What Does N Mean In Statistics
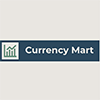
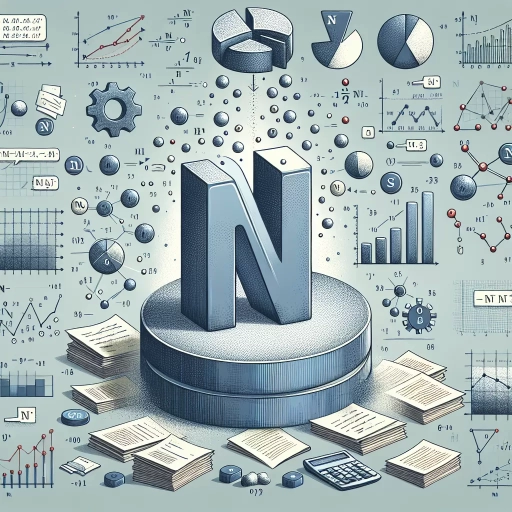
In the realm of statistics, the symbol 'n' holds a pivotal role, yet its significance can often be overlooked by those not deeply entrenched in statistical analysis. This article aims to illuminate the multifaceted nature of 'n', delving into its fundamental concept, its myriad applications, and the practical considerations that accompany its use. Understanding the concept of 'n' is crucial as it represents the sample size or the number of observations in a dataset, which directly influences the reliability and precision of statistical results. Beyond its definition, 'n' plays a critical role in various statistical methods, from hypothesis testing to confidence intervals, where its value can significantly impact the outcomes. However, practical considerations and challenges arise when determining an appropriate sample size, balancing between resource constraints and the need for accurate results. By exploring these aspects, this article will provide a comprehensive overview of 'n' in statistics, starting with a detailed examination of **Understanding the Concept of 'n' in Statistics**.
Understanding the Concept of 'n' in Statistics
Understanding the concept of 'n' in statistics is a foundational element that underpins the accuracy and reliability of statistical analyses. This crucial concept is multifaceted, encompassing several key aspects that are essential for any researcher or analyst to grasp. First, it is vital to delve into the **Definition and Basic Principles** of 'n', which involves understanding what 'n' represents in different statistical contexts. This foundational knowledge sets the stage for more advanced discussions. Next, distinguishing between **Types of 'n' (Sample Size vs. Population Size)** is critical, as these two concepts have distinct implications for data analysis and inference. Finally, recognizing the **Importance in Statistical Analysis** highlights how 'n' influences the power of tests, confidence intervals, and overall study validity. By exploring these three dimensions, one can gain a comprehensive understanding of how 'n' shapes statistical outcomes. Let us begin by examining the **Definition and Basic Principles** of 'n', which form the bedrock of statistical reasoning.
Definition and Basic Principles
In the realm of statistics, the concept of 'n' is fundamental and serves as a cornerstone for various statistical analyses. **Definition and Basic Principles** of 'n' are crucial for understanding its role and significance. Essentially, 'n' represents the sample size, which is the number of observations or data points in a sample. This sample is typically drawn from a larger population to make inferences about that population. The definition is straightforward: 'n' is the count of individual data points included in the analysis. The basic principles surrounding 'n' are rooted in statistical theory and practice. First, the sample size 'n' directly influences the precision and reliability of statistical estimates. A larger sample size generally leads to more accurate estimates because it reduces the standard error, thereby increasing the confidence in the results. This principle is encapsulated in the Central Limit Theorem (CLT), which states that as 'n' increases, the distribution of sample means approaches a normal distribution, regardless of the original population distribution. Another key principle is that 'n' affects the power of statistical tests. A higher 'n' provides greater statistical power, meaning it increases the likelihood of detecting significant differences or relationships if they exist. This is particularly important in hypothesis testing, where a larger sample size can help avoid Type II errors (failing to reject a false null hypothesis). Moreover, 'n' plays a critical role in determining the degrees of freedom in various statistical tests, such as t-tests and chi-square tests. Degrees of freedom are essential for selecting the appropriate critical values from statistical tables or using software to compute p-values. Incorrectly specifying 'n' can lead to incorrect conclusions about statistical significance. In practical terms, choosing an appropriate sample size 'n' involves balancing several factors, including cost, time constraints, and ethical considerations. Researchers often use power analysis to determine the minimum sample size required to detect a specified effect size with a desired level of power. This ensures that the study is adequately powered to answer the research question without wasting resources on an unnecessarily large sample. Understanding these principles helps statisticians and researchers design studies that are both efficient and effective. By carefully considering 'n', they can ensure that their analyses are robust, reliable, and capable of providing meaningful insights into the phenomena being studied. In summary, 'n' is not just a number; it is a critical component that underpins the validity and reliability of statistical analyses, making it an essential concept to grasp in the field of statistics.
