting reliable and representative research. They enable researchers to apply various sampling techniques like simple random sampling, stratified sampling, or cluster sampling with confidence that the sample will reflect the characteristics of the entire population. For example, in epidemiological studies aimed at understanding disease prevalence, a population-based sampling frame ensures that the sample is representative of different demographic groups within the population, leading to more accurate estimates and better-informed public health policies. Moreover, advancements in technology have improved the efficiency and accuracy of creating population-based sampling frames. Digital databases and data analytics tools facilitate the integration of multiple data sources, enhancing the completeness and up-to-dateness of the sampling frame. Additionally, these tools help in identifying and correcting errors or inconsistencies in the data, thereby improving the reliability of the sampling frame. In conclusion, population-based sampling frames are essential for conducting rigorous and representative research. By ensuring that every member of the target population is included in the frame, these frames provide a solid foundation for drawing samples that accurately reflect the characteristics of the broader population. While their construction presents several challenges, the benefits in terms of reduced bias and increased generalizability make them a cornerstone of statistical research methodologies. As data collection and analysis technologies continue to evolve, the potential for creating more accurate and comprehensive population-based sampling frames will only grow, further enhancing the quality of research outcomes.
2. List-Based Sampling Frames
List-based sampling frames are a fundamental component in the realm of statistical sampling, offering a structured and reliable method for selecting participants or units for a study. Unlike other types of sampling frames, list-based frames rely on pre-existing lists or databases that contain detailed information about the population of interest. These lists can be derived from various sources such as voter registration rolls, customer databases, census records, or membership lists. The primary advantage of list-based sampling frames is their precision and comprehensiveness. Since these frames are built from existing records, they often provide a complete and accurate representation of the target population. For instance, in market research, a company might use a customer database to create a sampling frame that includes all current customers, allowing for targeted and relevant data collection. Similarly, in epidemiological studies, health registries can serve as robust sampling frames to identify and sample individuals with specific health conditions. Another significant benefit of list-based sampling frames is their ability to facilitate stratified sampling. By categorizing the population into distinct subgroups based on the information available in the list (e.g., age, gender, geographic location), researchers can ensure that each subgroup is adequately represented in the sample. This stratification enhances the reliability and generalizability of the findings, as it helps to mitigate biases that might arise from underrepresentation or overrepresentation of certain segments. However, list-based sampling frames also come with some challenges. One major concern is the issue of outdated or incomplete data. Lists may not always reflect recent changes or updates, leading to potential biases if the sample is drawn from an outdated frame. For example, a voter registration list might not account for recent movers or new voters, which could skew the results of a political survey. Additionally, ensuring the privacy and confidentiality of individuals listed in these frames is crucial, especially when dealing with sensitive information. To address these challenges, researchers must regularly update and validate their lists. This involves cross-checking with other data sources and implementing robust data cleaning procedures to remove duplicates or incorrect entries. Furthermore, ethical considerations must be prioritized by anonymizing personal data and adhering to strict confidentiality protocols. In conclusion, list-based sampling frames offer a powerful tool for researchers seeking to conduct rigorous and accurate studies. Their ability to provide comprehensive and detailed representations of the target population makes them invaluable in various fields. However, it is essential to be mindful of the potential pitfalls associated with these frames and to take proactive steps to ensure their accuracy and ethical use. By doing so, researchers can leverage list-based sampling frames to gather high-quality data that supports informed decision-making and contributes meaningfully to their respective fields.
