What Is A Controlled Variable
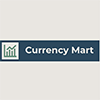
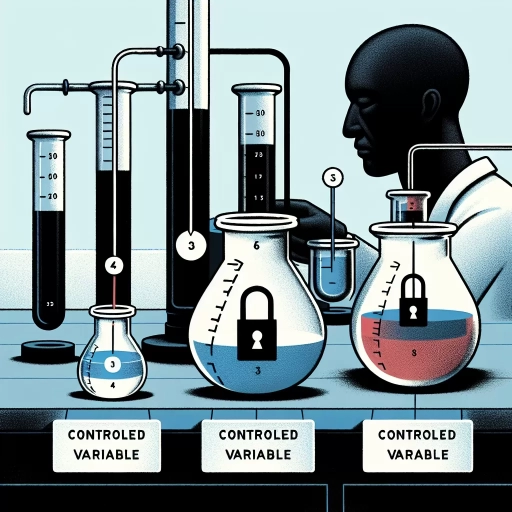
In the realm of scientific research, precision and control are paramount to ensuring the validity and reliability of experimental results. One crucial element in achieving this precision is the controlled variable, a factor that is deliberately kept constant to prevent it from influencing the outcome of an experiment. Understanding the concept of controlled variables is essential for any researcher aiming to draw accurate conclusions. This article delves into the intricacies of controlled variables, starting with a comprehensive explanation of what they are and why they are vital in experimental design. We will then explore the process of identifying and selecting appropriate controlled variables, highlighting the importance of careful consideration in this step. Finally, we will discuss how these variables are implemented in real-world research scenarios, illustrating their practical significance. By grasping these key aspects, researchers can ensure that their experiments yield meaningful data. Let us begin by understanding the concept of controlled variables, a foundational element in scientific inquiry.
Understanding the Concept of Controlled Variables
In the realm of scientific inquiry, understanding the concept of controlled variables is paramount for conducting rigorous and reliable experiments. Controlled variables are crucial elements that researchers must carefully manage to ensure the integrity and validity of their findings. This article delves into the definition and importance of controlled variables, highlighting their essential role in maintaining experimental consistency and accuracy. We will explore how controlled variables function within scientific experiments, enabling researchers to isolate the effects of independent variables on dependent variables. Additionally, we will clarify the distinctions between controlled variables and their counterparts, independent and dependent variables, to provide a comprehensive understanding of their interplay. By grasping these concepts, scientists can design experiments that yield meaningful results, contributing significantly to the advancement of knowledge in various fields. Understanding the concept of controlled variables is fundamental to scientific methodology, and this article aims to provide a clear and detailed explanation of this critical aspect of experimental design.
Definition and Importance
In the realm of scientific experimentation, understanding the concept of controlled variables is paramount for ensuring the validity and reliability of research findings. A controlled variable, also known as a constant or independent variable, is a factor that is intentionally kept constant or unchanged throughout an experiment to prevent it from influencing the outcome. This meticulous control allows researchers to isolate the effect of the independent variable on the dependent variable, thereby establishing cause-and-effect relationships. The importance of controlled variables cannot be overstated. By maintaining these constants, scientists can eliminate confounding variables that might skew results, leading to inaccurate conclusions. For instance, in a study examining the impact of light intensity on plant growth, factors such as temperature, water supply, and soil composition would be controlled to ensure that only light intensity affects the outcome. This precision helps in drawing clear, unbiased conclusions about the relationship between light and plant growth. Moreover, controlled variables enhance the reproducibility of experiments. When all extraneous factors are held constant, other researchers can replicate the study under identical conditions, verifying or challenging the original findings. This reproducibility is a cornerstone of scientific inquiry, as it fosters a community of trust and continuous improvement in knowledge. Additionally, controlling variables aids in minimizing experimental error. By stabilizing potential sources of variation, researchers reduce the likelihood of random fluctuations affecting their data. This not only increases the precision of measurements but also strengthens the statistical analysis, making it easier to detect significant effects. In practical terms, understanding controlled variables is crucial for real-world applications. For example, in medical research, controlling for age, gender, and pre-existing conditions helps in determining the efficacy and safety of new treatments. Similarly, in engineering, controlling environmental factors like humidity and temperature ensures that product testing reflects real-world performance accurately. In conclusion, the definition and importance of controlled variables are fundamental to rigorous scientific methodology. By meticulously controlling these constants, researchers ensure that their experiments yield reliable, reproducible results that contribute meaningfully to our understanding of various phenomena. This attention to detail is what distinguishes high-quality research from anecdotal observations, making it indispensable for advancing knowledge across diverse fields.
