What Is Relative Frequency
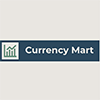
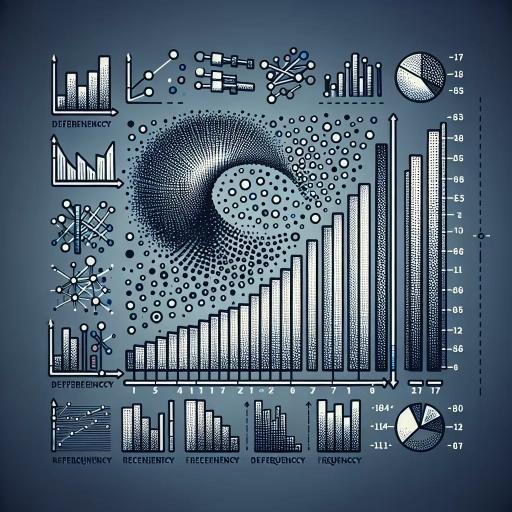
Relative frequency is a fundamental concept in statistics that helps us understand the distribution and occurrence of data within a dataset. It measures the proportion of times a particular value or category appears in relation to the total number of observations. This metric is crucial for analyzing and interpreting data, as it provides insights into the likelihood and prevalence of different outcomes. In this article, we will delve into the essence of relative frequency, starting with **Understanding Relative Frequency**, where we will explore its definition and significance. We will then move on to **Calculating Relative Frequency**, detailing the step-by-step process of how to compute this metric. Finally, we will discuss **Interpreting and Using Relative Frequency**, highlighting how this information can be applied in real-world scenarios to make informed decisions. By grasping these concepts, readers will gain a comprehensive understanding of relative frequency and its practical applications. Let's begin by **Understanding Relative Frequency**.
Understanding Relative Frequency
Understanding relative frequency is a fundamental concept in statistics that helps us grasp the distribution and occurrence of events within a dataset. This concept is crucial for making informed decisions and analyzing data effectively. In this article, we will delve into the definition and concept of relative frequency, exploring how it differs from other statistical measures and its significance in data analysis. We will also examine the mathematical representation of relative frequency, including the formulas and calculations involved, to provide a clear understanding of how it is computed. Additionally, we will discuss real-world applications of relative frequency, highlighting its importance in fields such as business, healthcare, and social sciences. By understanding these aspects, readers will gain a comprehensive insight into relative frequency and its practical uses, ultimately enhancing their ability to interpret and utilize data more accurately. Transitioning seamlessly into the core of our discussion, let's begin by exploring the intricacies of **Understanding Relative Frequency**.
Definition and Concept
**Definition and Concept** Relative frequency is a statistical measure that quantifies the proportion of times a particular value or category occurs within a dataset. It is an essential concept in understanding the distribution and characteristics of data, particularly when analyzing categorical or discrete variables. To define it more precisely, relative frequency is calculated by dividing the frequency of each category by the total number of observations in the dataset. This results in a value between 0 and 1, which can also be expressed as a percentage by multiplying by 100. The concept of relative frequency is crucial because it provides a normalized view of how often different values appear in the data. Unlike absolute frequency, which simply counts the number of occurrences, relative frequency allows for comparisons across different datasets or categories by standardizing the counts to a common scale. For instance, if you are analyzing survey responses where participants can choose from multiple categories (e.g., age groups), relative frequency helps you understand the proportion of respondents in each age group relative to the total number of respondents. In practical terms, relative frequency is often used in data visualization tools such as bar charts and pie charts to illustrate the distribution of data visually. For example, in a bar chart showing the relative frequencies of different age groups in a population, each bar's height would represent the proportion of individuals in that age group out of the total population. This visual representation makes it easier to identify trends and patterns within the data. Moreover, relative frequency plays a significant role in statistical inference and hypothesis testing. By understanding the relative frequencies of different outcomes or categories, researchers can make informed decisions about whether observed patterns are due to chance or if they reflect underlying phenomena. For instance, in medical research, relative frequencies might be used to compare the incidence rates of a disease across different treatment groups. In summary, relative frequency is a fundamental concept in statistics that helps in understanding and interpreting data by providing a proportional view of how often different values occur. Its application extends from simple data visualization to complex statistical analyses, making it an indispensable tool for anyone working with data. By grasping this concept, one can better comprehend the underlying structure of their dataset and make more accurate interpretations and decisions based on that data.
