What Does Ml Mean In Text
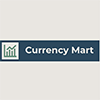
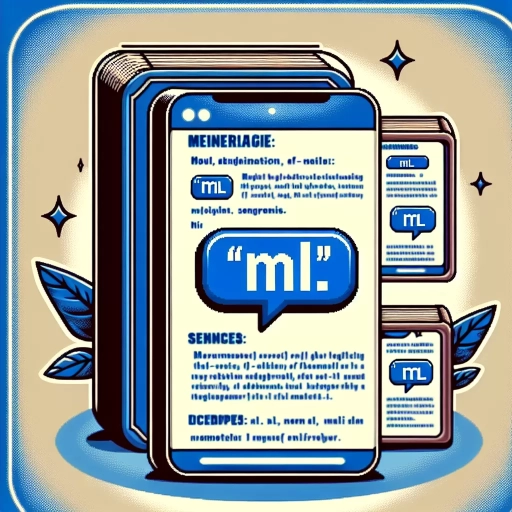
In the ever-evolving landscape of digital communication, acronyms and abbreviations have become an integral part of our daily interactions. Among these, "ML" stands out as a term that can be both ubiquitous and enigmatic, depending on the context. But what does "ML" mean in text? This question is more than just a curiosity; it delves into the heart of how we communicate, the history behind our language, and the practical applications that shape our interactions. To fully grasp the significance of "ML," it is essential to understand its context, explore its historical and evolutionary aspects, and examine its practical examples and real-world applications. By doing so, we can uncover the multifaceted nature of this term and its impact on modern communication. Let's begin by understanding the context of "ML" in text, which sets the stage for a deeper exploration into its broader implications.
Understanding the Context of "ML" in Text
In the vast and dynamic landscape of modern communication, abbreviations and acronyms have become integral to our daily interactions. Among these, "ML" stands out as a term that can be interpreted in multiple ways, depending on the context in which it is used. This ambiguity necessitates a deeper understanding of its various meanings to avoid confusion and ensure effective communication. This article delves into the multifaceted nature of "ML," exploring its common abbreviations and acronyms, its technical and scientific usage, and its informal and social media contexts. By examining these different dimensions, we can better grasp the nuances of "ML" and its significance in various settings. Whether it refers to machine learning in technical discourse, milliliters in scientific notation, or other meanings in social media and informal conversations, understanding the context of "ML" in text is crucial for clear and accurate communication. This article aims to provide a comprehensive overview of these contexts, ultimately enhancing our ability to navigate the complexities of "ML" in text.
Common Abbreviations and Acronyms
In the vast and ever-evolving landscape of digital communication, understanding common abbreviations and acronyms is crucial for effective and efficient interaction. These shorthand terms have become integral to our daily online interactions, whether through text messages, social media, or professional emails. For instance, "ML" itself can have multiple meanings depending on the context: it could stand for "Machine Learning" in a technical or academic setting, "Milk" in a culinary context, or even "Mile" in discussions about distance. However, in the realm of texting and informal communication, "ML" often stands for "Mobile Legends," a popular multiplayer online battle arena game. Other common abbreviations include "ASAP" (As Soon As Possible), "FYI" (For Your Information), and "BTW" (By The Way), which are frequently used to convey urgency, provide additional information, or add a casual tone to messages. Acronyms like "LOL" (Laugh Out Loud) and "OMG" (Oh My God) are staples in casual conversations, expressing emotions quickly and succinctly. In professional settings, acronyms such as "CEO" (Chief Executive Officer) and "HR" (Human Resources) are used to refer to job titles and departments without unnecessary elaboration. Understanding these abbreviations not only enhances communication clarity but also helps in avoiding misunderstandings. For example, if someone texts "I'll be there ASAP," it's clear they intend to arrive as quickly as possible, whereas "I'll be there BTW" might suggest they are coming but with no urgency implied. Similarly, in a work email, "Please refer this to HR" is much more concise than writing out the full department name. Moreover, being familiar with these abbreviations can make one appear more tech-savvy and adept at navigating modern communication channels. It also reflects an ability to adapt to evolving language trends, which is increasingly important in both personal and professional spheres. As technology continues to advance and new terms emerge, staying updated on common abbreviations and acronyms ensures that one remains connected and communicative in an ever-changing digital world. In summary, mastering common abbreviations and acronyms is essential for clear, efficient, and engaging communication in today's digital age. Whether it's deciphering the meaning of "ML" or using "FYI" to provide context, these shorthand terms play a significant role in how we interact online and offline. By understanding these terms, individuals can enhance their communication skills, avoid confusion, and stay relevant in a world where brevity and clarity are paramount.
