What Does Vqa Stand For
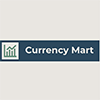
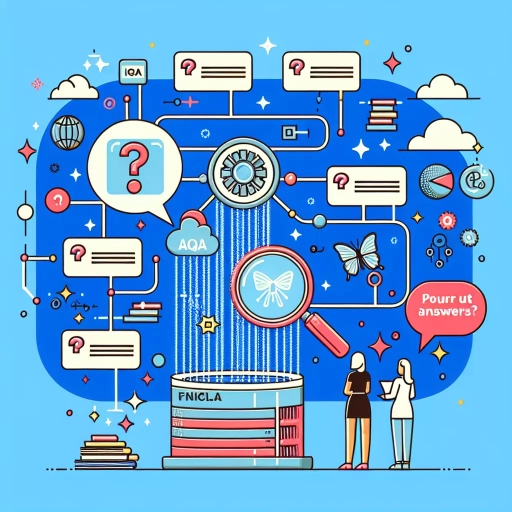
In the realm of artificial intelligence and machine learning, the acronym VQA has become increasingly significant, particularly in the fields of computer vision and natural language processing. VQA, or Visual Question Answering, is a complex task that involves training models to answer questions about images. This technology has far-reaching implications, from enhancing user experiences in search engines to aiding in medical diagnosis. To fully grasp the potential of VQA, it is essential to delve into its definition and context, understand the technical aspects that drive its functionality, and explore its real-world applications and future directions. In this article, we will first provide a comprehensive understanding of VQA: its definition, historical context, and the challenges it aims to address. We will then dive into the technical aspects of VQA, discussing the algorithms, datasets, and models that make this technology possible. Finally, we will examine real-world applications of VQA and speculate on its future directions, highlighting how it is transforming various industries. By exploring these facets, we can appreciate the full scope of what VQA stands for and its transformative impact on modern technology. Let us begin by **Understanding VQA: Definition and Context**.
Understanding VQA: Definition and Context
In the rapidly evolving landscape of artificial intelligence, Visual Question Answering (VQA) has emerged as a pivotal technology that bridges the gap between visual perception and natural language understanding. This innovative field enables machines to interpret and respond to questions about images, revolutionizing various industries from healthcare to education. To fully grasp the significance of VQA, it is essential to delve into its historical background, which traces the development of this technology from its early beginnings to its current state. Understanding the key components of VQA, such as image processing, question analysis, and answer generation, provides a deeper insight into how this complex system operates. Furthermore, exploring the industry applications of VQA reveals its potential to transform sectors like customer service, autonomous vehicles, and medical diagnostics. By examining these aspects, we can gain a comprehensive understanding of VQA and its multifaceted impact. This article aims to provide a detailed exploration of VQA, starting with its definition and context, to equip readers with a thorough understanding of this cutting-edge technology.
Historical Background of VQA
The historical background of Visual Question Answering (VQA) is deeply intertwined with advancements in artificial intelligence, computer vision, and natural language processing. The concept of VQA emerged as a natural extension of these fields, aiming to bridge the gap between visual perception and linguistic understanding. In the early 2000s, researchers began exploring ways to integrate computer vision and natural language processing to enable machines to understand and interpret visual content. This was driven by the increasing availability of large datasets and the development of more sophisticated machine learning algorithms. One of the pivotal moments in the evolution of VQA was the introduction of deep learning techniques, particularly convolutional neural networks (CNNs) for image processing and recurrent neural networks (RNNs) for text analysis. These models significantly improved the accuracy of image recognition tasks and language understanding, laying the groundwork for more complex tasks like VQA. The launch of datasets such as the Visual Question Answering (VQA) dataset in 2015 by researchers from Stanford University, Google, and the University of California, Berkeley, provided a standardized benchmark for evaluating VQA models. This dataset included a large collection of images paired with questions and answers, facilitating the development and testing of VQA algorithms. The subsequent years saw rapid progress in VQA research, with the introduction of various architectures that combined CNNs and RNNs to process both visual and textual inputs. Models like the "Attention-based" approach, which focuses on specific regions of an image relevant to the question being asked, further enhanced performance. The involvement of major tech companies and academic institutions led to significant investments in research, resulting in the development of more robust and efficient VQA systems. Moreover, the rise of transfer learning and pre-trained models such as BERT (Bidirectional Encoder Representations from Transformers) for language tasks and ResNet for image tasks has been instrumental in improving VQA performance. These pre-trained models can be fine-tuned for specific VQA tasks, leveraging knowledge learned from large-scale datasets to achieve better results. Today, VQA continues to evolve with advancements in multimodal learning, where models are designed to process multiple types of input simultaneously. This has opened up new applications in areas such as autonomous driving, healthcare diagnostics, and customer service chatbots. The historical trajectory of VQA highlights a collaborative effort between academia and industry, driven by technological innovations and the pursuit of creating intelligent systems that can interpret and respond to visual information in a human-like manner. Understanding this historical context is crucial for appreciating the current state and future potential of VQA technology.
