What Is Shock Free Data
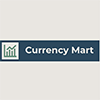
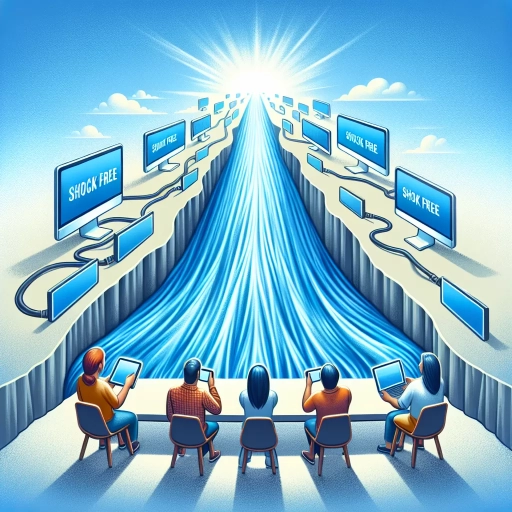
In today's data-driven world, the concept of shock-free data has emerged as a critical component in ensuring the reliability and integrity of information. Shock-free data refers to the ability of a system to maintain consistent and accurate data even in the face of unexpected events or disruptions. This concept is pivotal across various industries, from finance and healthcare to technology and manufacturing. To delve into the essence of shock-free data, it is essential to first understand its underlying principles. The article will explore three key aspects: **Understanding the Concept of Shock-Free Data**, which delves into the foundational elements and definitions; **Technologies and Methods for Achieving Shock-Free Data**, which examines the innovative solutions and strategies employed to achieve this state; and **Applications and Benefits of Shock-Free Data in Various Industries**, highlighting how different sectors leverage this concept to enhance their operations. By grasping these elements, we can better appreciate the significance of shock-free data and its role in maintaining robust and resilient systems. Let us begin by **Understanding the Concept of Shock-Free Data**.
Understanding the Concept of Shock-Free Data
In today's data-driven world, the concept of shock-free data has emerged as a critical component in ensuring the integrity and reliability of information. Understanding this concept involves delving into its definition and origins, examining its key characteristics, and appreciating its importance in modern data management. Shock-free data refers to the ability of a dataset to withstand sudden changes or disruptions without compromising its accuracy or consistency. This concept has its roots in various fields, including statistics and data science, where the need for robust data has been increasingly recognized. By exploring the definition and origins of shock-free data, we can better grasp how it evolved to meet the demands of contemporary data analysis. Key characteristics such as resilience, adaptability, and precision are essential in distinguishing shock-free data from other types of datasets. Moreover, the importance of shock-free data in modern data management cannot be overstated, as it ensures that decisions made based on this data are reliable and sustainable. Let us begin by examining the definition and origins of shock-free data to lay a solid foundation for our understanding.
Definition and Origins
**Definition and Origins** Shock-free data refers to a concept in data management and analytics where the goal is to ensure that data remains consistent, reliable, and free from sudden or unexpected changes that could disrupt analysis or decision-making processes. This concept is crucial in various fields such as finance, healthcare, and engineering, where accurate and stable data is essential for making informed decisions. The origins of shock-free data can be traced back to the early days of data analysis when researchers and analysts began to recognize the importance of data quality and stability. In the 1960s and 1970s, with the advent of computer systems and databases, the need for reliable data became more pronounced. As data collection and storage technologies evolved, so did the methods for ensuring data integrity. One of the key milestones in the development of shock-free data was the introduction of data normalization techniques in the 1980s. Data normalization involves transforming raw data into a consistent format to reduce errors and improve data quality. This practice helped in minimizing sudden changes or "shocks" in datasets, thereby enhancing the reliability of analytical outcomes. In recent years, advancements in big data analytics and machine learning have further emphasized the importance of shock-free data. Modern algorithms are highly sensitive to data quality, and any significant anomalies can lead to biased or inaccurate results. Consequently, techniques such as data cleansing, validation, and regularization have become integral parts of data preprocessing to ensure that datasets are robust and resilient against shocks. The concept of shock-free data also draws from statistical process control (SPC), which was pioneered by Walter Shewhart in the early 20th century. SPC involves monitoring processes to detect any deviations from expected norms, thereby preventing shocks or significant variations that could affect overall performance. In summary, the definition and origins of shock-free data are rooted in the historical evolution of data management practices aimed at ensuring consistency and reliability. From early normalization techniques to modern big data analytics, the pursuit of shock-free data has been driven by the need for accurate and dependable information across various disciplines. Understanding this concept is fundamental to appreciating the broader framework of shock-free data and its critical role in contemporary data-driven decision-making processes.
