What Is Manipulated Variable
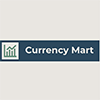
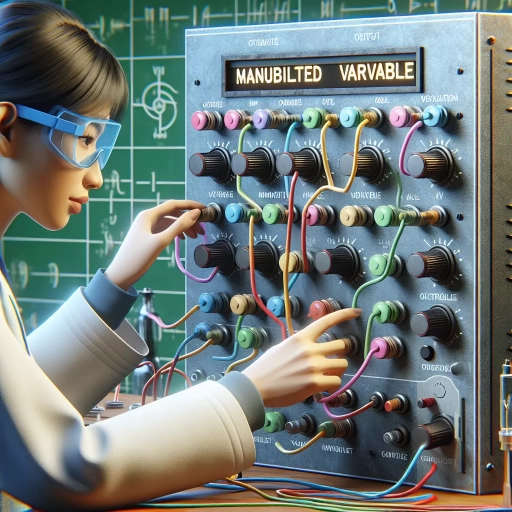
In the realm of scientific research and experimentation, understanding the concept of a manipulated variable is crucial for drawing meaningful conclusions. A manipulated variable, also known as an independent variable, is the factor that researchers intentionally change to observe its effect on the outcome or dependent variable. This concept is foundational in experimental design, allowing scientists to isolate cause-and-effect relationships. To delve into this topic effectively, it is essential to first understand the concept of manipulated variables, which involves grasping the theoretical framework behind their use. Next, identifying and selecting the appropriate manipulated variables is a critical step that ensures the validity and relevance of the experiment. Finally, implementing and analyzing these variables requires meticulous planning and statistical analysis to draw accurate conclusions. By exploring these aspects, researchers can ensure that their experiments are robust and yield reliable results. Let's begin by understanding the concept of manipulated variables in depth.
Understanding the Concept of Manipulated Variable
Understanding the concept of a manipulated variable is crucial in scientific research and experimental design. This key element plays a pivotal role in determining the outcomes of experiments and studies. To grasp its significance, it is essential to delve into three critical aspects: the definition and context of manipulated variables, their role in experimental design, and how they distinguish from independent variables. Firstly, understanding the definition and context of manipulated variables sets the foundation for their application. A manipulated variable is a factor that researchers intentionally change or control to observe its effect on the outcome or dependent variable. This concept is fundamental in establishing cause-and-effect relationships. Secondly, the role of manipulated variables in experimental design cannot be overstated. They are central to the structure of experiments, allowing researchers to isolate and measure the impact of specific factors on the outcome. This controlled environment helps in drawing reliable conclusions. Lastly, distinguishing manipulated variables from independent variables is vital for clarity and accuracy. While both terms are often used interchangeably, they refer to different aspects of an experiment. Independent variables are broader and include all factors that could influence the outcome, whereas manipulated variables are specific factors that are actively changed by the researcher. By exploring these facets, we can gain a comprehensive understanding of manipulated variables. Let's begin by examining the definition and context in which these variables operate.
Definition and Context
In the context of scientific research and experimentation, a manipulated variable is a critical component that helps researchers understand cause-and-effect relationships. **Definition:** A manipulated variable, also known as an independent variable, is the factor that the researcher intentionally changes or controls to observe its effect on the outcome or dependent variable. This variable is crucial because it allows scientists to isolate the impact of a specific factor on the phenomenon being studied. **Context:** In experimental design, the manipulated variable is central to establishing causality. For instance, in a study examining the effect of different fertilizers on plant growth, the type of fertilizer used would be the manipulated variable. By varying this factor (e.g., using different types of fertilizers) while keeping other conditions constant, researchers can determine whether changes in plant growth are directly attributable to the fertilizer used. This controlled approach helps eliminate confounding variables and ensures that any observed effects are due to the manipulated variable. Understanding the concept of a manipulated variable is essential for conducting rigorous and reliable scientific research. It enables researchers to draw conclusions about cause-and-effect relationships with confidence, which is vital for advancing knowledge in various fields such as biology, psychology, physics, and more. By carefully selecting and controlling the manipulated variable, scientists can ensure that their experiments are robust and that their findings are valid and generalizable. This precision in experimental design not only enhances the credibility of research but also contributes significantly to the body of scientific knowledge. In summary, the manipulated variable is a foundational element in scientific inquiry, allowing researchers to systematically investigate and understand complex phenomena through controlled experimentation.
