What Is Cause And Effect
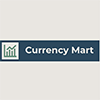
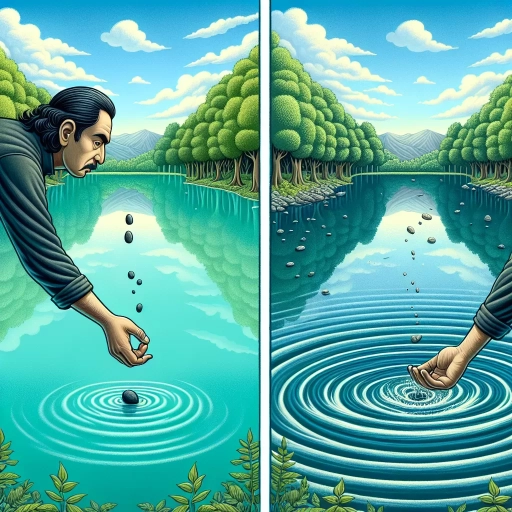
Understanding the intricate relationships between cause and effect is a fundamental aspect of critical thinking and problem-solving. This concept is ubiquitous, influencing various aspects of our lives, from scientific research to everyday decision-making. In this article, we will delve into the core principles of cause and effect, exploring how these relationships are identified and analyzed. We will begin by **Understanding the Concept of Cause and Effect**, laying the groundwork for a deeper comprehension of this foundational idea. Next, we will **Identify Causes and Effects in Various Contexts**, examining how these relationships manifest in different fields such as science, history, and personal experiences. Finally, we will **Analyze and Interpret Cause and Effect Relationships**, providing tools and strategies for evaluating the strength and significance of these connections. By grasping these concepts, readers will gain a clearer understanding of how causes lead to effects, enabling them to make more informed decisions and navigate complex situations more effectively. Let's start by **Understanding the Concept of Cause and Effect**.
Understanding the Concept of Cause and Effect
Understanding the concept of cause and effect is a fundamental aspect of human reasoning and problem-solving. This concept is pivotal in various fields, from science and philosophy to everyday decision-making. To grasp the full scope of cause and effect, it is essential to delve into three key areas: the definition and basic principles, the historical development of the concept, and its real-world applications. Starting with the **Definition and Basic Principles**, we explore how cause and effect are defined and the underlying mechanisms that govern their relationship. This foundational knowledge sets the stage for a deeper understanding of how causes lead to specific effects. The **Historical Development of the Concept** reveals how different cultures and thinkers have contributed to our current understanding, highlighting the evolution of thought over time. Finally, **Real-World Applications** demonstrate how cause and effect are applied in various contexts, such as scientific research, policy-making, and personal decision-making. By examining these three facets, we can gain a comprehensive understanding of this critical concept. Let us begin by examining the **Definition and Basic Principles** that form the bedrock of our understanding of cause and effect.
Definition and Basic Principles
**Definition and Basic Principles** Understanding the concept of cause and effect begins with a clear definition and an exploration of its fundamental principles. Cause and effect, also known as causality, is a relationship between events where one event, the cause, leads to another event, the effect. This relationship is foundational in various fields such as science, philosophy, and everyday life. The basic principle underlying cause and effect is that every effect has a preceding cause; this is often summarized by the Latin phrase "causa sine qua non," meaning "a cause without which the effect would not have occurred." In scientific inquiry, cause and effect are determined through empirical evidence and rigorous testing. For instance, in physics, the law of gravity states that objects attract each other with a force proportional to their mass and the distance between them. Here, the gravitational force is the cause, and the resulting motion or attraction is the effect. Similarly, in medicine, understanding the cause (e.g., a virus) and effect (e.g., symptoms of a disease) is crucial for diagnosis and treatment. Philosophically, the concept of cause and effect raises questions about determinism versus free will. Determinists argue that every event, including human decisions, is the inevitable result of prior causes and is therefore predetermined. On the other hand, proponents of free will argue that individuals have the ability to make choices that are not entirely determined by prior causes. In everyday life, recognizing cause and effect helps individuals make informed decisions and predict outcomes. For example, if a person notices that eating certain foods leads to digestive issues (the effect), they can avoid those foods (the cause) to prevent discomfort. This practical application underscores the importance of understanding causality in personal health, financial management, and social interactions. The principles of cause and effect also involve understanding potential confounding variables and the distinction between correlation and causation. Correlation does not imply causation; just because two events occur together does not mean one causes the other. For instance, the observation that ice cream sales increase during summer months does not mean that eating ice cream causes warmer weather. Instead, both phenomena are likely caused by a third factor—seasonal changes. In conclusion, grasping the definition and basic principles of cause and effect is essential for navigating various aspects of life. It enables us to identify relationships between events, make predictions, and take informed actions. By recognizing that every effect has a preceding cause, we can better understand the world around us and make more effective decisions. This foundational concept is a cornerstone of critical thinking and problem-solving across disciplines.