Role in Scientific Experiments
In scientific experiments, the role of controlled variables is pivotal in ensuring the integrity and reliability of the results. Controlled variables, also known as constants or fixed variables, are factors that are deliberately kept unchanged throughout the experiment to prevent any potential influence on the outcome. This meticulous control allows researchers to isolate the independent variable—the factor being tested—and observe its effect on the dependent variable—the outcome being measured—without any confounding effects. For instance, in a study examining how different temperatures affect the growth rate of a specific plant species, temperature would be the independent variable. To ensure accurate results, other factors such as light exposure, soil composition, water supply, and humidity must be controlled. By maintaining these variables at constant levels across all experimental groups, researchers can confidently attribute any observed differences in plant growth to the varying temperatures rather than other environmental factors. The importance of controlling variables extends beyond just isolating the effect of the independent variable; it also enhances the reproducibility of the experiment. When all extraneous variables are held constant, other researchers can replicate the study under identical conditions, thereby validating or challenging the initial findings. This reproducibility is a cornerstone of scientific methodology, as it allows for the verification and refinement of hypotheses over time. Moreover, controlling variables helps in minimizing experimental error. Uncontrolled variables can introduce random or systematic errors that may skew the results, leading to misleading conclusions. By controlling these variables, researchers can reduce variability within their data sets and increase the precision of their measurements. This precision is crucial for drawing meaningful conclusions and making informed decisions based on empirical evidence. In addition to these methodological benefits, controlling variables also fosters a deeper understanding of complex systems. By systematically varying one factor while keeping others constant, scientists can uncover intricate relationships between different components within a system. This approach has been instrumental in numerous groundbreaking discoveries across various fields, from physics and chemistry to biology and psychology. In summary, the role of controlled variables in scientific experiments is multifaceted and indispensable. By maintaining these variables at constant levels, researchers ensure that their findings are reliable, reproducible, and free from confounding influences. This rigorous control over experimental conditions not only enhances the validity of scientific studies but also contributes significantly to our broader understanding of the natural world.
Distinguishing from Independent and Dependent Variables
When delving into the realm of scientific experimentation, it is crucial to distinguish between independent and dependent variables, as this distinction forms the backbone of any well-designed study. The **independent variable** is the factor that the experimenter manipulates or changes to observe its effect on the outcome. It is essentially the cause or input in the experiment. For instance, if you are investigating how different levels of sunlight affect plant growth, the amount of sunlight would be your independent variable because you are intentionally varying it to see its impact. On the other hand, the **dependent variable** is the outcome or result that you measure in response to changes made to the independent variable. It represents the effect or output of the experiment. Continuing with our plant growth example, the dependent variable would be the height or biomass of the plants after exposure to different sunlight levels. This is what you are measuring to see how it changes in response to your manipulation of sunlight. Understanding this distinction is vital because it allows researchers to isolate cause-and-effect relationships and draw meaningful conclusions from their data. Without clear identification of independent and dependent variables, experiments can become muddled, leading to ambiguous results that fail to provide insights into the underlying mechanisms being studied. Moreover, recognizing these variables helps in controlling other factors that could influence the outcome—known as **controlled variables**—to ensure that any observed changes are indeed due to the manipulation of the independent variable rather than extraneous factors. For example, in our plant growth study, factors like soil type, water amount, and temperature would need to be controlled (kept constant) across all experimental groups to prevent them from confounding the results. In summary, distinguishing between independent and dependent variables is essential for conducting rigorous scientific experiments. By clearly defining these variables and controlling for other potential influences, researchers can establish robust cause-and-effect relationships and contribute meaningfully to their field of study. This foundational understanding is a critical component of mastering the concept of controlled variables, which ensures that experiments yield reliable and interpretable results.
Identifying and Selecting Controlled Variables
In the realm of scientific research, the meticulous identification and selection of controlled variables are crucial for ensuring the validity and reliability of experimental results. Controlled variables are factors that must be kept constant to prevent them from influencing the outcome of an experiment, thereby allowing researchers to isolate the effect of the independent variable on the dependent variable. This article delves into the essential aspects of identifying and selecting these variables, providing a comprehensive guide for researchers. We will explore **Factors to Consider in Experimental Design**, highlighting the importance of careful planning and consideration of potential confounding variables. Additionally, we will examine **Common Examples of Controlled Variables**, illustrating how these factors manifest in various scientific contexts. Finally, we will discuss **Tools and Methods for Identifying Controlled Variables**, outlining practical approaches to identifying and managing these critical elements. By understanding these concepts, researchers can enhance the rigor of their experiments, leading to more accurate and meaningful conclusions. Understanding the concept of controlled variables is fundamental to conducting robust scientific research, and this article aims to provide a detailed exploration of this critical aspect.
