What Does Ddf Mean
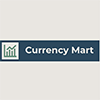
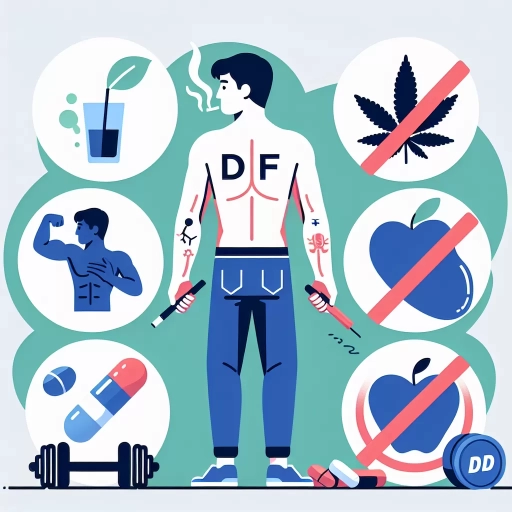
In today's fast-paced and technologically driven world, acronyms and abbreviations have become an integral part of our daily communication. One such term that has garnered significant attention across various industries is "DDF." Standing for "Data Distribution Facility," DDF plays a crucial role in the efficient management and dissemination of data. However, its significance extends beyond mere data handling; it has profound applications and implications that shape how we operate in fields such as finance, healthcare, and technology. This article delves into the multifaceted nature of DDF, starting with an in-depth exploration of **Understanding the Term "DDF"** to provide a solid foundation. We will then examine **Applications and Implications of DDF**, highlighting its practical uses and the impact it has on different sectors. Finally, we will discuss **Challenges and Future of DDF**, addressing the obstacles it faces and the potential advancements that could redefine its role in the future. By understanding these aspects, readers will gain a comprehensive insight into the importance and evolving landscape of DDF. Let's begin by **Understanding the Term "DDF"**.
Understanding the Term "DDF"
Understanding the term "DDF" requires a multifaceted approach, as it encompasses various dimensions that are crucial for a comprehensive grasp. To delve into the meaning and significance of "DDF," it is essential to explore its definition and origin, which provide the foundational understanding of what the term represents. Additionally, examining common usage contexts helps in appreciating how "DDF" is applied in different scenarios, highlighting its practical relevance. Furthermore, tracing the historical development of "DDF" offers insights into its evolution and the factors that have shaped its current usage. By integrating these perspectives, one can gain a thorough understanding of "DDF" and its importance. Let us begin by uncovering the definition and origin of "DDF," which serves as the cornerstone for our exploration.
Definition and Origin
**Understanding the Term "DDF"** To delve into the meaning and significance of "DDF," it is crucial to first grasp its definition and origin. The acronym "DDF" stands for "Drug-Device Combination" or "Drug-Device Combination Product," although it can also be interpreted as "Drug Delivery Formulation" in certain contexts. The term is predominantly used within the pharmaceutical and medical device industries. ### Definition A Drug-Device Combination Product is a therapeutic product that combines a drug and a device, where the drug is intended to be used in conjunction with the device. This integration can enhance the delivery, efficacy, and safety of the drug. Examples include insulin pumps, inhalers for asthma medications, and implantable drug-eluting stents. These products leverage the strengths of both components to provide better patient outcomes. ### Origin The concept of combining drugs and devices dates back several decades but has gained significant traction in recent years due to advancements in technology and materials science. The modern era of drug-device combinations began to take shape in the 1980s with the development of implantable devices such as pacemakers and drug-eluting stents. However, it was not until the late 1990s and early 2000s that regulatory frameworks began to formalize guidelines for these hybrid products. In the United States, the FDA plays a pivotal role in regulating these products through its Office of Combination Products (OCP), established in 2002. The OCP ensures that drug-device combinations meet stringent safety and efficacy standards by coordinating across different FDA centers responsible for drugs, devices, and biologics. ### Evolution and Impact The evolution of drug-device combinations has been driven by the need for more targeted and efficient drug delivery systems. For instance, inhalers have revolutionized the treatment of respiratory diseases by delivering medication directly to the lungs, reducing systemic side effects. Similarly, insulin pumps have transformed diabetes management by providing continuous glucose monitoring and precise insulin dosing. These innovations have not only improved patient compliance but also enhanced therapeutic outcomes. The integration of sensors, microelectronics, and nanotechnology into these devices has further expanded their capabilities, enabling real-time monitoring and personalized treatment plans. ### Regulatory Considerations The development and approval process for drug-device combinations is complex due to the dual nature of these products. Manufacturers must navigate multiple regulatory pathways, ensuring compliance with both drug and device regulations. This involves rigorous clinical trials to demonstrate safety and efficacy as well as post-market surveillance to monitor performance and adverse events. In conclusion, understanding the term "DDF" requires a deep dive into its definition, origin, and the evolving landscape of drug-device combinations. These products represent a significant advancement in healthcare technology, offering improved treatment options for various medical conditions. As technology continues to advance, we can expect even more sophisticated drug-device combinations that will further transform patient care.
