What Is N In Statistics
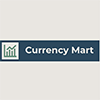
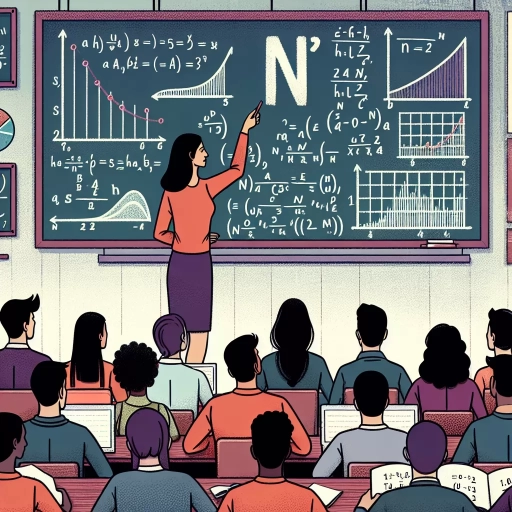
Understanding the Concept of n in Statistics
Understanding the concept of 'n' in statistics is a fundamental step in decoding the intricacies of statistical calculations and data analysis. This article will guide you to understand the definition and basic principles of 'n' in statistics, explore the nuances between the two types of 'n': sample size and population size, and elaborate the key importance of 'n' in statistical analysis. In the realm of statistics, 'n' plays a pivotal role. It is a cornerstone in measuring, analyzing, and predicting patterns from data, making it a crucial element no matter what field - research, business, or even social sciences. Statistics, as a discipline, single handedly rely on the effective interpretation of 'n'. As we delve deeper into this article, we aim to demystify it. First, we would shed light on the definition and underlying principles governing 'n' in statistics, that firmly establish the foundation of this concept.
Definition and Basic Principles
In understanding the concept of 'n' in statistics, one must drill down to the fundamentals of the principle, its definition, and its basic operations. The term 'n' is commonly used in statistics to denote a 'sample size' – the selection from a larger population that you analyze to draw inferences about the broader group. A crucial aspect of statistical analysis, the sample size has a significant impact on the reliability of your results and varies depending on the context and objectives of your study. The ability to determine an appropriate sample size, therefore, lies at the heart of effective statistical work. Ideally, you want 'n' to be large enough to provide a representative snapshot of the population but small enough to be manageable and cost-effective. More importantly, 'n' needs to be adequate such that the power of the statistical test you are using remains intact. In circumstances where 'n' is too small, statistical tests would not have enough power to detect a significant effect, even if it exists in reality. One of the fundamental principles associated with 'n' in statistical studies is the Law of Large Numbers. This principle posits that as 'n' increases, the sample increasingly represents the underlying population, providing more accurate estimates of the population parameters. Essentially, the larger the 'n', the closer the sample mean gets to the population mean. This is why extensive surveys spanning extensive geographic and demographic scopes, like the U.S. Census or large political polls, are crucial for drawing generalized conclusions about a population. Another critical principle related to 'n' is the Central Limit Theorem. This theorem asserts that when an infinite number of successive random samples are taken from a population, the sampling distribution of their means will approach a normal distribution, regardless of the shape of the population distribution. This principle often justifies the use of parametric tests in statistical analysis and delineates the conditions under which these tests are likely to be valid. That said, the manipulation of 'n' should always consider the ethical implications and avoid bias. Overfitting, for instance, occurs when 'n' is too small, and the statistician uses the same data for both hypothesis generation and testing, which might result in false positives. Conversely, the overuse of large 'n' sizes can amplify minute and irrelevant differences to statistical significance, risking misleading or overblown claims. Thus, understanding 'n' in statistics is central to ensuring the validity and reliability of your research, and ultimately, the decisions relying on your findings. In a world increasingly dependent on data, mastering these principles has never been more critical.
