What Ai Detector Does Turnitin Use
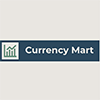
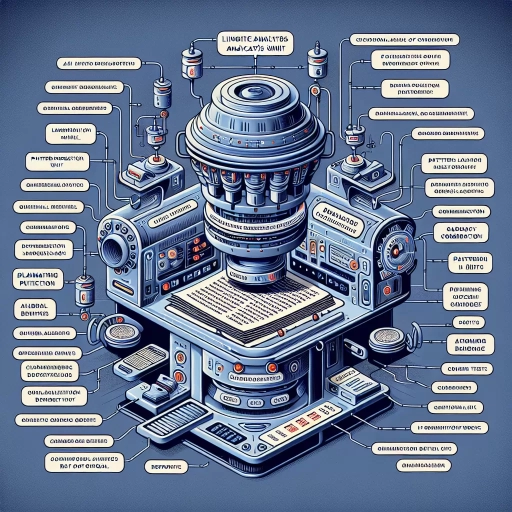
Introduction to Turnitin and AI Detection
Turnitin, an essential tool in today's education system, is highly regarded for its effectiveness in maintaining academic integrity. This introductory piece will shed light on Turnitin's purpose, how it integrates artificial intelligence for detection, and the historical context of these AI detection tools. Our first focus will be on Turnitin's role and functionality, as it serves more than just a plagiarism detection tool; it offers a comprehensive approach to keeping the educational ethic intact. The use of advanced artificial intelligence to detect academic dishonesty is of paramount importance in an era where digital manipulation is at its peak. Our discussion includes these AI's significant contributions to upholding valuable educational norms. Moreover, we will delve into the fascinating historical journey of how AI detection tools originated and have evolved, paving the way for sophisticated platforms like Turnitin. By understanding these facets, we can appreciate the sophistication of these systems and the crucial role they play in today's academic setting. Let us start our exploration with an overview of Turnitin's purpose and functionality.
Overview of Turnitin's Purpose and Functionality
Turnitin, as a leading AI detector, wields significant influence in the academic sector as a tool for maintaining robust and rigorous scholastic integrity. Primarily, it serves the purpose of apprehending potential instances of plagiarism within written work handed in by students. As such, the significant functionality of Turnitin revolves around the detection and prevention of intellectual property theft, ensuring students' work is authentic, original, and free from plagiarism. In that vein, Turnitin employs a sophisticated AI system designed to comb through an extensive database of academic papers, online articles, books and published resources while cross-referencing each one with the submitted text. What's more, the program goes the extra mile, not only recognizing exact matches but also incorporating semantic analysis to detect instances of patchwriting or paraphrasing that is too close to the source. It is equally adept at identifying collusion, where separate students submit significantly identical assignments which may not match any external source but are nonetheless not their individual work. Moreover, the artificial intelligence system of Turnitin never stops learning and improving. It continually assesses and adopts new patterns and structures based on the increasing volume of data being inputted into its system from academic papers across the globe. This ensures the system stays one step ahead of any evolving tactics to bypass the system. Another striking feature of Turnitin is its similarity report, which indicates the percentage of material in a submitted assignment that can be traced back to existing sources. This functionality empowers educators with invaluable insights into students' research and citation practices, aiding in the creation of more pointed feedback and addressing misconceptions. Additionally, Turnitin also offers 'Gradescope', an AI-facilitated grading tool, aimed at standardizing grading, increasing transparency, speeding up the evaluation process, and fostering feedback between educators and students. Unsurprisingly, these rigorous checking mechanisms have positioned Turnitin as a stalwart ally in upholding academic integrity. Not only does it serve as a preventive tool, dissuading students from engaging in plagiarism, but it also serves as a teaching tool, educating students on how to correctly manage and acknowledge sources, thereby cultivating a culture of authentic academic pursuit. In conclusion, the dual functionality of Turnitin as both a detector and a deterrent mirror its purpose of encouraging academic honesty and discouraging shortcut practices. By utilizing AI, it is consistently evolving, ensuring it remains an efficient tool in the prevention, detection, and education of plagiarism.