Factors to Consider in Experimental Design
When designing an experiment, several critical factors must be meticulously considered to ensure the integrity and reliability of the results. One of the most crucial aspects is identifying and selecting controlled variables, which are factors that could potentially influence the outcome of the experiment but are kept constant to isolate the effect of the independent variable. Firstly, **relevance** is paramount; controlled variables should be directly related to the research question or hypothesis. For instance, in a study examining the effect of light intensity on plant growth, variables such as temperature, water supply, and soil composition must be controlled because they can significantly impact plant growth. **Precision** in measurement is another key factor. The tools and methods used to measure and control variables must be accurate and reliable. Inaccurate measurements can lead to confounding results, making it difficult to draw valid conclusions. For example, using a thermometer with a high margin of error to control temperature could introduce unnecessary variability. **Consistency** across all experimental conditions is essential. Ensuring that all controlled variables are maintained at the same level for all experimental groups helps in eliminating any bias that might arise from differences in these variables. This consistency allows researchers to attribute any observed differences solely to the independent variable being tested. Additionally, **feasibility** should be considered. Controlling certain variables might be impractical or too costly, so researchers must weigh the importance of each variable against the resources available. In some cases, compromises may need to be made without compromising the validity of the experiment. **Ethical considerations** also play a significant role, particularly in experiments involving living organisms. Ensuring that controlled variables do not cause undue stress or harm is crucial for maintaining ethical standards and compliance with regulatory guidelines. Finally, **statistical analysis** should be taken into account during the design phase. Understanding how controlled variables will be analyzed statistically helps in ensuring that the data collected will be meaningful and interpretable. This includes considering sample size, randomization techniques, and appropriate statistical tests to validate the findings. By carefully considering these factors—relevance, precision, consistency, feasibility, ethical considerations, and statistical analysis—researchers can ensure that their experimental design is robust and capable of producing reliable results. This meticulous approach to identifying and selecting controlled variables is fundamental in scientific research, as it allows for the isolation of the independent variable's effect and enhances the validity of the conclusions drawn from the experiment.
Common Examples of Controlled Variables
When conducting scientific experiments, identifying and selecting controlled variables are crucial steps to ensure the integrity and reliability of the results. Controlled variables are factors that could potentially influence the outcome of an experiment but are kept constant to isolate the effect of the independent variable on the dependent variable. Here are some common examples of controlled variables across various fields: 1. **Temperature**: In many chemical reactions and biological experiments, temperature is a critical controlled variable. For instance, in a study on the effect of pH on enzyme activity, maintaining a constant temperature ensures that any observed changes are due to pH variations rather than thermal influences. 2. **Light Exposure**: Light can significantly impact plant growth, microbial cultures, and chemical reactions. In an experiment comparing the growth of plants under different soil types, keeping light exposure constant helps to isolate the effect of soil composition on plant growth. 3. **Humidity**: Humidity levels can affect the rate of chemical reactions, microbial growth, and even mechanical properties of materials. In a study on the durability of building materials, controlling humidity ensures that any observed differences in material performance are not due to variations in moisture levels. 4. **Pressure**: Pressure is another important controlled variable, particularly in physical and chemical experiments. For example, when studying the solubility of gases in liquids at different temperatures, maintaining constant pressure ensures that changes in solubility are solely due to temperature variations. 5. **pH Levels**: In biological and chemical experiments involving enzymes or chemical reactions, pH levels must often be controlled. For instance, when investigating the optimal pH for enzyme activity, keeping other factors such as temperature and substrate concentration constant allows researchers to accurately determine how pH affects enzyme performance. 6. **Volume of Reactants**: In chemical reactions, the volume of reactants can influence reaction rates and yields. By controlling the volume of reactants, researchers can ensure that any observed changes are due to the independent variable being tested rather than differences in reactant quantities. 7. **Time**: Time is a universal controlled variable across many types of experiments. Ensuring that all samples or trials are exposed to the same duration of treatment helps eliminate time as a confounding factor. For example, in a study comparing the effects of different fertilizers on plant growth, all plants should be grown for the same amount of time. 8. **Concentration of Substances**: The concentration of substances such as solutes or reactants can significantly impact experimental outcomes. In a study on the effect of salt concentration on seed germination, controlling the concentration ensures that any observed differences in germination rates are due solely to variations in salt levels. By meticulously controlling these variables, researchers can enhance the validity and reproducibility of their findings, thereby contributing to a more robust understanding of the phenomena under investigation. This careful management of controlled variables is essential for drawing accurate conclusions and making meaningful comparisons within an experiment.
