What Is Ddf
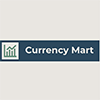
Understanding the Basics of DDF
Understanding the basics of DDF (Data Definition Framework) is crucial for anyone involved in data management and analysis. This framework provides a structured approach to defining, organizing, and utilizing data, which is essential for making informed decisions. To grasp the fundamentals of DDF, it is important to delve into three key areas: its definition and origins, its key components and structure, and its historical context and evolution. Firstly, understanding the definition and origins of DDF sets the foundation for comprehending its purpose and development. This involves exploring how DDF emerged as a response to the need for standardized data management practices. Secondly, examining the key components and structure of DDF reveals how it operates in practice. This includes understanding the various elements that make up the framework and how they interact to ensure data integrity and consistency. Lastly, considering the historical context and evolution of DDF provides insight into how it has adapted over time to meet changing data management needs. This perspective helps in appreciating the framework's current form and its potential future developments. By starting with the definition and origins of DDF, we can establish a clear understanding of its foundational principles before diving deeper into its components and historical development.
Definition and Origins
**Definition and Origins** Dynamic Data Fabric (DDF) is a cutting-edge technology designed to manage, integrate, and analyze vast amounts of data from diverse sources in real-time. At its core, DDF is an advanced data management system that leverages artificial intelligence, machine learning, and cloud computing to provide a unified view of data across an organization. This innovative approach allows for the seamless integration of structured and unstructured data, enabling businesses to make informed decisions quickly and efficiently. The origins of DDF can be traced back to the early 2000s when the need for more sophisticated data management solutions became apparent. As big data began to dominate the business landscape, traditional data management systems struggled to keep up with the sheer volume, velocity, and variety of data being generated. In response, tech innovators started developing new architectures that could handle these challenges. The term "Dynamic Data Fabric" emerged as a way to describe these next-generation systems that could dynamically adapt to changing data environments. Key components of DDF include data ingestion, processing, storage, and analytics. Data ingestion involves collecting data from various sources such as databases, IoT devices, social media platforms, and more. Advanced processing capabilities then transform this raw data into actionable insights using machine learning algorithms and real-time analytics. Storage solutions are designed to be scalable and flexible, often leveraging cloud infrastructure to handle large volumes of data efficiently. The evolution of DDF has been influenced by several technological advancements. Cloud computing has provided the necessary scalability and cost-effectiveness for handling big data. Artificial intelligence and machine learning have enabled DDF systems to learn from data patterns and make predictions or recommendations autonomously. Additionally, advancements in data virtualization have allowed DDF to integrate disparate data sources without the need for physical data movement or replication. In practice, DDF is used across various industries including finance, healthcare, retail, and manufacturing. For instance, in finance, DDF can help in fraud detection by analyzing transactional data in real-time. In healthcare, it can assist in patient care by integrating medical records with real-time health monitoring data. The flexibility and adaptability of DDF make it an indispensable tool for any organization looking to derive meaningful insights from their data assets. Understanding the basics of DDF is crucial for organizations aiming to leverage this powerful technology effectively. By grasping its definition and origins, businesses can better appreciate how DDF can transform their data management practices and drive strategic decision-making. As technology continues to evolve, the role of DDF will only become more pivotal in enabling organizations to stay competitive in a data-driven world.
Key Components and Structure
Understanding the basics of DDF (Data Distribution Facility) begins with grasping its key components and structure. At its core, DDF is a standardized framework designed to facilitate the efficient distribution and management of data across various systems and networks. The primary components include: 1. **Data Sources**: These are the origins of the data, which can range from databases, APIs, to real-time sensors. Data sources provide the raw information that needs to be processed and distributed. 2. **Data Processing**: This component involves transforming raw data into a usable format through cleaning, filtering, and aggregating. It ensures that the data is consistent and ready for distribution. 3. **Data Storage**: This refers to the repositories where processed data is stored. Common storage solutions include relational databases, NoSQL databases, and cloud storage services. 4. **Data Distribution**: This is the heart of DDF, responsible for delivering data to various endpoints such as applications, analytics tools, or other systems. Distribution mechanisms can include APIs, message queues, and file transfers. 5. **Metadata Management**: Metadata provides context about the data, including its origin, format, and usage history. Effective metadata management ensures that data is traceable and understandable. 6. **Security and Governance**: These components are crucial for ensuring that data is protected from unauthorized access and misuse. They involve implementing encryption, access controls, and compliance with regulatory standards. 7. **Monitoring and Logging**: Continuous monitoring and logging help in identifying issues, optimizing performance, and maintaining the integrity of the data distribution process. The structure of DDF typically follows a layered architecture: - **Ingestion Layer**: Collects data from various sources. - **Processing Layer**: Transforms and prepares the data for distribution. - **Storage Layer**: Holds processed data in a structured manner. - **Distribution Layer**: Delivers data to target systems or applications. - **Management Layer**: Oversees metadata, security, governance, and monitoring. Each layer interacts seamlessly to ensure that data flows efficiently from source to destination while maintaining quality and security. By understanding these key components and the layered structure of DDF, organizations can better manage their data assets, enhance decision-making processes, and improve overall operational efficiency. This foundational knowledge is essential for leveraging DDF effectively in modern data-driven environments.