Key Characteristics
**Key Characteristics of Shock-Free Data** Shock-free data is characterized by several key attributes that ensure its reliability, integrity, and usability. **Consistency** is a fundamental trait, where data remains accurate and uniform across different systems and platforms. This consistency prevents discrepancies that could lead to errors or misinterpretations. **Accuracy** is another critical characteristic, ensuring that the data reflects real-world conditions without any distortions or inaccuracies. **Timeliness** is also essential, as shock-free data must be available in real-time or near real-time to support immediate decision-making processes. **Reliability** is a cornerstone of shock-free data, meaning that the data can be trusted to provide consistent results under various conditions. This reliability extends to the sources of the data, which must be credible and transparent. **Completeness** ensures that all necessary information is included, avoiding gaps that could compromise the overall quality of the data. **Relevance** is crucial as well, ensuring that the data aligns with the specific needs and goals of the users. **Scalability** allows shock-free data to adapt seamlessly to growing demands without compromising its integrity. This scalability is particularly important in dynamic environments where data volumes can fluctuate significantly. **Security** is a vital characteristic, protecting the data from unauthorized access, tampering, or breaches that could compromise its integrity. **Interoperability** enables shock-free data to be shared and used across different systems and applications without losing its value or accuracy. **Transparency** in data collection and processing methods is also a key feature, allowing users to understand how the data was obtained and what it represents. This transparency builds trust and confidence in the data. Finally, **auditability** ensures that changes to the data can be tracked and verified, providing a clear history of modifications and updates. By combining these characteristics, shock-free data becomes a robust and dependable resource for making informed decisions and driving successful outcomes.
Importance in Modern Data Management
In the realm of modern data management, the importance of effective data handling cannot be overstated. As organizations increasingly rely on data-driven decision-making, the integrity, accessibility, and security of their data become paramount. Modern data management involves a multifaceted approach that includes data governance, quality assurance, and robust analytics. Data governance ensures that data is accurate, consistent, and compliant with regulatory standards, thereby reducing the risk of errors and legal repercussions. High-quality data is essential for making informed decisions; poor data quality can lead to misinformed strategies and significant financial losses. Moreover, with the advent of big data and the Internet of Things (IoT), the volume and complexity of data have skyrocketed, necessitating advanced tools and techniques for efficient data processing and analysis. Effective data management also involves ensuring data security and privacy. With cyber threats on the rise, protecting sensitive information from breaches and unauthorized access is critical. This includes implementing robust encryption protocols, access controls, and regular security audits. Additionally, compliance with data protection regulations such as GDPR and CCPA is mandatory to avoid hefty fines and reputational damage. Another crucial aspect is data accessibility. Modern data management systems must be designed to provide seamless access to relevant stakeholders while maintaining appropriate access controls. Cloud-based solutions have become popular due to their scalability, flexibility, and cost-effectiveness in storing and retrieving large volumes of data. These platforms also facilitate real-time collaboration and analytics, enabling organizations to respond swiftly to changing market conditions. In the context of "Understanding the Concept of Shock-Free Data," effective modern data management plays a pivotal role. Shock-free data refers to data that is free from sudden disruptions or anomalies that could skew analysis or decision-making processes. Achieving shock-free data requires rigorous quality checks, continuous monitoring for anomalies, and the implementation of predictive analytics to forecast potential disruptions. By integrating these practices into their data management strategies, organizations can ensure that their data remains reliable and consistent over time. Ultimately, the importance of modern data management lies in its ability to drive business innovation while mitigating risks associated with poor data quality or security breaches. By leveraging advanced technologies such as artificial intelligence (AI) and machine learning (ML), organizations can automate many aspects of data management, enhance data insights, and maintain a competitive edge in today's data-driven economy. In summary, robust modern data management is indispensable for ensuring that an organization's data is accurate, secure, accessible, and shock-free—qualities that are essential for making informed decisions and driving sustainable growth.
Technologies and Methods for Achieving Shock-Free Data
In the era of rapid technological advancement, ensuring shock-free data has become a paramount concern for organizations across various sectors. Achieving this goal involves a multifaceted approach that integrates several key technologies and methods. Data encryption and security protocols are fundamental in safeguarding sensitive information from unauthorized access and breaches. Real-time data processing and analytics enable swift detection and response to potential threats, allowing for proactive measures to maintain data integrity. Additionally, cloud computing and distributed systems provide scalable and resilient infrastructure, ensuring that data remains accessible and secure even in the face of disruptions. By leveraging these technologies, organizations can significantly reduce the risk of data shocks. This article will delve into these critical components, starting with the cornerstone of data protection: data encryption and security protocols.