Tools and Methods for Identifying Controlled Variables
When it comes to identifying and selecting controlled variables in scientific experiments, researchers rely on a variety of tools and methods to ensure the integrity and reliability of their findings. One of the primary tools is the **experimental design**, which involves carefully planning the structure of the experiment to isolate the independent variable's effect on the dependent variable. This includes setting up control groups and treatment groups, where the control group serves as a baseline against which the treatment group's results are compared. Another crucial method is **randomization**, which helps eliminate bias by randomly assigning participants or samples to different groups. This ensures that any differences between groups are due to chance rather than systematic errors. **Blinding** is another technique used to reduce bias; it involves hiding the group assignments from either the participants (single-blind) or both the participants and the researchers (double-blind) to prevent any conscious or unconscious influences on the results. **Statistical analysis** also plays a significant role in identifying controlled variables. Researchers use statistical tests to determine whether observed differences are statistically significant, thereby confirming whether the independent variable has a real effect on the dependent variable. Tools like **ANOVA (Analysis of Variance)** and **t-tests** are commonly used for this purpose. In addition, **data visualization** tools such as graphs and charts help in identifying patterns and trends that might indicate which variables need to be controlled. For instance, scatter plots can reveal correlations between variables, while bar charts can show differences in means across groups. **Literature review** is another essential method for identifying controlled variables. By examining previous studies related to the research question, researchers can identify variables that have been found to impact the outcome in similar experiments. This helps in anticipating potential confounding variables that need to be controlled. Finally, **pilot studies** serve as a preliminary step to test the experimental design and identify any issues with controlling variables before conducting the full-scale experiment. These small-scale trials allow researchers to refine their methods and ensure that all relevant variables are accounted for. By combining these tools and methods—experimental design, randomization, blinding, statistical analysis, data visualization, literature review, and pilot studies—researchers can effectively identify and control variables that might otherwise confound their results, leading to more accurate and reliable conclusions. This meticulous approach ensures that experiments are robust and capable of producing meaningful insights into the phenomena being studied.
Implementing Controlled Variables in Research
Implementing controlled variables in research is a crucial aspect of scientific inquiry, as it ensures the integrity and reliability of experimental results. Controlled variables are factors that are kept constant to prevent them from influencing the outcome of an experiment, thereby allowing researchers to isolate and measure the effect of the independent variable. However, achieving this level of control requires meticulous planning and execution. This article delves into the strategies for maintaining consistency in controlled variables, highlighting techniques that help researchers minimize variability and ensure reproducibility. It also addresses the challenges and potential pitfalls that can arise during the process, providing insights into common mistakes and how to avoid them. Additionally, it outlines best practices for effective control, offering practical advice on how to optimize experimental design. By understanding these key elements, researchers can enhance the validity of their findings and contribute meaningfully to their field. Transitioning seamlessly into the core concept, this discussion sets the stage for a deeper exploration of **Understanding the Concept of Controlled Variables**.
Strategies for Maintaining Consistency
Maintaining consistency is a crucial aspect of implementing controlled variables in research, as it ensures the reliability and validity of the findings. To achieve this, researchers must employ several strategies that help in standardizing the experimental conditions. First, **standard operating procedures (SOPs)** should be developed and strictly adhered to. These protocols outline every step of the experiment, from data collection to analysis, ensuring that each trial is conducted in an identical manner. This minimizes human error and variability, which can skew results. Another key strategy is **calibration and validation** of all equipment and instruments used in the experiment. Regular checks on instruments ensure that they are functioning correctly and consistently, thereby reducing any potential biases or inaccuracies. Additionally, **environmental control** is essential; factors such as temperature, humidity, and lighting should be kept constant across all trials to prevent external influences from affecting the outcomes. **Training and consistency among researchers** also play a significant role. All team members involved in the experiment should undergo thorough training to ensure they follow the same procedures and interpret data uniformly. This helps in reducing inter-rater reliability issues where different researchers might interpret or record data differently. Moreover, **data management** practices should be rigorous. Using standardized data collection forms and databases can help in maintaining uniformity in how data is recorded and analyzed. Automated systems can also be employed to minimize manual errors during data entry. Finally, **quality control checks** at various stages of the experiment are vital. Regular audits and peer reviews can help identify any deviations from the established protocols early on, allowing for prompt corrections before they impact the overall results. By implementing these strategies—SOPs, calibration/validation of equipment, environmental control, training of researchers, robust data management practices, and quality control checks—researchers can ensure that their experiments are conducted with high consistency. This not only enhances the credibility of their findings but also facilitates replication by other researchers, which is a cornerstone of scientific research. Consistency in controlled variables is what differentiates rigorous scientific inquiry from anecdotal evidence, making it indispensable for drawing reliable conclusions.
