What Is X Bar
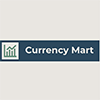
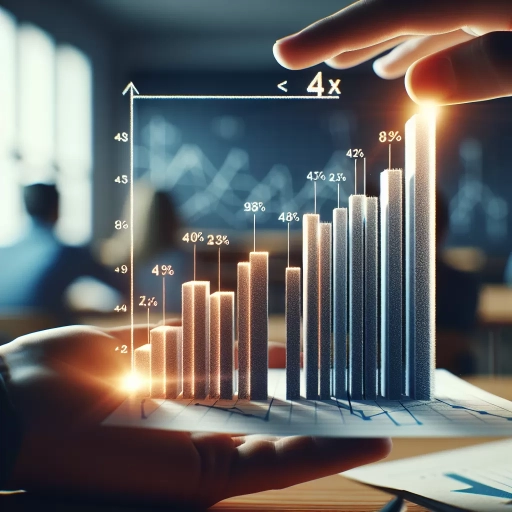
In the realm of statistics and quality control, the concept of "X Bar" (also known as the sample mean) plays a crucial role in understanding and analyzing data sets. This statistical measure is fundamental for assessing the central tendency of a sample, providing insights into the average performance or characteristic of a group. The article delves into the multifaceted nature of X Bar, starting with **Understanding the Concept of X Bar**, where we explore its definition, significance, and how it differs from other statistical measures. Next, we will provide a **Calculating X Bar: Step-by-Step Guide**, detailing the precise methodology for determining this value from raw data. Finally, we will examine **Practical Uses and Real-World Examples of X Bar**, highlighting how this concept is applied in various industries to improve processes and ensure quality standards. By grasping these aspects, readers will gain a comprehensive understanding of X Bar and its indispensable role in statistical analysis. Let's begin by **Understanding the Concept of X Bar**.
Understanding the Concept of X Bar
Understanding the concept of X Bar is crucial for anyone delving into statistical process control and quality management. This concept, often represented as \(\bar{X}\), is a cornerstone in monitoring and improving manufacturing processes. To fully grasp its significance, it is essential to explore three key aspects: its definition and origin, its statistical significance, and its common applications. Firstly, understanding the definition and origin of X Bar provides a foundational knowledge of how this concept evolved and why it remains vital today. This involves delving into the historical context and the mathematical principles that underpin it. Secondly, recognizing the statistical significance of X Bar highlights its role in detecting variations and ensuring process stability. This aspect is critical for maintaining high-quality standards in production. Lastly, examining the common applications of X Bar reveals how it is used in real-world scenarios to enhance efficiency and reliability. By exploring these facets, one can appreciate the comprehensive utility of X Bar in modern manufacturing. Let us begin by examining the definition and origin of this pivotal concept, which sets the stage for a deeper understanding of its broader implications.
Definition and Origin
**Definition and Origin** The concept of "X Bar" (often denoted as \(\bar{X}\)) is a fundamental statistical term that represents the mean or average of a sample. This concept is crucial in understanding statistical analysis, particularly in the context of quality control and data interpretation. The term "X Bar" originates from the field of statistics, where it is used to distinguish the sample mean from the population mean. In statistical notation, the capital letter "X" typically represents a random variable, while the bar over it (\(\bar{X}\)) signifies that it is an average of multiple observations. This distinction is important because it helps analysts differentiate between the sample mean and the population mean (\(\mu\)), which is the average of the entire population. The origin of this notation can be traced back to early statistical developments in the late 19th and early 20th centuries. Statisticians like Karl Pearson and Ronald Fisher contributed significantly to the formalization of statistical methods, including the use of symbols to represent different types of means. The use of \(\bar{X}\) as a symbol for the sample mean became standardized as statistical theory evolved and was widely adopted in various fields such as engineering, economics, and social sciences. Understanding the concept of X Bar is essential for several reasons. Firstly, it allows for the comparison between sample data and known population parameters, which is vital in hypothesis testing and confidence intervals. Secondly, it provides a basis for control charts in quality control processes, where monitoring the mean of samples helps in detecting deviations from expected standards. Lastly, it serves as a foundational element in more advanced statistical analyses such as regression analysis and time series analysis. In practical terms, calculating X Bar involves summing up all the values in a sample and then dividing by the number of observations. This simple yet powerful calculation provides a central tendency measure that can be used to summarize large datasets efficiently. For instance, in manufacturing quality control, X Bar charts are used to monitor whether the average output of a process remains within specified limits over time. In summary, the concept of X Bar is rooted in statistical theory and has become an indispensable tool across various disciplines. Its precise definition and widespread use underscore its importance in data analysis and decision-making processes. As a supporting concept to understanding broader statistical principles, grasping what X Bar represents is crucial for anyone working with data or involved in quality control initiatives.