Role in Experimental Design
In the context of experimental design, the role of the manipulated variable is pivotal in determining the causal relationship between variables. The manipulated variable, also known as the independent variable, is the factor that the researcher intentionally changes or controls to observe its effect on the outcome. This deliberate alteration allows researchers to isolate the impact of the manipulated variable on the dependent variable, which is the outcome being measured. For instance, in an experiment to study the effect of different fertilizer types on plant growth, the type of fertilizer would be the manipulated variable. By systematically varying the type of fertilizer and keeping other factors constant, researchers can determine whether and how different fertilizers influence plant growth. The manipulation of this variable is crucial for establishing cause-and-effect relationships. Without controlling for other variables and systematically changing the manipulated variable, it would be impossible to attribute any observed changes in the dependent variable to the manipulated variable. This controlled manipulation helps in minimizing confounding variables that could otherwise obscure the true relationship between the variables under study. Furthermore, the precision and consistency in manipulating the variable ensure that any observed effects are reliable and replicable, which is essential for scientific validity. In addition to its role in establishing causality, the manipulated variable also aids in generalizability. By carefully selecting and manipulating the variable, researchers can ensure that their findings are applicable to broader contexts. For example, if an experiment on the effect of different teaching methods on student performance is conducted by manipulating the teaching method (independent variable), the results can be generalized to other educational settings if the manipulation is well-controlled and representative. Moreover, the manipulated variable plays a key role in hypothesis testing. Researchers often formulate hypotheses based on theoretical expectations about how the manipulated variable will affect the dependent variable. The experimental design then tests these hypotheses by comparing outcomes across different levels of the manipulated variable. This process not only validates or refutes the hypothesis but also provides insights into the underlying mechanisms driving the observed effects. In summary, the manipulated variable is a cornerstone of experimental design, enabling researchers to establish causal relationships, ensure scientific validity, enhance generalizability, and test hypotheses. Its careful manipulation is essential for drawing meaningful conclusions and advancing knowledge in various fields of study.
Distinguishing from Independent Variables
When delving into the concept of manipulated variables, it is crucial to distinguish them from independent variables. While both terms are often used interchangeably, they carry distinct meanings within the context of experimental design and research methodology. A manipulated variable, also known as an independent variable, is a factor that the researcher intentionally changes or controls to observe its effect on the outcome or dependent variable. However, the term "independent variable" can sometimes be broader, encompassing any variable that is not dependent on other variables in the study. In contrast, a manipulated variable specifically refers to an independent variable that is actively altered by the researcher to test its impact. To illustrate this distinction, consider a study examining the effect of different light intensities on plant growth. Here, light intensity is both an independent and a manipulated variable because it is the factor being intentionally varied by the researcher. However, if the study also includes factors like soil type or temperature that are not being manipulated but are still independent variables, these would not be considered manipulated variables. Understanding this nuance is essential for clear communication and rigorous experimental design. It ensures that researchers accurately describe their methods and findings, avoiding confusion between variables that are merely independent and those that are actively manipulated to test hypotheses. By recognizing this difference, researchers can better control for extraneous factors and draw more reliable conclusions about cause-and-effect relationships. In summary, while all manipulated variables are independent variables, not all independent variables are necessarily manipulated. The precise identification of manipulated variables helps in designing experiments that can robustly test hypotheses and contribute meaningfully to scientific knowledge. This clarity is vital for maintaining the integrity and reliability of research outcomes.
Identifying and Selecting Manipulated Variables
Identifying and selecting manipulated variables is a crucial step in the research process, particularly in experimental design. This task involves careful consideration to ensure that the variables chosen are relevant, measurable, and capable of producing meaningful outcomes. To navigate this complex process effectively, researchers must adhere to specific criteria for selection, which include relevance to the research question, feasibility of manipulation, and ethical considerations. Additionally, understanding common examples in research can provide valuable insights into how manipulated variables have been successfully utilized in various studies. However, it is equally important to be aware of potential pitfalls and considerations that could compromise the validity and reliability of the research findings. By understanding these key aspects, researchers can make informed decisions that enhance the quality and impact of their studies. Therefore, it is essential to delve into the criteria for selecting manipulated variables to ensure a robust and well-designed research framework.
