What Is A Covariate
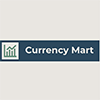
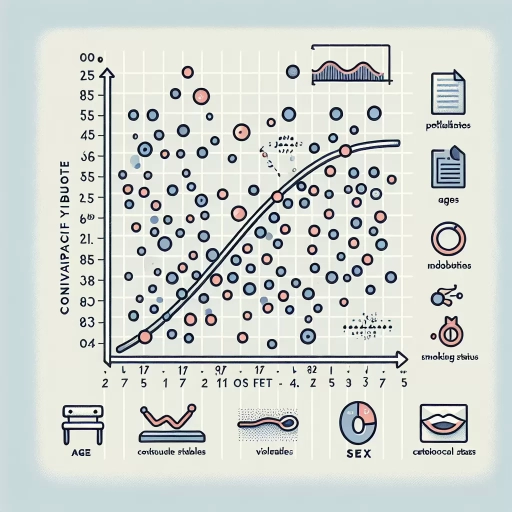
In the realm of statistical analysis and research, understanding the concept of a covariate is crucial for drawing accurate conclusions and making informed decisions. A covariate, often overlooked but fundamentally important, plays a significant role in controlling for extraneous variables that could influence the outcome of a study. This article delves into the definition and concept of a covariate, exploring what it is and how it functions within statistical models. We will also examine the various types and examples of covariates, highlighting their diverse applications across different fields. Furthermore, we will discuss the importance and applications of covariates, illustrating their impact on research outcomes and real-world scenarios. By grasping these key aspects, researchers and analysts can better design studies, interpret data, and ensure the validity of their findings. Let's begin by defining and conceptualizing what a covariate is, laying the groundwork for a deeper understanding of its significance in statistical analysis.
Definition and Concept of a Covariate
In the realm of statistical analysis, understanding the concept of a covariate is crucial for drawing accurate conclusions and making informed decisions. A covariate, often referred to as a control variable or confounding variable, is a variable that is related to both the independent and dependent variables in a study. This article delves into the definition and concept of a covariate, exploring its significance in three key areas: statistical context and terminology, its distinction from independent and dependent variables, and its pivotal role in regression analysis. Firstly, we will examine the statistical context and terminology surrounding covariates, clarifying how they fit into the broader framework of statistical research. This section will provide a foundational understanding of what covariates are and how they are identified. Next, we will differentiate covariates from independent and dependent variables, highlighting their unique role in experimental design and data analysis. This distinction is vital for researchers to ensure that their studies are well-controlled and that the effects of the independent variable are accurately measured. Finally, we will discuss the role of covariates in regression analysis, explaining how they help to adjust for extraneous factors that could influence the relationship between the independent and dependent variables. By incorporating covariates into regression models, researchers can enhance the precision and reliability of their findings. Understanding these aspects will provide a comprehensive view of covariates, starting with their **Statistical Context and Terminology**.
Statistical Context and Terminology
In the realm of statistical analysis, understanding the context and terminology is crucial for accurately interpreting and applying statistical concepts. When discussing covariates, it is essential to grasp the broader statistical framework in which they operate. A covariate, by definition, is a variable that is related to the outcome of interest but is not the primary variable being studied. This concept is often explored within the context of regression analysis, where covariates are used to control for confounding variables that could influence the relationship between the independent variable and the dependent variable. Statistical context involves recognizing the type of data being analyzed (continuous, categorical, etc.), the research question or hypothesis, and the appropriate statistical methods to use. For instance, in linear regression, covariates are included to adjust for their potential impact on the outcome variable, thereby providing a more precise estimate of the effect of the independent variable. The terminology associated with covariates includes terms like "confounding variable," "control variable," and "predictor variable," each highlighting different aspects of how covariates function within a statistical model. Key terms such as "correlation," "association," and "causation" are also integral to understanding covariates. Correlation refers to the statistical relationship between two variables, while association indicates a relationship that may not necessarily be causal. Causation implies that changes in one variable directly influence changes in another variable. In studies involving covariates, researchers aim to identify correlations and associations while controlling for potential confounders to better understand causal relationships. Moreover, statistical terminology like "regression coefficient," "standard error," and "p-value" are critical when interpreting the results of analyses involving covariates. The regression coefficient quantifies the change in the outcome variable for a one-unit change in the covariate, holding all other variables constant. The standard error provides a measure of variability around this coefficient, while the p-value indicates the probability of observing the results (or more extreme) assuming no real effect exists. Understanding these statistical concepts and terminology is vital for accurately defining and applying the concept of a covariate in research studies. By recognizing how covariates interact with other variables within a statistical model, researchers can draw more reliable conclusions about the relationships under investigation. This precision in understanding not only enhances the validity of research findings but also ensures that conclusions are based on sound statistical reasoning. In summary, grasping the statistical context and terminology surrounding covariates is fundamental for conducting rigorous and meaningful statistical analyses.
