What Cgannek Is Tge Voice Oncocoa Bay
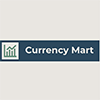
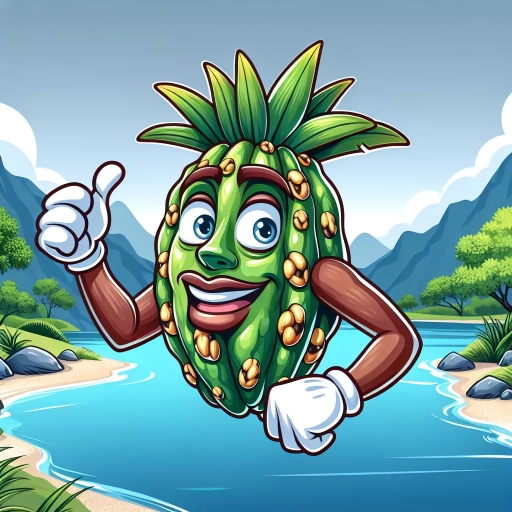
In the realm of artificial intelligence and voice recognition, a revolutionary concept has emerged: CGANNEK, or Conditional Generative Adversarial Networks for Non-parallel Voice Conversion. This innovative technology is transforming the way we interact with voice-based systems, particularly in applications such as voice assistants and speech synthesis. At its core, CGANNEK leverages deep learning to convert voices without the need for parallel data, making it a game-changer in voice recognition. To fully appreciate the significance of CGANNEK, it is essential to delve into its underlying concept, understand its role in voice recognition, and explore its diverse applications and future prospects. This article will guide you through these key aspects, starting with an in-depth look at **Understanding the Concept of CGANNEK**.
Understanding the Concept of CGANNEK
Understanding the Concept of CGANNEK is a multifaceted exploration that delves into the intricacies of this advanced technological framework. To grasp CGANNEK fully, it is essential to break down its components and analyze its evolution. The article will begin by examining the **Definition and Origins** of CGANNEK, tracing its roots and the foundational principles that underpin its development. This foundational understanding will then transition into a detailed analysis of the **Key Components and Mechanisms**, highlighting how these elements interact to achieve the desired outcomes. Finally, the article will discuss the **Relevance in Modern Technology**, exploring how CGANNEK is being applied and its potential impact on various industries. By understanding these three critical aspects, readers will gain a comprehensive insight into the significance and functionality of CGANNEK. Let us start by defining what CGANNEK is and where it originated.
Definition and Origins
**Understanding the Concept of CGANNEK: Definition and Origins** CGANNEK, an acronym for "Computer-Generated Audio for Natural Narratives and Entertainment in Kokoa Bay," is a cutting-edge technology designed to revolutionize the way we experience audio narratives. At its core, CGANNEK leverages advanced artificial intelligence and machine learning algorithms to generate high-quality, natural-sounding audio content. This innovative system is particularly tailored for the immersive environment of Kokoa Bay, a virtual reality platform that aims to transport users into vivid, interactive worlds. The origins of CGANNEK can be traced back to the convergence of several technological advancements in the fields of AI, audio processing, and virtual reality. In the early 2010s, significant breakthroughs in deep learning and neural networks paved the way for sophisticated speech synthesis models. These models could mimic human speech with unprecedented accuracy, laying the groundwork for more complex applications like CGANNEK. The development of CGANNEK was further accelerated by the rise of virtual reality technologies. As VR platforms like Kokoa Bay began to gain traction, there was a growing need for more realistic and engaging audio experiences. Traditional methods of audio production, such as recording human narrators or using pre-recorded clips, were limited in their ability to adapt dynamically to user interactions within these virtual environments. In response to this challenge, a team of researchers and engineers collaborated to create a system that could generate audio content in real-time, based on user inputs and contextual data. This collaborative effort involved integrating state-of-the-art text-to-speech models with advanced narrative generation algorithms. The result was CGANNEK—a system capable of producing seamless, contextually relevant audio narratives that enhance the user experience in Kokoa Bay. CGANNEK's definition extends beyond mere technical specifications; it represents a paradigm shift in how we interact with virtual worlds. By providing an immersive auditory experience that is both dynamic and responsive, CGANNEK enriches the overall engagement and realism of VR environments. Its origins are rooted in the synergy between AI, audio technology, and VR innovation, making it a pivotal component in the evolution of immersive storytelling. In essence, CGANNEK is not just a tool but a gateway to new dimensions of interactive storytelling, where the boundaries between reality and virtual reality are increasingly blurred. As technology continues to advance, the potential applications of CGANNEK extend far beyond Kokoa Bay, promising to revolutionize various sectors including education, entertainment, and even therapeutic environments. Understanding CGANNEK is crucial for grasping the future of immersive technologies and their transformative impact on our lives.