Challenges and Potential Pitfalls
When implementing controlled variables in research, several challenges and potential pitfalls must be carefully navigated to ensure the integrity and reliability of the study. One of the primary challenges is maintaining consistency across all experimental conditions. Even minor variations in the controlled variables can introduce unintended confounding factors, which may skew the results and lead to misleading conclusions. For instance, in a study examining the effect of temperature on plant growth, if the temperature is not precisely controlled, it could result in different growth rates that are not due to the independent variable being tested. Another significant pitfall is the risk of human error. Researchers may inadvertently introduce bias through their interactions with the experiment, such as handling samples differently or using slightly different techniques each time. To mitigate this, strict protocols and training are essential to standardize procedures and minimize variability. Additionally, technological malfunctions or equipment failures can disrupt the control of variables, necessitating robust backup systems and regular maintenance checks. Statistical control also poses a challenge. While statistical methods can help adjust for variations in controlled variables, they are not foolproof and require careful application. Misuse or misinterpretation of statistical techniques can lead to incorrect conclusions about the relationship between variables. Furthermore, ethical considerations must be taken into account; ensuring that all participants or subjects are treated fairly and consistently is crucial for maintaining the validity of the research. Moreover, the complexity of real-world systems often means that it is impractical or impossible to control all variables perfectly. In such cases, researchers must make informed decisions about which variables to prioritize and how to manage those that cannot be fully controlled. This involves a deep understanding of the underlying mechanisms and potential interactions between variables, as well as a clear justification for the choices made. Finally, the cost and resource constraints of conducting research can limit the extent to which variables can be controlled. High-precision equipment and extensive data collection may be beyond the budget of many researchers, forcing them to find creative solutions that balance control with feasibility. Despite these challenges, meticulous planning, rigorous methodology, and transparent reporting are key to overcoming potential pitfalls and ensuring that controlled variables contribute meaningfully to the research outcomes. By acknowledging and addressing these challenges proactively, researchers can enhance the reliability and generalizability of their findings.
Best Practices for Effective Control
When implementing controlled variables in research, adhering to best practices is crucial for ensuring the integrity and reliability of the study. Effective control begins with a thorough understanding of the variables involved and their potential interactions. First, clearly define and isolate the independent variable, which is the factor being manipulated to observe its effect on the dependent variable. Simultaneously, identify and control for extraneous variables that could influence the outcome, such as environmental conditions, participant characteristics, or equipment settings. This can be achieved through randomization, where participants or samples are randomly assigned to different groups to minimize bias and ensure that each group is representative of the population. Consistency is another key aspect; maintain uniform procedures and conditions across all experimental groups. This includes using standardized protocols for data collection and ensuring that all researchers involved in the study follow the same methodology. Additionally, consider using control groups or baseline measurements to provide a reference point against which changes can be measured. These groups help in distinguishing between the effects of the independent variable and any external influences. Data quality is also paramount. Implement robust data validation and verification processes to ensure accuracy and reliability. Regularly calibrate equipment, use multiple observers or automated systems to reduce human error, and conduct pilot studies to test the feasibility and effectiveness of the experimental design. Furthermore, document every step meticulously, including any deviations from the protocol, to facilitate transparency and reproducibility. Ethical considerations must not be overlooked. Obtain informed consent from participants, ensure their privacy and safety, and adhere to ethical guidelines set by relevant authorities. This not only enhances the credibility of the research but also builds trust among stakeholders. Finally, statistical analysis should be rigorous and appropriate for the data collected. Use statistical controls such as regression analysis to account for confounding variables and ensure that conclusions are drawn based on sound statistical evidence rather than anecdotal observations. By following these best practices—clearly defining variables, maintaining consistency, ensuring data quality, adhering to ethical standards, and conducting robust statistical analyses—researchers can significantly enhance the validity and reliability of their findings when implementing controlled variables in research. This meticulous approach not only strengthens the scientific rigor of the study but also contributes to the broader body of knowledge by providing reliable and generalizable results.