After Biometric What Is Next
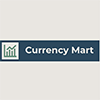
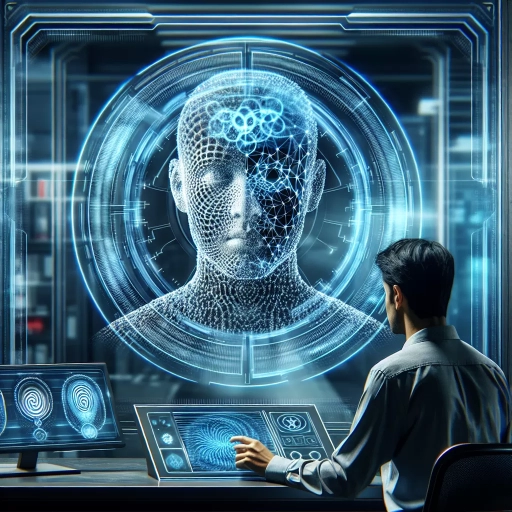
In the era of rapid technological evolution, biometrics has revolutionized the way we authenticate and secure our identities. However, as we continue to push the boundaries of innovation, it is imperative to explore what lies beyond this current frontier. The future of technology is poised to be shaped by several transformative trends. One of the most significant advancements is in Artificial Intelligence (AI) and Machine Learning (ML), which promise to enhance decision-making processes and automate complex tasks. Additionally, the emergence of Quantum Computing is set to redefine computational power, solving problems that were previously insurmountable. Furthermore, the integration of the Internet of Things (IoT) will seamlessly connect devices, fostering a more interconnected and efficient world. As we delve into these cutting-edge technologies, it becomes clear that the next wave of innovation will be driven by these key areas. Let us begin by examining the profound impact of **Advancements in AI and Machine Learning**, which are already reshaping industries and redefining the possibilities of what technology can achieve.
Advancements in AI and Machine Learning
In the rapidly evolving landscape of technology, advancements in Artificial Intelligence (AI) and Machine Learning (ML) are revolutionizing various aspects of our lives. These innovations are not just enhancing existing processes but also creating new paradigms for how we interact with data, technology, and each other. One of the significant impacts of AI and ML is seen in **Enhanced Predictive Analytics**, where sophisticated algorithms can forecast trends and outcomes with unprecedented accuracy, driving better decision-making across industries. Additionally, **Personalized User Experiences** are becoming the norm, as AI-driven systems tailor interactions to individual preferences, enhancing customer satisfaction and engagement. Furthermore, **Automated Decision-Making** is transforming operational efficiency by enabling machines to make informed decisions without human intervention, streamlining processes and reducing errors. As we delve into these advancements, it becomes clear that the future of technology is deeply intertwined with the continuous evolution of AI and Machine Learning. This article will explore these transformative aspects in detail, highlighting how they are shaping the future of technology and beyond. Transitioning into the heart of these advancements, we will examine the profound impact of AI and Machine Learning on our world.