Types of 'n' (Sample Size vs. Population Size)
In the realm of statistics, the concept of 'n' is pivotal and can refer to two distinct yet interconnected entities: sample size and population size. Understanding the difference between these two is crucial for accurate statistical analysis and interpretation. **Sample Size (n):** The sample size, denoted by 'n', represents the number of observations or data points selected from a larger population for the purpose of statistical analysis. This subset is chosen to make inferences about the characteristics of the entire population. For instance, if a researcher wants to understand the average height of adults in a country, they might select a random sample of 1,000 individuals to measure and analyze. Here, 'n' would be 1,000. The sample size is critical because it influences the precision and reliability of statistical estimates. A larger sample size generally provides more accurate estimates but also increases the cost and complexity of data collection. **Population Size (N):** In contrast, the population size, often represented by 'N', refers to the total number of individuals or units within the entire group of interest. Using the previous example, if the researcher aims to understand the average height of all adults in a country with a population of 50 million, then 'N' would be 50 million. The population size is essential for understanding the scope and context of the study. It helps in determining the representativeness of the sample and in calculating various statistical measures such as sampling error and confidence intervals. **Relationship Between Sample and Population Sizes:** The relationship between 'n' and 'N' is significant. When 'n' is a small fraction of 'N', it is generally easier to ensure that the sample is representative of the population. However, as 'n' approaches 'N', the sample becomes less distinguishable from the population itself, and the distinction between sample statistics and population parameters becomes less relevant. For example, if a researcher were to measure the heights of nearly all adults in a country (i.e., 'n' is close to 'N'), then the sample mean would be very close to the population mean. **Practical Considerations:** In practice, selecting an appropriate sample size involves balancing several factors including cost, time, and desired precision. Statistical power analysis can help determine an optimal 'n' that ensures reliable results without unnecessarily increasing the burden of data collection. Additionally, understanding both 'n' and 'N' is vital for avoiding common pitfalls such as overgeneralization or underestimation of variability within the population. In summary, while 'n' (sample size) and 'N' (population size) are distinct concepts in statistics, they are interrelated and both play crucial roles in statistical analysis. Accurately defining and understanding these terms is essential for conducting robust research and making informed decisions based on data. By recognizing the differences and relationships between sample and population sizes, researchers can ensure that their findings are reliable, generalizable, and meaningful.
Importance in Statistical Analysis
Statistical analysis is a cornerstone of modern research and decision-making, and its importance cannot be overstated. At the heart of statistical analysis lies the concept of 'n,' which represents the sample size or the number of observations in a dataset. Understanding 'n' is crucial because it directly influences the reliability, precision, and generalizability of statistical findings. Here’s why statistical analysis, particularly with a focus on 'n,' is so vital: Firstly, statistical analysis provides a systematic way to extract meaningful insights from data, which is essential in various fields such as medicine, social sciences, economics, and engineering. By analyzing data through statistical methods, researchers can identify patterns, trends, and correlations that would otherwise remain hidden. For instance, in clinical trials, understanding 'n' helps determine whether the sample size is sufficient to detect significant differences between treatment groups, thereby ensuring that conclusions drawn are reliable and not due to chance. Secondly, the accuracy of statistical inferences heavily depends on the sample size 'n.' A larger 'n' generally leads to more precise estimates and narrower confidence intervals, which are critical for making informed decisions. For example, in market research, a larger sample size can provide a more accurate representation of consumer preferences, allowing businesses to tailor their strategies effectively. Conversely, a small 'n' may result in biased or misleading conclusions that could have detrimental consequences. Thirdly, statistical analysis enables the quantification of uncertainty through measures like standard error and confidence intervals. These metrics are directly influenced by 'n,' highlighting the importance of adequate sample sizes in reducing uncertainty and increasing the confidence in findings. In policy-making, for instance, policymakers rely on robust statistical analyses to inform their decisions; a well-chosen 'n' ensures that policies are based on solid evidence rather than speculative assumptions. Moreover, statistical analysis facilitates the comparison of