What Is Advanced Cwb
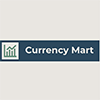
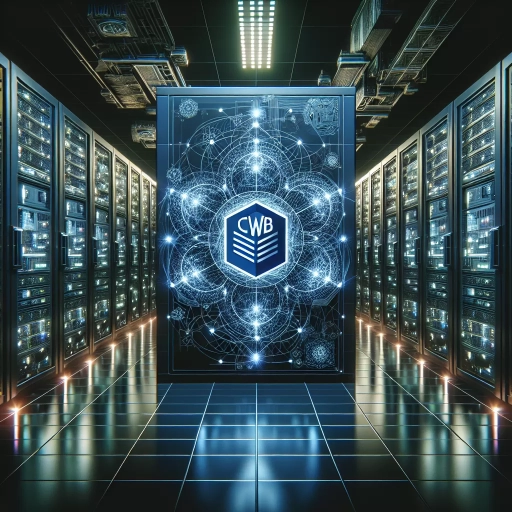
Advanced Continuous Wavelet Basis (CWB) is a sophisticated mathematical tool that has revolutionized various fields, including signal processing, image analysis, and data compression. This powerful technique leverages the principles of wavelet theory to decompose signals into different frequency components, allowing for precise analysis and manipulation. In this article, we will delve into the intricacies of Advanced CWB, starting with **Understanding the Basics of Advanced CWB**, where we will explore the fundamental concepts and mathematical underpinnings that make this technology so effective. We will then examine **Technological Advancements in CWB**, highlighting recent innovations that have enhanced its capabilities and expanded its applications. Finally, we will discuss **Practical Implementation and Future Directions**, outlining how Advanced CWB is being used in real-world scenarios and the potential avenues for future research and development. By understanding these aspects, readers will gain a comprehensive insight into the transformative potential of Advanced CWB. Let us begin by laying the groundwork with **Understanding the Basics of Advanced CWB**.
Understanding the Basics of Advanced CWB
Understanding the Basics of Advanced CWB (Certified Welding Bureau) is a multifaceted topic that requires a comprehensive approach to fully grasp its significance and applications. At its core, CWB certification is rooted in a rich historical context that has evolved over decades to ensure the highest standards of welding quality and safety. To delve into this subject, it is essential to explore three key areas: the definition and historical context of CWB, the key components and technologies involved, and the various industry applications and use cases. The definition and historical context provide a foundational understanding of how CWB has become a benchmark in the welding industry. This section will trace the origins of CWB, its development over time, and how it has adapted to technological advancements and changing industry needs. In addition to its historical roots, understanding the key components and technologies behind CWB is crucial. This includes examining the standards, testing protocols, and certification processes that ensure welds meet rigorous quality standards. Finally, exploring industry applications and use cases highlights the practical relevance of CWB across various sectors such as construction, manufacturing, and energy. This will illustrate how CWB certification impacts real-world projects and ensures safety and reliability. By examining these three aspects, readers will gain a holistic understanding of Advanced CWB, starting with its definition and historical context.
Definition and Historical Context
**Understanding the Basics of Advanced CWB: Definition and Historical Context** Advanced Continuous Welded Beam (CWB) technology represents a significant evolution in the field of structural engineering, particularly in the design and construction of steel buildings. To grasp the fundamentals of this innovative approach, it is essential to delve into its definition and historical context. **Definition:** Advanced CWB refers to a sophisticated method of connecting steel beams and columns using continuous welding techniques. Unlike traditional bolted or riveted connections, CWB involves welding the beams directly to the columns, creating a seamless and robust structural system. This method enhances the structural integrity, stability, and load-carrying capacity of the building frame. The continuous welds distribute stresses more evenly, reducing the risk of localized failures and improving overall performance under various loads, including seismic forces. **Historical Context:** The concept of continuous welding in structural engineering dates back to the mid-20th century, when advancements in welding technology made it feasible to produce high-quality welds on a large scale. However, early applications were limited due to concerns about weld quality, material properties, and the lack of standardized design guidelines. The 1960s and 1970s saw significant research and development efforts aimed at understanding the behavior of welded connections under different loading conditions. These studies led to the development of more reliable welding techniques and the establishment of design codes and standards. A pivotal moment in the history of CWB was the introduction of the American Institute of Steel Construction (AISC) specifications in the late 1970s. These guidelines provided engineers with a framework for designing and detailing continuous welded connections, ensuring consistency and safety across projects. The AISC specifications have since been updated regularly to reflect new research findings and technological advancements. The 1980s and 1990s witnessed widespread adoption of CWB in high-rise buildings and critical infrastructure projects due to its proven benefits in terms of structural performance and cost efficiency. Modern computational tools and finite element analysis have further refined the design process, allowing for more precise modeling and optimization of CWB systems. Today, advanced CWB is a cornerstone of modern steel construction, offering enhanced durability, reduced maintenance needs, and improved resistance to natural disasters such as earthquakes. As building codes continue to evolve and new materials are developed, the future of CWB looks promising, with ongoing research focused on integrating advanced materials like high-strength steels and innovative welding techniques such as robotic welding. In summary, understanding the definition and historical context of advanced CWB is crucial for appreciating its role in contemporary structural engineering. From its early beginnings to current applications, CWB has transformed the way steel structures are designed and constructed, ensuring safer, more resilient buildings that meet the demands of modern society.