Key Components of VQA
**Key Components of VQA** Visual Question Answering (VQA) is a complex task that involves several key components, each playing a crucial role in its overall functionality. At its core, VQA integrates computer vision and natural language processing to enable machines to understand and respond to questions about visual content. **Image Understanding** is the first critical component, where the system must accurately interpret the visual elements within an image. This involves object detection, scene understanding, and recognizing relationships between different objects. **Question Analysis** is another vital part, where the system parses the question to identify its intent, context, and the specific information being sought. This requires advanced natural language processing techniques to comprehend nuances in language. **Attention Mechanisms** are also essential in VQA systems. These mechanisms allow the model to focus on specific regions of the image that are relevant to the question being asked, thereby improving the accuracy of the answers. **Knowledge Retrieval** can be integrated to provide additional context or information that might not be immediately visible in the image but is necessary for answering the question accurately. For instance, if a question asks about the function of an object, the system may need to retrieve external knowledge about that object. **Model Architecture** is another key component, with popular architectures including those based on convolutional neural networks (CNNs) for image processing and recurrent neural networks (RNNs) or transformers for text processing. These architectures are often combined in a way that allows them to interact and share information effectively. **Training Data** is crucial for the performance of VQA models; high-quality datasets with diverse images and corresponding questions are necessary for training robust models. Datasets like VQA v2 and CLEVR have been instrumental in advancing research in this field. **Evaluation Metrics** are important for assessing the performance of VQA systems. Common metrics include accuracy, which measures how often the model provides correct answers, and other metrics like BLEU score or METEOR score, which evaluate the quality of generated answers. Finally, **Interpretability** and **Explainability** are becoming increasingly important as VQA models are applied in real-world scenarios where transparency is essential. Techniques such as visualizing attention maps help in understanding how the model arrives at its answers. In summary, the key components of VQA—image understanding, question analysis, attention mechanisms, knowledge retrieval, model architecture, training data, evaluation metrics, and interpretability—work together to create a sophisticated system capable of answering complex questions about visual content. Each component contributes uniquely to the overall performance and reliability of VQA systems.