Criteria for Selection
When identifying and selecting manipulated variables, several key criteria must be considered to ensure the integrity and effectiveness of the experiment. **Relevance** is paramount; the manipulated variable should directly relate to the research question or hypothesis. It must be **measurable** and **controllable**, allowing for precise adjustments and accurate data collection. **Feasibility** is another crucial factor; the variable should be practical to manipulate within the constraints of the experiment's resources and environment. The **ethical implications** of manipulating the variable must also be considered, ensuring that the experiment does not harm participants or violate ethical standards. Additionally, the **sensitivity** of the variable to changes is important; it should be responsive enough to produce noticeable effects. **Consistency** in application is vital to maintain reliability across different experimental conditions. Finally, the **validity** of the manipulated variable must be established, ensuring that it accurately represents the construct being studied. By carefully evaluating these criteria, researchers can select a manipulated variable that enhances the validity, reliability, and overall quality of their experiment. This meticulous selection process is essential for drawing meaningful conclusions and contributing valuable insights to the field of study.
Common Examples in Research
In the context of identifying and selecting manipulated variables, common examples in research play a crucial role in illustrating the concept and its practical application. A manipulated variable, also known as an independent variable, is the factor that researchers intentionally change or control to observe its effect on the outcome or dependent variable. Here are some common examples that highlight this principle: 1. **Educational Research**: In a study examining the impact of different teaching methods on student performance, the teaching method (e.g., traditional lecture vs. interactive learning) would be the manipulated variable. Researchers might compare student scores or engagement levels between these two groups to determine which method is more effective. 2. **Medical Research**: In clinical trials evaluating the efficacy of a new medication, the presence or absence of the medication is the manipulated variable. Participants are randomly assigned to either receive the medication or a placebo, and their health outcomes are compared to assess the medication's effectiveness. 3. **Psychological Studies**: A study investigating the effects of stress on cognitive function might use stress levels as the manipulated variable. Participants could be exposed to different levels of stress (e.g., through tasks designed to induce varying degrees of anxiety) and then tested on cognitive tasks to see how stress impacts performance. 4. **Environmental Science**: Researchers studying the effects of light intensity on plant growth might manipulate light exposure levels (e.g., high, medium, low) to observe how it affects plant height, leaf size, or other growth metrics. 5. **Marketing Research**: A company testing the impact of different pricing strategies on sales volume could manipulate the price point (e.g., discount, regular price, premium price) to see which pricing strategy results in higher sales. 6. **Nutritional Studies**: In an experiment examining how different diets affect weight loss, the type of diet (e.g., low-carb, low-fat, balanced) would be the manipulated variable. Participants would follow one of these diets for a specified period, and their weight loss would be measured and compared. These examples demonstrate how researchers systematically alter one variable while controlling others to isolate and measure its specific impact on an outcome. By doing so, they can draw conclusions about cause-and-effect relationships and make informed decisions based on empirical evidence. Understanding these common examples helps researchers design robust experiments that accurately identify and select manipulated variables, ensuring reliable and meaningful results.
Potential Pitfalls and Considerations
When identifying and selecting manipulated variables, it is crucial to be aware of several potential pitfalls and considerations to ensure the integrity and effectiveness of your research or experiment. **Confounding Variables**: One of the primary concerns is the presence of confounding variables, which can influence the outcome of your study independently of the manipulated variable. Failing to control for these variables can lead to biased results and incorrect conclusions. **Measurement Error**: Another pitfall is measurement error, which can arise from inaccurate or unreliable measurement tools. Ensuring that your measurement instruments are validated and calibrated is essential to avoid misleading data. **Sampling Bias**: The selection of participants or samples can also introduce bias if not done randomly or representatively. This can limit the generalizability of your findings and skew the results in favor of a particular subgroup. **Ethical Considerations**: Manipulating variables often involves human subjects, making ethical considerations paramount. Ensuring informed consent, minimizing harm, and respecting privacy are critical to maintaining ethical standards. **Statistical Power**: The sample size and statistical power of your study must be adequate to detect significant effects. Underpowered studies may fail to reveal true differences, leading to Type II errors. **Interactions and Moderators**: Ignoring potential interactions between the manipulated variable and other factors (moderators) can mask important effects or lead to misinterpretation of results. **Temporal and Spatial Factors**: Time and location can significantly impact the outcomes of your study. Seasonal variations, cultural differences, or temporal trends must be considered to avoid confounding effects. **Placebo and Hawthorne Effects**: In studies involving human subjects, placebo effects (where participants respond to perceived treatment) and Hawthorne effects (where participants alter behavior due to being observed) must be controlled through appropriate design strategies like blinding and control groups. **Resource Constraints**: Finally, practical considerations such as budget, time, and resource availability must be balanced against the need for rigorous methodology to ensure that the study is feasible yet robust. By carefully addressing these potential pitfalls and considerations, researchers can enhance the validity, reliability, and generalizability of their findings, ultimately contributing to more accurate and meaningful conclusions about the manipulated variables under investigation.