Key Components and Technologies
Understanding the basics of Advanced Continuous Wavelet Transform (CWB) necessitates a deep dive into its key components and technologies. At the heart of CWB lies the wavelet transform, a mathematical tool that decomposes signals into different frequency components, allowing for the analysis of both time and frequency domains simultaneously. This is achieved through the use of wavelet functions, which are scaled and shifted versions of a mother wavelet. The choice of the mother wavelet is crucial as it influences the resolution and accuracy of the analysis. One of the fundamental technologies supporting CWB is the Fast Wavelet Transform (FWT), an efficient algorithm that reduces computational complexity from \(O(n^2)\) to \(O(n)\), making real-time processing feasible. The FWT leverages a pyramidal algorithmic structure, where each level of decomposition involves filtering and downsampling, resulting in a hierarchical representation of the signal. Another critical component is the concept of multi-resolution analysis (MRA), which provides a framework for decomposing signals into various scales or resolutions. MRA ensures that CWB can capture both low-frequency trends and high-frequency details within a signal, making it particularly useful in applications such as image compression, noise reduction, and feature extraction. Advanced CWB also incorporates sophisticated filtering techniques, including orthogonal and bi-orthogonal filters. Orthogonal filters ensure that the decomposed sub-bands are uncorrelated, enhancing the efficiency of signal representation. Bi-orthogonal filters, on the other hand, offer greater flexibility in design, allowing for better adaptation to specific signal characteristics. Furthermore, modern implementations of CWB often integrate machine learning algorithms to enhance signal processing capabilities. For instance, neural networks can be trained to optimize wavelet coefficients for specific tasks such as denoising or classification. This synergy between wavelet analysis and machine learning enables more robust and adaptive signal processing systems. In addition to these core technologies, advanced CWB benefits from advancements in computational hardware. The advent of parallel processing architectures like GPUs and FPGAs has significantly accelerated wavelet transform computations, enabling real-time analysis of large datasets. This is particularly important in fields such as medical imaging, where timely diagnostics are critical. Lastly, software frameworks and libraries play a vital role in facilitating the implementation of CWB. Tools like MATLAB, Python libraries such as PyWavelets, and open-source platforms provide extensive support for wavelet analysis, including pre-built functions for various wavelet transforms and visualization tools. In summary, understanding the basics of advanced CWB requires familiarity with its key components—wavelet functions, Fast Wavelet Transform, multi-resolution analysis, filtering techniques—and how these are integrated with machine learning and computational advancements. By grasping these technologies, practitioners can leverage CWB to extract valuable insights from complex signals across diverse applications.
