What Is The Difference Between
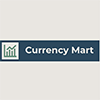
In the realm of comparative analysis, understanding the nuances between different concepts is crucial for informed decision-making and comprehensive knowledge. This article delves into the distinctions between two significant entities, highlighting their differences in definition and scope, methodology and approach, and the implications and consequences of these differences. By examining these three key areas, we can gain a deeper insight into how these entities function, their respective strengths and weaknesses, and how they impact various fields of study and practice. Starting with the foundational aspect, we will explore the differences in definition and scope, which sets the stage for understanding the broader context and applicability of each entity. This foundational understanding is essential as it influences how we perceive and utilize these concepts in real-world scenarios. Transitioning seamlessly from here, we will then delve into the distinctions in methodology and approach, followed by an analysis of the implications and consequences of each difference, providing a holistic view that is both informative and engaging.
Differences in Definition and Scope
In the realm of academic and professional discourse, the nuances of definition and scope are often overlooked, yet they play a crucial role in shaping our understanding and application of various concepts. This article delves into the differences in definition and scope, exploring how these variations impact our comprehension and utilization of ideas across different fields. To provide a comprehensive analysis, we will first examine the historical context in which these definitions and scopes have evolved, highlighting key milestones and influences that have shaped their development. Next, we will delve into theoretical frameworks that underpin these definitions, discussing how different schools of thought contribute to the diversity in scope and definition. Finally, we will explore practical applications, illustrating how these differences manifest in real-world scenarios and affect decision-making processes. By understanding these historical, theoretical, and practical dimensions, we can better appreciate the significance of differences in definition and scope, ultimately enhancing our ability to navigate complex intellectual landscapes effectively. This multifaceted approach will reveal the intricate dynamics at play, leading us to a deeper exploration of the differences in definition and scope.
1. Historical Context
The historical context of any concept or term is crucial in understanding its evolution, nuances, and the reasons behind its current definitions and scopes. When examining the differences in definition and scope of various terms, it is essential to delve into their historical backgrounds to uncover the influences, debates, and societal factors that have shaped their meanings over time. For instance, terms like "democracy" and "freedom" have undergone significant transformations since their inception in ancient Greece. Democracy, initially defined as direct rule by the people, has evolved to include representative forms where elected officials make decisions on behalf of the populace. This shift was influenced by the practical limitations of direct democracy in larger societies and the rise of modern nation-states. Similarly, the concept of freedom has expanded from its original focus on political liberty to encompass economic, social, and cultural dimensions. The Enlightenment thinkers such as John Locke and Jean-Jacques Rousseau played pivotal roles in broadening the scope of freedom, arguing for individual rights and the social contract. These philosophical underpinnings were later reinforced by historical events like the American and French Revolutions, which further solidified the notion of freedom as a fundamental human right. In contrast, terms related to science and technology often have their definitions and scopes refined through empirical research and technological advancements. For example, the term "atom" has undergone a radical transformation from its ancient Greek roots as an indivisible particle to the modern understanding of atomic structure, which includes protons, neutrons, and electrons. This evolution was driven by scientific discoveries and experiments conducted by scientists such as Dalton, Rutherford, and Bohr. Understanding these historical contexts not only provides a richer comprehension of how terms have been defined and redefined but also highlights the dynamic nature of language and knowledge. It underscores that definitions are not static entities but rather are shaped by ongoing debates, discoveries, and societal changes. By tracing the historical development of terms, we can better appreciate the complexities and nuances inherent in their current definitions and scopes, thereby fostering a more informed and nuanced discussion about their differences. This approach also encourages a critical examination of how historical contexts continue to influence contemporary understandings and applications of these terms in various fields.