Importance of AI Detection in Academic Integrity
As we delve deeper into the era of the internet and digital transformation, maintaining academic integrity has become both profoundly critical and dauntingly challenging. This is where AI detection, particularly via tools such as Turnitin, plays an indispensable role. Artificial Intelligence (AI) provides a cutting-edge approach towards ensuring authenticity and fairness in academic writing. Turnitin, a leading AI tool, uses proprietary algorithms and machine learning techniques to match and analyze text against a database that includes web pages, student papers, and various publications. Understanding the relevance of AI in academic integrity sheds light on the urgency of advanced plagiarism detection. Academic dishonesty such as plagiarism not only undermines the integrity of education but also hampers student growth and development. They propel the students into an erroneous belief that the route to academic success can be short-circuited rather than through meaningful engagement with the subject matter. The act, if unchecked, goes against the ethos of learning, discourages original thinking, dilutes creativity and incubates a culture of unethical practice. Artificial Intelligence based detection means such as Turnitin provide an automated, objective and efficient method of deterring and identifying plagiarism. The technology incorporates machine learning to map sentence structures, keywords, and semantics; comparing them with their mega repository. This helps in identifying matches or similar constructs, thereby flagging potential instances of plagiarism. Turnitin uses a proprietary algorithm that breaks down essays into smaller pieces and scrutinizes each part individually, checking for duplications and parallels in language. It then provides a similarity index specifying the percentage of identical content. Such an AI-based detection mode is not solely reliant on matching exact phrases or sentences but can intuit semantic structures and flag paraphrased intent as well. It also identifies situations where references and citations have been used illicitly, therefore, providing a comprehensive review of academic integrity. Moreover, AI’s ability to learn and adapt makes it a formidable tool in the fight against plagiarism. Over time, as AI tools like Turnitin ingest more data, they become smarter, faster and more accurate. This results in continual improvements in the detection and prediction of academic dishonesty. In conclusion, the importance of AI detection in reinforcing academic integrity cannot be stressed enough. AI tools like Turnitin are vital in making plagiarism detection more objective, efficient, and reliable. They help ensure the sanctity of scholarly discourse and fortify the foundations of academic honesty, equipping educators with the ability to keep abreast of sophisticated methods of dishonest submission. Therefore, AI detection stands as a firm pillar of support in upholding academic integrity in today's rapidly evolving educational landscape.
Historical Context of AI Detection Tools
Artificial Intelligence (AI) has achieved significant progress in various fields, including plagiarism and authenticity detection. AI detection tools have a profound historical context that allows us to appreciate its current complexities and sophistication. The advent of the internet brought forward an era of vast and easily accessible information. This made it increasingly difficult to monitor and maintain the authenticity of academic content leading to the development of plagiarism detection tools. Among these, Turnitin emerged in the late 1990s by a group of researchers as a groundbreaking tool to counteract plagiarism and uphold the sanctity of original work. The inception of AI detection tools like Turnitin, from its early days, aimed at creating a system that could effectively identify instances of plagiarism. In its initial stages, Turnitin operated on simple algorithms, essentially comparing strings of texts to a database of sources. However, as technology evolved, so did the level of sophistication and scope of AI detection tools. It started incorporating machine learning and AI principles in the early 21st century. These powerful computing principles unlocked a new level of capability in plagiarism checking. Turnitin integrated semantic analysis, contextual relevance, and a robust database of academic content to decipher not just direct plagiarism but also paraphrasing and sophisticated content manipulation. The late 2000s saw the introduction of the 'similarity score' - an AI-powered metric that gave more nuanced data to educators about potential plagiarism. Turnitin's AI capabilities continue to develop, penetrating deeper layers of content authenticity and introducing more advanced plagiarism detection techniques. The AI algorithms used by Turnitin can analyze a vast amount of data at lightning-fast speeds, enhancing its accuracy and increasing its reliability. The historical progression of AI detection tools is characterized by constant growth and technological developments, equipping it with a sharper understanding of language, content structure, and subtle intimations of plagiarism. With increasingly sophisticated counter-plagiarism measures, the AI incorporated in Turnitin emerged from the historical necessity driven by information expansion over the internet and the rising demand for authenticity in academic, research, and publishing sectors. Thus, understanding this historical context of the Turnitin and AI detection tools offers a fascinating perspective into the evolution and impact AI has had on the academic and publishing world. Future advancements in AI detection are already on the horizon; powered by new AI models, Turnitin and related tools will further perfect their accuracy and efficiency in detecting even the most subtle instances of plagiarism and promoting a culture of originality and intellectual honesty.
Technical Aspects of Turnitin's AI Detector
The article further delves into the technical aspects of Turnitin's AI detector to elucidate how it contributes to easing academic integrity assurance. The first chapter of our exploration revolves around the machine learning algorithms applied by Turnitin. An in depth look into how these algorithms function forms the crux of understanding how the tool spots plagiarism with remarkable precision. We then turn our focus onto the data sources and training models for AI detection to decipher how Turnitin's expansive database and the training models it adopts enhance its accuracy. Lastly, we highlight the integration of Turnitin with other plagiarism detection tools to understand how this conjunction widens its plagiarism detection net. Each segment of this exploration aim to cast light on the distinctive mechanisms incorporated by Turnitin in ensuring academic integrity. Beginning with an overview on the Machine Learning Algorithms employed by Turnitin, we seek to illustrate how the tool algorithmically detects plagiarised content and grades papers with an indisputable proficiency.