Industry Applications and Use Cases
Advanced Continuous Waveform (CWB) technology has far-reaching implications across various industries, transforming the way businesses operate and innovate. In the **telecommunications sector**, CWB is crucial for enhancing network reliability and efficiency. By enabling the transmission of high-speed data over long distances without signal degradation, CWB supports the rollout of 5G networks, ensuring seamless connectivity and low latency. This is particularly beneficial for applications such as real-time video streaming, IoT devices, and mission-critical communications. In **healthcare**, CWB plays a pivotal role in medical imaging technologies like MRI and PET scans. The precise control over waveforms allows for clearer, more detailed images, which are essential for accurate diagnoses and treatments. Additionally, CWB is used in medical devices such as pacemakers and implantable cardioverter-defibrillators (ICDs), where stable and reliable waveforms are critical for maintaining patient health. The **aerospace industry** also leverages CWB for navigation and communication systems. High-stability oscillators based on CWB ensure accurate positioning and timing, which are vital for satellite communications, GPS systems, and radar technologies. This precision is indispensable for safe and efficient air travel as well as space exploration. In **industrial automation**, CWB is integral to the operation of high-precision machinery. It enables the synchronization of complex manufacturing processes, ensuring that production lines run smoothly and efficiently. This is particularly important in industries such as automotive manufacturing, where precision timing is crucial for assembly line operations. Moreover, **scientific research** benefits significantly from CWB. In particle accelerators and spectroscopy, stable waveforms are necessary for precise measurements and experiments. For instance, in quantum computing, CWB helps maintain the coherence of quantum states, which is essential for advancing quantum technology. Lastly, **energy and utilities** sectors utilize CWB in smart grid systems to manage power distribution efficiently. By providing stable frequency references, CWB helps in synchronizing power generation and transmission, reducing the risk of grid failures and improving overall energy efficiency. In summary, Advanced CWB's versatility and reliability make it a cornerstone technology across diverse industries, driving innovation, efficiency, and precision in various applications. Understanding the basics of Advanced CWB is essential for harnessing its full potential and staying ahead in an increasingly technologically driven world.
Technological Advancements in CWB
Technological advancements in Continuous Welding (CWB) have revolutionized the manufacturing industry, offering unprecedented efficiency, precision, and cost-effectiveness. At the forefront of these developments are recent innovations and breakthroughs that have significantly enhanced the welding process. These advancements include the introduction of advanced robotic systems, high-speed welding techniques, and improved material sciences. Additionally, the integration of Artificial Intelligence (AI) and Machine Learning (ML) has enabled real-time monitoring and optimization of the welding process, reducing errors and improving quality. Furthermore, the seamless integration of CWB with other technologies such as 3D printing and Internet of Things (IoT) has opened up new possibilities for complex manufacturing tasks. As we delve into the specifics of these technological strides, it becomes clear that recent innovations and breakthroughs are the foundation upon which these advancements are built, setting the stage for a transformative future in CWB. Let us first explore the recent innovations and breakthroughs that have spearheaded this technological evolution.
Recent Innovations and Breakthroughs
Recent innovations and breakthroughs in the field of Continuous Wave Brazing (CWB) have significantly enhanced its efficiency, precision, and versatility. One of the most notable advancements is the integration of advanced robotics and automation. Modern robotic systems equipped with sophisticated sensors and AI algorithms can now perform complex brazing tasks with unprecedented accuracy and speed. These robots can adapt to various workpiece geometries and materials, ensuring consistent quality and reducing human error. Another critical breakthrough is the development of novel filler materials and fluxes. New generations of filler alloys have been engineered to offer improved strength, corrosion resistance, and thermal conductivity. Additionally, advanced fluxes with enhanced wetting properties and lower melting points have been introduced, facilitating smoother brazing processes and reducing the risk of defects. These advancements have expanded the range of materials that can be brazed, including high-strength alloys and ceramics, which are increasingly used in aerospace, automotive, and medical applications. The advent of laser-based CWB systems has also revolutionized the field. Laser brazing offers superior control over the heat input, allowing for precise temperature management and minimal thermal distortion. This technique is particularly beneficial for joining delicate or sensitive components, such as those found in electronic devices and medical implants. Furthermore, laser brazing can achieve higher joint strengths compared to traditional methods, making it an attractive option for critical applications where reliability is paramount. In addition to these technological improvements, significant strides have been made in process monitoring and control. Advanced thermal imaging and spectroscopy techniques enable real-time monitoring of the brazing process, allowing for immediate adjustments to ensure optimal results. This real-time feedback loop enhances process reliability and reduces the need for post-brazing inspection and rework. Moreover, the integration of Industry 4.0 technologies, such as IoT sensors and data analytics, has transformed CWB into a more intelligent and connected process. Real-time data from sensors can be analyzed to predict maintenance needs, detect anomalies, and optimize production workflows. This digital transformation not only improves efficiency but also enhances safety by identifying potential hazards before they occur. Lastly, environmental considerations have driven innovations in eco-friendly CWB practices. New fluxes and filler materials are being developed with reduced toxicity and lower environmental impact. Additionally, energy-efficient brazing techniques and equipment are being introduced to minimize energy consumption and reduce greenhouse gas emissions. These sustainable practices align with global efforts to reduce industrial carbon footprints while maintaining high-quality production standards. In summary, recent innovations in CWB have transformed the field by introducing advanced robotics, novel materials, laser-based techniques, enhanced process monitoring, Industry 4.0 integration, and eco-friendly practices. These breakthroughs collectively elevate the precision, efficiency, and sustainability of CWB, making it a cornerstone of modern manufacturing across diverse industries.