Data Encryption and Security Protocols
Data encryption and security protocols are crucial components in achieving shock-free data, ensuring that sensitive information remains protected and secure from unauthorized access. Data encryption involves converting plaintext data into unreadable ciphertext using algorithms such as Advanced Encryption Standard (AES) and RSA. This process ensures that even if data is intercepted, it cannot be deciphered without the decryption key. For instance, symmetric encryption methods like AES are widely used for bulk data encryption due to their efficiency and speed, while asymmetric encryption methods like RSA are often used for secure key exchange. Security protocols further enhance data protection by establishing standardized procedures for secure communication. Transport Layer Security (TLS) and Secure Sockets Layer (SSL) protocols, for example, are used to secure web traffic between servers and clients, preventing eavesdropping and tampering. Additionally, protocols like Secure/Multipurpose Internet Mail Extensions (S/MIME) and Pretty Good Privacy (PGP) are employed to secure email communications. Another key aspect is the implementation of access control mechanisms, such as role-based access control (RBAC) and multi-factor authentication (MFA), which restrict access to authorized personnel only. These measures prevent unauthorized users from accessing sensitive data, thereby reducing the risk of data breaches. Regular updates and patches to software and systems are also essential for maintaining robust security. Vulnerability management involves identifying and mitigating potential vulnerabilities before they can be exploited by malicious actors. This proactive approach ensures that any weaknesses in the system are addressed promptly, minimizing the risk of security breaches. Compliance with industry standards and regulations, such as the General Data Protection Regulation (GDPR) and the Payment Card Industry Data Security Standard (PCI DSS), is another critical aspect of data security. Adhering to these standards ensures that organizations follow best practices in data protection, thereby enhancing overall security posture. In summary, a combination of robust data encryption algorithms, stringent security protocols, access control mechanisms, regular system updates, and compliance with industry standards are essential for achieving shock-free data. These measures collectively ensure that sensitive information remains secure and protected against various threats, providing a robust defense against potential security breaches.
Real-Time Data Processing and Analytics
Real-time data processing and analytics are pivotal in achieving shock-free data, enabling organizations to respond swiftly and accurately to changing conditions. This technology involves the immediate collection, processing, and analysis of data as it is generated, allowing for instantaneous insights and decision-making. Key technologies driving real-time data processing include streaming data platforms such as Apache Kafka, Apache Storm, and Amazon Kinesis, which handle high-volume data streams efficiently. Additionally, in-memory computing solutions like SAP HANA and Apache Ignite accelerate data processing by storing data in RAM rather than on disk, significantly reducing latency. Advanced analytics tools, including machine learning algorithms and predictive analytics, are integral to real-time data processing. These tools analyze incoming data streams to identify patterns, anomalies, and trends in real-time, enabling proactive measures to mitigate potential disruptions. For instance, in financial services, real-time analytics can detect fraudulent transactions instantly, preventing financial losses. In healthcare, real-time monitoring of patient data can alert medical staff to critical changes in patient condition, ensuring timely interventions. The methodological approach to real-time data processing involves several steps: data ingestion from various sources, data processing using distributed computing frameworks like Apache Spark or Hadoop, and data visualization through dashboards and reports. Data quality checks are also crucial to ensure that the data being processed is accurate and reliable. This is often achieved through data validation rules and cleansing processes that run in parallel with the real-time processing pipeline. Moreover, cloud-based solutions such as AWS Lambda and Google Cloud Functions provide scalable infrastructure for real-time data processing, allowing organizations to handle variable workloads without significant upfront investment. These serverless architectures automatically scale resources based on demand, ensuring that the system remains responsive even during peak loads. In summary, real-time data processing and analytics are essential for achieving shock-free data by providing immediate insights that enable prompt action. By leveraging cutting-edge technologies and methodologies, organizations can maintain operational stability and make informed decisions in a rapidly changing environment. This capability is particularly valuable in sectors where timely responses are critical, such as finance, healthcare, and logistics, where delays can have significant consequences.