Common Usage Contexts
In the context of understanding the term "DDF," it is crucial to explore its common usage contexts, as these provide a deeper insight into its meaning and significance. DDF, which stands for "Drug-Device Combination" or "Drug Delivery Formulation," is frequently encountered in the pharmaceutical and medical industries. Here, it refers to products that combine a drug with a device to deliver the medication more effectively. For instance, insulin pumps and inhalers are examples of DDFs where the device is integral to the administration of the drug. In another context, particularly within online communities and dating profiles, DDF can also mean "Drug and Disease Free." This usage is more informal and pertains to personal health status, often used to assure potential partners about one's health condition. This context highlights the importance of transparency and safety in personal relationships. Additionally, DDF may appear in financial discussions, especially concerning bonds and securities. Here, it stands for "Discounted Dividend Flow," a method used in valuing stocks by discounting future dividend payments back to their present value. This financial context underscores the analytical aspect of investment strategies. Understanding these diverse contexts is essential because they illustrate how a single acronym can have multiple meanings depending on the field or community in which it is used. Recognizing these differences helps avoid confusion and ensures clear communication among stakeholders. For example, a healthcare professional discussing DDF in relation to drug delivery would need to distinguish it clearly from someone using the term in a dating profile or financial analysis. By acknowledging these various usage contexts, individuals can better navigate different conversations and ensure that their message is conveyed accurately and effectively. This multifaceted approach to understanding DDF enriches our comprehension of the term and its practical applications across various domains.
Historical Development
The term "DDF" (Drug-Device Combination) has its roots in a historical development that spans several decades, reflecting the evolution of medical technology and regulatory frameworks. In the early 20th century, pharmaceuticals and medical devices were largely treated as separate entities, each with its own set of regulations and development pathways. However, as medical science advanced and the need for more sophisticated treatments grew, the lines between drugs and devices began to blur. One of the pivotal moments in this historical development was the passage of the 1976 Medical Device Amendments to the Federal Food, Drug, and Cosmetic Act in the United States. This legislation established a comprehensive regulatory framework for medical devices, categorizing them into three classes based on their risk level and the level of control required to ensure their safety and effectiveness. This framework laid the groundwork for future innovations that would integrate drugs and devices. The 1980s saw significant advancements in biotechnology and materials science, leading to the development of more complex medical products that combined drug delivery with device technology. For instance, the introduction of drug-eluting stents revolutionized cardiovascular treatment by combining a mechanical device (the stent) with a pharmacological agent (the drug) to prevent restenosis. This innovation marked a turning point in the field, as it necessitated a more integrated approach to product development and regulation. In response to these advancements, regulatory bodies such as the U.S. Food and Drug Administration (FDA) began to adapt their guidelines to accommodate these hybrid products. The FDA established the Office of Combination Products in 2002 to oversee the regulation of products that combine drugs, devices, and biological products. This office ensures that these complex products meet stringent safety and efficacy standards through a coordinated review process involving multiple FDA centers. Throughout the 21st century, the development of DDFs has continued to accelerate, driven by advances in nanotechnology, biomaterials, and precision medicine. Products like insulin pumps, inhalers for respiratory diseases, and implantable drug delivery systems have become commonplace. These innovations have transformed patient care by offering more targeted, efficient, and sometimes even personalized treatment options. The historical development of DDFs is also marked by increased collaboration between pharmaceutical companies, medical device manufacturers, and regulatory agencies. This collaboration has facilitated the sharing of knowledge, resources, and best practices, ultimately speeding up the development process while ensuring that safety and efficacy standards are maintained. In summary, the concept of DDFs is rooted in a rich historical context that reflects the convergence of pharmaceutical science, device technology, and regulatory oversight. From legislative milestones to technological breakthroughs and evolving regulatory frameworks, this journey underscores the continuous effort to improve patient outcomes through innovative medical solutions. Understanding this historical development is crucial for appreciating the complexities and opportunities inherent in the term "DDF" today.
