What Is Ascending Order
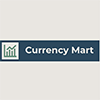
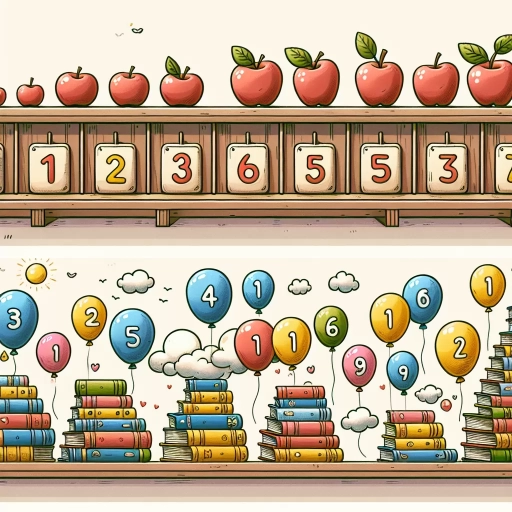
Understanding Ascending Order
Understanding the concept of ascending order is crucial in various aspects of life and professional sectors. Ascending order is an essential fundamental of mathematics and computer science, playing an indispensable role in data organization - a key factor driving the comprehensibility of our digital age. This article aims to provide a comprehensive understanding of ascending order by addressing its definition and the basic concept involved. It will also delve into practical examples of its application in everyday life. Lastly, we examine the critical role it plays in data organization in a wider context. By covering these three supporting ideas, the aim is to ensure a thorough grasp of what ascending order is, the practical aspects of its application, and the importance it holds in our modern era. Now let's venture into the roots of this concept by discussing the definition and basic construct of ascending order.
Definition and Basic Concept
An essential concept to understand in the study of ascending order is the basic definition and key concept. Fundamentally, ascending order refers to the arrangement of numbers or values from the smallest to the largest. This method of organization is extensively used in mathematics and data structures in computer science to simplify and streamline the processing or interpretation of information. Ascending order serves as a tool to better comprehend the correlation and differences among numbers, thereby facilitating efficient and accurate calculations. For example, if a data set includes the numbers 23,17,36, and 14, the ascending order of these numbers will be 14,17,23, and 36. It is of significant note to realize that ascending order is not limited to numerical values only. It can also be used for alphabets, dates, and other types of data. When applied to alphabets or words, the items are arranged from A to Z. For instance, if we have the words "apple," "grape," "banana," and "mango," arranged in ascending order, the sequence will be "apple," "banana," "grape," "mango." Meanwhile, for dates, they are arranged from the earliest to the latest. By understanding the basic definition and concept of ascending order, comprehension and application of this principle become undoubtedly easier. Whether it is for mathematical problems, computer programming tasks, or simple everyday tasks like organizing your documents, mastering the concept of ascending order equips individuals with a crucial skill that can help streamline various tasks. Knowing how to properly arrange things in ascending order is an instrumental part of data management, fostering a more organized and practical approach to analysing and interpreting information.
Examples in Everyday Life
Ascending order is pervasive in everyday life and understanding it can streamline your day-to-day activities, enhance decision making, and get you organized. To elucidate further, think about your routines. When listing your daily tasks or chores, you often start with what needs to be done first and then move on to the subsequent ones, thereby arranging them in ascending order of priority or time. Moreover, when you chronologically sort your emails or files on a computer, you're applying the principle of ascending order, with items being ranged from the earliest to the latest. Consider also financial transactions. Your bank statements, for example, are typically displayed in ascending order by date, with the earliest transactions at the top and the latest at the bottom, facilitating organized record-keeping and efficient tracking of expenses over time. Similarly, shopping becomes more manageable if you categorize your needs based on ascending order of importance, starting with essential items before luxuries, or by ascending order of price, from the least expensive to the most. Even the world of academia is replete with instances where the concept of ascending order holds relevance. In research, data collection often involves arranging collected data points from the smallest to the largest to ensure proper stratification and analysis. Furthermore, think about social scenarios. Browsing through a list of contacts in your phone or adding date entries in your personal diary, ascending order helps in keeping information systematically organized and simple to retrieve. And let's not forget sports. Marathon runners, cyclists, and race cars are ranked based on ascending order of time taken from the fastest (smallest duration) to the slowest (highest duration). The beauty of understanding ascending order lies in its versatility. It's a principle widely used in mathematics, but its application seeps into mundane tasks and activities, helping us bring structure and order to our busy lives. By recognizing its benefits and implementing the concept actively, we can optimize time management, enhance productivity, and streamline tasks, thereby leading a more organized and effective life.
