What Is Response Bias
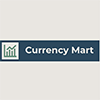
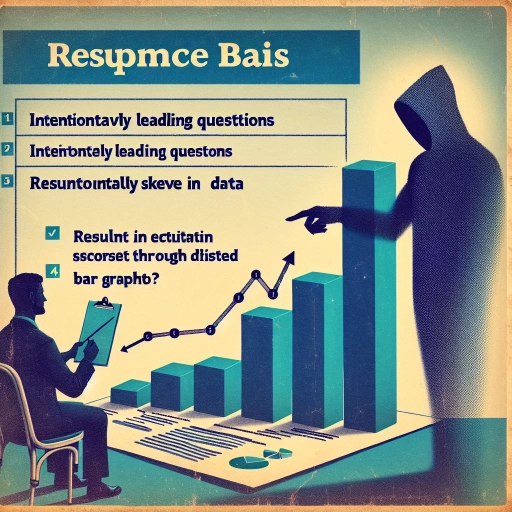
Response bias is a critical concept in research and data collection, influencing the accuracy and reliability of survey results, experimental outcomes, and other forms of data gathering. It refers to the systematic errors introduced into measurements when respondents react to a question in a way that is influenced by factors other than the true state of the phenomenon being measured. Understanding response bias is essential for researchers, policymakers, and anyone seeking to draw meaningful conclusions from data. This article delves into the intricacies of response bias, starting with a comprehensive definition and exploration of its various types. We will then examine the causes and consequences of response bias, highlighting how it can skew results and impact decision-making processes. Finally, we will discuss strategies and techniques for mitigating response bias, ensuring that data collected is as accurate and unbiased as possible. By grasping these elements, readers can better navigate the complexities of data interpretation and improve the quality of their research. Let us begin by understanding the definition and types of response bias.
Understanding Response Bias: Definition and Types
Understanding response bias is crucial for ensuring the accuracy and reliability of data collected through surveys, interviews, and other research methods. Response bias refers to the systematic errors introduced into survey results due to the way questions are asked or the way respondents answer them. This concept is multifaceted and can significantly impact the validity of research findings. To delve into this topic, it is essential to first define what response bias is, which involves understanding the underlying mechanisms that lead to biased responses. Next, identifying the common types of response bias, such as social desirability bias, acquiescence bias, and non-response bias, helps researchers anticipate and mitigate these issues. Finally, examining real-world examples of response bias illustrates how these biases manifest in practical scenarios, providing valuable insights for improving data collection techniques. By grasping these aspects, researchers can better design studies that yield more accurate and reliable data. Let us begin by exploring the definition of response bias in more detail.
1. Definition of Response Bias
**Definition of Response Bias** Response bias, a critical concept in the realm of survey research and data collection, refers to the systematic errors or distortions that occur when respondents provide answers that do not accurately reflect their true beliefs, attitudes, or behaviors. This phenomenon arises due to various factors, including the way questions are framed, the context in which they are asked, and the inherent biases of the respondents themselves. For instance, social desirability bias—a common type of response bias—occurs when individuals respond in a manner they believe will be viewed favorably by others, rather than honestly. Similarly, acquiescence bias happens when respondents tend to agree with statements regardless of their content, simply because they prefer to concur rather than disagree. The presence of response bias can significantly skew the results of surveys and studies, leading to misleading conclusions and potentially harmful decisions based on inaccurate data. Understanding and mitigating response bias is therefore essential for researchers to ensure the validity and reliability of their findings. By recognizing the potential for bias and employing strategies such as randomized response techniques, anonymous surveys, and carefully crafted questionnaires, researchers can minimize the impact of response bias and gather more accurate insights into the attitudes and behaviors they are studying. Ultimately, addressing response bias is a crucial step in maintaining the integrity of research and ensuring that data-driven decisions are based on truthful and representative information.