Enhanced Predictive Analytics
Enhanced Predictive Analytics represents a significant leap forward in the realm of data analysis, particularly when considered within the broader context of advancements in AI and machine learning. This sophisticated approach leverages advanced algorithms and machine learning techniques to predict future outcomes with unprecedented accuracy. Unlike traditional predictive analytics, which often rely on historical data and static models, enhanced predictive analytics integrates real-time data streams, dynamic modeling, and continuous learning to adapt to evolving trends and patterns. This capability is crucial in today's fast-paced business environment, where timely and accurate predictions can make the difference between market leadership and lagging behind. At its core, enhanced predictive analytics harnesses the power of artificial intelligence to analyze vast amounts of data from diverse sources, including IoT devices, social media, customer interactions, and more. Machine learning algorithms are trained on these datasets to identify complex relationships and anomalies that might elude human analysts. These models can then forecast everything from customer behavior and market demand to operational efficiencies and potential risks. For instance, in healthcare, enhanced predictive analytics can predict patient outcomes based on genetic profiles, medical histories, and real-time health monitoring data, enabling personalized treatment plans and improved patient care. Moreover, the integration of AI-driven predictive analytics with other technologies like natural language processing (NLP) and computer vision further enhances its capabilities. NLP can analyze unstructured data such as text from customer feedback or social media posts to gauge sentiment and predict consumer trends. Similarly, computer vision can analyze visual data from surveillance cameras or medical images to detect anomalies or predict maintenance needs in industrial settings. This multi-faceted approach ensures that predictive analytics is not just about forecasting but also about providing actionable insights that drive strategic decision-making. The impact of enhanced predictive analytics extends across various industries. In finance, it helps in fraud detection and risk assessment by identifying unusual transaction patterns in real-time. In retail, it optimizes inventory management by predicting demand fluctuations based on seasonal trends, weather forecasts, and consumer behavior. In manufacturing, it enables predictive maintenance by analyzing sensor data from equipment to anticipate failures before they occur. In conclusion, enhanced predictive analytics stands as a cornerstone of modern data-driven decision-making. By combining the strengths of AI, machine learning, and real-time data analysis, it offers unparalleled insights into future outcomes. As we continue to navigate the complexities of a rapidly changing world, this technology will play an increasingly vital role in helping organizations stay ahead of the curve and make informed decisions that drive growth and innovation.
Personalized User Experiences
In the wake of advancements in biometric technologies, the next frontier in enhancing user interactions lies in the realm of personalized user experiences, significantly bolstered by advancements in AI and machine learning. Personalized user experiences are tailored to meet the unique preferences, behaviors, and needs of individual users, creating a more intuitive and engaging interaction with digital platforms. AI algorithms, equipped with machine learning capabilities, can analyze vast amounts of user data to identify patterns and preferences. This data-driven approach allows for the dynamic adjustment of content, recommendations, and interface elements in real-time, ensuring that each user's experience is uniquely optimized. For instance, in e-commerce, AI-driven systems can analyze a user's browsing history, purchase behavior, and search queries to offer personalized product recommendations. Similarly, in streaming services, machine learning algorithms can predict user preferences based on viewing history and ratings, suggesting content that aligns with their tastes. This level of personalization not only enhances user satisfaction but also drives business outcomes by increasing engagement and conversion rates. Moreover, personalized user experiences extend beyond mere content curation; they also encompass adaptive interfaces that adjust to a user's interaction style. For example, AI can optimize the layout and navigation of a website or app based on how a user interacts with it, making it easier for them to find what they need quickly. Additionally, AI-powered chatbots and virtual assistants can provide personalized support by understanding the context of a user's queries and offering relevant solutions. The integration of AI and machine learning into personalized user experiences also enables continuous improvement through feedback loops. These systems can learn from user interactions over time, refining their models to deliver even more accurate and relevant experiences. This iterative process ensures that the personalization becomes increasingly sophisticated, aligning more closely with the evolving needs and preferences of users. In summary, the fusion of AI and machine learning with personalized user experiences represents a significant leap forward in how we interact with digital technologies. By leveraging these advancements, businesses can create highly tailored and engaging experiences that not only enhance user satisfaction but also drive meaningful business outcomes. As we move beyond biometric technologies, the future of user interaction is poised to be more intuitive, personalized, and responsive than ever before.