Mathematical Representation
**Mathematical Representation** Understanding relative frequency is deeply rooted in the mathematical representation of data, which provides a clear and quantifiable way to analyze and interpret statistical information. At its core, relative frequency is a measure that expresses the proportion of times a particular value or category occurs within a dataset. Mathematically, it is calculated by dividing the frequency of each category by the total number of observations. This can be represented as \( \text{Relative Frequency} = \frac{\text{Frequency of Category}}{\text{Total Number of Observations}} \). For instance, if a survey of 100 students reveals that 30 students prefer mathematics, the relative frequency for this preference would be \( \frac{30}{100} = 0.3 \) or 30%. To further illustrate this concept, consider a histogram or bar chart where each bar represents a category and its height corresponds to the frequency. By scaling these heights by the total number of observations, we transform these frequencies into relative frequencies, allowing for direct comparison across different datasets or categories. This transformation is crucial because it standardizes the data, making it easier to understand and communicate trends and patterns. In addition to histograms, other graphical tools such as pie charts and cumulative frequency distributions also rely on mathematical representations to visualize relative frequencies. For example, in a pie chart, each slice's area is proportional to its relative frequency within the dataset. Similarly, cumulative frequency distributions plot the running total of frequencies against each category, providing insights into how often different values occur up to a certain point. The mathematical representation of relative frequency extends beyond visual tools; it also forms the basis for more advanced statistical analyses. For instance, in probability theory, relative frequencies are used to estimate probabilities of events occurring. The law of large numbers states that as the sample size increases, the relative frequency of an event will converge to its true probability. This principle underpins many statistical tests and confidence intervals used in research and decision-making processes. Moreover, mathematical representations enable the application of statistical models and algorithms that rely on relative frequencies. For example, in machine learning algorithms like Naive Bayes classifiers, relative frequencies are used to calculate posterior probabilities based on prior probabilities and likelihoods. These models leverage mathematical representations to make predictions and classify data accurately. In summary, the mathematical representation of relative frequency is essential for understanding and analyzing data effectively. By converting raw frequencies into proportions, we gain a standardized measure that facilitates comparison and visualization across various datasets. This foundational concept in statistics not only enhances our ability to interpret data but also serves as a building block for more sophisticated statistical techniques and models.
Real-World Applications
Understanding relative frequency is crucial for a wide range of real-world applications, where data analysis and interpretation are paramount. In **medicine**, relative frequency helps in the assessment of treatment efficacy and side effects. For instance, if a new drug is tested on 1,000 patients and 800 show improvement, the relative frequency of successful treatment is 80%, which provides a clear metric for healthcare professionals to evaluate the drug's effectiveness. Similarly, in **public health**, relative frequency is used to track the prevalence of diseases and the impact of interventions. By analyzing the relative frequency of disease cases over time, health officials can identify trends, predict outbreaks, and allocate resources more efficiently. In **business and marketing**, relative frequency is essential for understanding consumer behavior and market trends. Companies use relative frequency to analyze customer purchasing patterns, helping them tailor marketing strategies and product offerings to meet consumer demands. For example, if a company finds that 70% of its customers prefer online shopping over in-store purchases, it can adjust its sales channels accordingly. This data-driven approach enhances customer satisfaction and drives business growth. In **education**, relative frequency aids in evaluating student performance and curriculum effectiveness. Teachers and administrators can use relative frequency to determine how many students are meeting certain benchmarks or to identify areas where additional support is needed. This helps in refining teaching methods and improving overall educational outcomes. Moreover, in **environmental science**, relative frequency is used to study the distribution of species and the impact of environmental changes. By analyzing the relative frequency of different species in an ecosystem, scientists can monitor biodiversity and detect early signs of ecological imbalance. This information is vital for conservation efforts and policy-making. In **engineering**, relative frequency is applied in quality control and reliability engineering. Manufacturers use relative frequency to assess the failure rates of components or products, which helps in improving design and manufacturing processes. For instance, if a particular component has a high relative frequency of failure, engineers can focus on redesigning or replacing it to enhance overall product reliability. Lastly, in **social sciences**, relative frequency is used to analyze demographic data and social trends. Researchers use this metric to understand population dynamics, such as migration patterns, employment rates, and social attitudes. This information is crucial for policymakers who need to make informed decisions about resource allocation and policy development. In summary, understanding relative frequency is not just an academic concept but a powerful tool with diverse real-world applications. It enables professionals across various fields to make data-driven decisions, improve processes, and drive positive outcomes. By leveraging relative frequency, individuals and organizations can gain deeper insights into complex data sets, leading to more effective strategies and better results.