Implementing and Analyzing Manipulated Variables
Implementing and analyzing manipulated variables is a crucial aspect of scientific research, ensuring the integrity and reliability of experimental findings. This process involves several key components that collectively contribute to the validity of the results. First, **Experimental Controls and Procedures** are essential for establishing a baseline against which the effects of the manipulated variable can be measured. This includes setting up control groups, defining protocols, and ensuring consistency in the experimental setup. Second, **Data Collection and Measurement Techniques** play a vital role in capturing accurate and relevant data. This involves selecting appropriate methods for data collection, calibrating instruments, and ensuring that the data collected is both reliable and valid. Finally, **Statistical Analysis and Interpretation** are necessary for drawing meaningful conclusions from the collected data. By applying statistical methods, researchers can identify patterns, test hypotheses, and interpret the significance of the findings. Understanding these elements is fundamental to conducting robust experiments, and it begins with the meticulous planning and execution of experimental controls and procedures.
Experimental Controls and Procedures
In the context of scientific research, particularly when implementing and analyzing manipulated variables, experimental controls and procedures are crucial for ensuring the validity and reliability of the findings. Experimental controls refer to the measures taken to minimize or eliminate extraneous variables that could influence the outcome of an experiment. These controls help isolate the effect of the manipulated variable, allowing researchers to draw clear conclusions about its impact. For instance, in a study examining the effect of light on plant growth, controlling for factors such as soil quality, water intake, and temperature ensures that any observed differences in growth can be attributed solely to variations in light exposure. Procedures, on the other hand, outline the step-by-step actions taken during the experiment. These include setting up the experimental design, collecting data, and analyzing results. A well-defined procedure ensures consistency across all trials and helps in replicating the study if needed. For example, in an experiment investigating the effect of different temperatures on enzyme activity, the procedure might involve preparing enzyme solutions under controlled conditions, exposing them to various temperatures for a specified duration, and then measuring their activity using a standardized assay method. To implement effective experimental controls and procedures, researchers often employ several strategies. One common approach is randomization, where participants or samples are randomly assigned to different treatment groups to eliminate bias. Another strategy is using a control group that does not receive the manipulated variable; this group serves as a baseline against which the effects of the manipulated variable can be compared. Additionally, researchers may use blinding techniques where either the participants or the experimenters (or both) are unaware of group assignments to prevent any unconscious biases from influencing the results. Furthermore, precise measurement tools and calibrated instruments are essential for accurate data collection. Regular calibration of equipment ensures that measurements are consistent and reliable. Data collection should also follow a systematic protocol to avoid human error and ensure that all variables are measured under identical conditions. Analyzing manipulated variables effectively requires careful consideration of statistical methods. Researchers use statistical tests to determine whether observed differences between treatment groups are significant and not due to chance. Techniques such as ANOVA (Analysis of Variance) or t-tests are commonly used to compare means across different groups. In summary, experimental controls and procedures are fundamental components of scientific research when analyzing manipulated variables. By controlling for extraneous variables and following a rigorous procedural framework, researchers can ensure that their findings are accurate and reliable. These practices not only enhance the validity of the study but also facilitate replication and verification by other researchers, which is essential for advancing scientific knowledge.