different groups or conditions. The concept of 'n' plays a pivotal role here as it affects the power of statistical tests to detect differences or effects. In educational research, comparing the performance of different teaching methods requires an appropriate 'n' to ensure that any observed differences are statistically significant and not merely due to random variation. Finally, understanding 'n' is essential for ethical considerations in research. Ensuring an adequate sample size helps avoid unnecessary exposure of participants to risks in studies where ethical concerns are paramount. For example, in medical research involving human subjects, an appropriate 'n' ensures that the study is neither underpowered (failing to detect real effects) nor overpowered (exposing more participants than necessary to potential harm). In conclusion, the importance of statistical analysis cannot be overstated, and the concept of 'n' is central to this importance. By understanding and appropriately selecting 'n,' researchers can ensure that their findings are reliable, precise, and generalizable. This, in turn, enhances the validity and utility of statistical analyses across diverse fields, ultimately contributing to better decision-making and more informed policies.
Applications of 'n' in Statistical Methods
The application of 'n' in statistical methods is a cornerstone of data analysis, enabling researchers to draw reliable conclusions from their data. This crucial parameter, often representing the sample size, plays a pivotal role in various statistical techniques. In this article, we will delve into three key areas where 'n' is indispensable: Sample Size Determination, Confidence Intervals and Margin of Error, and Hypothesis Testing and Significance Levels. Understanding how 'n' influences these methods is essential for ensuring the validity and precision of statistical analyses. For instance, determining an appropriate sample size ('n') is critical for achieving reliable estimates and generalizing findings to the population. This foundational step sets the stage for subsequent analyses, such as constructing confidence intervals and margins of error, which rely on 'n' to quantify uncertainty. Furthermore, 'n' impacts the power and significance levels in hypothesis testing, affecting the ability to detect true effects. By examining these applications, we can appreciate the multifaceted importance of 'n' in statistical methods. Let us begin by exploring how 'n' is determined in Sample Size Determination, a fundamental step that underpins all subsequent statistical analyses.
Sample Size Determination
Sample size determination is a critical component in statistical analysis, ensuring that the data collected is sufficient to draw reliable and accurate conclusions. The process involves calculating the number of observations or participants needed to achieve a desired level of precision and power in a study. This calculation depends on several key factors, including the desired margin of error, the confidence level, the variability of the data (often measured by standard deviation), and the effect size one wishes to detect. For instance, in hypothesis testing, a larger sample size increases the power of the test, making it more likely to detect a statistically significant effect if one exists. Conversely, a small sample size may lead to Type II errors, where a true effect is missed due to insufficient data. In surveys and opinion polls, determining an appropriate sample size helps ensure that the results are representative of the population and can be generalized with confidence. The formula for sample size determination varies depending on the type of study. For example, in estimating population proportions, the sample size \(n\) can be calculated using the formula \(n = \frac{(Z^2 \cdot p \cdot (1-p))}{E^2}\), where \(Z\) is the Z-score corresponding to the desired confidence level, \(p\) is the estimated proportion, and \(E\) is the margin of error. For continuous data, such as means, the formula incorporates the standard deviation and desired precision. In practice, researchers often use software tools or statistical tables to facilitate these calculations. Additionally, considerations such as cost constraints, ethical limitations, and practical feasibility must be balanced against statistical requirements. For instance, while a larger sample size may provide more precise estimates, it may also increase costs and logistical challenges. Moreover, sample size determination is not a one-time process; it can be iterative. Pilot studies or preliminary data analysis may be used to refine initial estimates and adjust the sample size accordingly. This adaptive approach ensures that resources are optimized while maintaining statistical rigor. In summary, sample size determination is an essential step in statistical research that ensures studies are adequately powered to detect meaningful effects and provide reliable results. By carefully considering the various factors involved and using appropriate statistical tools, researchers can ensure their findings are robust and generalizable, thereby enhancing the validity and impact of their work. This meticulous approach underscores the importance of 'n' in statistical methods, as it directly influences the reliability and applicability of research outcomes.