Cloud Computing and Distributed Systems
Cloud computing and distributed systems are pivotal technologies in achieving shock-free data, ensuring seamless and resilient data management. Cloud computing leverages a network of remote servers accessed over the internet to store, manage, and process data, rather than a local server or personal computer. This model allows for on-demand scalability, reduced costs, and enhanced reliability. Distributed systems, on the other hand, involve multiple interconnected nodes that work together to achieve common goals, such as data processing and storage. These systems are designed to be fault-tolerant and highly available, making them ideal for handling large volumes of data without interruptions. In the context of achieving shock-free data, cloud computing offers several key benefits. Firstly, cloud providers like AWS, Azure, and Google Cloud offer robust infrastructure with built-in redundancy and failover mechanisms. This ensures that data remains accessible even in the event of hardware failures or natural disasters. Secondly, cloud services provide automatic backups and disaster recovery options, which can be configured to run at regular intervals, thereby minimizing data loss. Additionally, cloud-based solutions often include advanced security features such as encryption, firewalls, and access controls to protect against cyber threats. Distributed systems further enhance the reliability of data management by distributing data across multiple nodes. This distribution allows for parallel processing and storage, which not only speeds up operations but also ensures that no single point of failure can bring down the entire system. Technologies like Hadoop and Apache Cassandra are examples of distributed systems that are designed for big data processing and storage. These systems use replication techniques to ensure that data is duplicated across multiple nodes, so if one node fails, others can take over without any disruption. Moreover, distributed systems can be configured to operate in different geographical locations, providing a global reach and reducing latency for users. This geographic distribution also helps in achieving higher availability since data can be accessed from multiple locations simultaneously. For instance, if a data center in one region experiences an outage due to a natural disaster or technical issue, users can still access their data from another region without any interruption. In summary, the combination of cloud computing and distributed systems provides a robust framework for achieving shock-free data. By leveraging the scalability, redundancy, and fault tolerance offered by these technologies, organizations can ensure that their data remains secure, accessible, and resilient against various types of disruptions. This is crucial in today's digital age where continuous access to data is essential for business operations and decision-making processes. Therefore, integrating cloud computing and distributed systems into data management strategies is a key step towards achieving shock-free data.
Applications and Benefits of Shock-Free Data in Various Industries
The integration of shock-free data across various industries has revolutionized the way businesses operate, enhancing efficiency, security, and decision-making. In the financial sector, shock-free data plays a crucial role in risk management and compliance, enabling institutions to predict and mitigate potential financial risks more accurately. In healthcare, it ensures patient data security and privacy, safeguarding sensitive information while facilitating better patient care. Meanwhile, in manufacturing, shock-free data drives predictive maintenance and quality control, optimizing production processes and reducing downtime. These applications underscore the transformative power of reliable, real-time data. By leveraging shock-free data, industries can navigate complexities with greater precision and confidence. Transitioning to the financial sector, we explore how risk management and compliance are significantly enhanced through the use of shock-free data, providing a robust framework for financial stability and regulatory adherence.
Financial Sector: Risk Management and Compliance
In the financial sector, risk management and compliance are paramount to maintaining stability and trust. The integration of shock-free data plays a crucial role in enhancing these processes. Shock-free data, characterized by its reliability, consistency, and real-time accuracy, helps financial institutions mitigate risks more effectively. For instance, advanced analytics powered by shock-free data enable institutions to predict market volatility and potential risks with greater precision. This allows for proactive measures to be taken, such as diversifying portfolios or implementing hedging strategies, thereby reducing the likelihood of significant financial losses. Compliance is another area where shock-free data excels. Regulatory requirements are stringent in the financial sector, and non-compliance can result in severe penalties. Shock-free data ensures that all transactions and operations are accurately recorded and reported in real-time, reducing the risk of errors or omissions that could lead to non-compliance. Automated compliance systems leveraging shock-free data can monitor transactions continuously, flagging any anomalies or potential violations before they become issues. This not only helps in adhering to regulatory standards but also enhances transparency and accountability within the organization. Moreover, shock-free data supports anti-money laundering (AML) and know-your-customer (KYC) protocols by providing a clear and consistent view of customer transactions and activities. This helps in identifying suspicious patterns more accurately, allowing financial institutions to take timely action against fraudulent activities. The reliability of shock-free data also fosters better decision-making at all levels of the organization, from risk assessment to investment strategies, ensuring that decisions are based on accurate and up-to-date information. In addition, the use of shock-free data in risk management and compliance can lead to cost savings. By reducing the need for manual intervention and minimizing errors, financial institutions can lower operational costs associated with risk management and compliance. Furthermore, the enhanced accuracy and timeliness of shock-free data can improve customer satisfaction by ensuring that transactions are processed efficiently and securely. Overall, the application of shock-free data in the financial sector's risk management and compliance functions is transformative. It enhances predictive capabilities, ensures regulatory adherence, supports AML/KYC protocols, improves decision-making, and reduces operational costs. As the financial landscape continues to evolve with increasing complexity and regulatory scrutiny, the role of shock-free data will become even more critical in maintaining the integrity and stability of financial systems.