Technical and Scientific Usage
In the realm of technical and scientific usage, "ML" often stands for Machine Learning, a subset of artificial intelligence that involves the development of algorithms and statistical models to enable machines to perform tasks without explicit instructions. This field has revolutionized various sectors by automating complex processes, enhancing predictive analytics, and improving decision-making capabilities. In scientific contexts, ML is frequently employed in data-intensive research such as genomics, climate modeling, and materials science. For instance, in genomics, ML algorithms can analyze vast amounts of genomic data to identify patterns and predict genetic predispositions to diseases. Similarly, in climate modeling, ML helps in predicting weather patterns and understanding the impacts of climate change by analyzing historical climate data and current trends. The technical applications of ML are equally profound. In software development, ML is used to create intelligent systems that can learn from user interactions, such as chatbots and recommendation engines. These systems continuously improve their performance based on the data they receive, making them more efficient and user-friendly over time. Additionally, ML plays a crucial role in cybersecurity by detecting anomalies in network traffic and identifying potential threats before they cause harm. The automotive industry also leverages ML for autonomous vehicles, enabling cars to recognize objects, navigate roads, and make decisions in real-time. Moreover, the integration of ML with other technologies like the Internet of Things (IoT) has opened up new avenues for innovation. For example, smart home devices use ML to learn occupants' preferences and adjust settings accordingly, while industrial IoT applications utilize ML for predictive maintenance, reducing downtime and increasing overall efficiency. Understanding the context of "ML" in text is essential because it allows readers to grasp the depth and breadth of its applications. Whether it's a scientific paper discussing the latest advancements in ML algorithms or a technical report detailing the implementation of ML in a specific industry, recognizing what "ML" stands for is crucial for comprehension. This understanding not only enhances the reader's knowledge but also facilitates better communication among professionals working in these fields. As technology continues to evolve, the significance of ML will only grow, making it imperative to be well-versed in its meaning and applications.
Informal and Social Media Contexts
In the realm of informal and social media contexts, the acronym "ML" can take on various meanings, reflecting the dynamic and often personalized nature of online communication. Unlike formal settings where acronyms are strictly defined, social media platforms like Twitter, Instagram, and text messaging apps allow for a more fluid interpretation of abbreviations. Here, "ML" might stand for "Much Love," a casual way to express affection or appreciation among friends. For instance, someone might end a message with "ML" to convey warmth and closeness. However, it's also possible that "ML" could be used in gaming communities to mean "Machine Learning," especially in discussions about AI-driven game mechanics or predictive analytics. This dual usage highlights the importance of understanding the specific context in which the term is used. Moreover, the evolution of language on social media is rapid and influenced by cultural trends and memes. For example, if a popular influencer or celebrity starts using "ML" in a particular way, it could quickly spread and become a temporary norm within their follower base. This ephemeral nature of online language underscores the need for continuous learning and adaptation when interpreting acronyms like "ML." Additionally, regional differences play a significant role in how acronyms are interpreted. In some regions or communities, "ML" might have a completely different meaning than in others. For instance, in certain parts of Asia, "ML" could refer to "Mobile Legends," a popular mobile game, while in other areas it might mean something entirely different. This regional variability further complicates but also enriches the landscape of informal communication. Understanding these nuances is crucial for effective communication in digital spaces. It requires a blend of cultural awareness, context sensitivity, and an openness to learning new expressions as they emerge. By recognizing that "ML" can mean different things depending on the platform, community, and cultural background, individuals can navigate online interactions more smoothly and avoid misunderstandings. This adaptability not only enhances personal connections but also fosters a more inclusive and engaging online environment where diverse voices can be heard and understood. In summary, the meaning of "ML" in informal and social media contexts is multifaceted and context-dependent. It can range from expressions of affection to references to technology or gaming, influenced by regional and cultural factors. Embracing this complexity allows for more meaningful and effective communication in the ever-evolving world of digital interactions.