Automated Decision-Making
Automated decision-making, a cornerstone of modern technological advancements, has revolutionized the way businesses and organizations operate. This paradigm shift, driven by significant strides in AI and machine learning, enables systems to make decisions autonomously, often with greater speed and accuracy than human counterparts. At its core, automated decision-making leverages sophisticated algorithms that analyze vast amounts of data to identify patterns, predict outcomes, and optimize processes. For instance, in the financial sector, AI-powered systems can scrutinize creditworthiness in real-time, approving or rejecting loan applications based on comprehensive risk assessments. Similarly, in healthcare, machine learning algorithms can analyze patient data to diagnose conditions more accurately and suggest personalized treatment plans. The integration of automated decision-making into various industries has been transformative. In retail, AI-driven systems personalize customer experiences by recommending products based on past purchases and browsing history, enhancing customer satisfaction and driving sales. In manufacturing, predictive maintenance algorithms can detect potential equipment failures, scheduling maintenance before downtime occurs, thereby reducing operational costs and improving overall efficiency. Furthermore, autonomous vehicles rely on advanced AI to make split-second decisions, ensuring safer and more efficient transportation. However, the rise of automated decision-making also raises important ethical considerations. Issues such as bias in algorithms, transparency in decision processes, and accountability for errors are critical concerns that must be addressed. Ensuring that AI systems are fair and unbiased is paramount; otherwise, they may perpetuate existing social inequalities. Transparency is equally important, as stakeholders need to understand how decisions are made to trust the outcomes. Regulatory frameworks are being developed to address these challenges, emphasizing the need for explainable AI and robust auditing mechanisms. Despite these challenges, the future of automated decision-making looks promising. As AI and machine learning continue to evolve, we can expect even more sophisticated applications across various sectors. For example, in education, AI could tailor learning experiences to individual students' needs, improving educational outcomes. In environmental conservation, AI can analyze satellite data to monitor deforestation and predict natural disasters, aiding in timely interventions. In conclusion, automated decision-making represents a significant leap forward in leveraging technology to enhance decision-making processes. While it presents numerous benefits such as increased efficiency and accuracy, it also necessitates careful consideration of ethical implications. As we move forward with integrating AI into more aspects of our lives, it is crucial to strike a balance between technological advancement and ethical responsibility. This balance will ensure that the full potential of automated decision-making is realized while safeguarding against its pitfalls.
Emergence of Quantum Computing
The emergence of quantum computing marks a significant milestone in the evolution of technology, promising to revolutionize various fields with its unparalleled capabilities. At the heart of this innovation lies the potential for **Unprecedented Processing Power**, enabling computers to solve complex problems that are currently beyond the reach of classical systems. This leap in computational prowess is set to transform industries by facilitating **Advanced Cryptography and Security**, ensuring data protection at levels previously unimaginable. Additionally, quantum computing will play a crucial role in the **Optimization of Complex Systems**, allowing for more efficient solutions in fields such as logistics, finance, and energy management. As these advancements unfold, they will also have a profound impact on **Advancements in AI and Machine Learning**, enabling these technologies to process vast amounts of data more effectively and make more accurate predictions, thereby driving further innovation and progress. This article delves into these transformative aspects of quantum computing, exploring how they are reshaping the technological landscape and paving the way for a future where computational limits are pushed to new boundaries.
Unprecedented Processing Power
The emergence of quantum computing marks a significant leap in technological advancement, particularly in terms of unprecedented processing power. Unlike classical computers, which rely on bits to process information in binary form (0s and 1s), quantum computers utilize quantum bits or qubits. These qubits can exist in multiple states simultaneously, allowing for the processing of vast amounts of data in parallel. This property, known as superposition, enables quantum computers to tackle complex problems that are currently intractable for classical systems. For instance, quantum computers can factor large numbers exponentially faster than their classical counterparts, a capability that has profound implications for cryptography and cybersecurity. Additionally, quantum computing's ability to simulate complex systems at the molecular level could revolutionize fields such as chemistry and materials science, leading to breakthroughs in drug discovery and the development of new materials. The potential applications are vast and varied, from optimizing complex logistics and financial models to enhancing machine learning algorithms. As quantum computing continues to evolve, it promises to unlock new frontiers in computational power, driving innovation across multiple industries and transforming the way we approach some of the world's most challenging problems. This shift towards quantum computing underscores the relentless pursuit of technological advancement and highlights the transformative impact that such innovations can have on our collective future.