Historical Development of the Concept
The concept of cause and effect has a rich historical development that spans across various disciplines and cultures. In ancient Greece, philosophers such as Aristotle and Plato explored the idea of causality, with Aristotle's "Four Causes" (material, formal, efficient, and final) being particularly influential. This framework laid the groundwork for understanding how events and phenomena are interconnected. In the Middle Ages, the concept evolved through the works of philosophers like Thomas Aquinas, who integrated Aristotelian ideas with Christian theology to explain the causal relationships between God, the universe, and human actions. The Renaissance saw a resurgence in scientific inquiry, with figures like Galileo Galilei and Isaac Newton contributing to a more empirical understanding of cause and effect through their work in physics. The Enlightenment period further solidified the concept with thinkers like David Hume, who introduced the idea of causation as a matter of habit and observation rather than inherent necessity. This philosophical shift paved the way for modern scientific methodologies that rely heavily on empirical evidence to establish causal relationships. In the 19th and 20th centuries, the concept of cause and effect became more nuanced with advancements in fields such as psychology, sociology, and economics. For instance, Sigmund Freud's psychoanalytic theory explored how past experiences (causes) influence present behaviors (effects), while sociologists like Émile Durkheim examined how social structures and norms shape individual actions. The development of statistical analysis and experimental design in the 20th century allowed for more precise measurement and testing of causal relationships. This was particularly evident in fields like medicine, where randomized controlled trials became the gold standard for determining the efficacy of treatments. Today, the concept of cause and effect continues to evolve with advancements in technology and data analysis. Big data analytics and machine learning algorithms enable researchers to identify complex patterns and potential causal links within vast datasets. Additionally, interdisciplinary approaches combining insights from psychology, neuroscience, economics, and other fields provide a more holistic understanding of how causes lead to effects across various domains. Understanding this historical development is crucial because it highlights how our comprehension of cause and effect has become increasingly sophisticated over time. By recognizing the contributions of past thinkers and the evolution of methodologies, we can better appreciate the complexity and depth of causal relationships in our contemporary world. This historical context also underscores the importance of ongoing research and critical thinking in refining our understanding of cause and effect, ultimately leading to more accurate predictions and interventions in various fields.
Real-World Applications
Understanding the concept of cause and effect is crucial for navigating various real-world applications across multiple disciplines. In **medicine**, cause and effect relationships are essential for diagnosing diseases and developing treatments. For instance, identifying the cause of a patient's symptoms allows healthcare professionals to prescribe the most effective treatment, thereby improving patient outcomes. Similarly, in **public health**, understanding the causes of epidemics helps in implementing preventive measures and developing vaccines to mitigate the spread of diseases. In **economics**, cause and effect analysis is vital for policy-making. Policymakers need to understand how changes in economic variables, such as interest rates or taxes, affect economic indicators like inflation or employment rates. This knowledge helps in crafting policies that promote economic stability and growth. In **environmental science**, recognizing the causes of climate change and pollution is critical for developing strategies to mitigate these issues. For example, understanding the impact of carbon emissions on global warming informs policies aimed at reducing greenhouse gas emissions. In **engineering**, cause and effect principles are applied to design and troubleshoot systems. Engineers must identify the causes of system failures to implement corrective measures, ensuring the reliability and safety of infrastructure and machinery. In **psychology**, understanding cause and effect helps in addressing mental health issues. Therapists use this concept to identify the underlying causes of behavioral problems and develop targeted interventions. In **education**, recognizing cause and effect relationships enhances teaching methods. Teachers can design more effective lesson plans by understanding how different instructional strategies impact student learning outcomes. Additionally, in **business management**, analyzing cause and effect helps in decision-making processes. Managers can identify the causes of performance issues or market trends to make informed decisions that drive business success. Overall, the concept of cause and effect is a foundational element in many fields, enabling professionals to make informed decisions, solve problems, and drive positive outcomes. By understanding these relationships, individuals can develop more effective solutions to real-world challenges, leading to improved results in various sectors.