Historical Context and Evolution
The historical context and evolution of DDF (Data Distribution Facility) are deeply intertwined with the advancements in data management and distribution technologies. Initially, DDF emerged in the 1980s as a response to the growing need for standardized data exchange formats, particularly within financial and trading communities. This period saw the rise of electronic data interchange (EDI) systems, which laid the groundwork for more sophisticated data distribution mechanisms. In the early 1990s, the introduction of XML (Extensible Markup Language) revolutionized data formatting and exchange by providing a flexible, human-readable format that could be easily parsed by machines. This shift enabled DDF to adopt XML as its core structure, enhancing its ability to handle complex data sets and ensuring interoperability across diverse systems. The late 1990s and early 2000s witnessed significant advancements in internet technologies, leading to the widespread adoption of web services and APIs. These developments allowed DDF to transition from traditional file-based exchanges to real-time, web-based data distribution. This evolution enabled faster, more reliable, and more scalable data dissemination, catering to the increasing demands of global financial markets and other data-intensive industries. As cloud computing gained prominence in the mid-2000s, DDF further evolved to leverage cloud infrastructure for enhanced scalability, security, and cost efficiency. Cloud-based DDF solutions enabled organizations to manage large volumes of data more effectively, ensuring high availability and redundancy. In recent years, the integration of artificial intelligence (AI) and machine learning (ML) has further transformed DDF. These technologies have enabled advanced data analytics, predictive modeling, and automated decision-making processes. Modern DDF systems now incorporate AI-driven tools for data validation, error detection, and optimization, significantly improving the accuracy and reliability of distributed data. Moreover, the advent of blockchain technology has introduced new possibilities for secure and transparent data distribution. By leveraging blockchain's decentralized architecture, DDF can ensure tamper-proof data integrity and traceability, which is particularly crucial in sectors like finance and healthcare where data security is paramount. Throughout its evolution, DDF has remained a cornerstone in facilitating efficient and reliable data exchange across various industries. Its adaptability to emerging technologies has ensured its relevance in an ever-changing digital landscape. Understanding the historical context and evolution of DDF is essential for grasping its current capabilities and future potential, especially as organizations continue to rely on robust data distribution mechanisms to drive their operations and strategic decisions.