2. Theoretical Frameworks
When delving into the differences in definition and scope between various concepts, it is crucial to establish a robust theoretical framework. Theoretical frameworks serve as the foundational structure that guides research, analysis, and interpretation. They provide a systematic way to understand and explain phenomena by outlining the relationships between variables and the underlying assumptions that shape our understanding. In the context of comparing definitions and scopes, two prominent theoretical frameworks often come into play: **Positivism** and **Constructivism**. Positivism, rooted in the scientific method, emphasizes empirical evidence and objective observation. It posits that knowledge can be derived from sensory experience and that there is a single, objective reality. This framework is particularly useful when comparing definitions that rely on quantifiable data or empirical research. For instance, when examining the differences between economic indicators such as GDP and GNP, a positivist approach would focus on the numerical values and statistical analyses to discern their distinct scopes. On the other hand, Constructivism takes a more subjective stance, arguing that knowledge is constructed through social interactions and cultural contexts. This framework acknowledges that reality is not fixed but is shaped by individual perceptions and collective agreements. Constructivism is invaluable when exploring definitions that are influenced by social norms, cultural values, or historical contexts. For example, when comparing the definitions of terms like "family" or "community," a constructivist approach would consider how these concepts are socially constructed and how their meanings vary across different cultures and societies. The interplay between these frameworks can also highlight the complexities of definitions. For instance, while a positivist might define "poverty" strictly in terms of economic metrics, a constructivist would argue that poverty encompasses not just financial status but also social exclusion, lack of access to resources, and subjective experiences of deprivation. This dual perspective enriches our understanding by revealing both the quantifiable aspects and the nuanced, context-dependent dimensions of the concept. Ultimately, the choice of theoretical framework depends on the nature of the concepts being compared and the research questions at hand. By leveraging both positivist and constructivist perspectives, researchers can develop a more comprehensive and balanced understanding of the differences in definition and scope, thereby enhancing the validity and relevance of their findings. This integrated approach ensures that the analysis is not only rigorous but also sensitive to the multifaceted nature of the phenomena under study.
3. Practical Applications
### Practical Applications The differences in definition and scope between various concepts often manifest in their practical applications, which can significantly impact how they are utilized and understood in real-world scenarios. For instance, when comparing the practical applications of **artificial intelligence (AI)** and **machine learning (ML)**, it becomes clear that while both are interconnected, they serve distinct purposes. AI, broadly defined, encompasses a wide range of techniques aimed at creating intelligent machines that can perform tasks typically requiring human intelligence. In contrast, ML is a subset of AI that focuses specifically on algorithms and statistical models enabling machines to learn from data without explicit programming. **In Healthcare:** AI is used for comprehensive patient care management, including diagnosis, treatment planning, and patient monitoring. For example, AI systems can analyze medical images to detect diseases like cancer at an early stage. On the other hand, ML algorithms are employed to predict patient outcomes based on historical data, helping healthcare providers make informed decisions. This distinction is crucial because while AI provides a holistic approach to healthcare management, ML enhances the precision and accuracy of specific medical tasks. **In Finance:** AI is applied in risk management and fraud detection across financial institutions. It can analyze vast amounts of data to identify patterns indicative of fraudulent activities. Meanwhile, ML models are used for credit scoring and portfolio optimization. These models learn from historical financial data to predict creditworthiness and optimize investment portfolios. Here again, the broader scope of AI allows for comprehensive risk assessment, while ML's focus on learning from data improves the accuracy of financial predictions. **In Education:** AI can personalize learning experiences by adapting educational content to individual students' needs and learning styles. This includes intelligent tutoring systems that offer real-time feedback. In contrast, ML is used to develop adaptive learning platforms that adjust the difficulty level of course materials based on student performance data. This differentiation highlights how AI enhances the overall educational experience through personalization, whereas ML fine-tunes the learning process through data-driven insights. Understanding these differences in practical applications is essential for leveraging these technologies effectively. By recognizing where AI provides a broad framework and where ML offers specialized capabilities, organizations can implement these technologies more strategically, leading to improved outcomes across various sectors. This nuanced understanding not only enhances the efficiency of these technologies but also ensures they are aligned with specific goals and objectives, thereby maximizing their potential impact.
Distinctions in Methodology and Approach
In the realm of academic and professional research, the methodologies and approaches employed can significantly impact the validity, reliability, and overall quality of the findings. This article delves into the distinctions in methodology and approach, highlighting three critical areas: research methods, analytical techniques, and data interpretation. Each of these components plays a pivotal role in shaping the research process and influencing the outcomes. By examining the various research methods, we can understand how different strategies for data collection and study design affect the scope and depth of research. Analytical techniques, on the other hand, reveal how data is processed and analyzed to extract meaningful insights. Finally, data interpretation underscores the importance of correctly understanding and presenting the results. These distinctions are not merely technical nuances but fundamental aspects that define the integrity and applicability of research. Understanding these differences is crucial for navigating the complexities of research and ensuring that studies are conducted with rigor and relevance. This exploration sets the stage for a deeper discussion on the differences in definition and scope, which are essential for aligning research objectives with appropriate methodologies.