Difference from Independent and Dependent Variables
In the context of statistical analysis and research, understanding the distinction between independent and dependent variables is crucial for designing and interpreting studies effectively. The **independent variable** is the factor that the researcher manipulates or changes to observe its effect on the outcome. It is often denoted as the predictor or explanatory variable because it predicts or explains the variation in the dependent variable. For instance, in an experiment to see how different levels of fertilizer affect plant growth, the amount of fertilizer applied would be the independent variable. On the other hand, the **dependent variable** is the outcome or response being measured in response to changes made to the independent variable. It is also known as the outcome or response variable because it responds to the changes in the independent variable. Continuing with the fertilizer example, plant growth (measured in terms of height or biomass) would be the dependent variable. To illustrate further, consider a study examining how exercise duration affects blood pressure. Here, exercise duration is the independent variable because it is being manipulated by the researcher, while blood pressure is the dependent variable because it is being measured as a response to different exercise durations. Understanding these roles is essential for ensuring that research questions are clearly defined and that data collection methods are appropriate. Misidentifying these variables can lead to flawed study designs and incorrect interpretations of results. For example, if a researcher mistakenly treats blood pressure as an independent variable and exercise duration as a dependent variable, they would be analyzing the data incorrectly and potentially drawing misleading conclusions. In contrast to these primary variables, **covariates** are additional variables that may influence the relationship between the independent and dependent variables but are not of primary interest in the study. Covariates can be either controlled for or accounted for in statistical analyses to ensure that the observed effects are due to the independent variable rather than other factors. For instance, in a study on exercise and blood pressure, age could be a covariate because it might also affect blood pressure levels independently of exercise duration. By distinguishing between independent and dependent variables and considering the role of covariates, researchers can enhance the validity and reliability of their findings, ensuring that their conclusions accurately reflect the underlying relationships being studied. This clarity is fundamental for conducting rigorous scientific research and for making informed decisions based on empirical evidence.
Role in Regression Analysis
In the context of regression analysis, a covariate plays a crucial role in understanding the relationship between the dependent variable and one or more independent variables. A covariate is essentially any variable that is related to the dependent variable and can influence the outcome of the regression model. The primary function of a covariate is to control for its effect on the dependent variable, thereby allowing researchers to isolate the impact of the independent variable(s) of interest. When included in a regression model, covariates help to reduce confounding bias by accounting for extraneous factors that might otherwise distort the relationship between the independent and dependent variables. For instance, in a study examining the effect of exercise on weight loss, age and gender could be considered covariates because they can influence both exercise habits and weight loss outcomes. By controlling for these covariates, researchers can obtain a more accurate estimate of how exercise affects weight loss independently of age and gender. The inclusion of covariates also enhances the precision and reliability of regression models. By adjusting for covariates, models become more robust and better reflect real-world scenarios where multiple factors interact. This is particularly important in fields such as medicine, economics, and social sciences where complex relationships between variables are common. Moreover, covariates can be used to test hypotheses about moderation effects. For example, a researcher might investigate whether the relationship between stress levels and anxiety differs based on age or gender by including interaction terms involving these covariates in the model. In practice, selecting appropriate covariates involves careful consideration of theoretical knowledge and empirical evidence. Researchers must identify variables that are likely to affect both the independent and dependent variables and ensure that these covariates are measured accurately and reliably. Mis-specification or omission of relevant covariates can lead to biased estimates and incorrect conclusions. In summary, covariates are essential components of regression analysis as they enable researchers to control for extraneous influences, enhance model precision, and explore complex relationships between variables. Their inclusion is critical for drawing valid inferences about the relationships under study and for ensuring that regression models accurately reflect real-world phenomena.