Role of AI and Machine Learning
In the realm of Technological Advancements in Continuous Waveform (CWB) systems, the integration of Artificial Intelligence (AI) and Machine Learning (ML) has revolutionized the landscape by enhancing efficiency, accuracy, and decision-making capabilities. AI and ML technologies play a pivotal role in optimizing CWB operations by automating complex processes, improving predictive maintenance, and enhancing data analysis. At the core of this transformation is the ability of AI to process vast amounts of data generated by CWB systems. Machine Learning algorithms can analyze these datasets to identify patterns, anomalies, and trends that might be overlooked by human operators. For instance, predictive maintenance models can forecast equipment failures, allowing for proactive repairs and minimizing downtime. This not only reduces operational costs but also ensures continuous production without interruptions. Moreover, AI-driven systems can optimize CWB parameters in real-time, adjusting variables such as frequency, amplitude, and waveform shape to achieve optimal performance. This dynamic adjustment is crucial in maintaining the quality of the waveform, which is essential for various applications including material processing, medical treatments, and communication systems. Another significant contribution of AI and ML is in the area of quality control. Advanced algorithms can monitor the output of CWB systems continuously, detecting any deviations from the desired specifications. This real-time monitoring enables immediate corrective actions, ensuring that the final product meets stringent quality standards. Furthermore, AI can enhance user experience through intuitive interfaces and automated workflows. For example, machine learning-based interfaces can learn user preferences and adapt to their needs over time, streamlining the operation process and reducing the learning curve for new operators. In addition to these operational benefits, AI and ML also contribute to innovation in CWB technology. By analyzing historical data and current trends, these technologies can suggest new waveform designs or operating conditions that could lead to breakthroughs in various fields. For instance, in medical applications, AI could help design waveforms that are more effective for specific treatments or patient conditions. Overall, the integration of AI and ML into CWB systems represents a significant leap forward in technological advancements. These technologies not only improve the efficiency and reliability of CWB operations but also open up new avenues for innovation and improvement, making them indispensable components of advanced CWB systems. As these technologies continue to evolve, we can expect even more sophisticated applications of AI and ML in the future, further enhancing the capabilities and impact of CWB technology.
Integration with Other Technologies
Integration with other technologies is a pivotal aspect of advancing Continuous Welding and Brazing (CWB) processes. As CWB evolves, its seamless integration with various cutting-edge technologies enhances efficiency, precision, and overall productivity. One key area of integration is with robotics and automation systems. By combining CWB with robotic arms and automated control systems, manufacturers can achieve higher levels of consistency and speed in welding and brazing operations. These systems can be programmed to perform complex tasks with minimal human intervention, reducing the risk of human error and increasing throughput. Another significant integration is with advanced sensing and monitoring technologies. The use of sensors and real-time monitoring systems allows for continuous quality control during the CWB process. These sensors can detect temperature variations, weld pool dynamics, and other critical parameters in real-time, enabling immediate adjustments to ensure optimal weld quality. This real-time feedback loop enhances the reliability and repeatability of the welding process. Furthermore, the integration of CWB with data analytics and artificial intelligence (AI) is transforming the industry. AI algorithms can analyze vast amounts of data generated during the welding process to predict potential defects, optimize welding parameters, and improve overall process efficiency. Machine learning models can be trained on historical data to identify patterns that may indicate impending issues or areas for improvement, allowing for proactive maintenance and quality assurance. Additionally, the incorporation of additive manufacturing (3D printing) into CWB workflows offers new possibilities for complex part fabrication. By integrating 3D printing with CWB, manufacturers can create intricate geometries that would be challenging or impossible to produce using traditional methods. This hybrid approach enables the creation of parts with unique properties and structures that can enhance performance in various applications. The integration of CWB with Industry 4.0 technologies such as the Internet of Things (IoT) and cloud computing also plays a crucial role. IoT devices connected to the welding equipment can transmit data to cloud-based platforms for centralized monitoring and analysis. This enables remote supervision, predictive maintenance, and collaborative workflows across different locations, fostering a more connected and efficient manufacturing environment. In conclusion, the integration of CWB with other advanced technologies is driving significant advancements in the field. By leveraging robotics, sensing technologies, AI, additive manufacturing, and Industry 4.0 solutions, manufacturers can achieve higher levels of precision, efficiency, and innovation in their welding and brazing operations. These integrations not only improve the quality and reliability of the final products but also pave the way for future technological breakthroughs in CWB.