Confidence Intervals and Margin of Error
In the realm of statistical analysis, understanding **Confidence Intervals** and **Margin of Error** is crucial for interpreting data accurately and making informed decisions. These concepts are intricately linked to the sample size, denoted by 'n', which plays a pivotal role in determining the reliability and precision of statistical estimates. A **Confidence Interval** provides a range of values within which a population parameter is likely to lie, with a specified level of confidence. For instance, if we are estimating the average height of a population based on a sample, a 95% confidence interval might suggest that the true average height lies between 170 cm and 180 cm. This interval is constructed using the sample mean and standard deviation, along with the critical value from a standard normal distribution or t-distribution, depending on the sample size. The **Margin of Error**, often denoted as 'E', is the maximum amount by which the sample statistic is expected to differ from the true population parameter. It is directly influenced by the sample size 'n'. A larger sample size reduces the margin of error, thereby increasing the precision of the estimate. Mathematically, the margin of error can be calculated using the formula \( E = z_{\alpha/2} \times \frac{\sigma}{\sqrt{n}} \), where \( z_{\alpha/2} \) is the critical value from the standard normal distribution corresponding to the desired confidence level, \( \sigma \) is the population standard deviation, and 'n' is the sample size. For example, if we want to estimate the average income of a city with a 95% confidence level and a margin of error of $1,000, increasing 'n' from 100 to 400 would significantly reduce the margin of error, making our estimate more reliable. The interplay between 'n', confidence intervals, and margin of error is vital in various applications across fields such as medicine, social sciences, and business. In clinical trials, for instance, researchers need to determine an appropriate sample size to ensure that their findings are statistically significant and reliable. A larger 'n' allows for narrower confidence intervals and smaller margins of error, which are essential for drawing accurate conclusions about treatment efficacy or safety. Similarly, in market research, companies use confidence intervals to estimate consumer preferences or market trends with a certain degree of precision. Here again, the choice of 'n' is critical as it directly affects the reliability and generalizability of the results. In summary, understanding how 'n' influences confidence intervals and margin of error is fundamental in statistical analysis. By carefully selecting an appropriate sample size, researchers can ensure that their estimates are precise and reliable, thereby facilitating better decision-making processes across diverse disciplines. This underscores the importance of 'n' in statistical methods, as it serves as a cornerstone for constructing robust and meaningful statistical inferences.
Hypothesis Testing and Significance Levels
Hypothesis testing is a cornerstone of statistical analysis, allowing researchers to make informed decisions about the validity of their hypotheses. At the heart of this process lies the concept of significance levels, which determine the threshold for rejecting a null hypothesis. In essence, hypothesis testing involves formulating two hypotheses: the null hypothesis (H0), which states that there is no effect or no difference, and the alternative hypothesis (H1), which posits the presence of an effect or difference. The significance level, often denoted as alpha (α), is the maximum probability of rejecting the null hypothesis when it is actually true—a Type I error. When conducting hypothesis tests, researchers must carefully select an appropriate significance level. Commonly, α is set to 0.05, meaning there is a 5% chance of rejecting the null hypothesis if it is true. However, this value can be adjusted based on the context and desired level of precision. For instance, in fields like medicine where false positives could have severe consequences, a lower α might be chosen to ensure greater confidence in the results. The significance level directly influences the critical region of the test statistic distribution. If the calculated test statistic falls within this critical region, the null hypothesis is rejected in favor of the alternative hypothesis. This decision is typically made by comparing the p-value—a measure of how likely it is to observe the test results assuming the null hypothesis is true—to the chosen significance level. If the p-value is less than α, the null hypothesis is rejected. Understanding and correctly applying significance levels are crucial for drawing accurate conclusions from data. Misinterpretation can lead to incorrect decisions and potentially misleading results. For example, if a study finds a statistically significant result at α = 0.05 but fails to account for multiple testing or other sources of error, it may overstate its findings. In practical applications across various fields such as psychology, economics, and biology, hypothesis testing with well-defined significance levels helps ensure that conclusions are based on robust evidence rather than chance occurrences. For instance, in clinical trials evaluating new treatments, hypothesis testing with stringent significance levels helps safeguard against approving ineffective or harmful treatments. Moreover, the sample size 'n' plays a critical role in hypothesis testing. A larger sample size generally increases the power of the test to detect true effects while maintaining control over Type I errors. This is because larger samples provide more precise estimates and reduce variability in test statistics. Therefore, when designing studies or experiments involving hypothesis testing, researchers must carefully consider both their desired significance level and required sample size to ensure reliable outcomes. In summary, hypothesis testing coupled with appropriate significance levels forms a powerful tool for statistical inference. By understanding how to set and interpret these levels correctly within the context of their research questions and sample sizes ('n'), researchers can make well-informed decisions that contribute meaningfully to their fields of study. This integration underscores the importance of 'n' in statistical methods as it directly impacts the reliability and validity of hypothesis testing outcomes.