Applications and Uses of DDF
The Applications and Uses of DDF (Difluorodiphenyl) are multifaceted and far-reaching, impacting various sectors of society. This versatile compound is not only a cornerstone in industrial and commercial applications but also plays a significant role in scientific research and development. Additionally, its presence is felt in everyday life through numerous consumer products. In the industrial and commercial sphere, DDF is utilized for its unique properties, enhancing manufacturing processes and product quality. In scientific research, it serves as a crucial reagent, facilitating groundbreaking discoveries. Meanwhile, in everyday life, DDF is embedded in consumer goods, contributing to their functionality and durability. This article will delve into these three key areas, starting with the industrial and commercial uses of DDF, where its impact is most pronounced. By exploring these applications, we can gain a deeper understanding of how DDF shapes our world across different domains. --- **Industrial and Commercial Uses**
Industrial and Commercial Uses
Industrial and commercial uses of DDF (Difluorodiphenyl) are diverse and critical, leveraging its unique chemical properties to enhance various processes and products. In the manufacturing sector, DDF is utilized as a precursor in the synthesis of advanced materials such as fluoropolymers, which are renowned for their exceptional thermal stability, chemical resistance, and non-stick properties. These fluoropolymers are integral in the production of high-performance coatings, seals, and linings used in chemical processing equipment, automotive components, and medical devices. In the electronics industry, DDF plays a crucial role in the development of advanced semiconductors and circuit boards. Its ability to form stable and durable fluorinated compounds makes it an essential component in the fabrication of microelectronic devices, ensuring reliable performance under extreme conditions. Additionally, DDF is used in the formulation of etching agents and cleaning solutions that are vital for maintaining the purity and precision required in semiconductor manufacturing. The pharmaceutical industry also benefits significantly from DDF, as it is involved in the synthesis of certain fluorinated drugs that exhibit enhanced bioavailability and metabolic stability. These drugs are critical in treating various diseases, including cancer and neurological disorders. Furthermore, DDF's derivatives are used as intermediates in the production of diagnostic imaging agents, such as fluorinated contrast agents for MRI and PET scans. In commercial applications, DDF's derivatives find extensive use in consumer products. For instance, fluorinated compounds derived from DDF are used in non-stick cookware coatings, ensuring food does not adhere to surfaces and making cleaning easier. These compounds are also incorporated into waterproofing treatments for fabrics and textiles, enhancing their durability and resistance to environmental elements. Moreover, DDF is utilized in the development of advanced lubricants and greases that provide superior performance under extreme temperatures and pressures. These lubricants are essential in industrial machinery, aerospace applications, and automotive systems, where reliability and efficiency are paramount. In environmental remediation, DDF-based compounds are employed as solvents for cleaning contaminated soil and groundwater due to their ability to dissolve a wide range of organic pollutants without leaving harmful residues. This application is particularly important for sites contaminated with hazardous chemicals. Overall, the industrial and commercial uses of DDF underscore its versatility and importance across multiple sectors. Its unique chemical attributes make it an indispensable component in various high-tech applications, contributing significantly to advancements in materials science, electronics, pharmaceuticals, consumer products, and environmental sustainability.
Scientific Research and Development
Scientific research and development (R&D) are the cornerstone of advancing knowledge and innovation, particularly in the context of technologies like DDF (Data-Driven Frameworks). R&D involves systematic investigation and experimentation aimed at discovering new knowledge or creating new products, processes, or services. In the realm of DDF, R&D is crucial for enhancing data analytics capabilities, improving decision-making processes, and optimizing operational efficiency. Through rigorous scientific methods, researchers can develop and refine DDFs to better handle complex data sets, integrate diverse data sources, and provide actionable insights. This process often involves iterative cycles of hypothesis formulation, experimentation, data collection, analysis, and validation. For instance, in healthcare, R&D in DDF can lead to the development of predictive models that identify high-risk patients or detect early signs of diseases, thereby improving patient outcomes and reducing healthcare costs. In industry, R&D in DDF can drive innovation by enabling companies to leverage big data for strategic decision-making. For example, in manufacturing, advanced DDFs can optimize production workflows, predict equipment failures, and enhance supply chain management. Similarly, in finance, DDFs developed through R&D can help detect fraud, predict market trends, and optimize investment strategies. The applications of R&D in DDF are vast and diverse. In environmental science, DDFs can be used to monitor climate changes, predict natural disasters, and develop sustainable resource management strategies. In education, R&D in DDF can lead to personalized learning systems that tailor educational content to individual students' needs and abilities. Moreover, the collaborative nature of R&D fosters interdisciplinary approaches where experts from various fields come together to tackle complex problems. This collaboration not only accelerates the development of DDFs but also ensures that these frameworks are robust, reliable, and applicable across different sectors. In summary, scientific research and development are essential for the advancement and application of DDFs. By continuously pushing the boundaries of what is possible with data-driven technologies, R&D ensures that DDFs remain a powerful tool for driving innovation and solving real-world problems.
