What Is A Quantitative Observation
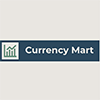
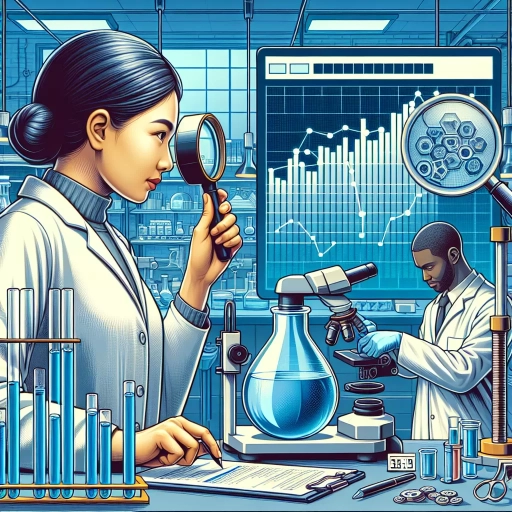
In the realm of scientific inquiry and data collection, quantitative observation stands as a cornerstone for gathering precise and measurable data. This method is crucial for researchers seeking to understand phenomena through numerical values, allowing for the analysis and comparison of data points. The article delves into the essence of quantitative observation, starting with its **Definition and Scope**, where we explore what constitutes this type of observation and its boundaries within various fields. We then examine **Methods and Tools for Conducting Quantitative Observations**, highlighting the techniques and instruments used to ensure accuracy and reliability. Finally, we discuss the **Importance and Challenges in Quantitative Observation**, shedding light on why this method is indispensable and the obstacles researchers may face. By understanding these aspects, readers will gain a comprehensive insight into the world of quantitative observation, beginning with its fundamental definition and scope.
Definition and Scope of Quantitative Observation
Quantitative observation is a methodological approach in research that involves the systematic collection and analysis of numerical data to understand phenomena. This approach is distinct from qualitative observation, which focuses on non-numerical data and interpretive analysis. In this article, we will delve into the definition and scope of quantitative observation, highlighting its key characteristics and applications across various fields. We will begin by distinguishing quantitative observation from its qualitative counterpart, emphasizing the unique methodologies and outcomes of each. Next, we will explore the key characteristics of quantitative data, including its precision, reliability, and generalizability. Finally, we will discuss the diverse applications of quantitative observation in fields such as psychology, economics, and healthcare, demonstrating its versatility and impact. By understanding these aspects, researchers can effectively utilize quantitative observation to gather robust and actionable insights. Let us start by examining the distinction between quantitative and qualitative observation, a crucial step in appreciating the unique value of each method.
Distinction from Qualitative Observation
**Distinction from Qualitative Observation** Quantitative observation stands in stark contrast to qualitative observation, primarily in its approach, methodology, and outcomes. While qualitative observation focuses on gathering detailed, contextual data through methods such as interviews, focus groups, and open-ended surveys, quantitative observation relies on numerical data and statistical analysis. In quantitative observation, researchers aim to measure variables using structured instruments like questionnaires with closed-ended questions, experiments, and surveys designed to yield numerical responses. This approach allows for the collection of large datasets that can be analyzed using statistical techniques to identify patterns, trends, and correlations. Unlike qualitative research, which seeks to understand the "why" behind phenomena through rich, descriptive data, quantitative research aims to answer "how many" or "how often" questions. For instance, in a study on student performance, a qualitative researcher might conduct in-depth interviews to understand the factors influencing academic success, whereas a quantitative researcher would use standardized tests and surveys to measure performance metrics such as grades and attendance rates. The data from quantitative observations are typically more generalizable to larger populations due to their systematic and replicable nature. Moreover, the tools used in quantitative observation are designed for precision and reliability. Researchers employ instruments like Likert scales, multiple-choice questions, and rating systems that ensure consistency across responses. This consistency enables the application of statistical methods to draw conclusions about the population being studied. In contrast, qualitative tools are more flexible but less structured, allowing for deeper insights into individual experiences but making generalization more challenging. The distinction also extends to the role of the researcher. In quantitative studies, the researcher acts as an objective observer, minimizing personal bias by adhering strictly to predefined protocols. In qualitative studies, the researcher often becomes an active participant, engaging closely with the subjects to gather nuanced information. This difference in researcher involvement significantly impacts the type of data collected and the conclusions drawn. In summary, while qualitative observation delves into the complexities of human experiences through detailed narratives, quantitative observation seeks to quantify phenomena using numerical data. This distinction is crucial for understanding the scope and application of each method within the broader context of research methodologies. By recognizing these differences, researchers can select the most appropriate approach based on their research questions and objectives, ensuring that their findings are robust and meaningful.