Practical Implementation and Future Directions
The practical implementation and future directions of advanced Continuous Welding and Brazing (CWB) technologies are pivotal in driving innovation and efficiency across various industries. To fully leverage these advancements, it is crucial to understand the steps involved in implementing advanced CWB, the challenges that may arise, and the potential solutions to overcome them. This article delves into these critical aspects, providing a comprehensive guide for practitioners and stakeholders. First, we will outline the steps for implementing advanced CWB, detailing the necessary preparations, equipment upgrades, and training requirements. Next, we will address the challenges that often hinder successful implementation, such as material compatibility issues and process control complexities, along with practical solutions to mitigate these problems. Finally, we will explore predictions for future development in CWB, including emerging technologies and trends that promise to further enhance productivity and quality. By navigating these key areas, readers will gain a holistic understanding of how to effectively integrate advanced CWB into their operations, setting the stage for improved performance and competitiveness. Let us begin by examining the steps for implementing advanced CWB.
Steps for Implementing Advanced CWB
Implementing Advanced Continuous Wavelet Transform (CWB) involves several meticulous steps that ensure the effective integration and utilization of this sophisticated signal processing technique. First, **understand the fundamentals**: Familiarize yourself with the theoretical underpinnings of wavelet analysis, including the types of wavelets, their properties, and how they decompose signals into different frequency components. This foundational knowledge is crucial for selecting the appropriate wavelet basis and parameters for your specific application. Next, **choose the right wavelet**: Select a wavelet that best suits your data characteristics. For instance, Haar wavelets are simple and often used for basic applications, while more complex wavelets like Daubechies or Coiflets might be necessary for detailed signal analysis. Consider factors such as the signal's frequency content, noise levels, and the desired level of detail. **Preprocess the data**: Ensure that your dataset is clean and prepared for analysis. This may involve filtering out noise, normalizing the data, and handling any missing values. Advanced CWB can be sensitive to data quality, so thorough preprocessing is essential. **Implement the wavelet transform**: Use software tools or libraries such as MATLAB, Python's PyWavelets, or R's wavelets package to perform the wavelet transform. These tools offer various functions to compute the discrete wavelet transform (DWT) and continuous wavelet transform (CWT), allowing you to decompose your signal into different scales and resolutions. **Analyze and interpret results**: Once you have transformed your data, analyze the resulting coefficients. The approximation coefficients provide a coarse representation of the signal, while detail coefficients capture finer details. Visualize these coefficients using plots or heatmaps to gain insights into the signal's structure and patterns. **Optimize parameters**: Fine-tune parameters such as the number of decomposition levels, thresholding methods for denoising, and the choice of mother wavelet based on your analysis goals. This iterative process ensures that you extract meaningful information from your data. **Integrate with other techniques**: Combine CWB with other signal processing methods or machine learning algorithms to enhance analysis capabilities. For example, using wavelet-based features as inputs to neural networks can improve pattern recognition tasks. **Validate and test**: Validate your implementation by comparing results with known benchmarks or through cross-validation techniques. Testing on different datasets helps ensure that your approach is robust and generalizable. Finally, **document and share**: Document your methodology thoroughly, including the rationale behind your choices and any challenges encountered. Sharing your findings and code can facilitate collaboration and contribute to the broader community's understanding of advanced CWB applications. By following these steps systematically, you can effectively implement advanced CWB in various fields such as biomedical signal processing, image analysis, and financial data analysis, unlocking deeper insights into complex data sets. This structured approach not only ensures practical implementation but also paves the way for future directions in research and application development.