Importance in Data Organization
The importance of data organization becomes prominent when understanding aspects like ascending order. A central principle that guides categorization, analysis, and interpretation of varying data, ascending order refers to the arrangement of numbers or information from the smallest to the largest. In its essence, it is the fundamental step towards effective data organization as it allows a smooth progression and a clear cut understanding of the data spectrum. Optimizing data organization through ascending order serves multiple benefits. Firstly, it creates an efficient information retrieval system, offering ease of accessibility in spotting the needed data. Secondly, the systematic arrangement aids in data comparison. With the data placed in rising order, comparing values or entries becomes straightforward and less laborious. Thirdly, it's pivotal in drawing critical insights and undertaking data analysis. When data is organized in ascending order, it’s easier to identify patterns, trends, and anomalies, thus facilitating a robust understanding of the data. Lastly, in a broader perspective, solid data organization promotes accuracy, enhancing the data's reliability and credibility. In cases where large volumes of data are involved, arranging it in ascending order can effectively manage and simplify bulky information. It eases navigation through the datasets and streamlines the process of data-handling, saving not only time but also reducing chances of error. For instance, in a corporate setting, sales records ordered from lowest to highest can help in traceability, target tracking, identification of smallest and largest sales, among others. In the modern data-driven world, progressing leaps in technology also mean an exponential rise in data. Understanding and leveraging this surge of information poses significant challenges. Here, the role of data organization strategies like ascending order becomes increasingly crucial. It's not just about stacking up numbers, but lending a structural form to the unstructured data. Thus, emphasizing reinforcing the pillars of data organization to harbor productivity, efficiency, and valuable insights. Efficient data organization, therefore, can be equated to good housekeeping. Just as items are arranged in a house for easy retrieval and aesthetic appeal, data too needs to be sorted for similar reasons. Understanding ascending order, in this context, is the stepping stone towards ensuring that your "data house" is kept in order. Such an approach can pivot faster and more accurate decision making, thereby proving to be a vital practice in the world that increasingly relies on comprehensive data interpretation.
Applications of Ascending Order
Understanding the concept of ascending order holds a significant role in multiple fields. The essence of Ascending Order not just reels in the boundaries of Math or Statistical analysis, but it can be used beneficially in computer science and real-world data handling as well. It acts as a robust tool for structuring, processing, and extracting meaningful insights from the data. Ascending order, which refers to the arrangement of numbers or other elements in a sequence from the smallest to the largest, is a universally accepted mode of data organization, instantly providing clarity and coherence. This article will explore the various applications of ascending order, focusing mainly on three key areas which are Mathematics and Statistics, Computer Science and Programming, and Real-World Data Analysis. Let's start our profound drive into the applications in Mathematics and Statistics.