Calculating Relative Frequency
Calculating relative frequency is a fundamental concept in statistics that helps in understanding the distribution of data within a dataset. It provides a clear insight into how often each value or category appears, making it easier to analyze and interpret the data. In this article, we will delve into the step-by-step process of calculating relative frequency, ensuring that readers grasp the methodology with clarity. Additionally, we will highlight common pitfalls and mistakes that can occur during this calculation, offering practical advice on how to avoid them. We will also explore the various tools and software available for calculating relative frequency, making the process more efficient and accurate. By understanding these key aspects, readers will gain a comprehensive understanding of relative frequency and its application in data analysis. Transitioning seamlessly from these foundational elements, we will ultimately guide you towards a deeper understanding of relative frequency itself.
Step-by-Step Process
When calculating relative frequency, understanding the step-by-step process is crucial for accuracy and clarity. Here’s a detailed breakdown of how to approach this calculation: 1. **Data Collection**: Begin by gathering your dataset, which could be in the form of a list, table, or any other organized format. Ensure that the data is relevant to the question or problem you are trying to address. 2. **Categorization**: If your data is not already categorized, group it into distinct categories or bins. For example, if you're analyzing exam scores, you might categorize them into ranges like 0-49, 50-69, 70-89, and 90-100. 3. **Frequency Count**: Count the number of occurrences in each category. This is known as the absolute frequency. For instance, if you have 10 scores in the 70-89 range, the absolute frequency for that category is 10. 4. **Total Count**: Calculate the total number of data points across all categories. This will be used as the denominator in your relative frequency calculation. 5. **Relative Frequency Calculation**: For each category, divide the absolute frequency by the total count. This gives you the relative frequency, which is often expressed as a percentage or decimal. For example, if there are 10 scores in the 70-89 range and a total of 50 scores, the relative frequency for that category would be \( \frac{10}{50} = 0.2 \) or 20%. 6. **Interpretation**: Analyze and interpret your results. Relative frequencies help in understanding how common each category is within the dataset. They can be used to create histograms, bar charts, or pie charts to visualize the distribution of data. 7. **Verification**: Double-check your calculations for accuracy. Ensure that the sum of all relative frequencies equals 1 (or 100% if expressed as a percentage), which confirms that your calculations are correct. By following these steps meticulously, you can ensure that your relative frequency calculations are accurate and meaningful, providing valuable insights into the distribution and characteristics of your data. This process not only helps in statistical analysis but also in making informed decisions based on data-driven evidence.
Common Pitfalls and Mistakes
When calculating relative frequency, it is crucial to avoid several common pitfalls and mistakes that can lead to inaccurate or misleading results. One of the most prevalent errors is **misunderstanding the concept of relative frequency itself**. Relative frequency is the proportion of times a particular value or category occurs in a dataset, expressed as a percentage or fraction. However, many individuals confuse it with absolute frequency, which is simply the count of occurrences. This confusion can result in incorrect interpretations and conclusions. Another significant mistake is **inadequate data cleaning**. Before calculating relative frequencies, it is essential to ensure that the data is free from errors, inconsistencies, and missing values. Failure to do so can skew the results, leading to an inaccurate representation of the data distribution. For instance, if there are duplicate entries or outliers that have not been addressed, the relative frequencies will be distorted. **Incorrect categorization** is another common pitfall. When dealing with categorical data, it is vital to ensure that categories are mutually exclusive and exhaustive. Overlapping categories or categories that do not cover all possible outcomes can lead to confusion and incorrect calculations. For example, in a survey about favorite colors, if "blue" and "light blue" are treated as separate categories without clear definitions, it may lead to inconsistent results. Additionally, **ignoring sample size** can significantly impact the reliability of relative frequency calculations. A small sample size may not be representative of the population, leading to biased results. It is important to ensure that the sample size is sufficient and representative to draw meaningful conclusions. **Rounding errors** can also be problematic. When calculating relative frequencies, precise calculations are necessary to avoid rounding errors that can accumulate and affect the overall accuracy of the results. Rounding too early in the calculation process can lead to significant discrepancies in the final percentages. Finally, **visual representation** should be approached with caution. While visual aids like bar charts and pie charts can help illustrate relative frequencies effectively, they must be used judiciously. Misleading visual representations can occur if the scales are not properly adjusted or if 3D effects are used inappropriately, which can distort the perception of the data. By being aware of these common pitfalls and taking steps to mitigate them—such as ensuring accurate data, proper categorization, sufficient sample size, precise calculations, and careful visual representation—researchers and analysts can ensure that their relative frequency calculations are accurate and reliable. This attention to detail is crucial for making informed decisions based on data analysis.