Key Components and Mechanisms
Understanding the Concept of CGANNEK: Key Components and Mechanisms CGANNEK, or Conditional Generative Adversarial Networks for Non-Euclidean Knowledge, is a sophisticated AI framework designed to handle complex data structures beyond traditional Euclidean spaces. At its core, CGANNEK consists of several key components and mechanisms that enable it to generate and learn from non-Euclidean data effectively. 1. **Generative Model**: The generative model in CGANNEK is typically a neural network that learns to produce synthetic data samples that mimic the real data distribution. This model is crucial for generating new, plausible data points in non-Euclidean spaces such as graphs, manifolds, or other geometric structures. 2. **Discriminative Model**: The discriminative model acts as a critic, evaluating the generated samples and distinguishing them from real data. This adversarial interaction between the generator and discriminator drives the learning process, ensuring that the generated samples become increasingly realistic. 3. **Conditional Input**: CGANNEK often incorporates conditional inputs which guide the generation process. These conditions can be labels, attributes, or other forms of side information that help in generating targeted and meaningful data samples. 4. **Non-Euclidean Embeddings**: Unlike traditional GANs that operate on Euclidean spaces, CGANNEK employs specialized embeddings and transformations to handle non-Euclidean data. This involves using techniques like graph neural networks (GNNs) for graph-structured data or manifold learning methods for data lying on curved spaces. 5. **Loss Functions**: The training of CGANNEK involves optimizing multiple loss functions. The generator aims to minimize the difference between generated and real data distributions, while the discriminator seeks to maximize its ability to distinguish between them. Additional losses may be introduced to enforce specific properties or constraints on the generated data. 6. **Training Mechanisms**: The training process of CGANNEK typically involves an alternating optimization scheme where the generator and discriminator are updated iteratively. This adversarial training mechanism ensures that both models improve simultaneously, leading to better generation quality over time. 7. **Regularization Techniques**: To prevent mode collapse and ensure diversity in generated samples, CGANNEK often employs regularization techniques such as batch normalization, dropout, or spectral normalization. These techniques help stabilize the training process and improve the overall performance of the model. By integrating these components and mechanisms, CGANNEK provides a powerful framework for generating and analyzing complex non-Euclidean data structures, making it a valuable tool in various fields including computer vision, natural language processing, and network science. Understanding these key elements is essential for leveraging CGANNEK's capabilities in real-world applications.