Industry Applications of VQA
**Industry Applications of VQA** Visual Question Answering (VQA) has emerged as a transformative technology with far-reaching implications across various industries, revolutionizing how we interact with and extract insights from visual data. In **healthcare**, VQA can be used to analyze medical images such as X-rays, MRIs, and CT scans, enabling doctors to quickly identify abnormalities and make accurate diagnoses. For instance, AI models trained on VQA can highlight specific areas of concern in images, reducing the time and effort required for manual analysis. In **retail**, VQA enhances customer service by enabling chatbots and virtual assistants to understand and respond to visual queries. Customers can upload images of products they are interested in, and the AI can provide detailed information about the product, including its availability, price, and features. This not only improves customer satisfaction but also streamlines the shopping experience. The **automotive industry** benefits significantly from VQA through advanced driver-assistance systems (ADAS). These systems use VQA to interpret visual data from cameras and sensors, enabling vehicles to detect obstacles, recognize traffic signs, and navigate safely. This technology is crucial for the development of autonomous vehicles, where accurate and real-time visual understanding is paramount. In **education**, VQA can create interactive learning experiences. For example, students can ask questions about images or videos related to their coursework, and the AI can provide detailed explanations and additional resources. This interactive approach makes learning more engaging and accessible. **Manufacturing** also sees significant gains with VQA, particularly in quality control and inspection processes. AI models can analyze images of products on the production line to detect defects or anomalies, ensuring that only high-quality products reach the market. This automation reduces the need for manual inspections, increasing efficiency and reducing costs. Furthermore, **security and surveillance** leverage VQA to enhance monitoring capabilities. AI systems can analyze footage from security cameras to identify suspicious activities, recognize individuals, and alert authorities in real-time. This proactive approach helps in preventing crimes and ensuring public safety. In **agriculture**, VQA is used to monitor crop health and detect early signs of disease or pests. By analyzing images of crops, farmers can receive timely alerts and take preventive measures, leading to better crop yields and reduced chemical usage. Overall, the applications of VQA are diverse and continue to expand as the technology advances. By integrating VQA into various sectors, industries can achieve greater efficiency, accuracy, and innovation, ultimately driving significant improvements in operational outcomes and customer experiences.
Technical Aspects of VQA
The field of Visual Question Answering (VQA) has emerged as a pivotal intersection of computer vision and natural language processing, aiming to enable machines to understand and respond to questions about visual content. This complex task involves not only recognizing objects and scenes but also interpreting their context and relationships. At the heart of VQA lie sophisticated machine learning algorithms that are designed to process and integrate both visual and textual information. These algorithms, such as those based on deep learning models, are crucial for achieving accurate and meaningful responses. However, their development is heavily reliant on high-quality data sources and datasets, which provide the necessary training ground for these models. Despite significant advancements, VQA still faces numerous challenges, including handling ambiguity, dealing with out-of-vocabulary terms, and ensuring robustness across diverse scenarios. Understanding these technical aspects is essential for advancing VQA capabilities. In this article, we will delve into the machine learning algorithms driving VQA, explore the critical role of data sources and datasets, and discuss the challenges inherent in developing these models. By examining these key components, we can gain a deeper understanding of VQA: its definition, context, and the ongoing efforts to enhance its performance.
Machine Learning Algorithms in VQA
**Technical Aspects of VQA** ### Machine Learning Algorithms in VQA Machine Learning (ML) algorithms are the backbone of Visual Question Answering (VQA) systems, enabling them to interpret and respond to questions about visual content. At the core of these systems are deep learning models that integrate both visual and textual information. One of the most prevalent architectures is the **Attention-based Model**, which focuses on specific regions of an image relevant to the question being asked. This model uses a combination of Convolutional Neural Networks (CNNs) for image feature extraction and Recurrent Neural Networks (RNNs) or Transformers for processing the question. The attention mechanism allows the model to weigh different parts of the image based on their relevance to the query, thereby improving accuracy. Another significant approach is **Graph Neural Networks (GNNs)**, which represent images as graphs where objects and their relationships are nodes and edges, respectively. GNNs can capture complex interactions between objects in a scene, enhancing the model's ability to reason about the visual content. **Multimodal Fusion** techniques are also crucial, as they combine features from both visual and textual inputs effectively. Methods like early fusion, where visual and textual features are combined at an early stage, or late fusion, where they are combined after separate processing, have shown promising results. **Transfer Learning** plays a vital role in VQA by leveraging pre-trained models such as VGG, ResNet, and BERT. These models are fine-tuned on VQA datasets to adapt to the specific task of answering questions about images. Additionally, **Ensemble Methods** have been employed to combine the predictions of multiple models, leading to improved performance and robustness. The use of **Adversarial Training** has also been explored to enhance model resilience against adversarial attacks. Furthermore, **Explainability Techniques** are becoming increasingly important in VQA to provide insights into how models arrive at their answers. Techniques such as saliency maps and feature importance scores help in understanding which parts of the image and question contribute most to the answer. This not only builds trust in the model but also aids in identifying potential biases and areas for improvement. In summary, the integration of various machine learning algorithms and techniques is essential for developing robust and accurate VQA systems. By leveraging attention mechanisms, graph neural networks, multimodal fusion, transfer learning, ensemble methods, adversarial training, and explainability techniques, these systems can effectively interpret visual content and provide meaningful answers to complex questions. As research continues to advance, we can expect even more sophisticated models that further bridge the gap between human perception and machine understanding.