Tools and Software for Calculation
When calculating relative frequency, having the right tools and software can significantly streamline the process and enhance accuracy. For those delving into statistical analysis, a variety of tools and software are available to facilitate these calculations. **Spreadsheets:** Microsoft Excel and Google Sheets are among the most popular tools for calculating relative frequency. These spreadsheet applications offer robust functions such as `COUNTIF` and `SUM` that can be combined to compute relative frequencies efficiently. For instance, you can use Excel's `COUNTIF` function to count the occurrences of each category and then divide by the total number of observations using the `SUM` function. **Statistical Software:** Programs like R and Python libraries such as Pandas and NumPy are highly versatile for statistical computations. In R, you can use the `table` function to create frequency tables and then normalize these frequencies to obtain relative frequencies. Similarly, in Python, Pandas' `value_counts` method can be used to count occurrences, and then you can normalize these counts by dividing by the total number of observations. **Online Calculators:** For quick and straightforward calculations, online relative frequency calculators are available. These tools often require minimal input—just the raw data or frequency counts—and provide immediate results. They are particularly useful for small datasets or when you need a quick check without diving into more complex software. **Graphical Tools:** Tools like Tableau or Power BI can also be used to calculate and visualize relative frequencies. These platforms allow you to connect your data sources, perform calculations, and create interactive dashboards that display relative frequencies in a clear and interpretable manner. **Specialized Statistical Packages:** Software packages such as SPSS (Statistical Package for the Social Sciences) and SAS (Statistical Analysis System) offer advanced statistical capabilities including relative frequency calculations. These packages are particularly useful for large-scale data analysis and provide extensive reporting features. In summary, whether you prefer the simplicity of online calculators, the flexibility of spreadsheet software, or the power of specialized statistical packages, there is a tool available to suit your needs when calculating relative frequency. By leveraging these tools effectively, you can ensure accurate and efficient computation of relative frequencies, which is crucial for understanding data distributions and making informed decisions.
Interpreting and Using Relative Frequency
In the realm of data analysis, understanding relative frequency is a cornerstone for making informed decisions and interpreting data effectively. Relative frequency, which measures the proportion of observations falling into different categories, is a powerful tool that helps in uncovering patterns, trends, and statistical significance within datasets. This article delves into the intricacies of interpreting and using relative frequency, exploring three key aspects that are crucial for its practical application. First, we will examine **Data Analysis and Visualization**, highlighting how relative frequency can be visually represented to enhance understanding and communication of data insights. Next, we will discuss **Statistical Significance and Trends**, explaining how relative frequency helps in identifying meaningful patterns and trends within data. Finally, we will explore **Decision Making and Practical Use**, demonstrating how relative frequency informs real-world decisions across various fields. By understanding these facets, readers will gain a comprehensive grasp of relative frequency, enabling them to leverage this statistical concept to drive informed decision-making and deeper insights into their data. Transitioning seamlessly into the heart of the matter, this article aims to illuminate the path to **Understanding Relative Frequency**.
Data Analysis and Visualization
Data analysis and visualization are pivotal components in the interpretation and application of relative frequency, a statistical measure that quantifies the proportion of observations falling into specific categories. When interpreting relative frequency, it is crucial to leverage data analysis techniques to uncover underlying patterns and trends within the data. This involves cleaning and preprocessing the data to ensure accuracy, followed by applying statistical methods such as descriptive statistics and inferential statistics to derive meaningful insights. For instance, calculating the relative frequency helps in understanding how often each category occurs in relation to the total number of observations, which can be particularly useful in categorical data analysis. Visualization plays an equally important role in this process. By transforming raw data into visual representations such as bar charts, pie charts, or histograms, analysts can more easily identify distributions, outliers, and correlations. For example, a bar chart displaying relative frequencies can visually illustrate which categories are most prevalent, allowing for quick and intuitive understanding of the data's structure. Interactive visualizations can further enhance this by enabling users to drill down into specific categories or filter data based on different criteria. Moreover, advanced visualization tools like heatmaps and scatter plots can help in identifying relationships between different variables when relative frequency is used in conjunction with other metrics. These visualizations not only facilitate better comprehension but also aid in communication; complex data insights can be presented in a clear and compelling manner to stakeholders who may not have a deep statistical background. In practical applications, data analysis and visualization combined with relative frequency are essential in various fields such as marketing, healthcare, and finance. For instance, in marketing research, relative frequency analysis can help determine customer preferences by categorizing responses to surveys or feedback forms. Visualizing these frequencies through pie charts or stacked bar charts provides immediate clarity on consumer trends and preferences. In healthcare, analyzing relative frequencies of patient outcomes can help clinicians understand treatment efficacy and identify potential risk factors. Visualizing these outcomes using Kaplan-Meier curves or survival plots allows for a clear depiction of how different treatments impact patient survival rates over time. Ultimately, the synergy between data analysis and visualization when interpreting relative frequency empowers decision-makers with actionable insights derived from robust statistical methods and clear visual representations. This integrated approach ensures that complex data sets are transformed into understandable and actionable information, driving informed decision-making across diverse domains.