Types and Examples of Covariates
When analyzing data, understanding the different types of covariates is crucial for drawing accurate conclusions and making informed decisions. Covariates are variables that can affect the outcome of a study, and they come in various forms. This article delves into three key distinctions: Continuous vs. Categorical Covariates, Demographic vs. Behavioral Covariates, and Environmental vs. Genetic Covariates. Each of these categories provides unique insights into how different variables interact with the outcome of interest. For instance, continuous covariates, such as age or blood pressure, can be measured on a continuous scale, while categorical covariates, like gender or treatment group, are classified into distinct categories. Demographic covariates, such as income or education level, often reflect static characteristics, whereas behavioral covariates, like exercise habits or smoking status, capture dynamic aspects of an individual's lifestyle. Additionally, environmental covariates, such as air pollution or socioeconomic status, and genetic covariates, like genetic markers or family history, highlight the interplay between external factors and innate traits. By understanding these distinctions, researchers can better control for confounding variables and enhance the validity of their findings. This article begins by exploring the fundamental difference between Continuous and Categorical Covariates, setting the stage for a deeper examination of these critical variables.
Continuous vs. Categorical Covariates
In the context of statistical analysis, covariates can be broadly classified into two primary types: continuous and categorical. Understanding the distinction between these two is crucial for accurate model specification and interpretation. **Continuous Covariates** are variables that can take any value within a given range, often measured on a numerical scale. Examples include age, height, weight, and blood pressure. These covariates are typically analyzed using linear or nonlinear models, where the relationship between the covariate and the outcome variable can be described by a continuous function. For instance, in a study examining the relationship between age and cognitive performance, age would be treated as a continuous covariate. Continuous covariates offer the advantage of capturing subtle variations in the data, allowing for more nuanced analyses. **Categorical Covariates**, on the other hand, are variables that take on distinct categories or levels. These can be further divided into binary (two categories) and multi-level categorical variables. Examples include gender (male/female), treatment group (control/intervention), and educational level (high school/college/university). Categorical covariates are often analyzed using dummy or indicator variables in regression models. For example, in a study comparing the outcomes of patients receiving different treatments, the treatment group would be a categorical covariate. Categorical covariates are useful for capturing discrete differences and are particularly important when the categories represent distinct groups or conditions. The choice between treating a covariate as continuous or categorical depends on the nature of the variable and the research question. Sometimes, a variable that is inherently continuous might be categorized for simplicity or to reflect meaningful thresholds (e.g., categorizing blood pressure into normal, prehypertensive, and hypertensive). However, this categorization can lead to loss of information and reduced precision in estimates. Conversely, treating a categorical variable as continuous can result in misleading interpretations if the underlying categories do not have a natural ordering or numerical meaning. In summary, continuous covariates provide detailed information about the relationship between variables across a range of values, while categorical covariates highlight differences between distinct groups. Proper identification and treatment of these covariates are essential for conducting robust and meaningful statistical analyses. By understanding whether a covariate is best represented as continuous or categorical, researchers can ensure that their models accurately reflect the underlying data and research hypotheses. This distinction is fundamental in various fields such as medicine, social sciences, and engineering, where accurate modeling of relationships is critical for drawing valid conclusions and making informed decisions.