Historical and Evolutionary Aspects of "ML"
Machine Learning (ML), a subset of artificial intelligence, has evolved significantly over the years, transforming from a theoretical concept to a ubiquitous technology that permeates various aspects of modern life. This article delves into the historical and evolutionary aspects of ML, providing a comprehensive overview that spans its origins and early usage, its adaptation in different fields, and its modern applications and trends. By exploring the **Origins and Early Usage** of ML, we uncover the foundational principles and pioneers who laid the groundwork for this technology. The **Adaptation in Different Fields** section highlights how ML has been integrated into diverse domains such as healthcare, finance, and transportation, revolutionizing processes and outcomes. Finally, **Modern Applications and Trends** reveal the cutting-edge advancements and future directions of ML, including deep learning and ethical considerations. Understanding these historical, adaptive, and contemporary facets of ML is crucial for grasping its current context and potential future impact. This journey through the evolution of ML sets the stage for **Understanding the Context of "ML" in Text**, enabling readers to appreciate the depth and breadth of this transformative technology.
Origins and Early Usage
The origins and early usage of Machine Learning (ML) are deeply rooted in the intersection of computer science, statistics, and cognitive psychology. The term "Machine Learning" was first coined by Arthur Samuel in 1959, who defined it as a field of study that gives computers the ability to learn without being explicitly programmed. However, the conceptual foundations of ML date back to the mid-20th century when Alan Turing proposed the Turing Test in 1950, which questioned whether machines could exhibit intelligent behavior indistinguishable from that of humans. In the 1950s and 1960s, pioneers like Frank Rosenblatt developed the perceptron, an early type of neural network that laid the groundwork for modern neural networks. The perceptron was designed to recognize patterns and learn from data, marking one of the first practical attempts at creating a machine that could learn. During this period, researchers also began exploring decision trees and rule-based systems, which are still fundamental components of ML today. The 1980s saw a significant resurgence in ML research with the introduction of backpropagation by David Rumelhart, Geoffrey Hinton, and Ronald Williams. This algorithm enabled efficient training of multi-layer neural networks, paving the way for more complex and powerful models. The rise of computational power and data storage capabilities further accelerated ML development, allowing for larger datasets and more sophisticated algorithms. Early applications of ML were diverse and included areas such as image recognition, natural language processing, and game playing. For instance, IBM's Deep Blue chess computer, which defeated Garry Kasparov in 1997, utilized ML techniques to analyze vast numbers of chess positions and predict optimal moves. Similarly, speech recognition systems began to emerge, leveraging ML to improve their accuracy over time. Throughout its evolution, ML has been influenced by various disciplines. Statistical learning theory, introduced by Vladimir Vapnik and Alexey Chervonenkis in the 1960s, provided a rigorous framework for understanding the capacity of learning algorithms. Cognitive science contributed insights into human learning processes, which were then applied to machine learning models. The early usage of ML was often limited by computational resources and data availability. However, with the advent of big data and cloud computing in the 21st century, ML has become a ubiquitous technology across industries. From recommendation systems in e-commerce to predictive analytics in healthcare, ML has transformed how we interact with technology and make decisions. In summary, the origins and early usage of Machine Learning reflect a rich tapestry of interdisciplinary research and innovation. From its conceptual beginnings with Turing to its practical applications today, ML has evolved significantly, driven by advances in algorithms, computing power, and data availability. This historical context underscores the dynamic nature of ML and its potential for continued growth and impact in the future.