Statistical Significance
**Understanding the Concept of X Bar: The Role of Statistical Significance** When delving into the concept of X Bar, which represents the mean of a sample of means, it is crucial to understand the underlying statistical principles that validate its significance. Statistical significance is a cornerstone in statistical analysis, serving as a benchmark to determine whether observed differences or relationships are due to chance or if they reflect real effects. In the context of X Bar, statistical significance helps in assessing whether the sample mean is representative of the population mean. Statistical significance is typically evaluated using hypothesis testing, where a null hypothesis (often stating no effect or no difference) is tested against an alternative hypothesis (suggesting an effect or difference). The p-value, a key metric in this process, indicates the probability of observing the results (or more extreme) assuming the null hypothesis is true. If the p-value falls below a predetermined significance level (commonly set at 0.05), the null hypothesis is rejected, suggesting that the observed difference is statistically significant. In X Bar analysis, this concept is particularly relevant when comparing sample means across different groups or over time. For instance, if you are analyzing the average production output (X Bar) from different manufacturing lines, statistical significance would help you determine if any observed differences between these lines are genuine or merely due to random variation. By calculating the standard error of the mean and using appropriate statistical tests such as ANOVA or t-tests, you can ascertain whether these differences are statistically significant. Moreover, understanding statistical significance enhances the reliability and generalizability of your findings. It ensures that conclusions drawn from sample data are not merely coincidental but reflect broader trends within the population. This is especially important in quality control scenarios where X Bar charts are used to monitor process stability and detect deviations from expected norms. In practice, achieving statistical significance involves careful consideration of sample size, data distribution, and test assumptions. Larger sample sizes generally provide more precise estimates and increase the likelihood of detecting significant effects if they exist. Additionally, ensuring that data meets necessary assumptions (such as normality for parametric tests) is vital for accurate interpretation. In summary, statistical significance is an indispensable tool for validating the reliability of X Bar analyses. By applying rigorous statistical methods and interpreting results within the context of hypothesis testing, researchers and practitioners can confidently determine whether observed patterns in sample means reflect true population characteristics or are merely artifacts of chance variation. This rigorous approach not only strengthens the validity of conclusions but also fosters informed decision-making across various fields where X Bar is utilized.
Common Applications
When delving into the concept of X Bar, it is crucial to understand its common applications across various fields. X Bar, often denoted as \(\bar{X}\), represents the mean of a sample and is a fundamental statistic in quality control and statistical process control (SPC). One of its most prevalent applications is in the manufacturing sector, where it is used to monitor and control production processes. For instance, in a factory producing widgets, X Bar charts are employed to track the average weight or dimension of the widgets over time. This helps in identifying any deviations from the desired mean, allowing for prompt corrective actions to ensure consistent product quality. In healthcare, X Bar charts are utilized in clinical settings to monitor patient outcomes and treatment efficacy. For example, tracking the average blood pressure or blood glucose levels of patients over time can help healthcare providers adjust treatment plans and improve patient care. This application is particularly useful in clinical trials where researchers need to assess the effectiveness of new treatments by comparing the mean outcomes of different groups. Another significant application of X Bar is in finance, where it is used to analyze stock prices and market trends. Financial analysts use moving averages (a form of X Bar) to smooth out price fluctuations and identify long-term trends. This helps investors make informed decisions by providing a clearer picture of market performance over time. In environmental monitoring, X Bar is used to track changes in air and water quality. By calculating the mean levels of pollutants such as particulate matter or nitrogen dioxide over a period, environmental agencies can assess the effectiveness of pollution control measures and make necessary adjustments. Furthermore, in education, X Bar can be applied to evaluate student performance. Teachers use mean scores to assess class performance on tests and assignments, helping them identify areas where students may need additional support. This data-driven approach enables more targeted teaching strategies and better student outcomes. The versatility of X Bar also extends to social sciences, where it is used in survey research to analyze public opinion and behavior. Researchers calculate the mean responses to survey questions to understand trends and patterns within a population, which can inform policy decisions and social programs. In summary, the concept of X Bar has far-reaching implications across multiple disciplines. Its ability to provide a snapshot of central tendency makes it an indispensable tool for monitoring, analyzing, and improving processes in manufacturing, healthcare, finance, environmental science, education, and social sciences. By understanding how X Bar is applied in these contexts, one can appreciate its significance in maintaining quality, making informed decisions, and driving continuous improvement.