1. Research Methods
When delving into the distinctions in methodology and approach, it is crucial to understand the various research methods that underpin these differences. Research methods are the systematic procedures used to collect, analyze, and interpret data, and they form the backbone of any scientific inquiry. There are primarily two types of research methods: qualitative and quantitative. **Qualitative Research Methods** focus on gaining a deeper understanding of the research subject through non-numerical data. These methods involve techniques such as interviews, focus groups, case studies, and content analysis. Qualitative research is particularly useful for exploring complex phenomena, understanding social dynamics, and capturing nuanced perspectives. For instance, ethnographic studies allow researchers to immerse themselves in a culture or community to gain rich, contextual insights that might not be captured through other means. **Quantitative Research Methods**, on the other hand, rely on numerical data and statistical analysis to draw conclusions. These methods include surveys, experiments, and statistical modeling. Quantitative research is ideal for testing hypotheses, measuring variables, and generalizing findings to larger populations. For example, randomized controlled trials (RCTs) are considered the gold standard in medical research because they provide robust evidence of cause-and-effect relationships between treatments and outcomes. **Mixed Methods** combine elements of both qualitative and quantitative approaches to leverage the strengths of each. This hybrid approach allows researchers to validate findings through multiple lenses, enhancing the reliability and validity of the results. Mixed methods are increasingly popular as they offer a more comprehensive understanding of complex research questions. The choice of research method depends on the research question, the nature of the data, and the goals of the study. Each method has its own set of advantages and limitations. For instance, qualitative methods provide depth but may lack generalizability, while quantitative methods offer breadth but might miss contextual nuances. Understanding these distinctions is essential for selecting the appropriate methodology and ensuring that the research design aligns with the objectives of the study. In addition to these primary categories, there are also **Action Research** and **Participatory Action Research**, which involve active engagement with the research participants to bring about change or improvement. These approaches are particularly relevant in fields like education, social work, and community development. Ultimately, the selection of a research method is not just a technical decision but also a philosophical one, reflecting the researcher's worldview and epistemological stance. By carefully choosing and combining different research methods, researchers can ensure that their studies are rigorous, relevant, and contribute meaningfully to the body of knowledge in their field. This thoughtful approach to methodology is critical for maintaining the integrity and impact of research findings.
2. Analytical Techniques
In the realm of analytical techniques, distinctions in methodology and approach are crucial for achieving accurate and meaningful results. Analytical techniques are the backbone of various scientific disciplines, including chemistry, biology, and physics, each requiring specific methods tailored to their unique objectives. For instance, in chemistry, techniques such as chromatography and spectroscopy are widely employed. Chromatography, which involves separating mixtures based on differential affinities towards a stationary phase and a mobile phase, is essential for identifying and quantifying components in complex samples. Spectroscopy, on the other hand, relies on the interaction between matter and electromagnetic radiation to provide detailed information about molecular structures and compositions. These techniques differ significantly in their underlying principles and applications; chromatography is often used for separation and purification, while spectroscopy is used for identification and structural analysis. In biology, techniques like PCR (Polymerase Chain Reaction) and sequencing are pivotal. PCR amplifies specific DNA sequences, allowing for the detection of genetic material even when present in minute quantities. Sequencing, whether it be Sanger sequencing or next-generation sequencing (NGS), provides detailed information about the nucleotide sequence of DNA or RNA molecules. These biological techniques are distinct from chemical ones due to their focus on genetic material rather than molecular structures. The choice of analytical technique also depends on the scale and complexity of the analysis. For example, in environmental science, techniques such as gas chromatography-mass spectrometry (GC-MS) and inductively coupled plasma mass spectrometry (ICP-MS) are used to analyze trace levels of pollutants in water and air samples. These methods offer high sensitivity and specificity but require sophisticated instrumentation and expertise. Moreover, the approach to data analysis can vary significantly depending on the technique used. Quantitative techniques like chromatography often involve calibration curves and statistical analysis to ensure accuracy and precision. Qualitative techniques such as spectroscopy may involve pattern recognition and comparison with reference spectra. In biological analyses, bioinformatics tools are frequently used to interpret sequencing data, aligning reads to reference genomes or identifying genetic variations. In summary, the distinctions in analytical techniques are rooted in their methodologies, applications, and the type of data they generate. Understanding these differences is essential for selecting the appropriate technique for a given problem, ensuring that the results are reliable and meaningful. Whether in chemistry, biology, or other fields, the meticulous choice of analytical technique is a cornerstone of scientific inquiry and discovery.