Adaptation in Different Fields
Adaptation is a fundamental concept that transcends various fields, from biology and psychology to technology and machine learning. In the context of machine learning (ML), adaptation refers to the ability of algorithms to adjust their performance based on new data or changing environments. This concept is deeply rooted in historical and evolutionary aspects, where organisms have evolved over time to better fit their environments. In biology, adaptation is the process by which organisms become better suited to their environments, often leading to increased survival and reproductive success. This natural selection drives evolutionary changes, such as the development of antibiotic resistance in bacteria or the adaptation of polar bears to Arctic climates. Similarly, in ML, algorithms like neural networks adapt through training data, adjusting weights and biases to improve their predictive accuracy. In psychology, adaptation is crucial for human learning and development. Cognitive adaptation theory suggests that individuals adapt their thinking and behavior in response to new information or experiences. This adaptive process is essential for problem-solving and decision-making. In ML, this parallels the way models adapt to new patterns in data, enabling them to make more accurate predictions over time. Technologically, adaptation is a key feature in many modern systems. For instance, recommendation systems adapt user preferences based on past interactions, while autonomous vehicles adapt their navigation strategies based on real-time traffic data. These systems leverage ML algorithms that continuously learn from feedback and adjust their outputs accordingly. Historically, the concept of adaptation in ML has evolved significantly. Early ML models were static and did not adapt well to changing conditions. However, with advancements in deep learning and reinforcement learning, modern ML models can now adapt dynamically. For example, reinforcement learning algorithms can adjust their strategies based on rewards or penalties received from the environment, much like how organisms adapt through natural selection. The evolutionary aspect of ML adaptation is also evident in the development of meta-learning algorithms. These algorithms learn how to learn from different tasks and environments, enabling them to adapt quickly to new situations. This meta-adaptation mirrors the evolutionary process where species develop generalizable traits that enhance their adaptability across various environments. In summary, adaptation in different fields—biology, psychology, technology, and machine learning—shares a common thread: the ability to adjust and improve performance in response to changing conditions. Understanding these historical and evolutionary aspects of adaptation provides valuable insights into how ML models can be designed to be more resilient and effective in real-world applications. By leveraging these principles, ML can continue to evolve and improve, much like how life on Earth has adapted over millions of years.
Modern Applications and Trends
In the realm of Machine Learning (ML), modern applications and trends have transformed the landscape, building upon the historical and evolutionary foundations laid over the decades. Today, ML is ubiquitous, permeating various sectors such as healthcare, finance, transportation, and consumer technology. One of the most significant trends is the rise of Deep Learning, a subset of ML that leverages neural networks to achieve unprecedented accuracy in tasks like image recognition, natural language processing, and speech recognition. This has led to breakthroughs in areas like medical imaging analysis, where AI can detect diseases more accurately and earlier than human clinicians. In finance, ML algorithms are used for risk assessment, fraud detection, and portfolio optimization, enhancing decision-making processes with data-driven insights. Another trend is the increasing adoption of Reinforcement Learning, which enables machines to learn from interactions with their environment through trial and error. This has been instrumental in developing autonomous vehicles and robots that can adapt to new situations without explicit programming. The integration of ML with Internet of Things (IoT) devices has also become a focal point, enabling smart homes and cities to optimize energy consumption, traffic flow, and public services based on real-time data. The surge in Big Data and the availability of computational power have further accelerated ML advancements. Cloud computing platforms now offer scalable infrastructure for training complex models, while open-source frameworks like TensorFlow and PyTorch democratize access to advanced ML tools. Additionally, Explainable AI (XAI) has emerged as a critical area of research, aiming to provide transparency into how ML models make decisions—a necessity for ethical AI deployment. Moreover, ethical considerations are becoming more prominent as ML's impact on society grows. There is a growing emphasis on fairness, accountability, and transparency in AI systems to mitigate biases and ensure equitable outcomes. Regulatory bodies are also stepping in to establish guidelines for the responsible use of AI technologies. Lastly, the convergence of ML with other technologies such as blockchain and edge computing is opening new avenues for secure data processing and real-time analytics. Edge AI, for instance, allows for faster processing by bringing computation closer to the source of data generation, which is crucial for applications requiring low latency like autonomous driving or smart manufacturing. In summary, modern applications and trends in ML reflect a dynamic field that continues to evolve rapidly. From enhancing healthcare diagnostics to driving autonomous vehicles, ML is revolutionizing industries while raising important questions about ethics and responsibility. As technology advances further, it is clear that ML will remain a cornerstone of innovation across diverse sectors.