Applications and Implications of DDF
The Applications and Implications of Digital Data Fabric (DDF) are multifaceted and far-reaching, impacting various aspects of modern society. At its core, DDF is a powerful tool that integrates disparate data sources into a unified, accessible framework, enhancing decision-making processes across different sectors. This article delves into three key areas where DDF has significant implications: Financial and Economic Impact, Technological Integration, and Societal and Cultural Influence. Firstly, the financial and economic impact of DDF is substantial. By providing real-time insights and predictive analytics, DDF can optimize business operations, reduce costs, and increase revenue. It enables more accurate risk assessments and investment strategies, which are crucial for financial institutions and businesses alike. This economic efficiency can lead to broader economic growth and stability. Secondly, DDF's technological integration is a game-changer. It leverages advanced technologies such as AI, blockchain, and cloud computing to create a robust and secure data environment. This integration not only improves data management but also fosters innovation by facilitating the development of new applications and services. Lastly, the societal and cultural influence of DDF cannot be overlooked. By democratizing access to data, DDF can empower communities and individuals, promoting transparency and accountability. It also raises important questions about data privacy and ethics, necessitating a balanced approach to its implementation. In this article, we will explore each of these dimensions in detail, starting with the **Financial and Economic Impact** of DDF, where we will examine how it transforms financial landscapes and drives economic prosperity.
Financial and Economic Impact
The financial and economic impact of Dynamic Data Fabric (DDF) is multifaceted and profound, transforming various sectors by enhancing data management, analytics, and decision-making processes. At its core, DDF integrates disparate data sources into a unified, real-time framework, enabling organizations to derive actionable insights more efficiently. This integration has significant implications for financial institutions, where accurate and timely data analysis is crucial for risk assessment, compliance, and investment strategies. For instance, banks can leverage DDF to aggregate customer data from multiple platforms, improving credit scoring models and fraud detection algorithms. This not only reduces operational costs but also enhances customer satisfaction by offering personalized financial services. In the broader economic landscape, DDF plays a pivotal role in fostering innovation and competitiveness. By providing real-time access to market trends and consumer behavior, businesses can respond swiftly to changing market conditions, thereby gaining a competitive edge. This agility is particularly beneficial in industries such as retail and manufacturing, where supply chain optimization is critical. For example, retailers can use DDF to monitor inventory levels, track consumer preferences, and predict demand fluctuations, leading to better inventory management and reduced stockouts or overstocking. Moreover, the economic impact of DDF extends to public policy and governance. Governments can utilize this technology to analyze vast amounts of data related to economic indicators, public health, and social welfare programs. This enables policymakers to make informed decisions that are data-driven rather than anecdotal, leading to more effective policy implementations and resource allocations. For instance, during the COVID-19 pandemic, governments could have used DDF to track infection rates, hospital capacity, and economic activity in real-time, facilitating more targeted and efficient responses. From an investment perspective, DDF also opens up new avenues for venture capital and private equity firms. By analyzing comprehensive datasets that include financial performance metrics, market trends, and competitive landscapes, investors can make more informed decisions about where to allocate their capital. This reduces the risk associated with investments and increases the likelihood of successful outcomes. Furthermore, the adoption of DDF has substantial implications for workforce development and job creation. As organizations increasingly rely on advanced data analytics, there will be a growing demand for professionals skilled in data science, machine learning, and related fields. This could lead to the creation of new job opportunities and the upskilling of existing employees, contributing positively to labor markets. In conclusion, the financial and economic impact of Dynamic Data Fabric is far-reaching and transformative. By enhancing data integration, analytics, and decision-making capabilities across various sectors, DDF drives efficiency, innovation, and competitiveness. Its applications span from financial services and retail to public policy and investment strategies, making it a critical component in the modern digital economy. As technology continues to evolve, the role of DDF in shaping economic outcomes will only become more pronounced.