2. Common Types of Response Bias
Response bias, a critical concept in research and data analysis, can significantly impact the validity and reliability of survey results. Among the various types of response biases, two common ones stand out: **Social Desirability Bias** and **Acquiescence Bias**. **Social Desirability Bias** occurs when respondents answer questions in a way that they believe will be viewed favorably by others. This bias arises from the desire to present oneself in a positive light, leading individuals to overreport socially desirable behaviors and underreport socially undesirable ones. For instance, in a survey about health habits, respondents might exaggerate their exercise frequency or downplay their consumption of unhealthy foods to align with societal norms. This bias can skew data, making it challenging to obtain accurate insights into actual behaviors and attitudes. Researchers often use techniques such as anonymous surveys or indirect questioning methods to mitigate this bias. **Acquiescence Bias**, on the other hand, refers to the tendency of respondents to agree with statements or questions regardless of their content. This bias is also known as "yea-saying" and can result from a variety of factors, including a desire to please the interviewer, lack of attention to the question's content, or simply a habit of agreeing. For example, in a customer satisfaction survey, some respondents might consistently rate all aspects of a service highly without critically evaluating each aspect. This can lead to inflated positive ratings that do not reflect genuine opinions. To combat acquiescence bias, researchers may use balanced scales that include both positive and negative statements or employ reverse-coded items that require respondents to disagree with certain statements. Understanding these biases is crucial for ensuring the integrity of research findings. By recognizing and addressing social desirability and acquiescence biases, researchers can implement strategies to minimize their impact, such as using multiple data collection methods, pretesting surveys for bias, and employing statistical adjustments. These efforts help in obtaining more accurate and reliable data, which is essential for making informed decisions in various fields, including marketing, healthcare, and social sciences. Ultimately, awareness of these biases enhances the overall quality of research and contributes to more meaningful insights into human behavior and attitudes.
3. Real-World Examples of Response Bias
Response bias, a pervasive issue in data collection and analysis, can significantly skew the results of surveys, experiments, and other research endeavors. Here are three real-world examples that illustrate the impact of response bias: 1. **Social Desirability Bias in Health Surveys**: In a study on smoking habits, researchers found that respondents often underreported their smoking frequency to align with societal norms of healthiness. For instance, a survey conducted by the Centers for Disease Control and Prevention (CDC) revealed that self-reported smoking rates were lower than actual rates measured through biochemical tests. This social desirability bias led to an underestimation of the true prevalence of smoking, highlighting the need for more objective measurement methods. 2. **Non-Response Bias in Political Polls**: During the 2016 U.S. presidential election, many polls underestimated Donald Trump's support due to non-response bias. Some voters who supported Trump were less likely to participate in polls or disclose their true preferences, leading to an inaccurate representation of public opinion. This bias was particularly evident among certain demographic groups who felt their views were not represented or valued in mainstream surveys. 3. **Confirmation Bias in Market Research**: A well-known example of confirmation bias can be seen in the case of New Coke, introduced by Coca-Cola in the 1980s. Market research indicated strong consumer preference for the new formula over the original, but these findings were heavily influenced by the way questions were framed and the selection of participants. Consumers were more likely to respond positively to questions that suggested New Coke was an improvement, reflecting their desire to please the researchers rather than their genuine preferences. The subsequent backlash against New Coke demonstrated how confirmation bias can lead to misguided business decisions. These examples underscore the importance of recognizing and mitigating response bias in various contexts. By understanding the different types of biases—such as social desirability, non-response, and confirmation biases—researchers can implement strategies like anonymous surveys, diverse sampling methods, and objective measurement tools to ensure more accurate and reliable data collection. Addressing response bias is crucial for drawing valid conclusions and making informed decisions based on empirical evidence.
Causes and Consequences of Response Bias
Response bias, a pervasive issue in research and data collection, arises from various complex factors that influence how individuals respond to surveys, interviews, and other forms of inquiry. Understanding the causes and consequences of response bias is crucial for ensuring the validity and reliability of research outcomes. This article delves into three key areas: psychological factors contributing to response bias, social and cultural influences on response bias, and the impact of response bias on research outcomes. Psychological factors, such as cognitive biases and emotional states, significantly shape how individuals perceive and respond to questions. Social and cultural influences, including norms and expectations, also play a critical role in shaping responses. Finally, the consequences of response bias can be far-reaching, affecting the accuracy and generalizability of research findings. By examining these aspects, researchers can better mitigate the effects of response bias and enhance the quality of their studies. Let us begin by exploring the psychological factors that contribute to this phenomenon, as they form the foundational layer of understanding response bias.