Challenges and Solutions
In the realm of Advanced Cooling Water Systems (CWB), several challenges arise that necessitate innovative solutions to ensure efficient and sustainable operations. One of the primary challenges is the issue of scaling and fouling, which can significantly reduce the heat transfer efficiency and increase maintenance costs. To address this, advanced water treatment technologies such as nanofiltration and reverse osmosis can be employed to remove impurities and reduce the likelihood of scaling. Additionally, the use of corrosion inhibitors and biocides can help mitigate the impact of microbial growth and metal corrosion. Another critical challenge is environmental sustainability. Traditional cooling systems often rely on large volumes of water, which can strain local water resources and harm aquatic ecosystems. Advanced CWB systems can incorporate air-cooled or hybrid cooling technologies that reduce water consumption. Furthermore, implementing closed-loop systems where water is continuously recycled and reused minimizes the environmental footprint. Energy efficiency is another significant concern. Advanced CWB systems can leverage advanced materials and designs to enhance heat transfer rates, thereby reducing the energy required for cooling. For instance, the use of microchannel heat exchangers or enhanced surface geometries can improve thermal performance while lowering energy consumption. From a practical implementation perspective, integrating these solutions requires careful planning and monitoring. Real-time data analytics and IoT sensors can be used to monitor system performance, detect anomalies early, and optimize cooling processes dynamically. Regular maintenance schedules should include inspections for signs of scaling or fouling, and predictive maintenance strategies can help prevent downtime. Looking towards future directions, advancements in materials science and digital technologies will play a pivotal role in enhancing CWB systems. Research into new materials with improved thermal conductivity and resistance to corrosion could lead to more efficient heat exchangers. Additionally, the integration of artificial intelligence and machine learning algorithms can optimize cooling operations based on real-time data, predicting and adapting to changes in load and environmental conditions. In conclusion, addressing the challenges in Advanced CWB systems through innovative solutions not only improves operational efficiency but also contributes to environmental sustainability and reduced energy consumption. As technology continues to evolve, the future of CWB will be characterized by smarter, more efficient, and environmentally friendly cooling solutions that meet the demands of a rapidly changing world.
Predictions for Future Development
As we delve into the future development of advanced Continuous Wave (CW) radar technology, several key trends and predictions emerge that will shape its practical implementation and future directions. One of the most significant advancements will be the integration of artificial intelligence (AI) and machine learning (ML) algorithms to enhance signal processing and target detection. AI-driven systems will enable real-time adaptive filtering, improving the accuracy and speed of target identification in complex environments. This integration will also facilitate autonomous operation, allowing CW radar systems to self-optimize their parameters for optimal performance under varying conditions. Another critical area of development will be the miniaturization and cost reduction of CW radar components. Advances in semiconductor technology and nanotechnology will lead to more compact, lightweight, and affordable devices. This miniaturization will expand the applicability of CW radar beyond traditional military and surveillance uses, making it viable for consumer electronics, automotive safety systems, and even wearable technology. For instance, compact CW radar modules could be integrated into smart home devices for motion detection or into vehicles for advanced driver-assistance systems (ADAS). The proliferation of the Internet of Things (IoT) will also play a crucial role in the future of CW radar. As IoT devices become more ubiquitous, there will be a growing need for robust and reliable sensing technologies that can operate within dense networks. CW radar, with its ability to penetrate obstacles and provide continuous monitoring, will be particularly well-suited for IoT applications such as smart cities, industrial automation, and healthcare monitoring. Furthermore, advancements in materials science and antenna design will significantly improve the performance of CW radar systems. New materials with enhanced electromagnetic properties will allow for the creation of more efficient antennas that can operate over wider frequency bands. This will enable CW radar to detect targets at greater distances and with higher resolution, making it an indispensable tool for long-range surveillance and early warning systems. In addition to these technological advancements, regulatory frameworks and standards will evolve to accommodate the increasing use of CW radar in various sectors. Governments and international bodies will need to establish clear guidelines for the safe and responsible deployment of CW radar technology, ensuring that it does not interfere with other wireless communication systems while maximizing its benefits. Finally, the convergence of CW radar with other sensing technologies such as lidar and optical sensors will create hybrid systems that offer unparalleled sensing capabilities. These hybrid systems will leverage the strengths of each technology to provide comprehensive situational awareness in diverse environments, from urban surveillance to environmental monitoring. In conclusion, the future development of advanced CW radar technology is poised to be driven by significant technological, regulatory, and market trends. As AI, miniaturization, IoT integration, advanced materials, regulatory frameworks, and hybrid sensing technologies continue to evolve, CW radar will become an even more indispensable tool across various industries, offering enhanced performance, versatility, and practicality. These advancements will not only expand the scope of applications but also ensure that CW radar remains at the forefront of sensing and detection technologies for years to come.