Everyday Life and Consumer Products
Everyday life is intricately woven with consumer products, each designed to enhance convenience, comfort, and efficiency. From the moment we wake up to the time we go to bed, we interact with a myriad of products that have been engineered to make our lives easier. For instance, the toothpaste we use in the morning contains surfactants that help in cleaning teeth effectively, while the shampoo we apply has emulsifiers that ensure a rich lather and thorough cleansing. The clothes we wear are treated with fabric softeners and stain repellents, making them more durable and easier to maintain. In the kitchen, consumer products play a crucial role in food preparation and storage. Cooking oils and margarines contain emulsifiers like mono- and diglycerides that help in blending ingredients smoothly, while baking products often include leavening agents to ensure light and fluffy textures. Refrigerators and freezers use advanced insulation materials to keep food fresh for longer periods. Even the packaging of food items, such as plastic wraps and aluminum foils, relies on specialized materials that prevent spoilage and maintain freshness. Personal care products are another significant category where consumer goods make a substantial impact. Skincare products like moisturizers and sunscreens contain emollients and UV protectants that help in maintaining healthy skin. Hair care products, including conditioners and hair dyes, use various chemicals to enhance texture and color. Makeup items such as foundations and lipsticks also rely on a blend of ingredients to provide desired effects. The role of consumer products extends beyond personal care to household maintenance. Cleaning agents like detergents and disinfectants contain surfactants and antimicrobial agents that help in removing dirt and killing germs. Air fresheners and deodorizers use fragrances and odor-neutralizing chemicals to keep homes smelling pleasant. Even the paint on our walls contains additives that improve durability and color retention. In the realm of technology, consumer electronics are ubiquitous. Smartphones, laptops, and televisions all rely on advanced materials such as semiconductors, display panels, and batteries to function efficiently. These devices are often encased in durable plastics or metals that protect them from damage while maintaining aesthetic appeal. The applications of DDF (Dioctyl Decyl Dimerate) in these everyday consumer products are multifaceted. As a versatile chemical compound, DDF can act as an emulsifier in personal care products like lotions and creams, ensuring uniform distribution of ingredients for better skin absorption. In food packaging, DDF can be used as a barrier coating to prevent moisture and oxygen from reaching the contents, thereby extending shelf life. Its use in household cleaning agents can enhance the effectiveness of detergents by improving their ability to emulsify oils and grease. In summary, consumer products are integral to our daily lives, offering solutions to various needs ranging from personal hygiene to household maintenance. The diverse applications of DDF across these products underscore its importance as a key component in enhancing their performance and efficacy. Whether it's in the kitchen, bathroom, or living room, DDF plays a silent yet crucial role in making our everyday lives more convenient and enjoyable.
Benefits, Challenges, and Future Perspectives
The exploration of benefits, challenges, and future perspectives in any field is a multifaceted endeavor that requires a comprehensive understanding of its various dimensions. This article delves into the intricacies of these aspects, providing a balanced view that highlights both the advantages and benefits, as well as the challenges and limitations. Additionally, it offers insights into future trends and innovations that are set to shape the landscape. By examining these three key areas, readers can gain a holistic understanding of the subject matter. The advantages and benefits section will explore how certain initiatives or technologies have positively impacted various sectors, enhancing efficiency, productivity, and overall quality. This will be followed by an analysis of the challenges and limitations, which will discuss the obstacles and drawbacks that need to be addressed. Finally, the future trends and innovations section will look ahead to emerging developments that promise to overcome current limitations and drive further progress. Transitioning first to the advantages and benefits, we will see how these positive outcomes have set the stage for continued growth and improvement.
Advantages and Benefits
The advantages and benefits of DDF (Data-Driven Frameworks) are multifaceted and significant, making them a cornerstone in modern business and technological landscapes. **Efficiency and Accuracy**: DDFs leverage advanced analytics and machine learning algorithms to process vast amounts of data, leading to more accurate decision-making. This precision reduces the likelihood of human error, thereby enhancing operational efficiency. **Scalability**: These frameworks are designed to handle large volumes of data, making them highly scalable. As organizations grow, DDFs can adapt seamlessly, ensuring that data-driven insights continue to support strategic decisions. **Real-Time Insights**: By processing data in real-time, DDFs provide immediate feedback, enabling businesses to respond swiftly to market changes, customer needs, and internal performance metrics. This agility is crucial in competitive environments where timely actions can make a substantial difference. **Cost Savings**: Automating data analysis through DDFs reduces the need for manual intervention, saving time and resources. Additionally, by identifying inefficiencies and optimizing processes, these frameworks can lead to significant cost savings over time. **Enhanced Customer Experience**: By analyzing customer behavior and preferences, DDFs help businesses tailor their offerings to meet specific needs, leading to higher customer satisfaction and loyalty. **Innovation**: The insights derived from DDFs often uncover new opportunities for innovation, whether it's developing new products, improving existing services, or exploring untapped markets. **Risk Management**: Advanced analytics within DDFs can predict potential risks and anomalies, allowing organizations to take proactive measures to mitigate them. This proactive approach to risk management helps in maintaining stability and continuity. **Compliance**: In regulated industries, DDFs can ensure that all data processes comply with legal and regulatory standards, reducing the risk of non-compliance and associated penalties. Overall, the benefits of DDFs are clear: they drive efficiency, accuracy, scalability, and innovation while enhancing customer experience and risk management capabilities. These advantages position DDFs as indispensable tools for any organization aiming to thrive in today's data-driven world.