Calculating X Bar: Step-by-Step Guide
Calculating the mean of a set of data, often denoted as \( \bar{X} \) or X Bar, is a fundamental statistical process that underpins many analytical and decision-making tasks. This step-by-step guide will walk you through the entire process, ensuring you have a comprehensive understanding of how to accurately calculate X Bar. The journey begins with **Data Collection and Preparation**, where you will learn how to gather and organize your data to ensure it is reliable and relevant. Next, we delve into the **Formula and Calculation Process**, detailing the mathematical steps required to compute X Bar. Finally, we explore **Interpreting the Results**, providing insights into how to understand and apply the calculated mean in various contexts. By following these structured steps, you will be equipped with the knowledge to confidently calculate X Bar and derive meaningful conclusions from your data. Let's start by focusing on the critical initial phase: **Data Collection and Preparation**.
Data Collection and Preparation
Data collection and preparation are crucial steps in statistical analysis, particularly when calculating X-bar, the average of a set of sample means. These processes ensure that the data used for calculations are accurate, reliable, and relevant. Here’s a detailed look at these critical phases: **Data Collection:** Data collection involves gathering information from various sources. This can be done through surveys, experiments, observational studies, or by extracting data from existing databases. The method chosen depends on the research question and the nature of the data required. For instance, if you are calculating X-bar for quality control in manufacturing, you might collect data on product dimensions or performance metrics from a production line. It is essential to ensure that the data collection process is systematic and unbiased to avoid skewing the results. Tools such as data loggers, sensors, and software applications can facilitate this process by automating data capture and reducing human error. **Data Cleaning:** Once the data are collected, they must be cleaned to remove any errors or inconsistencies. This involves checking for missing values, outliers, and incorrect entries. Missing values can be handled by imputation techniques such as mean or median imputation, while outliers may need to be verified against the original data source to determine if they are valid or errors. Data cleaning also includes ensuring that all data points are in the correct format and unit of measurement. For example, if some measurements are in inches and others in centimeters, they need to be standardized to a single unit before analysis. **Data Transformation:** After cleaning, the data may need transformation to make them suitable for analysis. This could involve normalizing the data to ensure that all variables are on the same scale, which is particularly important if you are dealing with variables of different units. Additionally, transformations like logarithmic or square root transformations might be necessary to stabilize variance or achieve normality if required by the statistical method being used. **Data Validation:** Validation is a critical step to ensure that the collected and cleaned data accurately reflect the real-world scenario being studied. This involves cross-checking the data against known benchmarks or using statistical tests to verify assumptions such as normality and homogeneity of variance. Validation helps in building confidence in the dataset and ensures that any subsequent calculations, including those for X-bar, are reliable. **Data Organization:** Finally, organizing the data into a structured format is essential for efficient analysis. This typically involves creating a dataset where each row represents an observation and each column represents a variable. Proper organization facilitates easy access and manipulation of the data during the analysis phase. In summary, thorough data collection and preparation are foundational to accurate statistical analysis. By ensuring that your data are systematically collected, cleaned, transformed, validated, and organized, you set a strong foundation for calculating X-bar and other statistical metrics with confidence. These steps not only enhance the reliability of your results but also streamline the analytical process, making it more efficient and effective.