Key Characteristics of Quantitative Data
Quantitative data is characterized by several key attributes that distinguish it from qualitative data and make it invaluable for scientific and statistical analysis. **Precision** is a fundamental characteristic, as quantitative data is expressed in numerical form, allowing for exact measurements and comparisons. This precision enables researchers to perform various mathematical operations, such as addition, subtraction, multiplication, and division, which are crucial for statistical analysis. **Objectivity** is another critical feature; quantitative data is collected using standardized methods and instruments, reducing personal biases and ensuring that the data collected is consistent and reliable. This objectivity enhances the credibility of the findings, making them more generalizable to larger populations. Quantitative data also exhibits **repeatability**, meaning that if the same measurement is taken multiple times under the same conditions, the results should be consistent. This repeatability is essential for establishing reliability and validity in research studies. Furthermore, quantitative data is **scalable**, allowing researchers to aggregate and analyze large datasets efficiently. This scalability is particularly useful in fields like economics, where trends and patterns over time need to be identified across vast populations. Another key characteristic is **comparability**; because quantitative data is numerical, it can be easily compared across different groups or over time. This comparability facilitates the identification of trends, correlations, and causal relationships, which are essential for drawing meaningful conclusions. In addition, quantitative data supports **hypothesis testing** through statistical methods such as t-tests, ANOVA, and regression analysis. These statistical tools enable researchers to test hypotheses rigorously and determine whether observed differences are statistically significant. The **granularity** of quantitative data also deserves mention; it can range from coarse to fine, depending on the level of detail required. For instance, while some studies may require precise measurements down to several decimal places, others might only need broad categories. Lastly, quantitative data is highly **amenable to visualization**, which aids in communicating complex information in a clear and concise manner. Graphs, charts, and other visual tools help researchers and stakeholders understand patterns and trends more intuitively. In summary, the key characteristics of quantitative data—precision, objectivity, repeatability, scalability, comparability, support for hypothesis testing, granularity, and amenability to visualization—make it a powerful tool for scientific inquiry and decision-making across various disciplines. These attributes ensure that quantitative data provides a robust foundation for rigorous analysis and reliable conclusions.
Applications Across Various Fields
Quantitative observation, a methodical approach to collecting numerical data, has far-reaching applications across various fields. In **medicine**, quantitative observations are crucial for clinical trials, where precise measurements of patient outcomes, such as blood pressure or medication efficacy, are essential for drawing reliable conclusions. **Economics** relies heavily on quantitative data to analyze market trends, GDP growth, and inflation rates, enabling policymakers to make informed decisions. In **psychology**, researchers use quantitative methods to measure cognitive functions, emotional responses, and behavioral patterns, providing insights into human behavior and mental health. **Environmental science** benefits from quantitative observations in monitoring climate change indicators like temperature, sea levels, and pollution levels, which help in predicting future environmental impacts. **Engineering** and **technology** fields utilize quantitative data to optimize system performance, test materials, and ensure safety standards are met. For instance, in aerospace engineering, precise measurements of stress, strain, and aerodynamic forces are critical for designing safe and efficient aircraft. **Social sciences** also employ quantitative methods to study demographic trends, social behaviors, and policy impacts, helping to understand societal dynamics and develop effective policies. Additionally, **education** research often involves quantitative observations to assess learning outcomes, teacher effectiveness, and educational program impacts. These diverse applications underscore the importance of quantitative observation in providing objective, measurable data that can be analyzed statistically to draw meaningful conclusions and drive decision-making processes.