Types of n: Sample Size and Population Size
Understanding the concept of 'n' in statistics requires a deep dive into two significant aspects; sample size and population size. Both are vital terms that influence the outcomes of a statistical analysis. The 'n' could signify 'N' or 'n,' representing the population size and the sample size, respectively. The population size (N) denotes the total number of individuals or units within your population of interest - essentially the total number you're studying. For instance, if you wish to study the average age of all employees in a firm with 1000 employees, your population size (N) will be 1000. On the other hand, the sample size (n) signifies a smaller group, a subset selected from the population size. A sample should effectively represent the population for your study to have a high degree of accuracy. Let's utilize our initial example. If you randomly select 100 employees out of 1000 to determine the average age, your sample size (n) will be 100. The importance of appropriately selecting 'n' whether sample or population size, cannot be overstated. It not only affects the precision and accuracy in your statistical outcomes but also determines the type of statistical tests you would employ within your study. Moreover, when handling substantial populations, selecting an appropriately large sample size is crucial to obtaining outcomes that adequately represent the whole. If your 'n' is too small, you risk getting skewed results that do not mirror the whole population satisfactorily. Conversely, if 'n' is taken as the whole population, it could lead to unnecessary complexities and resource expenses. It's also crucial to remember that sample size (n) and population size (N) play a crucial role in determining the margin of error and standard deviation in a statistical study. The larger the sample size, the smaller the standard deviation and margin of error, which means your results are likely to be more reliable. Therefore, an in-depth understanding of these types of 'n' empowers statistical analysts to make well-informed decisions about their sample and population sizes and consequently harbor accurate, reliable data analysis. Understanding the principles of 'n' in population and sample size aids in succinctly interpreting, representing, and generalizing data findings to the comprehensive population. Ultimately, it provides a strong foundation for decision making using statistical data.
Importance in Statistical Analysis
is an integral part of statistical analysis, offering in-depth insights into data sets and enabling effective decision-making. Accurate statistical analysis hinges upon understanding the concept of 'n' in statistics, as this represents the sample size of a given study or experiment. The significance of 'n' lies in its direct correlation to the reliability and validity of a statistical analysis. The larger the sample size, the more accurate and reliable the results and conclusions drawn from the analysis are likely to be. This is because larger samples can better counteract the potential influence of outliers or random errors, providing a more accurate representation of the population. However, it isn't as simple as just increasing the sample size. There should be a balance because too large a sample can also introduce error due to the complexities and logistical difficulties in handling a vast amount of data. Statistical analysis also has a critical role in hypothesis testing, where 'n' is necessary to calculate the significance of results. The precision of estimation improves as the value of 'n' increases, bolstering the strength of the evidence against the null hypothesis. Furthermore, understanding 'n' is crucial in statistical modeling, especially in regression analysis. Here, 'n' helps determine the number of predictor variables that can be included without overfitting the model. Moreover, statistical analysis, guided by the appropriate use of 'n', is essential for making sense of complex data structures in diverse fields. For instance, in the medical field, statistical analysis is fundamental in determining the efficacy of new treatments or drugs, where 'n' represents the number of subjects used in clinical trials. In business, companies utilize statistical analysis to understand consumer behaviour, market trends, or to evaluate operational efficiencies, where 'n' can denote the number of customers, transactions, or processes being analyzed. In conclusion, the importance of statistical analysis cannot be overstated, with every detail, even the ones that seem relatively small, like the concept of 'n', playing a vital role. Understanding and employing 'n' correctly guarantees more reliable and precise results, leading researchers and analysts right up to the doorstep of the truth they are seeking. It showcases the need to not only recognize the figures and calculations presented but also understanding the underlying principles and elements that make up these results.
Applications of n in Statistical Methods
the pervasiveness of data in the digital era has necessitated a deeper understanding of statistical methods and the integral role that sample size, often denoted by 'n', plays in these procedures. Diverse statistical applications are hinged upon the sample size to ensure accurate, reliable, and valid results. Particularly, 'n' has a significant role in hypothesis testing, impacts the construction of confidence intervals, and is indispensable in regression analysis. In hypothesis testing, 'n' influences the power of the test, dictating the probability of a correct reject or accept decision. In constructing confidence intervals, 'n' affects the precision and stability of the interval. Moreover, in regression analysis, 'n' impacts the robustness and the predictive capability of the model. Thus, understanding 'n' in statistical methods is a foundation we can't ignore. Our first area of focus is the role 'n' plays in hypothesis testing.