Demographic vs. Behavioral Covariates
When discussing covariates, it is crucial to distinguish between demographic and behavioral covariates, as each type provides different insights into the variables influencing a study or analysis. **Demographic covariates** refer to characteristics that describe the population being studied, such as age, gender, ethnicity, income level, education, and marital status. These variables are often static or change slowly over time and are used to understand how different demographic groups respond to treatments or interventions. For example, in a study examining the effectiveness of a new medication, demographic covariates like age and gender might be used to see if the drug's efficacy varies across different age groups or genders. On the other hand, **behavioral covariates** involve actions or habits that individuals exhibit, which can be more dynamic and subject to change. Examples include lifestyle choices like diet, exercise habits, smoking status, and adherence to treatment regimens. Behavioral covariates are particularly useful in studies where the focus is on how certain behaviors influence outcomes. For instance, in a research project investigating the impact of regular physical activity on cardiovascular health, behavioral covariates such as exercise frequency and type would be essential in understanding the relationship between physical activity and health outcomes. Understanding the distinction between these two types of covariates is vital because it allows researchers to control for various factors that could influence their results. By including both demographic and behavioral covariates in an analysis, researchers can gain a more comprehensive understanding of the complex interactions between different variables and the outcomes they are studying. This nuanced approach helps in identifying potential confounding variables and ensures that the conclusions drawn from the data are more accurate and reliable. In summary, while demographic covariates provide a snapshot of the population's static characteristics, behavioral covariates offer insights into the dynamic actions and habits that can significantly impact study outcomes.
Environmental vs. Genetic Covariates
When discussing covariates, it is crucial to distinguish between environmental and genetic factors, as both play significant roles in influencing outcomes in various studies. **Environmental covariates** refer to external factors that can affect the dependent variable in a study. These include lifestyle choices such as diet, exercise habits, smoking status, and exposure to pollutants. For instance, in a study examining the impact of air pollution on respiratory health, factors like urban vs. rural living conditions or proximity to industrial sites would be considered environmental covariates. These variables can be modified through interventions or policy changes and are often the focus of public health initiatives aimed at reducing disease risk. On the other hand, **genetic covariates** involve inherited traits that can influence study outcomes. These include genetic markers, mutations, or polymorphisms that may predispose individuals to certain conditions or affect their response to treatments. For example, in a clinical trial evaluating the efficacy of a new medication for a specific disease, genetic variations that affect drug metabolism or disease susceptibility would be considered genetic covariates. Understanding these genetic factors is essential for personalized medicine and can help in tailoring treatments to individual genetic profiles. Both types of covariates are critical for ensuring the validity and reliability of research findings. By controlling for environmental and genetic covariates, researchers can isolate the effect of the independent variable on the dependent variable, thereby reducing confounding and increasing the precision of their results. This distinction is particularly important in fields like epidemiology, where understanding the interplay between environmental exposures and genetic predispositions can inform prevention strategies and treatment protocols. Ultimately, recognizing and accounting for both environmental and genetic covariates enhances the robustness of research designs and contributes to a more comprehensive understanding of complex phenomena.
Importance and Applications of Covariates
The importance and applications of covariates in statistical analysis cannot be overstated. Covariates play a crucial role in enhancing the accuracy and reliability of models by accounting for variables that could otherwise skew results. By incorporating covariates, researchers can control for confounding variables, which are factors that may influence the relationship between the independent and dependent variables, thereby ensuring more robust and unbiased conclusions. This is particularly significant in real-world applications, where understanding the impact of covariates can inform policy decisions and research outcomes. For instance, in medical research, covariates such as age, gender, and pre-existing conditions can significantly affect the interpretation of treatment effects. Similarly, in economic studies, factors like income level and education can influence the analysis of economic indicators. By controlling for these confounding variables, researchers can draw more accurate and reliable conclusions. This article will delve into these aspects, starting with the critical role of covariates in controlling for confounding variables.