Challenges and Limitations
While the benefits of DDF (Data-Driven Frameworks) are numerous, it is crucial to acknowledge the challenges and limitations that accompany their implementation. One of the primary challenges is the **data quality issue**; DDFs rely heavily on accurate and comprehensive data, but poor data quality can lead to flawed insights and decision-making. Additionally, **data privacy and security concerns** are significant, as sensitive information must be protected from unauthorized access and breaches. The **complexity of integrating DDFs** with existing systems can also pose a challenge, requiring substantial IT resources and expertise. Furthermore, **organizational resistance to change** can hinder the adoption of DDFs, especially in traditional or conservative environments where there is a preference for established methods. Another limitation is the **need for continuous training and education**; employees must be equipped with the necessary skills to effectively use and interpret data-driven insights, which can be time-consuming and costly. Moreover, **ethical considerations** arise when using data-driven approaches, such as ensuring fairness and avoiding biases in algorithms. Lastly, **scalability issues** can emerge as organizations grow, requiring DDFs to adapt to increasing volumes of data and user demands without compromising performance. Addressing these challenges is essential for maximizing the potential benefits of DDFs and ensuring their successful integration into various sectors.
Future Trends and Innovations
As we delve into the future trends and innovations surrounding DDF (Data-Driven Finance), several key themes emerge that promise to reshape the financial landscape. **Artificial Intelligence (AI) and Machine Learning (ML)** will continue to play a pivotal role, enabling more accurate risk assessments, predictive analytics, and personalized financial services. For instance, AI-driven chatbots and virtual assistants will enhance customer engagement, while ML algorithms will optimize portfolio management and fraud detection. **Blockchain Technology** is another significant trend, offering enhanced security, transparency, and efficiency in financial transactions. The adoption of blockchain will facilitate the development of decentralized finance (DeFi) platforms, which could democratize access to financial services and reduce intermediaries. Additionally, **Internet of Things (IoT)** devices will integrate with financial systems to provide real-time data, enabling smarter financial decisions and more robust risk management. **Quantum Computing** is on the horizon, promising to solve complex financial problems at unprecedented speeds. This technology will revolutionize areas such as derivatives pricing, portfolio optimization, and risk analysis. Furthermore, **Cloud Computing** will continue to advance, providing scalable and secure infrastructure for data storage and processing, which is crucial for the vast amounts of data involved in DDF. The integration of **Big Data Analytics** with DDF will allow for deeper insights into market trends and consumer behavior, enabling more informed decision-making. **RegTech** (Regulatory Technology) will also gain prominence, helping financial institutions comply with regulations more efficiently and reducing the risk of non-compliance. However, these innovations come with their own set of challenges. Ensuring **Data Privacy** and security will be paramount as more sensitive information is processed through these advanced technologies. The need for **Cybersecurity** measures will intensify to protect against sophisticated cyber threats. Moreover, the ethical use of AI and ML must be addressed to avoid biases and ensure fairness in financial decision-making processes. Looking ahead, the future perspectives of DDF are promising but also fraught with complexities. As these technologies mature, they will likely lead to a more efficient, transparent, and inclusive financial system. However, it is crucial for stakeholders to address the challenges proactively to fully harness the benefits of these innovations. By doing so, DDF can drive significant growth and improvement in the financial sector, ultimately benefiting both institutions and consumers alike.