1. Psychological Factors Contributing to Response Bias
Response bias, a pervasive issue in various fields including psychology, sociology, and market research, is significantly influenced by psychological factors that shape how individuals respond to questions or stimuli. One of the primary psychological factors contributing to response bias is **social desirability bias**, where respondents tend to answer questions in a manner that they believe will be viewed favorably by others. This bias arises from the desire to present oneself in a positive light, leading to overreporting of socially desirable behaviors and underreporting of undesirable ones. For instance, in surveys about health habits, people may exaggerate their exercise frequency or downplay their alcohol consumption to align with societal norms. Another critical factor is **cognitive biases**, such as confirmation bias and anchoring bias. Confirmation bias leads respondents to selectively seek out and recall information that confirms their pre-existing beliefs, while anchoring bias causes them to rely too heavily on the first piece of information they receive when making subsequent judgments. These biases can skew responses by making them more consistent with existing mental frameworks rather than objective reality. For example, in political surveys, individuals might interpret neutral or ambiguous statements in a way that supports their political leanings due to confirmation bias. **Emotional states** also play a significant role in shaping responses. The affect heuristic suggests that people make judgments based on how they feel about an issue rather than on a thorough analysis of the facts. If a respondent is in a positive emotional state, they are more likely to provide optimistic responses, while negative emotions can lead to more pessimistic answers. This can be particularly relevant in customer satisfaction surveys where recent experiences or current mood can significantly influence feedback. Additionally, **personality traits** such as extraversion and neuroticism can influence response patterns. Extraverted individuals may be more likely to provide socially desirable answers to gain approval, whereas neurotic individuals might be more prone to negative or exaggerated responses due to their heightened sensitivity to stress and anxiety. **Cultural background** is another psychological factor that contributes to response bias. Different cultures have varying norms and values that affect how individuals perceive and respond to questions. For example, collectivist cultures may prioritize group harmony over individual honesty, leading to responses that reflect group consensus rather than personal opinions. Lastly, **motivation** plays a crucial role in determining the accuracy of responses. Respondents who are highly motivated—either intrinsically or extrinsically—tend to provide more thoughtful and accurate answers. Conversely, lack of motivation can result in careless or random responses, further exacerbating response bias. Understanding these psychological factors is essential for researchers and practitioners aiming to mitigate response bias and ensure the reliability and validity of their data. By acknowledging and addressing these biases through careful survey design, data collection methods, and analysis techniques, it is possible to reduce their impact and obtain more accurate insights into human behavior and attitudes.
2. Social and Cultural Influences on Response Bias
Social and cultural influences play a significant role in shaping response bias, as individuals' answers to surveys, questionnaires, and other forms of data collection can be influenced by their social environment and cultural norms. For instance, in collectivist cultures, where the group's interests are prioritized over individual interests, respondents may be more inclined to provide answers that align with the perceived expectations of their community rather than their true opinions. This phenomenon is known as "social desirability bias," where individuals tend to respond in ways they believe will be viewed favorably by others. In contrast, individualistic cultures may foster more honest responses as individuals are less concerned with conforming to group norms. Cultural differences also affect how questions are interpreted and answered. For example, in some cultures, directness is valued, while in others, indirectness and politeness are more important. This can lead to varying levels of acquiescence bias, where respondents from certain cultures are more likely to agree with statements regardless of their true beliefs simply because they prefer to avoid conflict or maintain social harmony. Additionally, the language used in surveys can introduce bias if it is not culturally sensitive or if it assumes a level of literacy that not all respondents possess. Moreover, social status and power dynamics within a society can influence response bias. In hierarchical societies, respondents may feel pressured to provide answers that they believe will be acceptable to those in positions of authority. This can result in "status quo bias," where respondents prefer to maintain the existing social order rather than risk challenging it by providing honest but potentially controversial answers. The impact of these social and cultural influences on response bias is multifaceted and can have significant consequences for data quality and interpretation. For researchers, understanding these biases is crucial for designing surveys that minimize their effects and ensure that the data collected accurately reflects the population being studied. Techniques such as using culturally sensitive language, ensuring anonymity, and employing diverse data collection methods can help mitigate these biases. However, even with such precautions, it is important for analysts to remain aware of the potential for social and cultural influences to skew responses and to consider these factors when interpreting the results. In conclusion, social and cultural influences are powerful determinants of response bias, affecting how individuals respond to surveys and other data collection tools. Recognizing these influences is essential for conducting reliable research and making informed decisions based on the data collected. By acknowledging and addressing these biases, researchers can enhance the validity and reliability of their findings, ultimately contributing to a more accurate understanding of the phenomena they are studying.