Role in Hypothesis Testing
In the realm of statistics, 'n' has a highly significant role, particularly in hypothesis testing. Hypothesis testing, a critical procedure in statistics, is employed to infer the outcome of a hypothesis performed on sample data from a larger population. The sample size 'n' in hypothesis testing is of utmost importance as it can significantly affect the test outcomes and the power of the test. The power of the test in statistical hypothesis testing is the probability that the test will reject the null hypothesis when the alternative hypothesis is true. The more substantial the 'n' or the sample size, the more power a hypothesis test has, meaning it is more probable to detect a significant effect if one is present. Therefore, increasing the sample size can lead to more accurate results. Hypothesis testing involves the estimation of various parameters such as mean, standard deviation, and variance based on the sample data. An appropriate 'n' value helps in achieving these estimates with greater accuracy and credibility. Determining an ideal sample size is not merely about increasing it to attain accuracy but also considering the feasibility, time, and cost involved. 'n' or the sample size in this context plays a key role in the trade-off between precision and resources. If the 'n' value is too small, it could lead to a statistical error - either presenting an effect when there isn't one (false positive) or failing to detect an effect when there is one (false negative). On the other hand, an unnecessarily large 'n' might be a waste of resources. Therefore, finding the right 'n' for hypothesis testing is essential to prevent errors and misinterpretation. It helps to achieve a balance between accuracy, resource allocation, and feasibility of conducting the study. It shapes the reliability of the test, and subsequently, the validity of the result. 'n,' thus symbolizing sample size, is a fundamental element in hypothesis testing and various applications of statistical methods. It makes its significance known not just in the theory but in the actual practice of statistics. Consideration of 'n' is pivotal to any statistical study or data analysis, underlining its necessity in this field of study.
Impact on Confidence Intervals
Confidence Intervals (CIs), a major application of n in Statistical Methods, are hugely impacted by the sample size, represented by 'n'. Understanding the impact of n on confidence intervals is crucial to the analysis of statistical data as it directly influences the quality and credibility of the study's results. A confidence interval is a range wherein, calculated through a specific statistical method, the true population parameter is likely to reside. It provides an estimate of the margin of error associated with a sample statistic. A compelling characteristic of confidence intervals is how the size of the sample impacts its width. A larger 'n' often results in a narrower confidence interval, rendering the estimate more precise. This precision is due to the ability of larger sample sizes to better represent the entire population, thus reducing potential bias and eliminating extreme values. It's virtually augmenting the accuracy of the experiment or survey by reducing the range where the true parameter might lie. The Central Limit Theorem accentuates this notion, elucidating that as the sample size increases, the distribution of the sample means approaches a normal distribution. This promotes a better estimation of the population mean, further enhancing the credibility and accuracy of the research. In contrast, a smaller 'n' can lead to a wider confidence interval, increasing the pool of values the population parameter may fall into and diminishing the overall precision. It offers less certainty about the accuracy of the parameter estimate due to potential influence of outliers and unrepresented subgroups in the population. Consequently, smaller sample sizes result in larger standard errors, expanding the confidence interval and reducing its reliability. To perceive the impact of 'n' on confidence intervals, consider a scenario with a hospital testing a new treatment regime. With a sizeable sample of patients, the results generated would likely be deemed reliable, and the treatment can be confidently assumed to work with the estimated effectiveness across the broader population. Suppose the same study was conducted, but with noticeably fewer patients; the observed effectiveness would be clouded in greater uncertainty, reflected in a broader confidence interval. The hospital cannot be as confident about the treatment's effectiveness, thus, posing a considerable risk. Essentially, having a larger 'n' in studies is not merely about achieving significance; it enhances the robustness of the study and credibility of the results by painting a more accurate picture of the parameters they represent. Therefore, a meticulous approach in determining the optimal sample size in studies is fundamental to ensure that the confidence intervals generated are reliable and meaningful, ultimately empowering better decision-making.