Practical Examples and Real-World Applications
In today's rapidly evolving technological landscape, machine learning (ML) has emerged as a pivotal force, transforming various aspects of our lives. This article delves into the practical examples and real-world applications of ML, highlighting its pervasive impact across different domains. We will explore how ML is revolutionizing technology, enabling innovations that were previously unimaginable. Additionally, we will examine the role of ML in everyday communication, where it enhances user experiences and streamlines interactions. Furthermore, we will discuss the cross-disciplinary uses of ML, demonstrating its versatility and potential to solve complex problems in diverse fields. By understanding these practical applications, readers will gain a deeper insight into the context of ML in text, appreciating its significance and the ways in which it is shaping our world. This comprehensive overview aims to provide a clear and engaging perspective on the multifaceted nature of ML, making it accessible and relevant to a broad audience. Transitioning seamlessly into the subsequent sections, this introduction sets the stage for a detailed exploration of ML's transformative power.
Machine Learning in Technology
Machine Learning (ML) is a cornerstone of modern technology, revolutionizing various industries with its ability to enable systems to learn from data without being explicitly programmed. At its core, ML involves algorithms that can identify patterns, make predictions, and improve performance over time based on the data they process. This technology has become indispensable in numerous practical applications and real-world scenarios. In healthcare, for instance, ML algorithms are used to analyze medical images such as X-rays and MRIs to detect diseases like cancer more accurately and earlier than human clinicians. Predictive analytics in healthcare also leverage ML to forecast patient outcomes, manage chronic conditions, and personalize treatment plans. For example, Google's AI-powered LYNA (Lymph Node Assistant) can detect breast cancer from mammography images with a high degree of accuracy, outperforming human radiologists in some cases. In finance, ML is crucial for fraud detection and risk assessment. Banks and financial institutions use ML models to analyze transaction patterns and identify anomalies that may indicate fraudulent activity. These models can also predict creditworthiness by analyzing a borrower's financial history and other relevant data points. For instance, PayPal uses ML to monitor transactions in real-time, significantly reducing the incidence of fraudulent activities. The retail industry benefits from ML through personalized marketing and customer service. Online retailers like Amazon and Netflix use ML algorithms to recommend products and content based on user behavior and preferences. These recommendations not only enhance the user experience but also drive sales by suggesting items that are likely to interest the customer. In transportation, ML is transforming the way we travel. Self-driving cars, such as those developed by Tesla and Waymo, rely heavily on ML to interpret sensor data from cameras, radar, and lidar to navigate roads safely. Additionally, ride-sharing services like Uber use ML to optimize routes, predict demand, and match drivers with passengers efficiently. Furthermore, ML is enhancing customer service through chatbots and virtual assistants. Companies like IBM and Microsoft offer AI-powered chatbots that can understand natural language and respond appropriately to customer inquiries. These chatbots learn from interactions over time, improving their ability to resolve issues without human intervention. In summary, Machine Learning is not just a buzzword; it is a powerful tool that is being applied across diverse sectors to solve complex problems, improve efficiency, and enhance decision-making. From healthcare diagnostics to financial risk management, from personalized retail experiences to autonomous vehicles, ML is revolutionizing how we live and work by enabling machines to learn from data and adapt to new situations. As the volume of data continues to grow exponentially, the potential applications of ML will only expand further, driving innovation and transformation in various aspects of our lives.