3. Impact of Response Bias on Research Outcomes
Response bias significantly impacts research outcomes, introducing systematic errors that can skew results and lead to misleading conclusions. This bias arises from various sources, including social desirability bias, where participants provide answers they believe are socially acceptable rather than their true opinions. For instance, in a study on health behaviors, respondents might overreport their adherence to healthy habits and underreport unhealthy behaviors to align with societal norms. This distortion can result in an inaccurate representation of the population's actual behaviors, leading researchers to develop interventions that may not effectively address the real issues. Another form of response bias is acquiescence bias, where participants tend to agree with statements regardless of their content. This can occur in surveys or questionnaires where respondents may not fully understand the questions or may be inclined to provide affirmative answers due to a desire for approval or ease. For example, in a customer satisfaction survey, acquiescence bias could lead to inflated satisfaction scores if respondents consistently agree with positive statements without critically evaluating them. Consequently, businesses might misinterpret these results and fail to identify genuine areas for improvement. Furthermore, response bias can also manifest as non-response bias, where certain groups within the sample population are less likely to participate or respond to surveys. This can happen if the survey method or content inadvertently excludes or discourages participation from specific demographics. For instance, an online survey might exclude older adults who are less familiar with digital technologies, leading to an underrepresentation of their views and experiences. As a result, research findings may not be generalizable to the broader population, limiting their applicability and validity. Additionally, the order in which questions are presented can influence responses due to primacy and recency effects. Primacy effect occurs when respondents are more likely to remember and be influenced by the first items in a series, while recency effect occurs when they are more influenced by the last items. For example, in a political survey, placing questions about economic policies before questions about social policies could lead respondents to frame their answers on social policies in terms of economic considerations rather than independent evaluations. This can result in biased interpretations of public opinion that do not accurately reflect the complexities of individual perspectives. To mitigate these impacts, researchers employ various strategies such as using multiple data collection methods, ensuring questionnaires are clear and unbiased, and implementing data validation techniques. Randomizing the order of questions or using balanced scales can help reduce primacy and recency effects. Moreover, pre-testing surveys with diverse groups can help identify potential biases before the main study is conducted. By acknowledging and addressing response bias proactively, researchers can enhance the reliability and generalizability of their findings, ultimately contributing to more informed decision-making across various fields. In conclusion, response bias has profound implications for research outcomes by introducing systematic errors that can mislead interpretations and applications of findings. Understanding the different forms of response bias—such as social desirability bias, acquiescence bias, non-response bias, and order effects—is crucial for developing robust research designs that minimize these biases. By employing rigorous methodologies and validation techniques, researchers can ensure that their studies provide accurate insights that reflect the true nature of the phenomena being investigated.
Mitigating Response Bias: Strategies and Techniques
Mitigating response bias is a crucial aspect of research and data collection, as it ensures the accuracy and reliability of the information gathered. Response bias can skew results, leading to misleading conclusions and potentially harmful decisions. To address this issue, several strategies and techniques must be employed. First, **designing unbiased questionnaires and surveys** is essential to prevent leading questions and ensure that respondents provide honest answers without influence. Second, **using data analysis techniques to detect bias** allows researchers to identify and correct for any biases that may have occurred during the data collection process. Finally, **best practices for minimizing response bias in studies** involve careful planning, execution, and review of the research methodology to prevent biases from the outset. By focusing on these key areas, researchers can significantly enhance the validity of their findings. Let's begin by exploring the critical role of **designing unbiased questionnaires and surveys** in ensuring the integrity of research data.
1. Designing Unbiased Questionnaires and Surveys
Designing unbiased questionnaires and surveys is a crucial step in mitigating response bias, ensuring that the data collected accurately reflects the true opinions and behaviors of the respondents. To achieve this, several key strategies must be employed. First, it is essential to use clear and concise language in the questions, avoiding ambiguity and technical jargon that might confuse respondents. This clarity helps prevent misinterpretation and ensures that all participants understand the questions in the same way. Second, questions should be neutral and free from leading language or loaded terms that could influence respondents' answers. For instance, instead of asking "How satisfied are you with our excellent customer service?" which implies a positive experience, ask "How would you rate our customer service?" This neutrality helps in gathering honest feedback without swaying the respondent's opinion. Third, the order of questions can significantly impact responses. To avoid order bias, it is advisable to randomize the sequence of questions or use a balanced approach where sensitive or controversial questions are interspersed with more neutral ones. This helps in preventing the context of previous questions from influencing subsequent responses. Fourth, ensuring anonymity or confidentiality can encourage honest responses. When respondents feel that their answers will not be traced back to them, they are more likely to provide truthful feedback without fear of judgment or repercussions. Additionally, pilot testing the questionnaire with a small group of participants can help identify any biases or issues before the full-scale survey is conducted. This allows for necessary adjustments to be made to ensure that the final version is unbiased and effective. Finally, using multiple response formats such as Likert scales, open-ended questions, and multiple-choice options can provide a more comprehensive understanding of respondents' opinions. This diversity in question types helps in capturing both quantitative and qualitative data, offering a richer insight into the subject matter. By adhering to these principles—clear language, neutrality, balanced question order, anonymity, pilot testing, and varied response formats—researchers can significantly reduce the risk of response bias and gather reliable data that accurately represents the population being studied. This meticulous approach not only enhances the credibility of the survey but also ensures that the findings are actionable and meaningful.