In Mathematics and Statistics
In mathematics and statistics, ordering data can significantly impact data analysis, giving order and structure to chaos. This ensures systematic data interpretation, leading to more accurate and precise results. In this light, one application of an ascending order that stands out is its role in data analysis and finding quantitative data measures. Ascending order's concept, which is arranging numbers or data points from the smallest to the highest, plays a profound role in calculating measures like median, mode, and percentile ranks. In statistic analysis, the data must be in ascending order to accurately calculate the median, the value that lies at the center of the data set. If the numbers are not arranged in ascending order, randomly scattered data would result in an miscalculated median value, ultimately affecting the data analysis. The proper alignment of data in ascending order reduces complications to the process, making it simpler and more manageable. Moreover, finding the mode, or the most frequently occurring value in a dataset, becomes analytically straightforward when data is arranged in ascending order. In case the dataset is large, arranging it in ascending order will facilitate quickly scanning through the data for repetitive values, saving time and efforts and also minimising the chances of errors. In addition, percentiles are also calculated more accurately when the data is in ascending order. In statistical reports, percentiles help measure the relative standing of a data value within a data set. For instance, the 25th percentile signifies that 25% of the data values in the data set are below that particular value. Thus, the arrangement of the data in ascending order precedes the percentile calculation. Lastly, plotting graphs and identifying trends becomes easier with data placed in ascending order. In charts like a box plot or a stem and leaf plot, data needs to be arranged in ascending order so that the distribution pattern of the data set becomes discernible. This way, outliers and trends can be easily detected, thereby assisting in drawing accurate conclusions. In conclusion, arranging data in ascending order is an integral part of statistics and mathematics, with extensive applications that facilitate the comprehension and interpretation of data. The ability to efficiently and effectively manipulate numerical data in ascending order is determinative of the accuracy and reliability of any statistical analysis.
In Computer Science and Programming
In Computer Science and Programming, the concept of ascending order finds numerous critical applications that augment the efficiency of the system. Optimizing operations, data structural behavior, and several algorithms employ this concept, thereby enhancing overall performance. Ascending order is crucial as it organizes data in a fundamental way rising from the lowest or smallest value to the highest or greatest value. Many sorting algorithms, such as bubble sort and merge sort, implement an ascending order strategy to sort the data, enabling faster searching and data manipulation. Database systems, which are the backbone of multiple organizations, use index structures like B-trees that store data in ascending order to ensure quick data retrieval and latency reduction. For instance, when a user queries a large product database for a specific product name, the system searches in an alphabetically sorted (ascending order) dataframe, consequently leading to expedited results and better customer satisfaction. In the world of programming, even simple programming constructs, like 'for' loops, often utilize the concept of ascending order. The loop typically starts with the smallest index and ascends to the final index, facilitating easier iteration through the data. Similarly, when we explore big data technologies, data is sorted in ascending order at numerous stages for better computational efficiency. Ascending order also plays a significant role in data visualization efforts as it allows to efficiently represent and understand data points on charts and graphs. Moreover, when applying certain mathematical algorithms, results must be in ascending order for the correct application of further operations or problem-solving methods. Binary search, widely used in numerous computing scenarios, also necessitates the data to be in ascending order to work effectively and provide accurate results. Furthermore, when programming applications perform operations that involve time-series data, the data is typically sorted in ascending order chronologically. This order makes for an easier comprehension of past patterns and prediction of future trends. Notably, financial and stock market analysis, climate modeling applications, and historical data studies all demand data arranged in ascending order. Thus, it is evident that ascending order finds far-reaching applications in Computer Science and Programming, directly contributing to the system's performance and efficiency. A simple concept yet so profound, it plays a substantial role in the information age, managing and simplifying numerous complexities.