Controlling for Confounding Variables
Controlling for confounding variables is a crucial aspect of statistical analysis, particularly when examining the relationship between an independent variable and a dependent variable. Confounding variables are factors that can influence both the independent and dependent variables, potentially leading to biased or misleading conclusions if not properly accounted for. To control for these variables, researchers employ various statistical techniques such as stratification, matching, and regression analysis. **Stratification** involves dividing the data into subgroups based on the confounding variable and analyzing the relationship within each subgroup. This method helps to isolate the effect of the independent variable by ensuring that comparisons are made within homogeneous groups regarding the confounder. **Matching** techniques, such as propensity score matching, pair observations with similar values on the confounding variables but differing in their exposure to the independent variable. This approach ensures that any observed differences between groups can be more confidently attributed to the independent variable rather than the confounder. **Regression analysis**, particularly multiple regression, is another powerful tool for controlling confounding variables. By including both the independent variable and confounding variables in the model, regression analysis allows researchers to estimate the effect of the independent variable while adjusting for the influence of confounders. Techniques like partial least squares regression and generalized linear models can also be used depending on the nature of the data. In addition to these methods, **instrumental variables** can be used in certain contexts where there is a variable that affects the independent variable but not directly the dependent variable, except through its effect on the independent variable. This approach helps to identify causal effects even in the presence of unobserved confounders. Controlling for confounding variables is essential because it enhances the validity and reliability of research findings. Without proper control, studies may overestimate or underestimate the true relationship between variables, leading to incorrect conclusions and potentially harmful decisions in fields such as medicine, policy-making, and social sciences. For instance, in clinical trials, failing to account for age or gender could lead to misleading results about the efficacy of a new drug. In summary, controlling for confounding variables is a critical step in statistical analysis that ensures the accuracy and generalizability of research findings. By employing appropriate methods such as stratification, matching, regression analysis, and instrumental variables, researchers can mitigate the impact of confounders and draw more reliable conclusions about causal relationships. This meticulous approach underscores the importance of covariates in research design and analysis.
Enhancing Model Accuracy and Reliability
Enhancing model accuracy and reliability is a critical aspect of statistical analysis, particularly when incorporating covariates into the model. Covariates, variables that are related to the outcome of interest but are not the primary focus of the study, play a pivotal role in improving model performance. By including relevant covariates, researchers can control for confounding variables, thereby reducing bias and increasing the precision of estimates. This is especially important in fields such as medicine, where understanding the impact of covariates like age, gender, and pre-existing conditions can significantly enhance the accuracy of predictive models for disease outcomes. For instance, in clinical trials, covariates such as baseline health status or genetic markers can influence treatment response. Including these covariates in the analysis allows for more nuanced interpretations of treatment effects, ensuring that any observed differences are due to the treatment itself rather than underlying factors. Similarly, in economic studies, covariates like income level, education, and employment status can provide a more comprehensive understanding of economic behaviors and outcomes. The inclusion of covariates also enhances model reliability by accounting for variability that might otherwise be attributed to random error. This leads to more robust and generalizable findings, as the model is better equipped to handle real-world complexities. Furthermore, advanced statistical techniques such as propensity score matching and regression adjustment can be employed to leverage covariates effectively, ensuring that the analysis is both rigorous and insightful. In addition to improving accuracy and reliability, covariates facilitate the identification of interaction effects and moderation, which can reveal subtle but significant relationships between variables. For example, in educational research, understanding how student performance is influenced by both socioeconomic status and access to resources can help policymakers design more targeted interventions. Overall, the strategic use of covariates is essential for developing models that are not only accurate but also reliable and applicable across diverse contexts. By carefully selecting and integrating relevant covariates into statistical models, researchers can produce findings that are more precise, interpretable, and actionable, ultimately contributing to better decision-making in various fields. This underscores the importance of covariates in enhancing model performance and highlights their critical role in advancing our understanding of complex phenomena.
Real-World Applications in Research and Policy
In the realm of research and policy, covariates play a crucial role in enhancing the accuracy and reliability of studies. Real-world applications of covariates are diverse and impactful, influencing various fields such as healthcare, economics, education, and environmental science. For instance, in healthcare research, covariates like age, gender, and pre-existing conditions are essential for understanding the efficacy of new treatments. By controlling for these variables, researchers can isolate the effect of the treatment itself, leading to more precise conclusions that inform clinical practice and policy decisions. In economics, covariates such as income level, education, and employment status help economists model complex relationships between economic indicators and policy interventions. This allows policymakers to design targeted interventions that address specific socio-economic needs. In education, covariates like socio-economic status and prior academic performance help educators evaluate the effectiveness of educational programs and policies, ensuring that resources are allocated efficiently to support student success. Additionally, in environmental science, covariates such as temperature, precipitation, and land use patterns are critical for understanding the impact of climate change and developing effective conservation policies. By incorporating these variables into their analyses, researchers can provide policymakers with robust evidence-based recommendations that drive meaningful change. Overall, the strategic use of covariates in research ensures that findings are contextually relevant and actionable, thereby bridging the gap between academic inquiry and practical policy implementation.