Practical Considerations and Challenges
When delving into the practical considerations and challenges of any research or data-driven project, several key factors must be meticulously addressed to ensure the integrity and reliability of the findings. First, **Sampling Bias and Representativeness** are crucial as they directly impact the generalizability of the results. Ensuring that the sample is representative of the population is essential to avoid skewed outcomes. Second, **Resource Constraints and Cost Implications** often pose significant barriers, as limited resources can restrict the scope and quality of the data collection process. Lastly, **Statistical Power and Precision** are vital for drawing accurate conclusions, as inadequate sample sizes or poor measurement tools can lead to misleading results. These considerations are interwoven and must be balanced carefully. For instance, resource constraints might force compromises on sample size, which in turn affects statistical power. Similarly, ensuring representativeness can be resource-intensive, highlighting the need for careful planning and allocation of resources. Understanding these challenges is fundamental to conducting rigorous and meaningful research. By acknowledging these practical considerations, researchers can better navigate the complexities involved and produce high-quality, reliable data. This begins with a thorough examination of **Sampling Bias and Representativeness**, as this foundational aspect sets the stage for all subsequent analyses.
Sampling Bias and Representativeness
**Sampling Bias and Representativeness** When delving into the realm of statistical analysis, particularly in the context of understanding what "n" means in statistics, it is crucial to address the critical concepts of sampling bias and representativeness. These elements are foundational to ensuring that any statistical inference drawn from a sample accurately reflects the characteristics of the population from which it is derived. Sampling bias occurs when the sample collected is not representative of the population, leading to skewed or misleading results. This can arise from various sources, including selection bias, where certain groups are more likely to be included or excluded from the sample, and non-response bias, where participants may not respond truthfully or at all. Representativeness is the cornerstone of reliable statistical analysis. A representative sample is one that mirrors the demographic, behavioral, and other relevant characteristics of the population. Achieving representativeness involves careful consideration of sampling methods, such as random sampling, stratified sampling, and cluster sampling, each designed to minimize bias and ensure that every member of the population has an equal chance of being selected. However, even with meticulous planning, challenges persist. For instance, in real-world scenarios, it may be impractical or impossible to achieve perfect representativeness due to constraints such as time, budget, and accessibility. Practical considerations further complicate the issue. In many cases, researchers must balance the need for a large sample size (denoted by "n" in statistical notation) with the feasibility of data collection. A larger sample size generally provides more reliable estimates but also increases costs and logistical challenges. Moreover, ensuring that participants are willing and able to participate can introduce additional biases if certain groups are systematically underrepresented or overrepresented. Addressing these challenges requires a multifaceted approach. Researchers must employ robust sampling techniques and validate their samples against known population parameters whenever possible. Weighting and adjustment methods can also be used to correct for identified biases post-data collection. Furthermore, transparency about potential biases and limitations is essential for maintaining the integrity of statistical findings. In conclusion, understanding and mitigating sampling bias while striving for representativeness are essential practical considerations in statistical analysis. By recognizing these challenges and implementing strategies to overcome them, researchers can enhance the validity and reliability of their findings, ensuring that "n" truly represents a meaningful and accurate snapshot of the population under study. This meticulous attention to detail is paramount for drawing valid inferences and making informed decisions based on statistical data.