Statistical Significance and Trends
When interpreting and using relative frequency, it is crucial to understand the concepts of statistical significance and trends. Statistical significance is a measure that helps determine whether the observed differences or relationships in data are due to chance or if they reflect a real effect. This is often assessed using hypothesis testing, where a null hypothesis (typically stating no effect) is compared against an alternative hypothesis (suggesting an effect). If the p-value, which indicates the probability of observing the data given that the null hypothesis is true, falls below a predetermined significance level (commonly 0.05), the null hypothesis is rejected, and the result is considered statistically significant. This distinction is vital because it helps researchers and analysts to distinguish between random fluctuations and meaningful patterns in their data. Trends, on the other hand, refer to the direction or pattern in which data changes over time or across different categories. Identifying trends involves analyzing how relative frequencies evolve, which can provide insights into underlying processes or changes in the population being studied. For instance, in market research, analyzing the relative frequency of customer preferences over several years can reveal emerging trends that may inform future product development or marketing strategies. Similarly, in healthcare, tracking the relative frequency of disease incidence can help identify trends that may indicate public health issues or the effectiveness of interventions. The interplay between statistical significance and trends is particularly important because it ensures that observed patterns are not merely coincidental but reflect genuine shifts. By combining these two concepts, analysts can make more informed decisions. For example, if a trend in customer preferences is statistically significant, it suggests that the observed change is unlikely to be due to random variation and thus warrants attention and action. Conversely, if a trend is not statistically significant, it may indicate that any observed changes are within the realm of chance and do not necessarily represent a real shift. In practice, interpreting relative frequency in conjunction with statistical significance and trends involves several steps. First, calculate the relative frequencies of different categories or time points to understand the distribution of data. Next, perform statistical tests to determine if any observed differences or trends are statistically significant. Finally, analyze these results to identify meaningful patterns and trends that can guide decision-making or further research. By integrating these methods, analysts can derive robust insights from their data, ensuring that their conclusions are both reliable and actionable. This integrated approach enhances the utility of relative frequency analysis, enabling more accurate and informed interpretations that drive meaningful outcomes.
Decision Making and Practical Use
Decision making is a critical aspect of daily life, and understanding relative frequency can significantly enhance the practical application of this process. Relative frequency, which measures the proportion of times an event occurs within a dataset, provides valuable insights that can guide informed decisions. For instance, in business, relative frequency can help managers determine the most common customer complaints or the frequency of product returns. This information can then be used to prioritize improvements and allocate resources more effectively. In healthcare, relative frequency of symptoms or treatment outcomes can aid clinicians in diagnosing conditions and selecting the most effective treatments. In personal finance, understanding the relative frequency of investment returns can help individuals make more informed decisions about their portfolios. By analyzing the historical data on stock performance, investors can gauge the likelihood of certain returns and adjust their strategies accordingly. Similarly, in education, teachers can use relative frequency to identify common misconceptions among students and tailor their teaching methods to address these areas more effectively. The practical use of relative frequency extends beyond these domains as well. In quality control, manufacturers use relative frequency to monitor defect rates and ensure that products meet quality standards. In marketing, it helps in understanding consumer behavior patterns and designing targeted campaigns. Even in everyday life, relative frequency can be applied to make better choices; for example, knowing the relative frequency of different types of weather can help individuals plan their activities more efficiently. Moreover, relative frequency is not just about raw data; it also involves interpreting trends and patterns. By analyzing these trends over time, decision-makers can anticipate future outcomes and make proactive decisions. For instance, a retailer might notice that a particular product has a high relative frequency of sales during certain months and stock up accordingly to meet demand. In conclusion, integrating relative frequency into decision-making processes adds a layer of precision and reliability. It allows individuals and organizations to move beyond intuition and anecdotal evidence, instead relying on empirical data to drive their choices. Whether in business strategy, healthcare diagnostics, or personal planning, the practical application of relative frequency enhances decision quality and leads to more favorable outcomes. By leveraging this statistical tool, decision-makers can navigate complex scenarios with greater confidence and accuracy.