Advanced Cryptography and Security
As we navigate the evolving landscape of technology, the emergence of quantum computing underscores a critical need for advanced cryptography and security measures. Quantum computing, with its potential to solve complex problems at unprecedented speeds, poses a significant threat to traditional cryptographic systems. Current encryption methods, such as RSA and elliptic curve cryptography, rely on mathematical problems that are difficult for classical computers to solve but could be vulnerable to quantum attacks. For instance, Shor's algorithm, a quantum algorithm discovered by Peter Shor, can factor large numbers exponentially faster than any known classical algorithm, thereby compromising the security of many cryptographic protocols. To address this challenge, researchers are developing new cryptographic techniques that are resistant to quantum attacks. One promising approach is the use of lattice-based cryptography, which relies on the hardness of problems related to lattices in high-dimensional spaces. Another method involves code-based cryptography, such as the McEliece cryptosystem, which has been shown to be resilient against both classical and quantum attacks. Additionally, hash-based signatures and multivariate polynomial cryptography are being explored as potential solutions. The transition to quantum-resistant cryptography is not merely a theoretical exercise but a practical necessity. Organizations and governments are already beginning to implement these advanced cryptographic systems to ensure long-term security. For example, the National Institute of Standards and Technology (NIST) has initiated a process to standardize post-quantum cryptographic algorithms, recognizing the urgency of this issue. Beyond algorithmic advancements, the integration of advanced security protocols is crucial. This includes the adoption of hybrid approaches that combine classical and quantum-resistant algorithms to provide both immediate and future-proof security. Furthermore, secure key management and distribution systems must be developed to handle the complexities of quantum-resistant keys. In conclusion, the advent of quantum computing necessitates a paradigm shift in cryptography and security. By investing in advanced cryptographic techniques and implementing robust security protocols, we can safeguard our digital infrastructure against the potential threats posed by quantum computers. This proactive approach ensures that as quantum computing emerges, our security frameworks will be ready to meet the challenge, protecting sensitive information and maintaining trust in our increasingly interconnected world.
Optimization of Complex Systems
The optimization of complex systems is a critical frontier in the era of technological advancement, particularly as we witness the emergence of quantum computing. Complex systems, characterized by their intricate interdependencies and nonlinear behaviors, pose significant challenges in terms of optimization. Traditional methods often fall short due to the sheer scale and complexity of these systems, leading to suboptimal solutions that fail to leverage the full potential of available resources. Here, quantum computing steps in as a game-changer. Quantum algorithms, such as the Quantum Approximate Optimization Algorithm (QAOA) and the Variational Quantum Eigensolver (VQE), are designed to tackle optimization problems that are intractable for classical computers. These algorithms exploit quantum phenomena like superposition and entanglement to explore vast solution spaces exponentially faster than their classical counterparts. In practical terms, this means that quantum computing can be applied to optimize complex networks, such as those found in logistics, finance, and energy distribution. For instance, in logistics, quantum computers can quickly find the most efficient routes for delivery fleets, reducing fuel consumption and lowering emissions. In finance, they can optimize portfolio management by analyzing vast datasets to predict market trends and minimize risk. Similarly, in energy distribution, quantum algorithms can optimize grid management to ensure stable and efficient power supply. Moreover, the optimization of complex systems extends beyond these domains into fields like materials science and drug discovery. Quantum computers can simulate the behavior of materials at the molecular level, allowing for the discovery of new materials with unique properties. This capability is particularly promising for developing more efficient solar cells, batteries, and other critical technologies. In drug discovery, quantum computing can accelerate the process of identifying potential drug candidates by simulating complex molecular interactions, thereby speeding up the development of new treatments. The integration of quantum computing into the optimization of complex systems also underscores the importance of interdisciplinary collaboration. Researchers from diverse fields—physics, computer science, engineering, and mathematics—must work together to develop practical applications that leverage quantum computing's unique capabilities. This collaborative approach not only accelerates innovation but also ensures that solutions are tailored to real-world problems. In conclusion, the optimization of complex systems through quantum computing represents a significant leap forward in technological capability. By harnessing the power of quantum algorithms, we can solve problems that were previously unsolvable, leading to breakthroughs across various industries and fields. As we continue to explore the potential of quantum computing, it becomes clear that this technology is not just a tool for the future but a transformative force that is already reshaping our present.