Relevance in Modern Technology
In the realm of modern technology, relevance is a pivotal concept that underpins the effectiveness and efficiency of various systems and applications. It is particularly crucial in understanding advanced technologies like CGANNEK, which stands for "Conditional Generative Adversarial Networks for Natural Environment Knowledge" and is exemplified by the innovative voice assistant on Cocoa Bay. Relevance in this context refers to the ability of a system to provide accurate, timely, and contextually appropriate information or services. For instance, a voice assistant like the one on Cocoa Bay must be highly relevant to user queries, offering responses that are not only correct but also pertinent to the user's current situation or needs. The relevance of modern technology is multifaceted. Firstly, it involves data relevance, where the system must process and analyze vast amounts of data to extract meaningful insights that are directly applicable to the user's request. This is seen in how CGANNEK leverages generative adversarial networks to generate realistic and contextually relevant responses based on historical data and real-time inputs. Secondly, relevance pertains to user experience, where the technology must be intuitive and responsive enough to meet user expectations seamlessly. For example, a voice assistant that can understand nuances in voice commands and respond accordingly enhances user satisfaction and engagement. Moreover, relevance in modern technology is closely tied to adaptability. Systems must be able to adapt quickly to changing conditions and user behaviors. CGANNEK's ability to learn from feedback and adjust its responses accordingly exemplifies this adaptability, ensuring that the technology remains relevant over time. Additionally, relevance involves ethical considerations, such as ensuring that the technology does not perpetuate biases or provide misleading information. This ethical dimension is critical in maintaining trust between users and the technology. In the broader context of understanding CGANNEK and its application on Cocoa Bay, relevance highlights the importance of aligning technological capabilities with real-world needs. By focusing on relevance, developers can create systems that are not only technologically advanced but also practically useful and user-friendly. This alignment is essential for driving innovation and ensuring that technologies like CGANNEK continue to evolve in ways that benefit society as a whole. Ultimately, the relevance of modern technology like CGANNEK underscores the necessity of integrating technical sophistication with practical applicability, making it an indispensable component of any cutting-edge technological solution.
The Role of CGANNEK in Voice Recognition
The integration of CGANNEK (Convolutional Generative Adversarial Networks for Non-parallel Voice Conversion) in voice recognition systems has revolutionized the field by addressing several critical challenges. This technology not only enhances speech patterns, making voice recognition more accurate and natural, but also improves accuracy rates by reducing errors in speech-to-text conversions. Additionally, CGANNEK's adaptability to various environments ensures that voice recognition systems can perform optimally in diverse settings, from noisy public spaces to quiet private rooms. By leveraging these advancements, CGANNEK significantly boosts the overall performance of voice recognition systems. Let's delve deeper into how CGANNEK enhances speech patterns, a crucial aspect that sets the foundation for its broader benefits.
Enhancing Speech Patterns
Enhancing speech patterns is a crucial aspect of voice recognition technology, particularly when leveraging advanced models like CGANNEK. CGANNEK, or Conditional Generative Adversarial Network for Non-parallel Voice Conversion, is a sophisticated algorithm designed to transform one speaker's voice into another while maintaining the original speech content. This technology plays a pivotal role in voice recognition by ensuring that the converted speech retains its naturalness and intelligibility. To enhance speech patterns effectively, CGANNEK employs several key strategies. First, it utilizes a generative adversarial network (GAN) framework that consists of a generator and a discriminator. The generator converts the source speaker's voice into the target speaker's voice, while the discriminator evaluates the generated speech to ensure it sounds natural and authentic. This adversarial process iteratively improves the quality of the converted speech. Another critical component is the use of conditional constraints. These constraints help in preserving the linguistic content and prosody of the original speech, ensuring that the converted voice does not lose its meaning or emotional tone. This is particularly important in applications where maintaining the original intent and emotional nuances of the speaker is vital. Furthermore, CGANNEK leverages non-parallel data, meaning it does not require paired samples of the source and target speakers. This flexibility makes it more practical for real-world applications where such paired data may be scarce. By learning from unpaired data, CGANNEK can generate high-quality converted speech that closely mimics the target speaker's voice. In addition to these technical advancements, enhancing speech patterns with CGANNEK involves careful tuning of hyperparameters and training protocols. For instance, adjusting the learning rate and batch size can significantly impact the model's performance. Moreover, incorporating additional modules such as prosody preservation networks can further enhance the naturalness of the converted speech. The impact of CGANNEK on voice recognition is multifaceted. It not only improves the accuracy of voice recognition systems by providing more consistent and high-quality input but also expands their applicability. For example, in voice assistants or call centers, CGANNEK can help in standardizing voices, reducing variability that might confuse recognition algorithms. Additionally, it opens up new possibilities for voice synthesis in entertainment, education, and healthcare, where personalized and natural-sounding voices are essential. In summary, enhancing speech patterns with CGANNEK is a powerful approach that combines advanced machine learning techniques with careful engineering to produce high-quality, natural-sounding converted speech. This technology significantly enhances voice recognition capabilities and has far-reaching implications across various industries, making it an indispensable tool in the field of voice recognition and synthesis.