Use in Regression Analysis
Regression analysis is a robust statistical model widely utilized in various fields to predict, explain, or establish the relationship between a dependent variable and one or more independent variables. In the context of regression analysis, 'n' often denotes the total number of observations or dataset size. The 'n' provides key insights regarding the reliability and viability of the regression analysis. An adequate 'n' size affirms that the obtained results are not by mere chance, bringing about statistical power and reducing type II errors. Furthermore, a larger 'n' increases the precision of the estimated regression coefficient, ensuring the model's accuracy. In building regression models, the selection of an appropriate 'n' size becomes greatly significant. An inadequate sample size may lead to skewed results, causing misinterpretation of data. The central limit theorem infers that as 'n' approaches infinity, the distribution of the data tends to approximate a normal distribution, irrespective of the original distribution shape. Therefore, to reasonably apply regression analysis, a larger 'n' size is more desirable, fostering accurate predictions. However, the number 'n' is not an arbitrary value. A calculated approach in selecting its size gives room for handling the trade-off between bias and variance, an integral aspect of model performance. A sample size that is too large can lead to overfitting, a statistical issue wherein the model becomes too flexible and incorporates random error present in the data. On the other hand, a smaller sample size might suffer from underfitting issues, oversimplifying the model, and failing to capture relevant patterns. Therefore, the utilization of 'n' in regression analysis is not a straightforward process. It involves careful deliberation and intuitive understanding of the data. Through rigorous testing and validation, assessing outliers, conducting bootstrap methods, and acknowledging possible confounding variables, one can significantly enhance the effectiveness of the regression model. In essence, the 'n' in regression analysis encapsulates myriad considerations, converging towards the pursuit of sound statistical reasoning and data interpretation.
Practical Considerations and Limitations of n
about choosing the right sample size in research is a common dilemma. Though 'n' might seem like just a number, it plays a crucial role in deriving conclusions with precision and power. This article provides practical considerations and highlights some limitations while working with 'n'. Three key areas are explored: Determining the optimal sample size, dealing with small sample sizes and contending with ethical and resource constraints. Often, researchers are faced with the question of how large a sample should be. The subsequent paragraph on determining optimal sample size provides insights on how to decide upon the right 'n', ensuring statistical significance without publicizing inaccurate or misleading results. However, sometimes due to various constraints, it's not feasible to have the 'ideal' sample size. The section on managing small sample sizes is aimed at researchers in this predicament - offering methodological solutions to combat this issue. Finally, one cannot overlook ethical and resource constraints which pose significant challenges to the 'n' in a study. The following paragraphs delve deeper into these considerations, providing a comprehensive guide to address these challenges when conducting research. First, let's explore the complex subject of determining an optimal sample size.
Determining Optimal Sample Size
When determining the optimal sample size in statistical studies, several considerations and limitations factor in. These practical aspects revolve around the pivotal "n" variable, representing the size of the sample group. The "n" adopted in any given research should be informed by the intrinsic characteristics of the subject population, purposed to yield as accurate results as possible. Firstly, a sample group must be sufficiently sizable to facilitate the statistical power needed in observing an effect should one exist. For instance, if the effect size is small, a larger n would be required for the effect to be observed. On the flip side, if the effect size is large, a smaller sample group could suffice. Therefore, one must carefully estimate the effect size as it is crucial in determining a suitable n. Secondly, the diversity within the group population should be considered. If the population under study is highly diverse with a broad range of characteristics, a larger sample size would be necessary to ensure representative inclusion of all elements. Conversely, a smaller, more homogeneous population could suffice with a smaller n. Another important criterion is the type of statistic being used. For instance, studies using averages often require smaller sample sizes than studies relying on extreme scores. Since extreme scores are less frequent, a larger sample size is needed to capture a sufficient number of these. However, statistical theory is not the only determinant of sample size. Practical considerations such as time, cost, and available resources also deeply influence the n-figure. For example, in a situation where obtaining a large number of respondents might prove financially draining or logistically challenging, a compromise may be struck between statistical theory and practical constraints. Furthermore, there are limitations to consider. For one thing, increasing sample size yields diminishing returns once a certain threshold is met. If "n" is too large, it can result in a waste of resources, and any additional data collected may not significantly enhance the precision of the results. On the other hand, if "n" is too small, there might be a risk of statistical errors, which could lead to inaccurate conclusions being drawn from the study. The science of determining optimal sample size is indubitably an intricate one that demands a fine balance between statistical fidelity and practical feasibility. It weighs philosophical, economic, and statistical aspects to equal measure. Pertinently, while larger samples usually provide more accurate results, the considerations highlighted underscore that the optimal sample size is not necessarily the largest one. Instead, it's one conformed to the unique particulars of each study, ensuring the most accurate, efficient, and manageable outfit for realizing meaningful, useful findings.