In Real-World Data Analysis
The application of ascending order is palpably perceptible within the riveting realm of real-world data analysis. This order, largely characterized by the arrangement of numbers or data from smallest to largest, plays a pivotal role in the endeavor to assemble, interpret, and communicate vast volumes of information. Crucially, it aids in turning raw data into usable information that can be applied to support decision-making processes, strategy formulation, and real-time actions in a wide variety of sectors. In the sphere of data analysis, understanding the order of the data is of paramount importance, lending clarity and coherence to the patterns and trends that might otherwise remain obscured. For instance, in financial data analysis, the use of ascending order is integral and even ubiquitous. Analysts, when dealing with vast finances, might resort to ranking data in ascending order to identify the least and most performing sectors, fluctuations in quarterly sales, or perhaps the evolution of stock market prices. Visualizing this ordered data in such a context could efficiently enable investors to monitor financial trends and accordingly adapt their investment strategies, thereby maximizing potential returns and robustly mitigating associated risks. Similarly, in environmental data analysis, pertinent variables such as temperature, levels of pollution, or species population can be arranged in ascending order to identify patterns over time or across various locations. Such an arrangement not only facilitates the clear discernibility of data, but it also provides the opportunity for accurate predictions or forecasts about future trends. Understanding the implications of such trends is crucial to devising sustainable environmental strategies and policies. Moreover, the medical field also derives significant benefit from the application of ascending order in data analysis. By arranging patient information, such as age, weight, or disease severity, in ascending order, healthcare providers can optimize their patient care strategies. This could contribute to enhancing the efficiency of medical treatments, ensuring fair prioritization of patients, and furthering medical research. Furthermore, ascending order finds its utility in educational data analysis as well. When scores of students are arranged in ascending order, it can significantly expedite the task of identifying trends, uncovering the best performing students, or discerning areas of improvement. From curriculum planning to strategic intervention formulation, educators can leverage such ordered data to ensure the efficacy of teaching methods and the overall improvement of student performance. In conclusion, the applications of ascending order in the real-world data analysis are manifold, pulsating through myriad sectors of society. It integrates succinctly into the fabric of distinct disciplines, not only enriching the analytical processes but also refining the decision making for the betterment of various industries, the environment, healthcare systems, and educational institutes. The power vested in this humble concept of ascending order, thus, extends far beyond mere numerical organization, permeating deep into our understanding and management of the world around us.
Practical Steps to Arrange Data in Ascending Order
Organizing data in ascending order can be an important task, whether for academic, professional, or personal endeavors. In pursuing this objective, it's essential to follow a systematic approach to ensure the accuracy and ease of the process. This article presents practical steps that can assist you in arranging data in ascending order, diving into three vital segments: Manual Sorting Techniques, Using Software Tools and Algorithms, and Common Mistakes to Avoid. Initially, we tackle the manual sorting techniques where the traditional methods of sorting by hand are shown to be an effective but labor-intensive way of getting the job done. This is particularly suitable for smaller data sets. Transitioning into the more sophisticated, we later discuss how software tools and algorithms can make this process faster and more efficient, even when dealing with large volumes of data. Lastly, we highlight common pitfalls in this activity and how to avoid them to secure accuracy in your data arrangement. Let us now delve into Manual Sorting Techniques and explore its benefits and limitations.
Manual Sorting Techniques
Manual sorting techniques serve as the bedrock foundation for arranging data in ascending order. These methodologies, which date back to early computational practices, have become incredibly useful for data organization both within and outside the digital landscape. Manual sorting can encompass a variety of strategies, but for the sake of managing data sequentially, two primary techniques prove particularly effective: bubble sort and insertion sort. The bubble sorting technique, named due to the manner in which smaller value 'bubbles' towards the top, is one of the most informal and straightforward ways to organize items in ascending order. The process begins at the list's start and compares each pair of adjacent items, swapping their positions if they are in the incorrect sequence. This continues until the entire list is sorted in a preferred order. While bubble sort can be time-consuming, particularly when working with larger amounts of data, its straightforwardness and simplicity make it a desirable option for small-scale manual sorting tasks. On a more efficient end of the spectrum, there's insertion sort. This technique involves partitioning the data into a sorted and unsorted region. The method starts with the first element in the list, considering it as a sorted segment. It then iterates through the unsorted portion, comparing each element with the sorted partition and inserting it into the correct position. What makes it beneficial is its low overhead, and it performs particularly well when working with smaller and partially sorted lists. These two manual sorting techniques provide a robust starting point for managing data, whether dealing with numerical, alphabetical, or any other type of measurable data. They work off practical logic, giving a fundamental understanding of how the sorting process operates. Specifically, bubble sort provides a basic introduction to data comparison and swapping, while insertion sort offers a more expedient strategy for sorting smaller data lists. Utilizing these techniques in tandem can yield better data organization and enhanced understanding of manual sorting processes. Thus, taking the time to understand and implement manual sorting methods forms an integral part of any process that aims at arranging data in ascending order.