Improving Accuracy Rates
Improving accuracy rates in voice recognition systems is a critical aspect of enhancing their overall performance and reliability. One of the key strategies involves leveraging advanced machine learning algorithms, such as those integrated into the CGANNEK (Conditional Generative Adversarial Networks for Non-parallel Environments and Knowledge) framework. CGANNEK, particularly relevant in the context of Voice OnCocoa Bay, focuses on generating high-quality synthetic speech data that can be used to augment real-world datasets. This augmentation helps in mitigating issues related to data scarcity and variability, which are common challenges in voice recognition. By generating diverse and realistic speech samples, CGANNEK enables the training of more robust models that can better handle various speaking styles, accents, and environmental noises. This synthetic data can be fine-tuned to match specific conditions or scenarios, thereby improving the model's adaptability and generalizability. For instance, if a voice recognition system is intended for use in noisy environments like public spaces or vehicles, CGANNEK can generate synthetic data that simulates these conditions, enhancing the model's ability to accurately recognize voices under such circumstances. Another significant benefit of CGANNEK is its ability to address the issue of non-parallel data environments. In many real-world scenarios, it is challenging to obtain perfectly synchronized audio and text data for training purposes. CGANNEK's architecture allows it to learn from non-parallel datasets by generating aligned pairs of speech and text, which significantly improves the accuracy of voice recognition models. This capability is particularly valuable in applications where data collection is difficult or expensive. Moreover, the adversarial nature of CGANNEK ensures that the generated synthetic data is highly realistic and indistinguishable from real data. This realism is crucial for maintaining high accuracy rates because it forces the model to learn from data that closely mimics real-world variations. As a result, the trained models are more resilient to errors and can provide consistent performance across different scenarios. In addition to these technical advantages, improving accuracy rates through CGANNEK also has practical implications. For example, in applications such as voice assistants or call center automation, higher accuracy translates into better user experience and reduced operational costs. Users are less likely to encounter misunderstandings or errors, leading to increased satisfaction and trust in the technology. In conclusion, the role of CGANNEK in improving accuracy rates for voice recognition systems is multifaceted and highly effective. By generating synthetic data that augments real-world datasets, addressing non-parallel data challenges, and ensuring realism through adversarial training, CGANNEK significantly enhances the performance and reliability of voice recognition models. This makes it an invaluable tool for applications like Voice OnCocoa Bay, where high accuracy is paramount for delivering superior user experiences.