3. Data Interpretation
When it comes to distinctions in methodology and approach, data interpretation stands out as a critical component that can significantly influence the outcomes of any research or analysis. Data interpretation involves the process of assigning meaning to the data collected, which can vary widely depending on the methodology and approach employed. For instance, in quantitative research, data interpretation often relies on statistical analysis to derive conclusions. Here, researchers use tools like regression analysis, hypothesis testing, and confidence intervals to interpret numerical data. This approach is particularly useful in fields such as economics, medicine, and social sciences where precise measurements are crucial. In contrast, qualitative research adopts a more interpretive approach to data interpretation. This methodology focuses on understanding the meaning and context behind the data, often through techniques like thematic analysis, content analysis, or narrative analysis. Qualitative researchers may use open-ended interviews, focus groups, or observational studies to gather rich, detailed data that provides insights into people's experiences and perspectives. This approach is commonly used in fields such as anthropology, sociology, and education where understanding complex social phenomena is key. Another significant distinction lies in the use of mixed methods research, which combines both quantitative and qualitative approaches to data interpretation. This hybrid methodology allows researchers to leverage the strengths of each approach—quantitative methods for generalizability and qualitative methods for depth and context. Mixed methods research can provide a more comprehensive understanding of a research question by integrating numerical data with narrative insights. For example, in healthcare research, mixed methods might be used to analyze patient outcomes (quantitative) alongside patient experiences and perceptions (qualitative), offering a holistic view of healthcare effectiveness. The choice of methodology and approach in data interpretation also impacts the validity and reliability of the findings. Quantitative methods are often praised for their objectivity and replicability but may lack the nuance needed to capture complex social dynamics. On the other hand, qualitative methods offer rich contextual insights but may be criticized for their subjectivity and limited generalizability. Mixed methods aim to balance these trade-offs by triangulating different types of data to enhance the robustness of the findings. Ultimately, the distinction in data interpretation methodologies reflects broader philosophical differences about what constitutes knowledge and how it should be pursued. Understanding these differences is essential for researchers to select an appropriate methodology that aligns with their research questions and objectives, ensuring that their interpretations are accurate, meaningful, and contribute significantly to their field of study. By acknowledging and respecting these distinctions, researchers can produce high-quality, impactful research that advances our understanding of various phenomena across diverse disciplines.
Implications and Consequences of Each Difference
In the realm of academic and policy discourse, the nuances of definitions and scopes can have far-reaching implications and consequences. These differences, often subtle yet profound, influence various aspects of our societal fabric. This article delves into the multifaceted impacts of such disparities, exploring how they shape policy making, public perception, and future research. The distinctions in definitions and scopes can significantly alter the trajectory of policy decisions, affecting the allocation of resources and the implementation of laws. Additionally, these variations can sway public opinion, influencing how issues are perceived and addressed by the general populace. Furthermore, they play a crucial role in guiding future research, determining the focus and methodologies employed by scholars. By examining these three critical areas—impact on policy making, effects on public perception, and influence on future research—we can better understand the broader implications of differences in definition and scope. This analysis underscores the importance of clarity and consistency in these foundational elements, highlighting their transactional relationship with the broader landscape of differences in definition and scope.
1. Impact on Policy Making
The impact on policy making is a critical dimension where differences in various contexts can have profound implications. When examining the distinctions between different approaches, ideologies, or systems, it becomes evident that these variations significantly influence the policy-making process. For instance, in the realm of economic policy, the divergence between Keynesian and neoliberal ideologies shapes fiscal and monetary policies in fundamentally different ways. Keynesian policies often advocate for government intervention during economic downturns to stimulate growth, whereas neoliberal policies emphasize market forces and minimal government interference. This dichotomy can lead to vastly different policy outcomes; Keynesian policies might result in increased public spending and social welfare programs, while neoliberal policies could lead to deregulation and tax cuts. Similarly, in environmental policy, the contrast between proactive and reactive approaches can have far-reaching consequences. Proactive policies, such as those championed by the European Union's Green Deal, focus on preventive measures and long-term sustainability goals. These policies often involve stringent regulations, investment in renewable energy, and carbon pricing mechanisms. On the other hand, reactive policies tend to address environmental issues after they have become severe problems. This reactive approach can lead to more costly and less effective solutions, exacerbating environmental degradation. In healthcare policy, the differences between universal healthcare systems and private insurance models also have significant implications. Universal healthcare systems, like those in many European countries, ensure comprehensive coverage for all citizens, often resulting in better health outcomes and lower administrative costs. In contrast, private insurance models prevalent in countries like the United States can lead to higher healthcare costs, unequal access to care, and a greater burden on individuals and families. Moreover, differences in political systems—such as democratic versus authoritarian regimes—can drastically alter the policy-making landscape. Democratic systems typically involve more transparent and participatory processes, allowing for diverse voices to be heard and represented. This can lead to more inclusive and equitable policies. Authoritarian regimes, however, often centralize power and suppress dissent, resulting in policies that may benefit a select few at the expense of the broader population. In conclusion, the differences that arise from various approaches, ideologies, and systems have profound impacts on policy making. These variations not only shape the content of policies but also influence their effectiveness, equity, and long-term consequences. Understanding these differences is crucial for policymakers and stakeholders seeking to create policies that are responsive to societal needs and conducive to sustainable development. By acknowledging and addressing these disparities, we can work towards more informed decision-making processes that ultimately benefit the greater good.