"ML" in Everyday Communication
In everyday communication, Machine Learning (ML) plays a pivotal role, often operating behind the scenes to enhance and personalize our interactions. One of the most evident examples is in virtual assistants like Siri, Alexa, and Google Assistant. These AI-driven tools use ML algorithms to understand voice commands, learn user preferences, and provide tailored responses. For instance, if you frequently ask your virtual assistant for the weather forecast in the morning, it will adapt to anticipate this request and offer the information proactively. Another significant application of ML is in email and messaging platforms. Spam filters, for example, employ ML to identify and filter out unwanted emails based on patterns and user feedback. This not only helps in maintaining a clean inbox but also protects users from potential phishing attacks. Additionally, predictive text features in messaging apps like WhatsApp or Facebook Messenger use ML to suggest words or phrases as you type, making communication faster and more efficient. Social media platforms also leverage ML extensively. Algorithms analyze user behavior to curate personalized feeds, ensuring that you see content that is most relevant to your interests. This personalization extends to advertisements as well, where ML helps in targeting ads based on user profiles and browsing history, making them more effective for businesses. Furthermore, ML is integral in customer service chatbots that many companies use to handle inquiries. These chatbots can understand natural language inputs and respond accordingly, providing immediate support and reducing the need for human intervention in routine queries. This not only improves customer satisfaction but also allows human customer support agents to focus on more complex issues. In the realm of language translation, ML has revolutionized real-time communication across different languages. Tools like Google Translate use sophisticated ML models to translate text and speech accurately, breaking down language barriers and facilitating global communication. This is particularly useful for travelers, international businesses, and global communities. Lastly, ML enhances security in communication by detecting anomalies and potential threats. For example, banks use ML to monitor transactions for signs of fraud, alerting users and preventing unauthorized activities. Similarly, cybersecurity systems employ ML to identify and block malicious software or phishing attempts, safeguarding sensitive information. In summary, ML is deeply embedded in various facets of everyday communication, from virtual assistants and email filters to social media algorithms and customer service chatbots. Its ability to learn from data and adapt to user behavior makes it an indispensable tool for enhancing efficiency, personalization, and security in our daily interactions.
Cross-Disciplinary Uses of "ML"
Machine Learning (ML) has transcended its traditional boundaries to become a versatile tool across various disciplines, revolutionizing how we approach complex problems and make informed decisions. In **healthcare**, ML algorithms are used to analyze medical images, diagnose diseases more accurately, and predict patient outcomes. For instance, deep learning models can detect early signs of cancer from mammography images, while natural language processing (NLP) helps in extracting insights from electronic health records. In **finance**, ML is employed for risk assessment, fraud detection, and portfolio optimization. Predictive models can forecast stock prices and identify potential market trends, enabling more informed investment decisions. Additionally, NLP is used to analyze financial news and sentiment analysis to gauge market sentiment. **Retail** and **marketing** also benefit significantly from ML. Personalized recommendation systems, powered by collaborative filtering and content-based filtering, enhance customer experience by suggesting products based on past behavior and preferences. Customer segmentation and churn prediction models help businesses tailor their strategies to retain customers and attract new ones. In **environmental science**, ML is crucial for monitoring climate change, predicting weather patterns, and optimizing resource usage. For example, satellite imagery analysis using ML can track deforestation rates and detect early signs of natural disasters like wildfires or hurricanes. **Transportation** systems are being transformed with the integration of ML. Autonomous vehicles rely on ML to interpret sensor data, navigate through traffic, and make real-time decisions. Predictive maintenance models help in scheduling vehicle maintenance, reducing downtime and improving overall efficiency. **Education** is another domain where ML is making a significant impact. Adaptive learning systems adjust the difficulty level of course materials based on individual student performance, ensuring a more personalized learning experience. Automated grading systems free up instructors' time, allowing them to focus on teaching rather than administrative tasks. Furthermore, **government** agencies use ML for public safety, crime prediction, and policy analysis. Predictive policing models help in identifying high-crime areas and allocating resources more effectively. NLP is used in analyzing public feedback on policies, providing valuable insights for policymakers. These cross-disciplinary applications of ML underscore its potential to drive innovation and solve real-world problems across diverse fields. By leveraging large datasets and advanced algorithms, ML continues to enhance decision-making processes, improve operational efficiency, and open new avenues for research and development. As technology advances, the scope of ML's impact is likely to expand further, transforming industries in ways that were previously unimaginable.