Healthcare: Patient Data Security and Privacy
In the realm of healthcare, patient data security and privacy are paramount. The sensitive nature of medical information necessitates robust safeguards to protect against unauthorized access, breaches, and misuse. This is particularly crucial in an era where digital health records are becoming the norm, offering convenience but also increasing vulnerability. Healthcare providers must adhere to stringent regulations such as the Health Insurance Portability and Accountability Act (HIPAA) in the United States, which mandates the secure handling of protected health information (PHI). This includes implementing advanced encryption methods for both data in transit and at rest, ensuring that only authorized personnel have access to patient records through multi-factor authentication and role-based access controls. Moreover, healthcare organizations are increasingly leveraging cutting-edge technologies like blockchain and artificial intelligence to enhance data security. Blockchain technology, for instance, provides an immutable ledger that can track all transactions related to patient data, making it easier to detect any unauthorized changes or breaches. Artificial intelligence can help in monitoring systems for anomalies and predicting potential security threats before they occur. The benefits of securing patient data extend beyond compliance; they also foster trust between patients and healthcare providers. When patients feel confident that their personal and medical information is safe, they are more likely to share accurate and comprehensive details, leading to better diagnosis and treatment outcomes. Additionally, secure data management enables seamless sharing of information among healthcare providers, facilitating coordinated care and reducing errors. In the context of shock-free data, ensuring the integrity and confidentiality of patient data is essential for maintaining the reliability and accuracy of health records. Shock-free data refers to data that remains consistent and reliable across different systems and environments without experiencing significant disruptions or losses. In healthcare, this means that patient data must be accurately captured, stored, and transmitted without any corruption or loss, which is critical for making informed clinical decisions. By integrating shock-free data principles into healthcare systems, organizations can ensure that patient information remains consistent and reliable across various platforms and devices. This not only enhances patient safety but also improves the overall efficiency of healthcare services by reducing errors and delays associated with data inconsistencies. In conclusion, the security and privacy of patient data are foundational elements in modern healthcare. By leveraging advanced technologies and adhering to strict regulatory standards, healthcare providers can safeguard sensitive information while ensuring the integrity and reliability of health records. This aligns perfectly with the concept of shock-free data, where maintaining consistent and accurate information is crucial for delivering high-quality care.
Manufacturing: Predictive Maintenance and Quality Control
In the realm of manufacturing, predictive maintenance and quality control are pivotal in ensuring operational efficiency, reducing downtime, and enhancing product reliability. Predictive maintenance leverages advanced technologies such as IoT sensors, machine learning algorithms, and data analytics to forecast potential equipment failures before they occur. By monitoring real-time data on machinery performance, manufacturers can schedule maintenance during less critical periods, thereby minimizing disruptions to production. This proactive approach not only extends the lifespan of equipment but also reduces maintenance costs and improves overall plant reliability. Quality control, on the other hand, is a systematic process aimed at ensuring that products meet specified standards and requirements. Advanced data analytics and machine learning play crucial roles here as well. For instance, quality control systems can analyze data from various stages of production to identify patterns or anomalies that might indicate potential defects. This allows for early intervention and correction, significantly reducing the likelihood of defective products reaching the market. Additionally, quality control data can be used to optimize production processes, leading to improved product consistency and reduced waste. The integration of shock-free data—a concept that ensures data integrity and accuracy without interruptions or losses—further enhances these processes. Shock-free data ensures that all critical information is captured and analyzed continuously, providing a comprehensive view of manufacturing operations. This uninterrupted flow of data is essential for maintaining the accuracy of predictive models and ensuring that quality control measures are always effective. In industries where even minor disruptions can have significant impacts, such as automotive or aerospace manufacturing, the reliability of shock-free data is paramount. Moreover, the applications of predictive maintenance and quality control extend beyond immediate operational benefits. They contribute to a broader strategy of digital transformation within manufacturing. By harnessing the power of data analytics and machine learning, manufacturers can move towards Industry 4.0 standards, characterized by smart factories and interconnected systems. This not only improves current processes but also opens up new avenues for innovation and growth. In summary, predictive maintenance and quality control are essential components of modern manufacturing, significantly enhanced by the concept of shock-free data. These technologies collectively drive efficiency, reliability, and innovation, positioning manufacturers for success in an increasingly competitive and technologically advanced landscape.