Machine Learning Algorithms Used by Turnitin
Turnitin, a software widely used by academic and professional institutions to detect plagiarism, employs a variety of cutting-edge machine learning (ML) algorithms in its Artificial Intelligence (AI) detection system. The primary ML algorithm used by Turnitin is Supervised Learning Algorithm. This method uses previously labeled input and output data to predict future outputs for the new data. Turnitin uses this algorithm to train its AI detector with a vast repository of databases to recognize patterns and detect similar syntax. This enables Turnitin’s algorithm to learn which documents are similar to others and which are plagiarized. Additional to Supervised Learning, Turnitin uses the Latent Semantic Analysis (LSA) algorithm, which aids in identifying semantic similarities across various documents. LSA can decrypt underlying meaning, allowing the system to cross-verify assignments based not only on verbatim phrases but also on the similarity of ideas or concepts. In the context of textual analysis and plagiarism detection, such an advanced algorithm offers a more nuanced, concept-based approach that stretches beyond checking verbatim matches. Markedly, another crucial algorithm employed by Turnitin is the K-Nearest Neighbor (KNN) algorithm. KNN is used in instances where Turnitin's AI detector needs to classify an unmarked or unlabeled piece of work. Based on the 'K' nearest data points in relation to a submission where 'K' represents the set number of neighbors decided by the program, KNN classifies the new submission. Thus, KNN allows Turnitin to categorize documents dependant on their semblance to other works. Lastly, the Bayesian Classification algorithm is also employed by Turnitin, a probabilistic algorithm that utilizes statistical methods to predict the classification of new data based on past data. Hereby, recognizing the likelihood of a document matching or not matching existing work. This ability is pivotal to Turnitin's function as it facilitates its ability to consider a certain document's entirety, making sense of individual sentences, and comprehending the feature of various assignments in different fields. The complexity of these ML algorithms showcases Turnitin's cutting-edge capacity to capture various forms of plagiarism, from verbatim copying to paraphrasing and idea-smuggling. By combining these algorithms and continuously training them using a wide array of academic databases, Turnitin can provide a robust and reliable tool to ensure academic integrity.
Data Sources and Training Models for AI Detection
The technical aspects of Turnitin’s AI detector are primarily focused on its data sources and the training models used for AI detection. Excellent data sources are crucial in any AI or machine learning system as they serve as the backbone of the AI detection process. Turnitin’s AI detector, per se, relies on vast databases containing billions of web pages, articles, books, and a wide range of academic papers. Such a massive amount of data is pivotal for data comparison, ensuring the system's accuracy in checking for potential plagiarism scenarios. Nevertheless, the data is continually evolving with new information added to the databases, which makes the detector dynamically adaptive to the changing research environments. The real power, however, lies in the AI detection techniques, which is a broad and profound area that covers several key elements. For one, algorithms training is an important part of the equation, leveraging vast data sources to learn how to detect plagiarism effectively. The AI is trained using machine learning algorithms that learn to identify patterns of plagiarism and can adapt to evolving trends in academia. The process of training an AI model involves the application of supervised learning techniques. One of the significant models used in the detector is the neural network model, whose structure consists of interconnected artificial neurons mirroring a human brain's neural structure. These artificial neurons are trained to a point they can interpret data inputs in a contextually dynamic manner. Moreover, decision trees a prevalent tool in the machine learning dimension are utilized to facilitate data classification tasks within the AI detector. They prove instrumental in predicting outcomes and extensively used based on their low computational requirements. Additionally, Turnitin’s AI detector harnesses the power of Natural Language Processing (NLP), a subfield of AI that deals with the interaction between computers and humans using natural language. NLP supports the detector by thoroughly analyzing students’ submissions, interpreting, and relating them to an array of sourced contextual references. Furthermore, every processed input gets analyzed for semantic and syntactic structures due. In sum, the technical expanse of Turnitin's AI detection revolves around substantial data sourcing and the implementation of intricate training models like neural networks, decision trees, and NLP backed systems. This synthesis of advanced algorithms, vast data sources and intricate machine learning models ensure the thorough and accurate detection of any potential plagiarism cases, making Turnitin’s AI detection among the most sophisticated in the academic world.