Using Software Tools and Algorithms
In the pursuit of arranging data in ascending order, employing software tools and algorithms have been proven incredibly valuable. With the advancement of technology, humans have developed and utilized a variety of tools and algorithms that simplify complex problems and tasks, primarily in the field of information technology. Data organization has become less strenuous thanks to the presence of multiple software tools and efficient algorithms. These resources facilitate the process, making it feasible to implement within a few steps, and ensure that it is performed accurately without any errors. Software tools range from simple spreadsheet programs like Microsoft Excel to more complex database management systems like SQL Server, MySQL, or Oracle. These tools have inherent features that allow data sorting in ascending and descending order, eliminating the need for manual sorting, which can be lengthy and error-prone. For instance, the 'Sort & Filter' function in Excel provides an accessible means to arrange data promptly. Furthermore, various algorithms aid in sorting data in an organized manner. Iterative algorithms, such as Bubble Sort, Selection Sort, and Insertion Sort, and divide-and-conquer algorithms like Quick Sort and Merge Sort, are prime examples. These algorithms have different time complexities; some operate well with a small amount of data, while others are designed to handle large datasets effectively. Interestingly, programming languages such as Python, Java, and JavaScript have in-built functions that allow sorting of data components swiftly. Python, for instance, has a sort() function that organizes data elements from lowest to highest as a default, thus aiding in achieving ascending order swiftly and efficiently. When dealing with enormous volumes of unorganized data, using these software tools and algorithms becomes all the more crucial. They form the backbone of data analysis, enhancing the efficiency of data sorting and paving the way for deeper insights. By leveraging these tools and algorithms, businesses and individuals can optimize their data organization, making it more streamlined, accurate, and insightful for further conclusions and projections. In conclusion, software tools and algorithms play an instrumental role in arranging data in ascending order. With their ability to handle a wide spectrum of data, from small volumes to large data sets, these tools have made data arrangement simpler, faster, and more precise. Therefore, understanding the functionality and application of these tools is an excellent practical approach towards achieving efficient data sorting.
Common Mistakes to Avoid
Ensuring that data is arrayed in ascending order contributes significantly to seamless data management and interpretation. However, common mistakes often undermine the essence of this simplistic yet impactful practice. One prevalent blunder is assuming that all data types can be arrayed in ascending order using the same approach. On the contrary, the methodologies differ among strings, numerical data, and dates. Another pitfall to avoid involves neglecting the null or missing values. Oftentimes, these null values are overlooked during arrangements, leading to inaccurate results. Equally, wrongly assuming that the program or the software being used automatically arranges data in ascending order is a common mistake that results in incorrect data interpretation. Overreliance on visual checks is another error that individuals dealing with data in ascending order typically fall into. Visual checks, though beneficial, cannot be trusted fully, especially when dealing with an enormous amount of data. This prompts the need to embrace formula checks or even software to alleviate possible human errors of oversight. Inaccuracy in data input is another issue; minor mistakes like typing the wrong figure or inverting numbers may lead to subsequent errors in the entire data arrangement process. Also, failure to routinely update and cross-check information can lead to the exploitation of outdated information, hence incorrect results. It is worth noting that arranging data in ascending order goes hand in hand with polished recording techniques. If data is entered inconsistently, like varying date formats, the process of arranging data becomes harder and less accurate. The last but not least mistake to evade is the disregard for double-checking your Arranged data. This is what makes the difference between a meticulous data analyst and a careless one. The significance of avoiding these errors cannot be underscored enough. If these mishaps sneak into your data arrangement practice, they compromise the entire data analysis process. Therefore, their avoidance ensures accurate, streamlined, and dependable data, which in turn ensures valid decisions and conclusions drawn from the data. By steering clear of these pitfalls, you glean more accurate insights from your data, contributing to more informed decision-making processes and strategies, an indispensable element in today's data-driven world. The cardinal rule is to always stay vigilant and meticulous while dealing with ascending order data. This way, you sidestep these common traps, and remain a step ahead in your data analysis journey.