Integration of Internet of Things (IoT)
The Integration of Internet of Things (IoT) has revolutionized various aspects of modern life, transforming how we live, work, and interact with our environment. At the heart of this transformation are three key areas: Smart Home and City Solutions, Real-Time Data Monitoring and Analysis, and Enhanced Industrial Automation. Smart Home and City Solutions leverage IoT to create intelligent living spaces and urban infrastructures, enhancing comfort, safety, and efficiency. Real-Time Data Monitoring and Analysis enable organizations to make informed decisions by processing vast amounts of data in real-time, driving operational excellence. Meanwhile, Enhanced Industrial Automation optimizes production processes, reducing costs and improving productivity. These advancements are not standalone; they are intricately linked with broader technological trends. As IoT continues to evolve, it is increasingly intertwined with Advancements in AI and Machine Learning, which further amplify its potential by enabling more sophisticated automation, predictive analytics, and intelligent decision-making. This synergy between IoT, AI, and Machine Learning is poised to redefine the future of technology and innovation.
Smart Home and City Solutions
The integration of the Internet of Things (IoT) has revolutionized the concept of smart homes and cities, transforming them into highly efficient, sustainable, and interconnected ecosystems. At the heart of these smart solutions lies a network of sensors, devices, and data analytics that work in harmony to enhance daily life. In smart homes, IoT enables seamless automation where lighting, heating, cooling, and security systems can be controlled remotely through mobile apps. For instance, smart thermostats like Nest learn occupants' schedules and preferences to optimize energy consumption, while smart locks like August ensure secure entry and exit without the need for physical keys. These devices communicate with each other to create a cohesive living environment that is both comfortable and energy-efficient. Extending this concept to smart cities, IoT plays a pivotal role in urban planning and management. Smart traffic management systems use real-time data from sensors and cameras to optimize traffic flow, reducing congestion and travel times. Public transportation systems are also enhanced with IoT, allowing for real-time tracking and scheduling adjustments based on passenger demand. Additionally, smart waste management systems equipped with sensors monitor waste levels in bins, ensuring that collections are made only when necessary, thereby reducing waste disposal costs and environmental impact. Energy-efficient streetlights that adjust their brightness based on natural light availability further contribute to the sustainability of urban infrastructure. Moreover, IoT in smart cities fosters public safety through intelligent surveillance systems that can detect anomalies and alert authorities promptly. For example, smart parking solutions guide drivers to available spots, reducing the time spent searching for parking and lowering emissions from idling vehicles. The integration of IoT also supports healthcare by enabling remote monitoring of patients and providing timely interventions through wearable devices and telemedicine platforms. In essence, the integration of IoT in smart homes and cities not only improves the quality of life but also drives economic growth by enhancing operational efficiency and reducing resource consumption. As technology continues to evolve, we can expect even more innovative applications of IoT that will further transform urban living into a more sustainable, connected, and intelligent experience. This seamless integration underscores the potential of IoT to shape the future of our living spaces, making them smarter, greener, and more responsive to our needs.