Methods and Tools for Conducting Quantitative Observations
Conducting quantitative observations is a meticulous process that relies on several key methods and tools to ensure accuracy and reliability. At the heart of any quantitative study are the measurement instruments and scales that researchers use to collect data. These tools must be carefully selected and validated to ensure they capture the desired variables accurately. Additionally, statistical analysis techniques play a crucial role in interpreting the collected data, allowing researchers to draw meaningful conclusions. Technology and software also significantly enhance the data collection process, offering advanced tools for data management, analysis, and visualization. By combining these elements—measurement instruments, statistical analysis, and technological tools—researchers can conduct robust and informative quantitative observations. This article will delve into each of these components, starting with the critical role of measurement instruments and scales in setting the foundation for reliable data collection.
Use of Measurement Instruments and Scales
In the realm of quantitative observations, the use of measurement instruments and scales is paramount for collecting accurate and reliable data. These tools enable researchers to quantify variables, ensuring that observations are systematic, consistent, and reproducible. Measurement instruments can range from simple tools like thermometers and rulers to complex devices such as spectrophotometers and psychometric tests. Each instrument is designed to measure specific attributes or phenomena, such as temperature, length, light absorption, or cognitive abilities. Scales, on the other hand, provide a framework for categorizing and quantifying data. They can be nominal (categorizing without implying order), ordinal (implying order but not equal intervals), interval (having equal intervals but no true zero point), or ratio (having equal intervals and a true zero point). For instance, a Likert scale is an ordinal scale used in surveys to measure attitudes or opinions on a continuum from strongly disagree to strongly agree. Interval and ratio scales offer more precision; for example, temperature measured in Celsius or Fahrenheit is an interval scale, while weight measured in kilograms is a ratio scale. The choice of measurement instrument and scale depends on the research question and the nature of the data being collected. For instance, in psychology, standardized tests like IQ tests use complex scales to measure cognitive abilities accurately. In physics, precise instruments like micrometers are used to measure small lengths with high accuracy. The reliability and validity of these instruments are crucial; reliability refers to the consistency of measurements over time, while validity ensures that the instrument measures what it is supposed to measure. Calibration of measurement instruments is also essential to ensure accuracy. This involves comparing the readings of the instrument against known standards to adjust for any deviations. Regular maintenance and periodic recalibration help maintain the precision of these tools over time. Furthermore, the use of measurement instruments and scales facilitates data analysis. Quantitative data collected through these tools can be subjected to statistical analysis, allowing researchers to draw meaningful conclusions and make informed decisions. For example, in medical research, blood pressure measurements using a sphygmomanometer can be analyzed statistically to identify trends and correlations with other health indicators. In summary, measurement instruments and scales are indispensable in quantitative observations, enabling researchers to collect precise, reliable, and meaningful data. By selecting appropriate instruments and scales tailored to their research objectives, researchers can ensure the integrity and usefulness of their findings. This meticulous approach underscores the importance of methodological rigor in quantitative research, ultimately contributing to the advancement of knowledge across various disciplines.