3. Frame Errors and Limitations
When discussing the types and characteristics of sampling frames, it is crucial to address the potential frame errors and limitations that can significantly impact the accuracy and reliability of research findings. Frame errors refer to the discrepancies between the sampling frame and the target population, which can lead to biased or incomplete data. One common type of frame error is **undercoverage**, where certain segments of the target population are not included in the sampling frame. For instance, in a study aiming to survey all residents of a city, if the sampling frame only includes landline phone numbers, it may exclude younger residents who primarily use mobile phones or those without any phone service at all. This undercoverage can result in a biased sample that does not accurately represent the entire population. Another significant issue is **overcoverage**, where individuals or units are included in the sampling frame more than once or are included when they should not be. This can happen if a database contains duplicate entries or if individuals are listed under different categories (e.g., both as individuals and as part of a household). Overcoverage can lead to an inflated sample size and skew the results, making it difficult to draw valid conclusions. **Non-response errors** also pose a challenge. Even with a well-constructed sampling frame, some individuals may refuse to participate or cannot be reached. This non-response can introduce bias if those who do not respond differ systematically from those who do. For example, in a survey about health habits, individuals with poor health might be less likely to respond, leading to an overestimation of the population's overall health. In addition to these errors, there are several limitations inherent in sampling frames. **Outdated information** is a common limitation; databases used for sampling frames may not be updated regularly, leading to inaccuracies such as incorrect addresses or contact details. This can result in wasted resources and reduced response rates. **Sampling frame mismatch** is another limitation where the sampling frame does not align with the research objectives. For example, if a study aims to understand consumer behavior but uses an employee database as its sampling frame, the results will likely be irrelevant and misleading. Finally, **cost and accessibility constraints** can limit the quality of a sampling frame. Constructing an ideal sampling frame that includes every member of the target population can be expensive and time-consuming. Researchers often have to balance between achieving comprehensive coverage and managing resources effectively. In conclusion, understanding and addressing frame errors and limitations are essential steps in ensuring that a sampling frame accurately represents the target population. By recognizing potential pitfalls such as undercoverage, overcoverage, non-response errors, outdated information, sampling frame mismatch, and cost constraints, researchers can take proactive measures to mitigate these issues and enhance the validity of their research findings. This careful consideration helps in creating robust and reliable data that support meaningful conclusions about the population being studied.
Best Practices for Constructing and Using Sampling Frames
When constructing and using sampling frames, several best practices are crucial to ensure the integrity and reliability of the data collected. At the heart of these practices lies the meticulous identification of the target population, which sets the foundation for all subsequent steps. This involves clearly defining the demographic, geographic, and other relevant characteristics of the population to be sampled. However, identifying the target population is just the beginning. Ensuring frame coverage and accuracy is equally important, as it involves verifying that the sampling frame includes all members of the target population and that the information within the frame is up-to-date and correct. Additionally, addressing non-sampling errors, such as non-response bias and measurement errors, is vital to maintain the validity of the data. By focusing on these three key areas—identifying the target population, ensuring frame coverage and accuracy, and addressing non-sampling errors—researchers can create robust sampling frames that yield reliable and actionable insights. Let's start by delving into the critical step of identifying the target population.
1. Identifying the Target Population
Identifying the target population is a crucial step in constructing and using sampling frames, as it sets the foundation for ensuring that the sample accurately represents the broader group you aim to study. The target population refers to the entire group of individuals or entities that you are interested in understanding or describing. To identify this population, you must clearly define its boundaries and characteristics. This involves specifying demographic criteria such as age, gender, geographic location, and other relevant attributes that distinguish your population from others. For instance, if you are conducting a study on the health habits of young adults in urban areas, your target population would be individuals aged 18-35 living in cities. It is essential to be precise in this definition to avoid including or excluding individuals who do not fit your criteria. Once defined, this population serves as the universe from which your sample will be drawn. Understanding the target population also helps in identifying potential biases and ensuring that your sampling frame is comprehensive and representative. For example, if you are studying consumer behavior among families with children, you need to consider factors like household income, family size, and parental education levels to ensure that your sample reflects the diversity within this population. Moreover, accurately identifying the target population allows for better resource allocation and data collection strategies. It helps in determining the appropriate sampling method—whether it should be random, stratified, or cluster sampling—based on the characteristics of your population. This step also aids in estimating sample size requirements and ensuring that data collection tools are tailored to capture relevant information from this specific group. In practice, researchers often use existing data sources such as census records, administrative databases, or previous studies to help delineate their target population. These sources can provide valuable insights into demographic distributions and other key characteristics that define your population of interest. Ultimately, the precision with which you identify your target population directly impacts the validity and reliability of your research findings. A well-defined target population ensures that your sampling frame is robust and that any conclusions drawn from your sample can be generalized back to the larger group with confidence. Therefore, investing time and effort into this initial step is crucial for constructing a reliable sampling frame that supports high-quality research outcomes.