Formula and Calculation Process
When delving into the realm of statistical analysis, particularly in the context of quality control and process monitoring, understanding the formula and calculation process for key metrics is paramount. One such crucial metric is the X-bar, or sample mean, which serves as a cornerstone in statistical process control (SPC). The X-bar calculation is a straightforward yet powerful tool that helps in assessing the central tendency of a dataset. To calculate X-bar, you start by summing all the individual data points within your sample. This involves adding up each measurement or observation to obtain the total sum. Next, you divide this total sum by the number of observations in your sample, denoted as 'n'. The formula for X-bar is thus: \( \bar{X} = \frac{\sum_{i=1}^{n} X_i}{n} \), where \( X_i \) represents each individual data point and 'n' is the sample size. This step-by-step process ensures that you are averaging out the variability within your sample to get a representative value. For instance, if you are monitoring the production line of a manufacturing plant and measuring the weights of products, calculating X-bar would give you an average weight that reflects the overall performance of the process. The significance of X-bar extends beyond mere calculation; it forms the basis for more advanced statistical analyses such as control charts. Control charts, like the X-bar chart, help in identifying whether a process is in control or if there are deviations that need attention. By plotting X-bar values over time, you can visually inspect trends and anomalies, enabling timely interventions to maintain process stability. Moreover, understanding how to calculate X-bar is essential for comparing different samples or batches. It allows you to determine if there are significant differences between them, which can be critical in quality assurance and improvement initiatives. For example, if you notice that the X-bar values for different production shifts are significantly different, it may indicate a need for adjustments in the production process or training for operators. In summary, calculating X-bar involves a simple yet robust mathematical process that underpins many statistical analyses. By accurately determining the sample mean using this formula, you gain valuable insights into your data's central tendency, enabling informed decisions that drive process improvement and quality control. This foundational knowledge is indispensable for anyone working with data in various fields, from manufacturing to healthcare, where precise monitoring and analysis are critical for success.
Interpreting the Results
When interpreting the results of calculating \( \bar{X} \) (X bar), it is crucial to understand the context and implications of this statistical measure. \( \bar{X} \) represents the mean of a sample, providing a snapshot of the central tendency of the data set. Here’s how to interpret these results effectively: 1. **Central Tendency**: The value of \( \bar{X} \) gives you the average value of your sample. This helps in understanding where the data points tend to cluster. For instance, if you are analyzing exam scores, a high \( \bar{X} \) indicates that students generally scored well. 2. **Comparison with Population Mean**: If you have access to the population mean (\( \mu \)), comparing it with \( \bar{X} \) can provide insights into how representative your sample is. Significant deviations might suggest sampling bias or that your sample does not accurately reflect the population. 3. **Standard Deviation and Variability**: While \( \bar{X} \) tells you about central tendency, it does not provide information about variability. Calculating the standard deviation (\( s \)) or range can help understand how spread out the data points are from the mean. 4. **Confidence Intervals**: Constructing confidence intervals around \( \bar{X} \) allows you to estimate the population mean with a certain level of confidence. This is particularly useful in inferential statistics where you want to make conclusions about the population based on sample data. 5. **Practical Applications**: In real-world scenarios, interpreting \( \bar{X} \) can guide decision-making. For example, in quality control, if the mean of a sample of product weights is significantly different from the target weight, it may indicate a need for adjustments in manufacturing processes. 6. **Limitations**: It’s important to recognize that \( \bar{X} \) is sensitive to outliers and skewed distributions. If your data set contains extreme values or is not normally distributed, other measures like the median might be more appropriate for understanding central tendency. 7. **Statistical Significance**: When comparing means across different samples or against a known population mean, statistical tests such as t-tests or ANOVA can help determine if any observed differences are statistically significant. By carefully interpreting these results within the context of your study or application, you can derive meaningful insights from your data and make informed decisions based on robust statistical analysis. This step is essential in ensuring that your conclusions are reliable and actionable.