Technological Integration
Technological integration is a pivotal aspect of modern innovation, particularly in the context of Data-Driven Frameworks (DDF). As organizations increasingly rely on data to drive decision-making, the seamless integration of various technologies becomes essential for maximizing efficiency, accuracy, and scalability. This integration involves the harmonious convergence of disparate systems, tools, and platforms to create a cohesive ecosystem that leverages data analytics, artificial intelligence, cloud computing, and the Internet of Things (IoT). In DDF, technological integration enables real-time data processing and analysis, allowing for swift insights and adaptive strategies. For instance, integrating IoT devices with advanced analytics platforms can provide continuous feedback loops, enhancing operational performance in industries such as manufacturing and logistics. Similarly, the integration of machine learning algorithms with big data repositories facilitates predictive analytics, helping businesses anticipate market trends and customer behaviors more accurately. Moreover, cloud computing plays a crucial role in technological integration by providing scalable infrastructure that supports the storage and processing of vast amounts of data. This cloud-based architecture ensures that data is accessible across different departments and locations, fostering collaboration and reducing silos within organizations. Additionally, the integration of cybersecurity measures with DDF ensures that sensitive data is protected against breaches and unauthorized access, maintaining trust and compliance with regulatory standards. The applications of technological integration in DDF are far-reaching. In healthcare, for example, integrating electronic health records with AI-driven diagnostic tools can lead to more accurate diagnoses and personalized treatment plans. In finance, the integration of blockchain technology with traditional banking systems enhances security and transparency in transactions. These integrations not only improve operational efficiency but also drive innovation by enabling new services and products that were previously unimaginable. However, the implications of such integration are multifaceted. On one hand, it necessitates significant investments in infrastructure and talent acquisition, as well as ongoing training to ensure that employees are adept at using these integrated systems. On the other hand, it opens up new avenues for automation, potentially displacing certain job roles while creating others that require advanced technical skills. In conclusion, technological integration is a cornerstone of DDF, enabling organizations to harness the full potential of their data assets. By integrating diverse technologies into a unified framework, businesses can achieve greater agility, better decision-making capabilities, and enhanced competitiveness in their respective markets. As technology continues to evolve, the importance of seamless integration will only grow, making it imperative for organizations to prioritize this aspect in their strategic planning and development initiatives.
Societal and Cultural Influence
Societal and cultural influences play a pivotal role in shaping the applications and implications of DDF (Data-Driven Frameworks), which are increasingly integral to various sectors such as business, healthcare, education, and governance. These frameworks rely on data analytics to inform decision-making processes, but their effectiveness is deeply intertwined with the societal and cultural contexts in which they are implemented. For instance, in culturally diverse societies, DDFs must be sensitive to local norms and values to avoid biases that could lead to discriminatory outcomes. In healthcare, for example, data-driven models used for patient diagnosis and treatment planning need to account for cultural differences in health behaviors and perceptions of illness. Similarly, in education, DDFs used to personalize learning experiences must consider the socio-cultural backgrounds of students to ensure equitable access to educational resources. Moreover, societal trust in technology and data privacy regulations significantly influence the adoption and impact of DDFs. In regions with stringent data protection laws, such as the European Union's GDPR, DDFs must adhere to strict guidelines to maintain public trust and comply with legal requirements. Conversely, in societies where data privacy is less of a concern, the implementation of DDFs may be more rapid but could also raise ethical dilemmas regarding personal data misuse. Cultural attitudes towards technology also shape how DDFs are perceived and utilized. For example, in some cultures, there is a strong emphasis on human intuition over algorithmic decision-making, which could affect the acceptance of DDFs in certain industries. On the other hand, societies that embrace technological innovation may see faster integration of DDFs across various sectors, leading to more efficient operations and better outcomes. Furthermore, the economic landscape of a society impacts the resources available for developing and implementing DDFs. In developed economies with robust technological infrastructures, there is often greater investment in data analytics and AI, enabling more sophisticated applications of DDFs. In contrast, developing economies might face challenges in implementing these frameworks due to limited resources and infrastructure. The ethical implications of DDFs are another critical area where societal and cultural influences come into play. As these frameworks become more pervasive, there is growing concern about issues like algorithmic bias, job displacement due to automation, and the potential for surveillance states. Societies with strong ethical frameworks and regulatory bodies are better positioned to mitigate these risks compared to those without such safeguards. In conclusion, the applications and implications of DDFs are profoundly influenced by societal and cultural factors. Understanding these influences is crucial for developing and implementing DDFs that are not only effective but also ethical and equitable. By acknowledging and addressing these factors, we can harness the full potential of data-driven frameworks while ensuring they align with the values and needs of diverse societies around the world.