Data Sources and Datasets for VQA
**Data Sources and Datasets for VQA** The efficacy of Visual Question Answering (VQA) systems heavily relies on the quality and diversity of the data sources and datasets used for training and evaluation. High-quality datasets are crucial for developing robust models that can accurately answer questions about images. One of the most prominent datasets in this field is the **VQA v2 dataset**, which contains over 1.1 million questions and answers on 204,721 images from the COCO (Common Objects in Context) dataset. This dataset is particularly valuable due to its balanced distribution of questions across different categories, ensuring that models are not biased towards specific types of questions. Another significant dataset is **Visual Genome**, which provides a comprehensive annotation of objects, attributes, and relationships within images. This dataset includes over 100,000 images with detailed annotations, making it an invaluable resource for training VQA models that require a deep understanding of visual context. **CLEVR (Compositional Language and Elementary Visual Reasoning)** is another notable dataset designed to test the compositional reasoning capabilities of VQA models. It consists of synthetic images with objects of different shapes, colors, and sizes, along with questions that require complex reasoning. In addition to these datasets, **GQA (GuessWhat?!)** and **OK-VQA (Open-Ended Visual Question Answering)** offer unique challenges. **GQA** focuses on object detection and scene understanding by providing questions that require identifying specific objects within an image. **OK-VQA**, on the other hand, includes open-ended questions that demand more nuanced and context-dependent answers, pushing the boundaries of what VQA models can achieve. The diversity in these datasets ensures that VQA models are exposed to a wide range of visual and linguistic variations, enhancing their ability to generalize across different scenarios. Furthermore, ongoing efforts to create more specialized datasets, such as those focused on medical images or specific domains like fashion or cooking, are expanding the applicability of VQA beyond general-purpose scenarios. In summary, the availability of diverse and high-quality datasets such as VQA v2, Visual Genome, CLEVR, GQA, and OK-VQA is essential for advancing the technical aspects of VQA. These datasets not only provide the necessary data for training robust models but also serve as benchmarks for evaluating their performance and identifying areas for improvement. As research continues to evolve, the development of new datasets will remain a critical component in pushing the boundaries of what VQA can achieve.
Challenges in Developing VQA Models
**Challenges in Developing VQA Models** Developing Visual Question Answering (VQA) models poses several significant challenges that underscore the complexity and nuance of this interdisciplinary field. One of the primary hurdles is the **multimodal nature** of VQA, which requires integrating both visual and textual information seamlessly. This integration demands sophisticated architectures capable of extracting meaningful features from images and understanding the context provided by questions. The **semantic gap** between visual and linguistic representations often leads to discrepancies in how models interpret and align these different modalities, necessitating innovative solutions to bridge this gap effectively. Another challenge is **dataset quality and diversity**. High-quality VQA datasets are crucial but often limited by factors such as annotation bias, variability in question types, and the need for diverse and representative samples. For instance, datasets like VQA v2.0 and CLEVR have been instrumental in advancing research, but they still suffer from certain biases that can affect model generalizability. Moreover, **annotating datasets** is a labor-intensive process that requires careful consideration to ensure accuracy and relevance. **Ambiguity and subjectivity** in questions and answers also complicate the development of VQA models. Unlike traditional QA tasks where answers are often clear-cut, VQA questions can have multiple valid answers depending on the context or perspective. This subjectivity makes it challenging to evaluate model performance objectively and necessitates more nuanced evaluation metrics that account for these variations. Additionally, **adversarial attacks** and **robustness** are critical concerns. VQA models must be resilient against adversarial examples designed to mislead them, which can significantly impact their reliability in real-world applications. Ensuring robustness involves not only developing more secure architectures but also understanding the vulnerabilities inherent in current models. **Explainability** is another key challenge; as VQA models become increasingly complex, understanding their decision-making processes becomes more difficult. This lack of transparency can hinder trust in these models, especially in critical domains such as healthcare or autonomous vehicles where interpretability is paramount. Finally, **scalability** remains a significant issue as datasets grow larger and more diverse. Efficiently processing large volumes of data while maintaining performance is essential for practical deployment scenarios. Advances in hardware and software technologies are crucial for overcoming these scalability challenges. Addressing these challenges requires a multifaceted approach that combines advances in computer vision, natural language processing, machine learning, and human-computer interaction. By tackling these issues head-on, researchers can develop more robust, reliable, and interpretable VQA models that meet the demands of real-world applications.