Dealing with Small Sample Sizes
Dealing with small sample sizes, also known as 'n' in statistics, can pose specific challenges in any type of research. Statisticians must be particularly careful when working with smaller sample sizes due to potential issues related to statistical power, variance, and reliability. However, there are practical considerations and limitations to bear in mind when working with such limited data sets. One of the most critical considerations when dealing with small sample sizes is the issue of statistical power. Simply put, statistical power refers to the likelihood that a study will detect an effect when there is an effect there to be detected. In studies with smaller sample sizes, it is all too possible to fail to detect a significant effect due to the limited statistical power. This could lead to incorrect conclusions or even the disregarding of valuable insights. Another crucial aspect to mull over is variance. Smaller sample sizes tend to have more variance as compared to larger sample sizes. It implies that, when limited data points are available, the data tends to vary widely, which might lead to the generation of inaccurate conclusions. This elevated level of variance is largely due to random noise present within the data, which becomes more pronounced and influential when fewer data points are available. The reliability of the data can also be a pressing concern when dealing with small sample sizes. The lesser the sample size, the greater the chance that the sample may not be representative of the wider population. Consequently, any conclusions or insights gleaned may not be broadly applicable and could potentially misrepresent the overall population, leading to skewed results. Furthermore, one cardinal limitation associated with small sample sizes is the inability to conduct more complex statistical analyses. Given that advanced analytical techniques often require large amounts of data to generate robust findings, the lack of sufficient data inherent with small sample sizes may rule out these methodologies. In these cases, researchers may have to resort to using simpler, less nuanced analytical techniques, which might not be capable of unearthing more profound insights. Despite these challenges and limitations, it essential to note that smaller sample sizes do not invalidate a study or its results. On the contrary, they simply necessitate extra caution, thorough planning, strategic analytical approaches, and, most importantly, an acknowledgment of the limitations imbued. Moreover, small samples can be beneficial in preliminary research stages, where the primary goal is to explore a phenomena or generate hypotheses that can be tested on a larger scale in the future. Thus, while dealing with a small 'n' in statistics, one must be alert about these considerations and consider the appropriate balance between sample size, statistical power, and the objectives of the study.
Ethical and Resource Constraints
While exploring "n" in statistical studies, we encounter several practical considerations and limitations that shape the execution and interpretation of results. Among these, ethical and resource constraints pose significant challenges. Ethical constraints primarily involve ensuring the security and confidentiality of data, upholding consent, and avoiding harm to participants during or after the research process. This is often complex in large-scale statistical studies where personal data are involved. Strict penalties for ethical violations necessitate stringent data coding, encryption, and usage procedures that could potentially limit exploratory data analysis or the use of certain sophisticated statistical models. On the other hand, resource constraints affect an array of operational aspects from sampling methods to data analysis. The resources in question could be time, skilled personnel, availability of appropriate research tools or even finance to sponsor the project. These constraints could significantly reduce the scale of study ("n") leading to smaller sample sizes, stratified sampling methodologies, and simpler analytical models affecting the study’s validity and reliability. For example, inadequate resources may limit a study's ability to survey a large and diverse population, potentially leading to sample bias, where the sample inadequately represents the whole population. This biased sampling constrains the capability to confidently assert any derived conclusions to the broader population, thus reducing the external validity of the study. Moreover, resource constraints can affect the study's data analysis. The use of advanced statistical models and technologies require time, expert input, and financial investment, which if lacking, may limit the kind and complexity of statistical analysis that the study can undertake. Hence, there can be a compromise on the insights drawn and their applicability, leading to a gap between practical implications and research findings that could be otherwise if expansive resources were available. Furthermore, in some cases, ethical and resource constraints may intersect. For instance, financial barriers may hinder the implementation of cutting-edge data protection measures, leading to potential ethical concerns. Similarly, to conform to ethical standards by adequately anonymizing data, valuable time resources may be consumed, restraining other aspects of the project. Therefore, while understanding 'n' in statistics, it's pivotal to consider the practical limitations of ethical and resource constraints. These not only affect the study's execution but also significantly impact its results, validity, and reliability. As researchers, understanding and mitigating these constraints could dramatically change the payoff of the statistical study.