2. Ensuring Frame Coverage and Accuracy
Ensuring frame coverage and accuracy is a critical component of constructing and using sampling frames effectively. A well-crafted sampling frame must comprehensively capture the population of interest while minimizing errors and biases. To achieve this, several best practices should be adhered to. First, it is essential to define the target population clearly and precisely. This involves specifying the criteria for inclusion and exclusion to ensure that the frame accurately reflects the population you aim to study. For instance, if you are conducting a survey on college students, your frame should include all enrolled students but exclude non-students and alumni. Next, the source of the sampling frame must be reliable and up-to-date. Using outdated or incomplete lists can lead to undercoverage or overcoverage, both of which can skew your results. For example, using last year's student directory might miss new students or include those who have graduated. Regularly updating your frame from trusted sources such as official records or databases helps maintain its accuracy. Another crucial aspect is ensuring that the frame is free from duplicates and errors. Duplicates can lead to over-sampling certain individuals, while errors in data entry or collection can result in incorrect contact information or demographic details. Implementing data validation checks and using unique identifiers can help mitigate these issues. Additionally, it is important to consider the potential for non-response and frame non-coverage. Some individuals may not be reachable through the chosen frame (e.g., those without internet access if an online survey is used), which could introduce bias into your sample. Strategies such as multiple contact methods (e.g., email, phone, mail) and follow-up attempts can help maximize response rates. Moreover, ensuring that the sampling frame is representative of the population's diversity is vital. This involves checking for any systematic exclusions that might occur due to the method of frame construction. For example, if a survey relies on social media platforms for recruitment, it may miss older adults who are less likely to use these platforms. Finally, it is beneficial to conduct pilot tests and pre-tests on a small subset of the sampling frame before full-scale implementation. This allows for the identification and rectification of any issues related to coverage and accuracy before they affect the entire study. By adhering to these best practices—clearly defining the target population, using reliable sources, ensuring data integrity, addressing non-response and non-coverage issues, maintaining representativeness, and conducting pilot tests—you can significantly enhance the quality of your sampling frame. This ultimately leads to more accurate and reliable research findings that better reflect the characteristics of your population of interest.
3. Addressing Non-Sampling Errors
When constructing and using sampling frames, one of the critical aspects to address is non-sampling errors. These errors arise from sources other than the sampling process itself and can significantly impact the accuracy and reliability of survey results. Non-sampling errors include issues such as non-response, measurement errors, and data processing errors. **Non-Response Errors:** One of the most common types of non-sampling errors is non-response, where some members of the sample do not provide data. This can occur due to various reasons such as lack of interest, unavailability, or refusal to participate. To mitigate this, researchers should employ strategies like multiple follow-ups, incentives for participation, and ensuring that the survey is user-friendly and relevant to the respondents. For instance, using multiple contact methods (e.g., email, phone, mail) can increase response rates by catering to different preferences. **Measurement Errors:** Measurement errors occur when there is a discrepancy between the true value and the observed value due to flaws in the data collection instrument or process. This could be due to poorly worded questions, inadequate training of interviewers, or biases in the measurement tools. To address this, it is essential to pre-test the survey instruments thoroughly to identify and correct any ambiguities or biases. Additionally, ensuring that interviewers are well-trained and using standardized protocols can help minimize variability in data collection. **Data Processing Errors:** Data processing errors involve mistakes made during the handling and analysis of data. These can include coding errors, data entry mistakes, and incorrect application of statistical methods. Implementing robust quality control measures such as double data entry, automated checks for inconsistencies, and thorough validation processes can help reduce these errors. Furthermore, using advanced statistical software that includes built-in validation tools can also enhance data integrity. Incorporating best practices for addressing non-sampling errors into the construction and use of sampling frames is crucial for ensuring the validity and reliability of research findings. By proactively managing non-response through effective follow-up strategies, minimizing measurement errors through rigorous pre-testing and training, and implementing stringent data processing protocols, researchers can significantly enhance the quality of their data. This not only improves the accuracy of their conclusions but also builds trust in their research among stakeholders and the broader scientific community. Ultimately, addressing non-sampling errors is an integral part of constructing a robust sampling frame that supports high-quality research outcomes.