Challenges and Future of DDF
The future of Distributed Data Fabric (DDF) is fraught with both promise and challenge, as it navigates the complex landscape of regulatory compliance, technological innovation, and risk management. As DDF continues to evolve, it must address stringent regulatory challenges that ensure data privacy and security while fostering trust among users. Simultaneously, technological advancements are driving the development of more sophisticated DDF systems, enhancing their efficiency and scalability. However, these advancements also introduce potential risks that must be mitigated through robust security measures and ethical practices. Understanding these interrelated factors is crucial for harnessing the full potential of DDF. This article delves into these critical aspects, starting with the regulatory challenges that form the foundation of a compliant and trustworthy DDF ecosystem. Transitioning to the first of these key areas, regulatory challenges pose a significant hurdle for the widespread adoption of DDF. Ensuring compliance with evolving data protection laws and standards is essential for maintaining public trust and avoiding legal repercussions. This necessitates a thorough examination of current regulations and their implications on DDF implementation. --- **Regulatory Challenges** **Technological Advancements** **Potential Risks and Mitigations**
Regulatory Challenges
**Regulatory Challenges** The development and deployment of Digital Distribution Frameworks (DDF) face significant regulatory challenges that can hinder their adoption and effectiveness. One of the primary issues is the lack of standardized regulations across different jurisdictions. As DDFs often operate in a global context, they must comply with a myriad of local laws and regulations, which can be complex and sometimes conflicting. For instance, data privacy laws such as the General Data Protection Regulation (GDPR) in Europe and the California Consumer Privacy Act (CCPA) in the United States impose stringent requirements on how personal data is collected, processed, and shared. Ensuring compliance with these diverse regulatory frameworks while maintaining operational efficiency is a daunting task. Another challenge arises from the evolving nature of technology itself. Regulatory bodies often struggle to keep pace with the rapid advancements in digital technologies, leading to a gap between the current state of technology and the existing legal frameworks. This can result in outdated regulations that fail to address new issues or provide adequate guidance for emerging technologies. For example, blockchain technology, which is increasingly being integrated into DDFs for enhanced security and transparency, is still largely unregulated in many countries, creating uncertainty for businesses looking to leverage this technology. Moreover, intellectual property rights pose another significant regulatory hurdle. DDFs frequently involve the distribution of digital content, such as software, music, and videos, which are protected by copyright laws. Ensuring that these rights are respected while facilitating seamless distribution can be challenging. Piracy and unauthorized use of digital content remain prevalent issues, and regulatory frameworks must be robust enough to protect creators' rights without stifling innovation. Additionally, cybersecurity regulations are becoming increasingly stringent as DDFs handle sensitive data and operate within critical infrastructure sectors. Compliance with cybersecurity standards and guidelines, such as those set by the National Institute of Standards and Technology (NIST), is crucial but can be resource-intensive. The constant threat of cyber-attacks and data breaches necessitates continuous monitoring and updating of security protocols, adding another layer of complexity to regulatory compliance. Finally, the issue of interoperability between different systems and platforms also presents a regulatory challenge. For DDFs to function efficiently, they must be able to interact seamlessly with various other systems. However, achieving interoperability while adhering to different regulatory standards can be difficult. This requires coordinated efforts among stakeholders, including regulatory bodies, industry players, and technology developers, to establish common standards and guidelines. In summary, the regulatory landscape for DDFs is complex and multifaceted. Addressing these challenges will require collaborative efforts between policymakers, industry leaders, and technology innovators to create a balanced regulatory environment that fosters innovation while ensuring compliance with legal and ethical standards. By navigating these regulatory hurdles effectively, DDFs can realize their full potential in transforming how digital goods and services are distributed globally.