Practical Uses and Real-World Examples of X Bar
The X Bar, a statistical tool derived from the X-bar chart, is a versatile and powerful instrument with a wide range of practical applications across various fields. This article delves into the real-world uses of the X Bar, highlighting its significance in three key areas: Quality Control in Manufacturing, Research and Data Analysis, and Decision Making in Business. In the realm of Quality Control in Manufacturing, the X Bar is crucial for monitoring and maintaining consistent product quality by tracking the mean of sample data over time. It helps manufacturers identify deviations from the norm, enabling swift corrective actions to ensure high standards are met. Beyond manufacturing, the X Bar plays a vital role in Research and Data Analysis by providing a clear visual representation of data trends, facilitating the identification of patterns and anomalies. Additionally, in Decision Making in Business, the X Bar aids executives in making informed decisions by offering insights into process stability and performance metrics. By understanding these applications, businesses can leverage the X Bar to enhance their operations and drive continuous improvement. Let's start by exploring how the X Bar is indispensable in Quality Control in Manufacturing.
Quality Control in Manufacturing
Quality control in manufacturing is a critical process that ensures products meet specified standards and requirements, thereby enhancing customer satisfaction, reducing costs, and improving overall efficiency. At the heart of effective quality control lies the use of statistical tools and methodologies, one of which is the X-bar chart. This tool is particularly useful for monitoring and controlling the mean of a process over time. In practical terms, X-bar charts are employed in various manufacturing settings to track the average performance of a process. For instance, in an automotive assembly line, an X-bar chart can be used to monitor the average weight of car parts produced. By plotting the mean weights over time, quality control teams can quickly identify any deviations from the target mean, indicating potential issues such as equipment malfunctions or material inconsistencies. This early detection allows for prompt corrective actions, preventing the production of defective parts and maintaining consistent product quality. Real-world examples illustrate the efficacy of X-bar charts in different industries. In the pharmaceutical sector, these charts are used to ensure that medication tablets have consistent weights and active ingredient concentrations. For example, a pharmaceutical company might use an X-bar chart to monitor the average weight of tablets produced in each batch. If the chart shows a trend of increasing or decreasing weights over time, it could signal a problem with the manufacturing process that needs immediate attention. Similarly, in food processing, X-bar charts help maintain quality standards by monitoring parameters such as fill levels in packaging or the average size of food items. A company producing canned goods might use an X-bar chart to ensure that cans are filled to the correct volume consistently. Any deviation from the target fill level could indicate issues with filling machinery or raw material supply, which can then be addressed promptly. The implementation of X-bar charts also extends to service industries where process stability is crucial. For example, in healthcare settings, these charts can be used to monitor patient wait times or average treatment durations. By analyzing these metrics over time, healthcare providers can identify inefficiencies in their processes and implement improvements to enhance patient care. In addition to its practical applications, the use of X-bar charts aligns with broader quality management frameworks such as Six Sigma and Total Quality Management (TQM). These frameworks emphasize continuous improvement and data-driven decision-making, principles that are inherently supported by the statistical insights provided by X-bar charts. Overall, the integration of X-bar charts into quality control processes enhances manufacturing efficiency by providing real-time data on process performance. This enables swift identification and resolution of issues, ensuring that products consistently meet quality standards. As a result, companies can maintain high levels of customer satisfaction while reducing waste and improving overall operational excellence.