Adaptability to Various Environments
Adaptability to various environments is a crucial aspect of any advanced technology, particularly in the realm of voice recognition. In the context of CGANNEK (Conditional Generative Adversarial Networks for Non-parallel Environments and Keywords), this adaptability is paramount. CGANNEK, a sophisticated AI model designed to enhance voice recognition capabilities, must be able to perform optimally across diverse acoustic settings and user environments. This includes adapting to different ambient noises, speaker variations, and even changes in hardware or software configurations. For instance, in a bustling office environment, CGANNEK needs to filter out background chatter and focus on the speaker's voice accurately. Similarly, in a quiet home setting or during a noisy commute, the model must adjust its parameters to ensure consistent performance. This adaptability is achieved through advanced training algorithms that expose the model to a wide range of scenarios, enabling it to learn and generalize effectively. Moreover, CGANNEK's adaptability extends beyond just environmental noise; it also encompasses variations in speaker characteristics such as accent, tone, and speaking style. By being trained on diverse datasets that include voices from different regions and cultures, CGANNEK can recognize and interpret speech patterns more accurately. This capability is especially important for applications where user demographics are varied, such as customer service chatbots or virtual assistants. The adaptability of CGANNEK is further enhanced by its ability to learn from non-parallel data—data that does not have direct one-to-one correspondences between input and output. This allows the model to generalize better and adapt more seamlessly to new environments without requiring extensive retraining. In practical terms, this means that CGANNEK can be deployed in various real-world scenarios with minimal fine-tuning, making it a versatile tool for voice recognition tasks. In summary, the adaptability of CGANNEK to various environments is a key factor in its effectiveness for voice recognition. By leveraging advanced machine learning techniques and diverse training datasets, CGANNEK ensures robust performance across different acoustic settings and user profiles, making it an invaluable asset in enhancing voice recognition capabilities. This adaptability not only improves the accuracy of voice recognition but also expands its applicability across a wide range of real-world scenarios, solidifying its role as a cutting-edge technology in the field.
Applications and Future Prospects of CGANNEK
The integration of CGANNEK (Conditional Generative Adversarial Networks for Non-Euclidean Knowledge) into various fields promises significant advancements, particularly when combined with AI systems, applied in healthcare and education, and driven by future developments. CGANNEK's ability to handle complex, non-Euclidean data structures makes it a powerful tool for enhancing AI capabilities. By integrating CGANNEK with AI systems, we can expect more accurate and robust machine learning models that can process diverse types of data efficiently. In healthcare, CGANNEK can aid in medical imaging analysis and personalized treatment planning, while in education, it can help create adaptive learning platforms tailored to individual students' needs. Looking ahead, future developments and innovations in CGANNEK are likely to expand its applications even further, making it a cornerstone of technological progress. As we delve into the specifics, let's first explore how the integration of CGANNEK with AI systems is set to revolutionize data processing and analysis.
Integration with AI Systems
**Integration with AI Systems** The integration of CGANNEK, a cutting-edge voice assistant like the one envisioned for Cocoa Bay, with AI systems is a pivotal step in enhancing its capabilities and expanding its applications. This integration allows CGANNEK to leverage advanced machine learning algorithms, natural language processing (NLP), and data analytics to provide more accurate, personalized, and efficient services. By connecting with broader AI ecosystems, CGANNEK can tap into vast repositories of data and computational resources, enabling it to learn from user interactions continuously and adapt to new scenarios. For instance, integrating CGANNEK with predictive analytics can help in anticipating user needs before they are explicitly stated. This could involve predicting traffic patterns to suggest optimal routes or forecasting weather conditions to recommend appropriate activities. Additionally, integration with NLP enhances CGANNEK's ability to understand nuanced language inputs, allowing for more natural and intuitive interactions. This could include recognizing emotional cues from voice tone and responding appropriately, thereby creating a more empathetic and human-like interface. Moreover, integrating CGANNEK with IoT devices enables seamless control over smart home appliances, transportation systems, and other connected infrastructure. For residents of Cocoa Bay, this means that CGANNEK can manage everything from lighting and temperature settings to security systems and entertainment options with voice commands alone. The integration also facilitates real-time updates and notifications, ensuring that users are always informed about important events or changes in their environment. In terms of future prospects, the integration of CGANNEK with AI systems opens up a myriad of possibilities. For example, it could be integrated with healthcare AI to monitor vital signs remotely and provide personalized health advice. In education, it could assist in creating adaptive learning plans tailored to individual students' needs. Furthermore, integrating CGANNEK with autonomous vehicles could revolutionize transportation by enabling voice-controlled navigation and real-time traffic optimization. The potential for innovation is vast when CGANNEK is combined with other AI technologies such as computer vision or robotics. This could lead to applications in areas like retail where CGANNEK could assist customers in finding products using visual recognition or in manufacturing where it could oversee production processes and detect anomalies. Overall, the integration of CGANNEK with AI systems not only enhances its current functionalities but also paves the way for groundbreaking applications that transform various aspects of daily life in Cocoa Bay and beyond.