Integration with Other Plagiarism Detection Tools
Advanced technology has enabled integration with other plagiarism detection tools, making Turnitin's AI Detector more effective in identifying academic dishonesty. This seamless integration creates a comprehensive system where the AI detector collaborates with other tools to check for content similarity, while also leveraging machine learning techniques to discern various forms of plagiarism. Besides, it broadens the plagiarism detection spectrum, ensuring all submitted content is thoroughly scrutinized, which guarantees peak academic integrity. Turnitin’s functionally rich application programming interfaces (APIs) facilitate the integration with external plagiarism detection tools like Grammarly, Copyscape, and ProWritingAid, among others. This opens up a wider web of resources that complement Turnitin’s existing capabilities. The amalgam of these diverse tools produces a robust AI detector, well-armed to deal with sophisticated methods of plagiarism. From copied phrases and paraphrased texts to clone and CTRL+C plagiarism, Turnitin’s integrated AI detector capably determines any semblance of plagiarism. In the technical aspect, the integration process utilizes an intelligent algorithm that operates on deep learning models. The AI detector learns from vast quantities of data from the connected tools and employs this knowledge to identify subtle patterns of plagiarism that a basic similarity index would overlook. The AI interface utilizes Natural Language Processing (NLP) to understand and interpret human language in the text, thereby adding a layer of cognitive intelligence in identifying paraphrasing or restructuring of sentences which would otherwise slip through text-matching algorithms. This integrated system not only boosts the detector's overall plagiarism detection capabilities but also helps in reducing the error rate. The integrated tools utilize various databases and information repositories worldwide, each applying different techniques to cross-check the content’s originality. Turnitin's AI detector, fed with this disparate data, refines the plagiarism detection process, which significantly reduces false positives and negatives, ensuring more accurate results. Through this technical integration with other plagiarism detection tools, Turnitin’s AI detector becomes a powerful, multifunctional tool that innovatively maintains academic integrity. Its capability to pull resources and machine learning techniques from diverse tools offers an unrivaled check against plagiarism. Therefore, education institutions can have absolute confidence in the thoroughness and dependability of their plagiarism detection process, attributing to Turnitin's AI detector.
Effectiveness and Limitations of Turnitin's AI Detector
Turnitin's AI detector greatly revolutionized the academic and publishing sectors, boasting the capabilities to scour vast databases and trace sources to detect plagiarism. However, as with any technology, it has its highlights and areas of improvement. This article will focus on three crucial aspects: the success stories and case studies on AI detection, potential false positives and false negatives, and the promising future of AI detection enhancement. Initially, we dive into various success stories, emphasizing how Turnitin's AI detector has improved academic honesty and intellectual property rights. We will take an in-depth look at case studies from educational and professional settings to further highlight its effectiveness. These accounts narrate the triumph of technology in nurturing a respectful and responsible academic and professional world. As we progress, there's a need to discuss the system's susceptibilities - particularly false positives and negatives, revealing the detector's limitations. Lastly, we'll gaze upon the horizon of AI detection technology, envisioning the future improvements anticipated for these systems. Now, let's turn our attention to the authentic triumphs brought about by Turnitin's AI detector in various sectors.
Success Stories and Case Studies on AI Detection
Artificial Intelligence (AI) application in academic integrity and plagiarism detection has significantly evolved over time, particularly with platforms such as Turnitin. They have employed AI detector, leveraging the expansive potential of machine learning and natural language processing (NLP) to enhance the process of identifying similarities and borrowed content. Several success stories echo the effectiveness of Turnitin's AI detector. The most recent, from the University of North Texas, illustrates how the detector successfully flagged several hundred research papers and essays for potential plagiarism that had initially slipped past human monitoring. The AI detector not only detected verbatim plagiarism but also paraphrased data, cross-language plagiarism, and even ghostwritten content. The most striking part of this success is its accuracy, as corroborated by the university's subsequent manual checks. Furthermore, the University of Sydney reported a substantial decline in academic dishonesty cases following the introduction of Turnitin's AI detector. Another prominent success narrative came from the University of Groningen where the AI detector effectively identified a sophisticated form of academic dishonesty. Here, students were found guilty of 'contract cheating'. Unlike traditional plagiarism, students engaged third-party essay mills to write original content, making it a highly elusive form of academic dishonesty. However, Turnitin's AI detector outperformed manual detection owing to its artificial intelligence that could correlate patterns and similarity indices within a massive repository of globally submitted essays. Conversely, there are some contextual limitations related to false positives, which Turnitin is continually striving to minimize. The AI detector may mistakenly flag common phrases or statistics as plagiarism, leading to false alarms. Moreover, with a lack of understanding idiomatic expressions, the AI detector may not identify plagiarism if the content is deeply paraphrased. Also, there is the inherent limitation of language proficiency since the performance accuracy varies with different languages. However, these limitations notwithstanding, the overall effectiveness and the advances seen in Turnitin's AI detector corroborates its high utility at maintaining the sanctity of academic content and fairness. It allows resources to be channeled more efficiently by reducing the workload for manual checks, increasing the range and scope of plagiarism checks, and freeing educators to engage more deeply in imparting knowledge. In a nutshell, the merits far outweigh the drawbacks, positioning Turnitin's AI as an indispensable tool in academic integrity enforcement.