2. Using Data Analysis Techniques to Detect Bias
**Using Data Analysis Techniques to Detect Bias** Detecting bias in data is a critical step in ensuring the integrity and reliability of research findings. Response bias, which arises from the way questions are asked or the context in which they are presented, can significantly skew results. To mitigate this issue, researchers employ various data analysis techniques that help identify and address potential biases. One of the primary methods is **statistical analysis**. Techniques such as regression analysis and correlation studies can reveal patterns that may indicate bias. For instance, if responses to certain questions consistently correlate with demographic variables like age or gender, it may suggest that the questions are biased towards particular groups. **Data visualization** tools are also invaluable; plots and charts can visually highlight discrepancies in response patterns that might not be immediately apparent from raw data. **Item response theory (IRT)** is another powerful tool for detecting bias. IRT models assess how individuals respond to different items on a survey or test, allowing researchers to identify items that may be biased against certain groups. This method helps in pinpointing specific questions or items that contribute to overall bias. **Differential item functioning (DIF) analysis** is a subset of IRT that specifically examines whether different groups respond differently to the same item, even when they have the same underlying trait or ability. By identifying items with significant DIF, researchers can either revise or remove these items to ensure fairness and reduce bias. **Machine learning algorithms** can also be leveraged to detect subtle biases in large datasets. These algorithms can identify complex patterns and anomalies that might indicate biased responses. For example, clustering algorithms can group similar responses together, revealing clusters that may correspond to biased subgroups. Moreover, **sensitivity analysis** is crucial for understanding how robust the findings are to different assumptions and methods. By varying the parameters and models used in analysis, researchers can determine if the results are consistent across different scenarios, thereby validating the absence of significant bias. In addition to these technical approaches, **qualitative methods** such as focus groups and cognitive interviews provide valuable insights into how respondents interpret questions and whether they perceive any biases. These qualitative data can complement quantitative analyses by offering a deeper understanding of the context in which responses are given. Finally, **pre-testing surveys** with diverse pilot groups helps in identifying potential biases before the full-scale survey is conducted. This step allows for the refinement of survey instruments to ensure they are fair and unbiased. By combining these data analysis techniques—statistical analysis, data visualization, IRT, DIF analysis, machine learning algorithms, sensitivity analysis, qualitative methods, and pre-testing surveys—researchers can comprehensively detect and mitigate response bias. This multi-faceted approach ensures that the data collected is reliable and reflects the true opinions and behaviors of the respondents without undue influence from biased questions or contexts.
3. Best Practices for Minimizing Response Bias in Studies
When conducting studies, minimizing response bias is crucial to ensure the accuracy and reliability of the data collected. Here are three best practices that researchers can employ to mitigate this issue: 1. **Anonymity and Confidentiality**: Ensuring participant anonymity and confidentiality can significantly reduce response bias. When respondents feel that their answers will not be traced back to them, they are more likely to provide honest and unbiased responses. This can be achieved through the use of anonymous surveys, online questionnaires with secure encryption, or the use of third-party data collection services. By guaranteeing privacy, researchers can encourage participants to answer questions truthfully without fear of judgment or repercussions. 2. **Neutral Question Framing**: The way questions are framed can influence respondents' answers, leading to biased responses. To avoid this, questions should be phrased in a neutral manner, avoiding leading language or loaded terms that might sway the respondent's answer. For example, instead of asking "How satisfied are you with our excellent customer service?" which implies a positive experience, ask "How would you rate our customer service?" This approach helps in gathering unbiased feedback by not influencing the respondent's perception. 3. **Randomization and Control Groups**: Implementing randomization and control groups can help in identifying and mitigating response bias. Randomizing the order of questions or the presentation of stimuli can prevent order effects where the sequence of questions influences responses. Additionally, using control groups allows researchers to compare responses between different conditions, helping to isolate any biases that may arise from specific treatments or interventions. This method ensures that any observed effects are due to the variables being studied rather than extraneous factors. By incorporating these strategies into study design, researchers can enhance the validity of their findings, ensuring that the data collected accurately reflects the true opinions and behaviors of the participants. These best practices not only improve the quality of research but also contribute to more reliable and generalizable results.