Identifying Causes and Effects in Various Contexts
Identifying causes and effects is a fundamental aspect of understanding various phenomena across different disciplines. This critical thinking skill allows us to analyze relationships, predict outcomes, and make informed decisions. In science and technology, cause and effect help researchers understand the mechanisms behind natural processes and technological innovations. In social sciences and human behavior, it aids in comprehending societal dynamics and individual actions. Within economic systems, it enables policymakers to evaluate the impact of economic policies and decisions. By examining these contexts, we can gain a deeper insight into how causes lead to specific effects, thereby enhancing our ability to navigate complex problems effectively. This article will delve into these areas, starting with the intricacies of cause and effect in science and technology, where the precise identification of causal relationships is crucial for advancing knowledge and innovation.
Cause and Effect in Science and Technology
In the realm of science and technology, understanding cause and effect is crucial for advancing knowledge and innovation. This fundamental concept allows researchers to identify the underlying mechanisms that drive phenomena, enabling them to predict outcomes, solve problems, and develop new technologies. For instance, in physics, the cause-and-effect relationship between force and acceleration is a cornerstone of Newton's laws of motion. Here, the application of a force (cause) results in a change in acceleration (effect), which is essential for designing everything from simple machines to complex spacecraft. In biology, cause-and-effect relationships are pivotal in understanding disease mechanisms. For example, the discovery that certain genetic mutations (cause) can lead to specific diseases (effect) has revolutionized genetic engineering and personalized medicine. Similarly, in environmental science, the cause-and-effect link between greenhouse gas emissions (cause) and global warming (effect) informs policies aimed at mitigating climate change. In technology, this principle is applied in software development through debugging processes. When a software bug (cause) leads to an error or malfunction (effect), developers must trace back the sequence of events to identify and fix the root cause. This methodical approach ensures that software systems are reliable and efficient. Moreover, in engineering, understanding cause-and-effect relationships is vital for designing safe and functional systems. For example, in civil engineering, the cause-and-effect relationship between structural stress (cause) and material failure (effect) guides the construction of buildings and bridges that can withstand various loads and environmental conditions. The study of cause and effect also underpins scientific inquiry through the scientific method. Researchers formulate hypotheses based on observed causes and effects, then test these hypotheses through experiments designed to isolate variables and measure outcomes. This systematic approach ensures that conclusions are based on empirical evidence rather than speculation. Furthermore, in fields like medicine and public health, understanding cause-and-effect relationships helps in developing effective treatments and prevention strategies. For instance, identifying the causal link between smoking (cause) and lung cancer (effect) has led to widespread public health campaigns aimed at reducing smoking rates. In summary, the concept of cause and effect is a foundational element in science and technology, enabling scientists and engineers to understand complex phenomena, predict outcomes, and develop innovative solutions. By meticulously identifying and analyzing these relationships, researchers can advance our understanding of the world and drive technological progress. This rigorous approach ensures that scientific knowledge is reliable, applicable, and continually evolving to meet new challenges.
Cause and Effect in Social Sciences and Human Behavior
In the realm of social sciences and human behavior, understanding cause and effect is crucial for analyzing and predicting various phenomena. Causes are the events or conditions that lead to specific outcomes, while effects are the resulting consequences. For instance, in sociology, poverty can be identified as a cause that leads to a range of effects such as poor health outcomes, limited educational opportunities, and increased crime rates. Similarly, in psychology, stress can be a cause that results in effects like anxiety, depression, and decreased productivity. The interplay between cause and effect is often complex and multifaceted. For example, economic policies can have far-reaching effects on societal well-being. A policy aimed at reducing unemployment might have the effect of boosting consumer spending, which in turn could lead to economic growth. However, if not carefully managed, such policies could also have unintended effects like inflation or increased income inequality. In the context of human behavior, understanding cause and effect helps in developing interventions and strategies to address social issues. For instance, recognizing that lack of education is a cause of unemployment can lead to the implementation of educational programs as an effect to reduce unemployment rates. Similarly, understanding that social isolation can be a cause of mental health issues can prompt the development of community programs aimed at fostering social connections as an effect to improve mental health. Moreover, the study of cause and effect in social sciences often involves longitudinal research and statistical analysis to establish causal relationships. Researchers use methods such as regression analysis and experimental designs to isolate variables and determine how changes in one variable affect another. This rigorous approach ensures that conclusions drawn about causes and effects are based on empirical evidence rather than speculation. Furthermore, cultural and environmental factors play significant roles in shaping the causes and effects observed in human behavior. Cultural norms, for example, can influence how individuals respond to stress or economic hardship. Environmental factors such as access to healthcare or educational resources also significantly impact the outcomes of various causes. Therefore, any comprehensive analysis of cause and effect must consider these contextual elements. In conclusion, identifying causes and effects in social sciences and human behavior is essential for understanding societal dynamics and developing effective solutions to social problems. By meticulously examining the relationships between causes and their resulting effects, researchers and policymakers can create targeted interventions that address root causes rather than just symptoms. This nuanced understanding not only enhances our ability to predict future outcomes but also empowers us to make informed decisions that promote positive change across various contexts.