2. Effects on Public Perception
The differences between various concepts, whether they be scientific theories, political ideologies, or technological advancements, can have profound effects on public perception. Public perception is shaped by how information is presented, the sources of that information, and the cultural context in which it is received. For instance, in the realm of science, the distinction between climate change and global warming significantly influences public understanding and concern. Climate change encompasses a broader range of environmental impacts, including rising sea levels and extreme weather events, whereas global warming specifically refers to the increase in Earth's average surface temperature. This distinction can lead to varying levels of urgency and action among the public; those who understand the broader implications of climate change are more likely to advocate for comprehensive environmental policies compared to those who focus solely on global warming. Similarly, in politics, the differences between socialism and communism can dramatically alter public perception due to historical associations and media portrayals. Socialism is often viewed as a more moderate ideology that seeks to balance economic equality with individual freedoms, while communism is frequently associated with authoritarian regimes and the suppression of personal liberties. These perceptions can sway public opinion on policy issues such as healthcare reform or economic redistribution, with socialism generally receiving more support in democratic societies due to its perceived compatibility with democratic values. Technological advancements also play a crucial role in shaping public perception. The distinction between artificial intelligence (AI) and machine learning (ML) is a case in point. AI is often seen as a broader field that includes ML but also encompasses other areas like natural language processing and robotics. ML, on the other hand, is a subset of AI focused on algorithms that allow systems to learn from data without being explicitly programmed. The general public may view AI with a mix of awe and apprehension due to its potential to transform various aspects of life, while ML is seen as a more tangible and less intimidating concept because it is more narrowly defined. In each of these examples, the nuances in terminology and definition significantly impact how the public perceives and responds to these concepts. Clear communication and accurate representation of these differences are essential for fostering informed public discourse and ensuring that policies and actions are based on a thorough understanding of the issues at hand. Misunderstandings or conflations of these terms can lead to misinformation, mistrust, and ineffective solutions, highlighting the importance of precise language in shaping public perception and driving meaningful change.
3. Influence on Future Research
The influence on future research stemming from the differences between various concepts or methodologies is profound and multifaceted. Understanding these distinctions not only clarifies current knowledge gaps but also sets the stage for innovative investigations. For instance, in fields like psychology, the differentiation between nature and nurture has significant implications for research design. Recognizing that genetic predispositions interact with environmental factors can lead researchers to adopt more holistic approaches, integrating both biological and social sciences. This interdisciplinary perspective can uncover complex mechanisms underlying human behavior, fostering a more comprehensive understanding of mental health and development. In the realm of technology, distinctions between artificial intelligence (AI) and machine learning (ML) are crucial for advancing computational capabilities. While AI encompasses a broader spectrum of intelligent systems, ML is a subset focused on algorithms that learn from data. This distinction guides researchers in developing more specialized ML models that can adapt to diverse datasets, thereby enhancing predictive accuracy and decision-making processes. Furthermore, this clarity helps in addressing ethical concerns related to AI, such as bias and transparency, by focusing on the specific ML techniques that need improvement. In environmental science, the differences between climate change and global warming have critical implications for policy-making and research agendas. Climate change refers to broader alterations in Earth's climate system, including temperature fluctuations, while global warming specifically pertains to the increase in global temperatures. This distinction allows researchers to target their studies more effectively, whether it's investigating the impacts of rising temperatures on ecosystems or examining the broader climatic shifts that influence weather patterns. By focusing on these nuances, scientists can provide more precise data and recommendations for mitigating climate-related issues, thereby informing more effective policy interventions. Moreover, these differences often highlight areas where current methodologies may be insufficient or outdated. For example, in medical research, the distinction between correlation and causation is essential for validating treatment efficacy. Recognizing that correlation does not imply causation prompts researchers to employ more rigorous experimental designs, such as randomized controlled trials, to establish causal relationships between treatments and outcomes. This ensures that new therapies are based on robust evidence, enhancing patient safety and treatment effectiveness. In conclusion, understanding the differences between various concepts and methodologies has a profound influence on future research. It enables scientists to refine their approaches, address knowledge gaps more precisely, and develop innovative solutions to complex problems. By acknowledging these distinctions, researchers can advance their fields with greater accuracy and relevance, ultimately contributing to a deeper understanding of the world and better solutions for its challenges.