Real-World Applications and Future Directions
In the rapidly evolving landscape of artificial intelligence, Visual Question Answering (VQA) has emerged as a pivotal technology with far-reaching implications across various sectors. This article delves into the real-world applications and future directions of VQA, highlighting its transformative potential in diverse fields. We explore how VQA is revolutionizing healthcare and medical imaging by enabling precise diagnostics and personalized patient care. Additionally, we examine its role in customer service and support, where it enhances user experience through intelligent and responsive interactions. Looking ahead, we discuss the future trends and innovations that are set to further amplify the impact of VQA. By understanding these applications and directions, readers will gain a comprehensive insight into the multifaceted nature of VQA. To fully appreciate these advancements, it is essential to first grasp the fundamental concepts underlying this technology, which we will address in the subsequent section: Understanding VQA: Definition and Context.
VQA in Healthcare and Medical Imaging
**Visual Question Answering (VQA) in Healthcare and Medical Imaging** Visual Question Answering (VQA) is revolutionizing the healthcare sector, particularly in medical imaging, by enhancing diagnostic accuracy and streamlining clinical workflows. In this domain, VQA involves training AI models to interpret medical images such as X-rays, MRIs, and CT scans, and then answering specific questions about these images. This technology leverages deep learning algorithms to analyze complex visual data and provide precise answers to queries posed by healthcare professionals. For instance, a radiologist might ask a VQA system to identify the presence of a tumor in an MRI scan or to measure the size of a fracture in an X-ray image. The system processes the image data and generates a response based on its learned patterns from extensive training datasets. The integration of VQA in healthcare offers several benefits. Firstly, it aids in reducing the workload of radiologists and clinicians by automating routine tasks such as image annotation and preliminary diagnosis. This allows medical professionals to focus on more complex cases and improve patient care. Secondly, VQA enhances diagnostic accuracy by minimizing human error and providing consistent interpretations across different images. Additionally, it facilitates better patient communication by enabling healthcare providers to explain diagnoses more clearly using visual aids. Moreover, VQA has the potential to democratize access to quality healthcare by enabling remote consultations and telemedicine services. In regions with limited access to specialized medical professionals, VQA systems can assist local healthcare workers in interpreting medical images accurately. This not only improves the speed of diagnosis but also ensures that patients receive timely and appropriate treatment. Looking ahead, future directions for VQA in healthcare involve further advancements in AI algorithms to handle more nuanced and context-dependent questions. Integrating VQA with other AI technologies like natural language processing (NLP) could enable more sophisticated interactions between healthcare providers and AI systems. For example, a clinician could engage in a dialogue with the VQA system to refine diagnoses or explore different treatment options based on image analysis. Furthermore, the integration of VQA with electronic health records (EHRs) could provide a holistic view of patient data, combining visual insights from medical images with textual information from patient histories. This comprehensive approach would significantly enhance personalized medicine by allowing for more informed decision-making. In conclusion, VQA is poised to transform the landscape of medical imaging and healthcare diagnostics. Its real-world applications are already evident in improved diagnostic accuracy, reduced workload for healthcare professionals, and enhanced patient care. As this technology continues to evolve, it holds immense promise for revolutionizing the way we approach healthcare diagnostics and treatment planning.