Data Collection and Measurement Techniques
Data collection and measurement techniques are crucial components in the implementation and analysis of manipulated variables. These techniques ensure that the data gathered is accurate, reliable, and relevant to the research or experimental objectives. Here are some key methods: 1. **Surveys and Questionnaires**: These tools are used to collect self-reported data from participants. They can be administered online or in person and are particularly useful for gathering information about attitudes, behaviors, and perceptions. 2. **Observational Studies**: Observers record behaviors or phenomena in natural or controlled settings. This method is valuable for studying real-world interactions without influencing the subjects' behavior. 3. **Experiments**: Controlled experiments involve manipulating one or more variables while keeping other factors constant to measure the effect on an outcome variable. This is a gold standard for establishing causality. 4. **Sensors and IoT Devices**: These technologies collect data automatically from various environments, such as temperature sensors, GPS trackers, or smart home devices, providing continuous and precise measurements. 5. **Interviews**: In-depth interviews allow for detailed, qualitative insights into participants' experiences and opinions. They can be conducted face-to-face or remotely. 6. **Secondary Data Collection**: Utilizing existing datasets from previous studies, databases, or public records can save time and resources while providing valuable comparative insights. 7. **Statistical Sampling**: Selecting a representative sample from a larger population ensures that the findings can be generalized to the broader group. Techniques include random sampling, stratified sampling, and cluster sampling. 8. **Psychometric Testing**: Standardized tests measure psychological attributes such as intelligence, personality traits, or cognitive abilities. These tests are designed to ensure reliability and validity. 9. **Biometric Data**: Collecting physiological data through tools like heart rate monitors, EEGs, or facial recognition software provides objective measures of physical responses. 10. **Content Analysis**: Analyzing text, images, or videos from various sources (e.g., social media posts, news articles) helps in understanding trends, sentiments, and patterns within large datasets. Each technique has its strengths and limitations; choosing the right method depends on the research question, available resources, and the nature of the manipulated variable being studied. For instance, if the goal is to understand how a new marketing strategy affects consumer behavior, surveys combined with observational studies might be ideal. Conversely, if the focus is on measuring the impact of a new medication on blood pressure levels, controlled experiments with biometric data collection would be more appropriate. In summary, effective data collection and measurement techniques are essential for implementing and analyzing manipulated variables accurately. By selecting the most suitable methods based on the research objectives and ensuring high-quality data collection processes, researchers can draw reliable conclusions and make informed decisions.
Statistical Analysis and Interpretation
Statistical analysis and interpretation are crucial components in the process of implementing and analyzing manipulated variables. When conducting experiments or studies, researchers often manipulate one or more variables to observe their effects on the outcome. Statistical analysis provides the tools to quantify these effects, test hypotheses, and draw meaningful conclusions. First, **data collection** is essential, where data points are gathered from the manipulated variable and the outcome variable. This data is then subjected to various statistical tests such as t-tests, ANOVA (Analysis of Variance), regression analysis, and non-parametric tests depending on the nature of the data and research questions. These tests help determine whether the observed differences between groups are statistically significant, indicating that the manipulated variable has a real effect. **Descriptive statistics** are used to summarize the data, providing insights into central tendencies (mean, median) and variability (standard deviation, variance). This initial step helps in understanding the distribution of the data and identifying any outliers or anomalies that might affect the analysis. **Inferential statistics** allow researchers to make generalizations about the population based on sample data. Techniques like confidence intervals and hypothesis testing enable researchers to estimate population parameters and determine whether observed effects are likely due to chance or if they reflect a true relationship between the manipulated variable and the outcome. **Regression analysis** is particularly useful when examining the relationship between multiple variables. It helps in understanding how changes in the manipulated variable(s) affect the outcome variable while controlling for other potential confounding variables. This method also provides coefficients that quantify the strength and direction of these relationships. **Interpretation** of statistical results involves understanding the practical significance of the findings. While statistical significance indicates that an effect is unlikely due to chance, it does not necessarily imply practical significance. Researchers must consider the effect size and how it translates into real-world implications. Moreover, **visualizing data** through plots and graphs enhances interpretation by providing a clear visual representation of trends and patterns. Tools like scatter plots, bar charts, and box plots help in identifying correlations, outliers, and distribution shapes which can be critical in understanding complex relationships. Finally, **validity and reliability** of the statistical analysis are paramount. Ensuring that the sample is representative of the population and that measurement tools are reliable helps in maintaining internal validity. External validity, which pertains to generalizability, is also crucial for applying findings beyond the study context. In summary, statistical analysis and interpretation are indispensable for understanding the impact of manipulated variables. By employing a range of statistical techniques and ensuring rigorous methodology, researchers can draw accurate and meaningful conclusions that contribute to scientific knowledge and practical applications.