Resource Constraints and Cost Implications
Resource constraints and cost implications are pivotal considerations when delving into the practical challenges of statistical analysis, particularly in understanding what "n" means in statistics. The term "n" refers to the sample size, which is a critical factor in determining the reliability and generalizability of statistical findings. However, obtaining an adequate sample size is often hampered by resource constraints. For instance, collecting data from a large sample can be time-consuming and expensive, especially if it involves surveys, interviews, or experiments that require significant human and material resources. These constraints can lead to smaller sample sizes, which may result in less precise estimates and reduced statistical power to detect significant effects. Moreover, the cost implications of resource constraints extend beyond just financial expenditure. Time is another valuable resource; researchers may have limited time to collect and analyze data, which can compromise the quality of the study. Additionally, ethical considerations may impose constraints on sample size; for example, studies involving human subjects must adhere to strict ethical guidelines that may limit the number of participants. These constraints can force researchers to make trade-offs between sample size and other aspects of study design, such as the depth of data collection or the complexity of analysis. The interplay between resource constraints and cost implications also affects the choice of statistical methods. For example, certain advanced statistical techniques require large datasets to produce reliable results, but smaller samples might necessitate simpler methods that are less robust. This can lead to a trade-off between the accuracy of findings and the feasibility of data collection. Furthermore, resource limitations can influence the decision to use secondary data or rely on existing datasets, which may not perfectly align with the research question but offer a more cost-effective solution. In practical terms, these challenges underscore the importance of careful planning and resource allocation in statistical research. Researchers must balance the need for robust statistical analysis with the realities of limited resources. This involves setting clear research objectives, selecting appropriate statistical methods that are feasible given the available resources, and ensuring that data collection protocols are efficient and effective. By acknowledging and addressing these resource constraints and cost implications upfront, researchers can enhance the validity and reliability of their findings despite these challenges. Ultimately, understanding these practical considerations is essential for conducting high-quality statistical research. It highlights the need for a nuanced approach that considers not just the statistical techniques but also the broader context in which research is conducted. By doing so, researchers can navigate the complexities of resource constraints and cost implications to produce meaningful and impactful results that contribute to the advancement of knowledge in their field.
Statistical Power and Precision
In the realm of statistical analysis, two critical concepts that underpin the reliability and accuracy of research findings are **statistical power** and **precision**. These metrics are essential for evaluating the robustness of a study and ensuring that conclusions drawn from data are valid. **Statistical power**, often denoted as \(1 - \beta\), refers to the probability that a test correctly rejects the null hypothesis when it is false. In practical terms, high statistical power means that a study is more likely to detect an effect if there is one, thereby reducing the risk of Type II errors (failing to detect a true effect). Achieving adequate statistical power is crucial because it directly impacts the confidence one can have in the results; low power can lead to inconclusive or misleading findings. On the other hand, **precision** pertains to the consistency or reproducibility of measurements. It is quantified by the standard error or confidence interval, which indicates how much variation exists in the estimates obtained from a sample. High precision means that repeated measurements would yield similar results, enhancing the reliability of the study's outcomes. Precision is particularly important in practical considerations because it influences how accurately researchers can estimate population parameters from sample data. For instance, in clinical trials, precise estimates of treatment effects are vital for making informed decisions about patient care. However, achieving both high statistical power and precision poses several challenges. One major practical consideration is **sample size**; larger samples generally provide greater statistical power and precision but come at a higher cost and may be logistically challenging to obtain. Researchers must balance these factors when designing their studies. Another challenge is **data quality**; noisy or biased data can severely undermine both power and precision, necessitating rigorous data collection and cleaning protocols. Additionally, **effect size** plays a significant role in determining statistical power. Smaller effect sizes require larger sample sizes to achieve the same level of power, which can be a significant practical hurdle. Furthermore, **research design** itself can impact both power and precision; well-designed studies with appropriate controls and randomization can enhance both metrics but may be more complex to execute. In conclusion, statistical power and precision are interrelated yet distinct aspects of statistical analysis that are critical for ensuring the validity and reliability of research findings. While high power helps in detecting true effects, high precision ensures that estimates are consistent and reliable. Addressing the practical challenges associated with these concepts—such as sample size, data quality, effect size, and research design—is essential for conducting robust and meaningful studies that contribute valuable insights to various fields of research. By carefully considering these factors, researchers can enhance the credibility and impact of their work.