Research and Data Analysis
Research and data analysis are the backbone of understanding and interpreting the X Bar, a statistical tool used to monitor and control processes. In the context of quality control, the X Bar chart is a powerful instrument for tracking the average performance of a process over time. Here’s how research and data analysis play a crucial role in its practical uses and real-world examples: **Research Foundation**: The development and application of X Bar charts are rooted in rigorous research. Statisticians like Walter Shewhart, who pioneered control charts, conducted extensive studies to understand process variability and how to distinguish between common cause and special cause variations. This foundational research has been built upon by numerous studies that validate the efficacy of X Bar charts in various industrial settings. **Data Collection**: Effective use of X Bar charts begins with meticulous data collection. Researchers and practitioners must gather accurate and reliable data points representing the process averages over multiple samples. This involves careful sampling strategies, precise measurement techniques, and robust data recording systems. For instance, in manufacturing, data might include dimensions of parts, while in healthcare, it could involve patient outcomes. **Data Analysis**: Once data is collected, thorough analysis is essential. This involves calculating the grand average (X Bar) and determining the upper and lower control limits (UCL and LCL). Advanced statistical software and tools facilitate this process, enabling quick identification of trends, shifts, or anomalies in the data. Researchers often employ techniques such as regression analysis or time series analysis to further understand the dynamics of the process. **Practical Applications**: The insights derived from X Bar charts have numerous practical applications across industries. For example, in automotive manufacturing, X Bar charts help monitor the average weight of car parts to ensure they meet quality standards. In healthcare, these charts can track patient recovery times post-surgery to identify any deviations from expected outcomes. In finance, they might be used to monitor transaction processing times to optimize operational efficiency. **Real-World Examples**: Real-world examples illustrate the impact of X Bar charts. For instance, a semiconductor manufacturer used X Bar charts to monitor the thickness of silicon wafers. By identifying and addressing deviations early, they significantly reduced defect rates and improved overall product quality. Similarly, a hospital used X Bar charts to track infection rates among patients undergoing surgeries, leading to the implementation of new hygiene protocols that reduced infection rates by 30%. **Continuous Improvement**: Research and data analysis also drive continuous improvement when using X Bar charts. By regularly reviewing and updating control limits based on new data, organizations can refine their processes and adapt to changing conditions. This iterative approach ensures that the X Bar chart remains a relevant and effective tool for maintaining high-quality standards. In summary, the integration of research and data analysis is crucial for the effective use of X Bar charts. From the foundational research that underpins these statistical tools to the meticulous data collection and analysis required for their application, these elements combine to provide actionable insights that drive quality improvement in various real-world contexts. By leveraging these insights, organizations can enhance their processes, improve product quality, and achieve operational excellence.
Decision Making in Business
Decision making in business is a critical process that involves evaluating options, assessing risks, and selecting the best course of action to achieve organizational goals. Effective decision making is pivotal for driving growth, innovation, and sustainability. In the context of quality control and process improvement, tools like the X Bar chart play a significant role. An X Bar chart, also known as an average chart, is used in statistical process control (SPC) to monitor the average performance of a process over time. This tool is particularly useful in manufacturing and service industries where consistency and quality are paramount. For instance, in a manufacturing plant producing automotive parts, an X Bar chart can help monitor the average dimensions of components such as engine blocks or pistons. By plotting the average measurements over time, quality control teams can quickly identify any deviations from the standard specifications. If the average measurements start to drift away from the target value, it may indicate a problem with the production process, such as a malfunctioning machine or a change in raw material quality. This early detection allows for prompt corrective actions, thereby preventing the production of defective parts and reducing waste. In real-world scenarios, companies like Toyota and General Electric have successfully integrated X Bar charts into their quality control processes. For example, Toyota uses SPC tools, including X Bar charts, to ensure that their assembly lines produce vehicles with consistent quality. By monitoring key performance indicators such as engine performance and body panel alignment, Toyota can maintain high standards of quality and reliability. Similarly, General Electric employs SPC techniques to optimize its manufacturing processes across various sectors, including aerospace and healthcare. These companies demonstrate how the practical application of X Bar charts can lead to improved product quality, reduced costs, and enhanced customer satisfaction. Moreover, the use of X Bar charts extends beyond manufacturing. Service industries such as healthcare and finance also benefit from this tool. For example, a hospital might use an X Bar chart to monitor the average response time of emergency services or the average recovery time of patients after surgery. By analyzing these averages over time, healthcare providers can identify trends and anomalies that may indicate areas for improvement in patient care. In conclusion, decision making in business is significantly enhanced by the use of analytical tools like the X Bar chart. By providing real-time data on process averages, these charts enable businesses to make informed decisions that drive quality, efficiency, and innovation. Whether in manufacturing or service sectors, the practical application of X Bar charts has proven to be a valuable asset in maintaining high standards of performance and customer satisfaction. As businesses continue to evolve and face new challenges, the integration of such statistical tools will remain essential for making data-driven decisions that foster growth and success.