Technological Advancements
**Technological Advancements** In the context of "Challenges and Future of DDF" (Data-Driven Frameworks), technological advancements play a pivotal role in shaping the landscape of data-driven decision-making. DDF, which stands for Data-Driven Frameworks, refers to the structured approaches and methodologies that organizations use to leverage data for strategic planning, operational efficiency, and innovation. The rapid evolution of technology has significantly enhanced the capabilities of DDFs, addressing several challenges while opening up new avenues for future growth. One of the most impactful technological advancements is the rise of **Big Data Analytics**. With the exponential increase in data generation from various sources such as IoT devices, social media, and sensors, big data analytics tools have become indispensable. These tools enable organizations to process and analyze vast amounts of data quickly, providing insights that were previously unattainable. For instance, machine learning algorithms can now predict customer behavior with high accuracy, allowing businesses to tailor their marketing strategies more effectively. **Cloud Computing** has also revolutionized the way DDFs operate by providing scalable, on-demand computing resources. Cloud platforms offer robust infrastructure for data storage, processing, and analytics, reducing the need for expensive hardware and minimizing downtime. This flexibility is crucial for organizations dealing with fluctuating data volumes and computational demands. Another significant advancement is **Artificial Intelligence (AI)** and **Machine Learning (ML)**. AI-driven systems can automate complex tasks within DDFs, such as data cleansing, feature engineering, and model training. ML models can learn from historical data to make predictions and optimize business processes in real-time. For example, AI-powered chatbots can handle customer inquiries more efficiently than human agents, freeing up resources for more strategic activities. **Internet of Things (IoT)** technology has further expanded the scope of DDFs by integrating physical devices into the data ecosystem. IoT devices generate real-time data that can be used to monitor and control operations in industries like manufacturing, healthcare, and logistics. This real-time data enables organizations to make immediate decisions based on current conditions rather than historical trends. Despite these advancements, there are challenges that need to be addressed. **Data Security** remains a critical concern as the volume and sensitivity of data increase. Ensuring the integrity and confidentiality of data is essential to maintain trust and compliance with regulatory standards. Additionally, **Ethical Considerations** around AI and ML must be carefully managed to avoid biases and ensure fairness in decision-making processes. Looking ahead, the future of DDFs is promising but also fraught with challenges. As technology continues to evolve, we can expect even more sophisticated tools for data analysis and decision-making. **Quantum Computing**, for instance, holds the potential to solve complex problems that are currently unsolvable with traditional computing methods. However, this also means that organizations will need to invest in continuous learning and adaptation to stay ahead. In conclusion, technological advancements have transformed the landscape of DDFs by enhancing their capabilities and addressing several challenges. As we move forward, it is crucial for organizations to stay agile and adapt to emerging technologies while addressing the ethical and security concerns associated with them. By doing so, they can fully leverage the potential of DDFs to drive innovation and growth in an increasingly data-driven world.
Potential Risks and Mitigations
**Potential Risks and Mitigations** As the Distributed Data Fabric (DDF) continues to evolve and play a pivotal role in modern data management, it is crucial to acknowledge the potential risks associated with its implementation and operation. One of the primary risks is **data security**. With DDF, data is distributed across multiple nodes, which can increase the attack surface. To mitigate this, robust encryption protocols and access controls must be implemented to ensure that only authorized personnel can access sensitive information. Additionally, regular security audits and penetration testing can help identify vulnerabilities before they are exploited. Another significant risk is **data consistency and integrity**. Since DDF involves distributed data storage, ensuring that all nodes have consistent and up-to-date information can be challenging. This can be addressed through the use of consensus algorithms and real-time data synchronization mechanisms. Furthermore, implementing robust error detection and correction techniques can help maintain data integrity across the network. **Scalability** is another area of concern. As the volume of data grows, DDF systems must be able to scale efficiently to handle increased loads. This can be mitigated by designing systems with modular architecture, allowing for easy addition of new nodes as needed. Cloud-based solutions can also provide on-demand scalability, ensuring that resources are allocated dynamically based on demand. **Interoperability** issues may arise when integrating DDF with existing systems or different data formats. To overcome this, standards-based approaches should be adopted to ensure seamless communication between various components. Developing APIs and data adapters that support multiple formats can facilitate smooth integration and reduce compatibility risks. Moreover, **regulatory compliance** is a critical consideration. DDF systems must adhere to various data protection regulations such as GDPR, HIPAA, and others depending on the jurisdiction. Ensuring compliance involves implementing policies that align with these regulations, conducting regular audits, and maintaining transparent data handling practices. Finally, **vendor lock-in** is a risk that should not be overlooked. Relying heavily on a single vendor for DDF solutions could lead to dependency issues if the vendor experiences disruptions or changes its business model. Mitigating this involves diversifying vendors, using open-source solutions where possible, and maintaining a flexible architecture that allows for easy migration if necessary. In conclusion, while DDF offers numerous benefits in terms of data management and scalability, it is essential to be aware of the potential risks involved. By implementing robust security measures, ensuring data consistency, designing for scalability, fostering interoperability, adhering to regulatory requirements, and avoiding vendor lock-in, organizations can effectively mitigate these risks and fully leverage the capabilities of DDF in their operations. Addressing these challenges proactively will be crucial for the future success and widespread adoption of DDF technologies.