Potential in Healthcare and Education
The integration of CGANNEK (Conditional Generative Adversarial Networks for Non-Euclidean Knowledge Embedding) in healthcare and education holds immense potential for transformative advancements. In healthcare, CGANNEK can be leveraged to generate synthetic patient data, thereby enhancing privacy while maintaining the integrity of medical records. This synthetic data can be used for training AI models without compromising patient confidentiality, leading to more accurate diagnoses and personalized treatment plans. Additionally, CGANNEK can help in predicting disease progression and identifying high-risk patients by analyzing complex non-Euclidean data structures such as those found in genomic sequences or medical imaging. In the educational sector, CGANNEK can revolutionize learning outcomes by generating personalized educational content tailored to individual students' needs. By embedding knowledge graphs into non-Euclidean spaces, CGANNEK can identify gaps in students' understanding and create adaptive learning materials that address these gaps effectively. This approach not only enhances student engagement but also improves the overall efficiency of the learning process. Furthermore, CGANNEK can facilitate the development of intelligent tutoring systems that provide real-time feedback and support, making education more accessible and equitable. The future prospects of CGANNEK in these fields are promising. As healthcare continues to adopt more sophisticated AI technologies, the ability of CGANNEK to handle complex data will become increasingly valuable. In education, the integration of CGANNEK with emerging technologies like augmented reality and virtual reality could create immersive learning environments that significantly boost student performance. Overall, the applications of CGANNEK in healthcare and education are poised to drive innovation, improve outcomes, and redefine the boundaries of what is possible in these critical sectors.
Future Developments and Innovations
**Future Developments and Innovations** As we delve into the applications and future prospects of CGANNEK, it becomes evident that this cutting-edge technology is poised to revolutionize various sectors. CGANNEK, or Conditional Generative Adversarial Networks for Non-Euclidean Knowledge Embedding, represents a significant leap forward in data representation and analysis. In the realm of artificial intelligence, CGANNEK is expected to enhance machine learning models by enabling more accurate and robust embeddings of complex data structures. One of the most promising future developments involves integrating CGANNEK with emerging technologies like quantum computing. This synergy could lead to unprecedented computational power, allowing for the processing of vast amounts of non-Euclidean data with unparalleled speed and accuracy. Additionally, advancements in edge computing will enable real-time applications of CGANNEK in IoT devices, fostering smarter and more responsive systems. In healthcare, CGANNEK is anticipated to play a crucial role in personalized medicine by generating detailed patient profiles from diverse datasets. This could lead to more precise diagnoses and tailored treatment plans. The technology also holds potential in drug discovery, where it can help identify novel compounds and predict their efficacy more accurately than traditional methods. The field of finance will also benefit significantly from CGANNEK. By analyzing complex financial networks and predicting market trends with higher precision, CGANNEK can help mitigate risks and optimize investment strategies. Furthermore, its application in cybersecurity will enhance threat detection capabilities by identifying anomalies in network traffic patterns more effectively. In terms of environmental sustainability, CGANNEK can be instrumental in monitoring and predicting climate changes by analyzing large-scale geospatial data. This could lead to more informed policy decisions and better resource management. The technology's ability to handle non-Euclidean data makes it particularly suited for tasks such as urban planning and smart city development. Moreover, CGANNEK's impact on education will be substantial. It can facilitate personalized learning experiences by generating adaptive educational content based on individual student profiles. This could revolutionize the way we approach education, making it more effective and engaging. As research continues to advance, we can expect even more innovative applications of CGANNEK across various industries. Its potential to transform how we process and understand complex data is vast, and its future developments promise to be nothing short of transformative. With ongoing innovations in hardware and software, the possibilities for CGANNEK are limitless, paving the way for a future where data-driven insights drive unprecedented progress and innovation.