Potential False Positives and False Negatives
Turnitin's AI detector uses a myriad of algorithms and techniques, one of them being language processing, to identify possibly plagiarized content in academic works. However, like any AI-driven system, on certain occasions, it is prone to creating potential 'False Positives' and 'False Negatives'. A 'False Positive' refers to a situation where Turnitin's AI detector mistakenly flags a piece of content as plagiarized when actually it is not. This situation can occur due to various factors. A common cause attributed to this would be the incorrect identification of commonly used phrases or industry jargon as copied content. For instance, in a medical paper, certain terminologies or procedural phrases could be misinterpreted as plagiarism just because they have been mentioned in other papers. Also, another common cause would be the incorrect assessment of correctly referenced or cited quotes. These scenarios often put innocent students under scrutiny, causing unwarranted stress. 'False Negatives', on the other hand, occur when the system fails to identify an instance of plagiarism, thus allowing a dishonest act to go unnoticed. Turnitin's AI detector usually does an impressive job at identifying similarities and possible copied content from various sources available online. But there is always a possibility that it might miss plagiarized content if the source from where the information has been copied is not included in its database. Another potential scenario would be the failure to detect instances of 'paraphrasing plagiarism', where the plagiarizing student has merely reworded the copied content, making it difficult for the system to spot it. These potential instances of 'False Positives' and 'False Negatives' highlight certain limitations of Turnitin's AI detector, illustrating that while its effectiveness is high, it is not infallible. It's paramount to comprehend these limitations when interpreting the similarity reports provided by the program. These false alarms, on both ends, necessitate human intervention for the final verification to ensure the validity of the results, indicating that AI tools should not fully replace human judgment, but rather serve as supporting mechanisms in academic integrity processes.
Future Developments and Improvements in AI Detection
As technology evolves, the role of Artificial Intelligence (AI) in content integrity verification, like Turnitin's AI Detector, becomes increasingly critical. The realm of AI is continually expanding and with it the sophistication, reach, and precision of AI for plagiarism detection. The future enhancements in AI detection models aim at overcoming the current limitations and refining the system's efficiency further. Presently, Turnitin's AI detector primarily identifies word-for-word plagiarism, self-plagiarism, and paraphrased copying, but struggles to detect sophisticated forms of academic dishonesty, such as contracted cheating or ghostwriting. However, further developments in AI detection can be game-changing. Impending improvements in AI detection technology contemplate using machine learning algorithms that can understand the context and the semantics of a textual content, rather than just focusing on similarity indexes. This will broaden AI's ability to detect more complex forms of content duplication, like the crafting of 'original' content using multiple sources, as well as intentional content manipulation, aimed at tricking traditional detection models. Furthermore, researchers are exploring deep learning technologies to improve AI's ability to learn from human feedback, to increase its understanding of user intent, and to refine its contextual perception of content. This would make it possible for AI detectors to apprehend subtle nuances, minimise false positives, and enhance its accuracy. Additionally, advances in AI predictive analytics could become instrumental in detecting plagiarism even before it occurs, by interpreting a student's writing style and detecting any unusual changes that might suggest the work isn't original. The next generation AI detectors could even integrate with other modern technologies, such as blockchain, to securely store academic records and ensure data credibility. This would facilitate a reliable checking system across different academic institutions worldwide, thereby creating a robust academic integrity network. AI's capability to use Natural Language Processing (NLP) to understand, interpret, and generate human language is also promising. Future AI detectors could use updated NLP to analyse the semantic structure of a text, making it far more efficient in identifying sophisticated plagiarism attempts, such as stitching from multiple sources to create 'original' content. In essence, future developments and improvements in AI detection technology aspire to overcome current limitations, expand its reach, and augment its efficiency to an extent where no act of academic dishonesty goes unnoticed – pushing the boundaries of AI detection beyond just content similarity and into the realm of content impropriety detection.