Cause and Effect in Economic Systems
In economic systems, the principle of cause and effect is a fundamental concept that helps understand the dynamics and outcomes of various economic activities. A cause in an economic context can be a policy change, technological innovation, shift in consumer preferences, or external factors such as natural disasters or global events. The effects of these causes can manifest in multiple ways, influencing economic indicators like GDP, inflation rates, employment levels, and overall economic stability. For instance, a government's decision to implement a tax cut (cause) can lead to increased consumer spending and business investment (effect), potentially boosting economic growth. Conversely, an increase in interest rates by a central bank (cause) may reduce borrowing and spending (effect), slowing down economic activity. Technological advancements (cause) can enhance productivity and efficiency (effect), leading to higher output and lower costs. However, they can also lead to job displacement in certain sectors (effect). The interplay between causes and effects in economic systems is often complex and multifaceted. For example, a rise in global oil prices (cause) can lead to higher production costs for manufacturers (effect), which may result in higher prices for consumers (effect). This, in turn, could reduce consumer spending on other goods and services (effect), affecting overall demand and economic activity. Understanding these cause-and-effect relationships is crucial for policymakers, businesses, and individuals to make informed decisions. By analyzing the potential effects of different causes, policymakers can design more effective policies to achieve desired economic outcomes. Businesses can anticipate market changes and adjust their strategies accordingly. Individuals can better navigate the economic landscape by understanding how various factors might impact their financial well-being. Moreover, the study of cause and effect in economics highlights the importance of considering both short-term and long-term implications. While a policy might have immediate positive effects, its long-term consequences could be detrimental. For example, fiscal stimulus packages (cause) may provide short-term economic boosts (effect) but could lead to increased national debt and future economic instability (effect). In conclusion, identifying and understanding the causes and effects in economic systems is essential for predicting and managing economic outcomes. By recognizing the intricate relationships between different economic variables, stakeholders can make more informed decisions that contribute to sustainable economic growth and stability. This understanding not only aids in policy formulation but also helps businesses and individuals adapt to changing economic conditions, ultimately fostering a more resilient and dynamic economy.
Analyzing and Interpreting Cause and Effect Relationships
Analyzing and interpreting cause and effect relationships is a crucial skill in various fields, including science, economics, and social sciences. This complex process involves identifying the causal links between events or variables, understanding the mechanisms behind these relationships, and avoiding common pitfalls that can lead to misinterpretation. To achieve this, it is essential to employ robust methods for identifying causal relationships, which form the foundation of any cause and effect analysis. Additionally, being aware of common pitfalls such as confounding variables, reverse causality, and selection bias is vital to ensure the accuracy of the analysis. Finally, utilizing appropriate tools and techniques, such as regression analysis, experimental design, and data visualization, can significantly enhance the effectiveness of the analysis. By mastering these elements, researchers and analysts can draw reliable conclusions about cause and effect. This article will delve into these critical aspects, starting with the methods for identifying causal relationships.
Methods for Identifying Causal Relationships
Identifying causal relationships is a crucial aspect of analyzing and interpreting cause and effect relationships. Several methods are employed to establish these connections, each with its own strengths and limitations. **Randomized Controlled Trials (RCTs)** are considered the gold standard for determining causality. By randomly assigning participants to treatment or control groups, RCTs minimize confounding variables, allowing researchers to isolate the effect of the intervention. **Instrumental Variables Analysis** is another method used when randomization is not feasible. This approach leverages an instrumental variable that affects the treatment but not the outcome directly, thereby helping to identify causal effects. **Regression Discontinuity Design** is useful in scenarios where treatment assignment is based on a continuous variable and there is a clear cutoff point. By comparing outcomes just above and below this threshold, researchers can estimate the causal effect of the treatment. **Difference-in-Differences** involves comparing the change in outcomes over time between a treatment group and a control group, which helps to account for unobserved confounding variables that are constant over time. **Propensity Score Matching** is a statistical technique that matches individuals with similar characteristics in treatment and control groups, thereby reducing selection bias and allowing for more accurate causal inferences. **Structural Equation Modeling (SEM)** integrates multiple regression equations to model complex relationships between variables, enabling researchers to test hypotheses about causal pathways. **Natural Experiments** take advantage of real-world events or policies that create quasi-experimental conditions, such as changes in laws or economic conditions, to study causal effects without the need for randomization. **Granger Causality Tests** are used in time series analysis to determine if one variable can be used to forecast another variable, indicating potential causality. Each of these methods has its own set of assumptions and requirements but collectively provides a robust toolkit for identifying and interpreting causal relationships. By carefully selecting and applying these methods, researchers can draw more reliable conclusions about cause and effect, which is essential for informed decision-making across various fields such as medicine, economics, and social sciences.