Real-Time Data Monitoring and Analysis
In the era of rapid technological advancement, the integration of Internet of Things (IoT) has revolutionized various sectors by enabling real-time data monitoring and analysis. This capability is a cornerstone of modern IoT applications, allowing for immediate insights and actionable decisions. Real-time data monitoring involves the continuous collection and processing of data from numerous sources, such as sensors, devices, and other connected systems. This data is then analyzed using advanced algorithms and machine learning techniques to extract meaningful patterns, trends, and anomalies. The significance of real-time data monitoring and analysis cannot be overstated. In industrial settings, it allows for predictive maintenance, where equipment health is monitored continuously to anticipate potential failures before they occur, thereby reducing downtime and increasing overall efficiency. In healthcare, real-time monitoring of patient vital signs can lead to early detection of critical conditions, enabling timely interventions that save lives. Similarly, in smart cities, real-time traffic monitoring helps optimize traffic flow, reducing congestion and improving air quality. One of the key benefits of real-time data analysis is its ability to provide instant feedback. For instance, in manufacturing, real-time quality control can detect defects as they occur, allowing for immediate adjustments to the production line. This not only improves product quality but also reduces waste and enhances customer satisfaction. Furthermore, real-time analytics can be integrated with other technologies like artificial intelligence (AI) and big data to create sophisticated decision-making systems that adapt dynamically to changing conditions. The integration of IoT with real-time data monitoring and analysis also enhances security. By continuously monitoring network traffic and device activity, organizations can detect and respond to cyber threats in real-time, significantly reducing the risk of data breaches. Additionally, IoT devices equipped with real-time analytics can self-diagnose security vulnerabilities and implement patches or updates without human intervention. From a business perspective, real-time data analysis offers a competitive edge by enabling organizations to respond swiftly to market changes. For example, retailers can monitor sales data in real-time to adjust inventory levels, pricing strategies, and marketing campaigns on the fly. This agility allows businesses to stay ahead of competitors and better serve their customers. In conclusion, the integration of IoT with real-time data monitoring and analysis is a powerful combination that drives efficiency, innovation, and competitiveness across various industries. By leveraging this technology, organizations can make data-driven decisions quickly, improve operational performance, and deliver superior outcomes. As we continue to navigate the complexities of a connected world, the importance of real-time data monitoring and analysis will only continue to grow, making it an indispensable tool for any forward-thinking organization.
Enhanced Industrial Automation
Enhanced industrial automation, a cornerstone of modern manufacturing, has been significantly bolstered by the integration of the Internet of Things (IoT). This synergy has transformed traditional industrial processes into highly efficient, data-driven operations. At its core, IoT enables the seamless communication between various devices and systems within an industrial setting, creating a network of interconnected sensors, machines, and control systems. This interconnectedness allows for real-time monitoring and control, enabling immediate responses to changes in production conditions. For instance, IoT sensors can detect anomalies in machinery performance, triggering predictive maintenance schedules that prevent costly downtime and enhance overall equipment effectiveness. The integration of IoT also facilitates advanced analytics and artificial intelligence (AI) applications. By leveraging big data analytics, industries can gain deep insights into their operations, optimizing processes such as supply chain management, inventory control, and quality assurance. AI algorithms can analyze vast amounts of data from IoT devices to predict trends, identify bottlenecks, and suggest improvements. This not only improves operational efficiency but also enhances product quality by ensuring that every stage of production adheres to stringent standards. Moreover, IoT-driven automation enhances worker safety and productivity. Wearable devices and smart sensors can monitor worker health and environmental conditions in real-time, alerting supervisors to potential hazards before they become critical. Additionally, augmented reality (AR) and virtual reality (VR) technologies, integrated with IoT, provide workers with interactive training modules and real-time guidance during complex tasks, reducing errors and improving skill acquisition. Another significant benefit of IoT in industrial automation is the ability to implement Industry 4.0 concepts such as smart factories and digital twins. Smart factories are fully automated facilities where all aspects of production are managed through a centralized IoT system, ensuring maximum efficiency and minimal human intervention. Digital twins—virtual replicas of physical systems—allow for simulation-based testing and optimization before actual implementation, reducing the risk of errors and improving overall system reliability. In summary, the integration of IoT into industrial automation has revolutionized the manufacturing landscape by introducing unprecedented levels of efficiency, precision, and innovation. As industries continue to adopt these technologies, they are poised to achieve higher productivity, better product quality, enhanced worker safety, and significant cost savings. The future of industrial automation is undoubtedly tied to the evolving capabilities of IoT, promising a brighter, more efficient tomorrow for global manufacturing.