VQA in Customer Service and Support
**VQA in Customer Service and Support** In the realm of customer service and support, Visual Question Answering (VQA) is revolutionizing how businesses interact with their clients. VQA, which stands for Visual Question Answering, involves using artificial intelligence to answer questions based on visual data such as images or videos. This technology is particularly valuable in customer service scenarios where visual explanations can be more effective than text-based ones. For instance, when a customer contacts a tech support service with an issue related to a product's assembly or operation, VQA can provide immediate and accurate visual guidance. By analyzing images or videos provided by the customer, VQA systems can identify the problem and offer step-by-step visual instructions for resolution. This not only enhances the customer experience but also reduces the time and effort required by support agents to resolve issues. Moreover, VQA can be integrated into chatbots and virtual assistants to make them more intuitive and user-friendly. For example, a customer might upload a photo of a damaged product to an online support platform; the VQA system can then analyze the image and provide an instant assessment of the damage along with instructions on how to proceed with a return or repair. In addition to troubleshooting, VQA can also be used for product demonstrations and tutorials. Companies can create interactive visual guides that allow customers to ask questions about specific parts of a product or process, receiving detailed visual responses in real-time. This enhances customer engagement and satisfaction by providing clear and concise information tailored to their needs. Looking ahead, the future of VQA in customer service holds significant promise. As AI technologies continue to advance, we can expect more sophisticated VQA systems that can handle complex queries and provide personalized support experiences. The integration of VQA with other technologies like augmented reality (AR) could further enhance these capabilities, allowing customers to receive immersive and interactive support experiences that simulate real-world interactions. Overall, the application of VQA in customer service represents a significant leap forward in terms of efficiency, effectiveness, and customer satisfaction. By leveraging visual data to answer questions and provide support, businesses can deliver more personalized and engaging experiences that set them apart from competitors and drive long-term customer loyalty. As this technology continues to evolve, it will undoubtedly play an increasingly crucial role in shaping the future of customer service and support.
Future Trends and Innovations in VQA
**Future Trends and Innovations in VQA** Visual Question Answering (VQA) is poised to undergo significant transformations driven by cutting-edge technologies and emerging trends. One of the most promising areas is the integration of multimodal learning, where VQA systems will leverage not just visual data but also incorporate auditory, textual, and even tactile inputs to provide more comprehensive and accurate responses. This holistic approach will enhance the robustness of VQA models, enabling them to handle complex queries that require a deeper understanding of context. Another trend is the adoption of explainable AI (XAI) in VQA. As AI becomes more pervasive, there is a growing need for transparency and accountability in AI decision-making processes. XAI will allow VQA systems to provide insights into how they arrive at their answers, fostering trust and reliability. This is particularly crucial in high-stakes applications such as healthcare and finance, where the accuracy and rationale behind AI-driven decisions are paramount. Advancements in deep learning architectures, such as transformers and attention mechanisms, will continue to play a pivotal role in improving VQA performance. These models have already shown remarkable capabilities in natural language processing and computer vision tasks, and their application in VQA is expected to yield substantial improvements in accuracy and efficiency. The rise of edge computing and IoT devices will also impact VQA, enabling real-time processing and analysis of visual data at the edge rather than relying on cloud-based services. This shift will reduce latency, enhance privacy, and make VQA more accessible in various real-world scenarios, from smart homes to autonomous vehicles. Furthermore, the increasing availability of large-scale datasets and the development of more sophisticated data augmentation techniques will drive innovation in VQA. These datasets will include diverse and nuanced visual content, allowing models to learn from a broader range of scenarios and improve their generalizability. Lastly, ethical considerations will become more prominent as VQA becomes more integrated into daily life. Ensuring that VQA systems are fair, unbiased, and respectful of privacy will be essential. Researchers and developers must prioritize ethical AI practices to avoid perpetuating existing social inequalities and to build trust with users. In summary, the future of VQA is marked by a convergence of advanced technologies, ethical considerations, and real-world applications. As these trends and innovations evolve, we can expect VQA to become an indispensable tool across various industries, enhancing decision-making processes and improving human-computer interaction.