Common Pitfalls in Cause and Effect Analysis
When analyzing cause and effect relationships, several common pitfalls can undermine the accuracy and reliability of your conclusions. One of the most significant pitfalls is **correlation vs. causation confusion**. Just because two events are correlated does not mean that one causes the other; there may be a third variable at play or simply a coincidental relationship. For instance, if you observe that ice cream sales and the number of people wearing shorts are both higher in summer, it doesn't mean that eating ice cream causes people to wear shorts or vice versa. Instead, both are likely influenced by the warmer weather. Another pitfall is **oversimplification**. Cause and effect relationships are often complex and multifaceted, involving numerous variables and interactions. Reducing these relationships to simplistic cause-and-effect chains can lead to misleading conclusions. For example, attributing a rise in crime rates solely to economic downturns ignores other potential factors such as changes in policing strategies, social cohesion, or demographic shifts. **Confirmation bias** is another common issue. Analysts may selectively seek out data that supports their preconceived notions while ignoring contradictory evidence. This biased approach can lead to flawed analyses that reinforce existing beliefs rather than uncovering true causal relationships. Additionally, **temporal ambiguity** can complicate cause and effect analysis. Determining the sequence of events is crucial; if the supposed effect precedes the cause, the relationship is likely spurious. For instance, if a study suggests that a new policy led to improved outcomes but fails to account for pre-existing trends or other interventions that occurred before the policy was implemented, it may incorrectly attribute causality. **Lack of control groups** or inadequate sampling methods can also skew results. Without a proper control group for comparison, it's challenging to isolate the true impact of a supposed cause. Similarly, if the sample size is too small or not representative of the broader population, findings may not generalize well. Furthermore, **reverse causality** must be considered. Sometimes the supposed effect actually causes the supposed cause. For example, if a study finds that people who exercise regularly have lower blood pressure, it might be that lower blood pressure leads people to exercise more rather than the other way around. Lastly, **intervening variables** and **mediating factors** can obscure true causal relationships. These variables can either enhance or diminish the effect of the supposed cause on the supposed effect. Ignoring these intermediaries can result in incomplete or inaccurate analyses. By being aware of these pitfalls—correlation vs. causation confusion, oversimplification, confirmation bias, temporal ambiguity, lack of control groups, reverse causality, and intervening variables—analysts can take steps to ensure more rigorous and reliable cause and effect analyses. This includes using robust statistical methods, considering multiple perspectives and data sources, and carefully controlling for potential confounding factors to draw more accurate conclusions about causal relationships.
Tools and Techniques for Effective Analysis
Effective analysis of cause and effect relationships relies heavily on a suite of tools and techniques that enhance the accuracy, depth, and clarity of the analysis. One fundamental tool is **correlation analysis**, which helps identify whether there is a statistical relationship between variables. However, it is crucial to distinguish correlation from causation using techniques such as **regression analysis**, which can control for confounding variables and provide insights into the direction and magnitude of the relationship. **Causal inference methods**, such as propensity score matching and instrumental variables, are also essential for establishing causality. These methods help in isolating the effect of the cause by accounting for potential biases and confounding factors. **Time series analysis** is another valuable technique, particularly when examining how events unfold over time. It allows analysts to identify patterns, trends, and potential causal relationships between variables that evolve dynamically. **Qualitative methods**, including case studies and content analysis, offer a more nuanced understanding by delving into the context and mechanisms underlying cause and effect relationships. These methods are particularly useful when dealing with complex phenomena where quantitative data may be limited or insufficient. **Data visualization tools** play a critical role in communicating findings effectively. Techniques such as scatter plots, bar charts, and causal diagrams help in illustrating the relationships between variables in a clear and interpretable manner. Additionally, **sensitivity analysis** ensures that the conclusions drawn are robust by testing how changes in assumptions or parameters affect the results. **Machine learning algorithms**, such as decision trees and neural networks, can also be employed to uncover hidden patterns and relationships within large datasets. These algorithms can handle complex interactions between multiple variables, thereby providing a comprehensive view of cause and effect dynamics. Finally, **triangulation**, which involves using multiple methods and data sources to validate findings, enhances the reliability and validity of the analysis. By combining these tools and techniques, analysts can conduct a thorough and rigorous examination of cause and effect relationships, leading to more accurate interpretations and actionable insights.