Statistical Analysis Techniques
Statistical analysis techniques are fundamental tools for conducting quantitative observations, enabling researchers to extract meaningful insights from data. These techniques involve various methods to summarize, analyze, and interpret numerical data. **Descriptive statistics** provide an overview of the data set, including measures such as mean, median, mode, and standard deviation, which help in understanding the central tendency and variability of the data. **Inferential statistics**, on the other hand, allow researchers to make conclusions about a population based on a sample of data. This includes hypothesis testing and confidence intervals, which are crucial for determining whether observed differences are statistically significant. **Regression analysis** is another powerful technique used to model the relationship between variables. Simple linear regression examines the relationship between one independent variable and one dependent variable, while multiple linear regression involves more than one independent variable. **Correlation analysis** measures the strength and direction of the linear relationship between two variables, often quantified using Pearson's correlation coefficient. For categorical data, **chi-square tests** are employed to determine if there is a significant association between two variables. **ANOVA (Analysis of Variance)** and **MANOVA (Multivariate Analysis of Variance)** are used to compare means among three or more groups to see if at least one group mean is different from the others. **Time series analysis** is used for data that varies over time, helping in identifying trends, seasonal patterns, and anomalies. **Survival analysis** is particularly useful in fields like medicine and engineering to analyze the time until an event occurs. Advanced statistical techniques include **machine learning algorithms** such as clustering, decision trees, and neural networks, which can handle complex datasets and uncover hidden patterns. **Bayesian statistics** provide a framework for updating probabilities based on new data, offering a flexible approach to statistical inference. In addition to these techniques, various software tools and programming languages like R, Python (with libraries such as Pandas and Scikit-learn), SPSS, and SAS are essential for conducting statistical analyses efficiently. These tools facilitate data manipulation, visualization, and modeling processes, making it easier to apply statistical methods to real-world problems. Overall, mastering statistical analysis techniques is essential for any researcher aiming to derive actionable insights from quantitative data. By selecting the appropriate statistical method and leveraging the right tools, researchers can ensure that their findings are robust, reliable, and meaningful.
Technology and Software in Data Collection
In the realm of quantitative observations, technology and software play a pivotal role in data collection, enhancing both the efficiency and accuracy of the process. Advanced tools such as mobile apps, web-based platforms, and specialized software suites have revolutionized how researchers gather, analyze, and interpret data. For instance, mobile apps like SurveyMonkey and Google Forms enable researchers to create and distribute surveys quickly, allowing for real-time data collection from diverse populations. These tools often include features such as automated data entry, which reduces manual errors and speeds up the analysis phase. Moreover, software solutions like SPSS, R, and Python libraries (e.g., Pandas, NumPy) are indispensable for statistical analysis. These platforms offer robust capabilities for data manipulation, modeling, and visualization, facilitating deeper insights into the collected data. For example, SPSS provides advanced statistical procedures including regression analysis and factor analysis, while R and Python libraries offer extensive libraries for machine learning and data visualization. Additionally, technologies such as IoT devices and sensors are increasingly being used to collect quantitative data in various fields. In environmental studies, sensors can monitor parameters like temperature, humidity, and air quality continuously. Similarly, in healthcare research, wearable devices can track vital signs and physical activity levels over extended periods. Data management systems (DMS) and customer relationship management (CRM) tools also contribute significantly to quantitative observation by organizing and structuring large datasets efficiently. These systems ensure data integrity by enforcing consistency checks and validation rules during entry. Furthermore, cloud-based services like AWS and Google Cloud provide scalable infrastructure for storing and processing large datasets securely. These platforms offer advanced analytics services such as Amazon SageMaker or Google Cloud AI Platform that can handle complex computations required for quantitative analysis. In summary, technology and software are essential components of modern quantitative observation methods. They streamline data collection processes through automated tools, enhance analytical capabilities with sophisticated statistical software, and ensure robust data management practices. By leveraging these technologies effectively, researchers can conduct more precise and comprehensive quantitative observations than ever before.
Importance and Challenges in Quantitative Observation
Quantitative observation is a cornerstone of scientific research, offering a systematic and data-driven approach to understanding phenomena. However, its importance is often underscored by the challenges it presents. This article delves into the critical aspects of quantitative observation, highlighting three key areas: enhancing objectivity and reliability, addressing potential biases and errors, and interpreting and communicating results effectively. Enhancing objectivity and reliability is fundamental to the integrity of quantitative research. By employing rigorous methodologies and standardized protocols, researchers can minimize subjective influences and ensure that findings are consistent and reproducible. This not only strengthens the validity of the data but also builds trust within the scientific community. Addressing potential biases and errors is another crucial challenge. Researchers must be vigilant in identifying and mitigating sources of bias, whether they arise from sampling methods, measurement tools, or data analysis techniques. Moreover, acknowledging and correcting errors is essential for maintaining the accuracy and credibility of the research. Finally, interpreting and communicating results effectively is vital for translating quantitative findings into meaningful insights. This involves using appropriate statistical methods to analyze data, drawing clear conclusions based on evidence, and presenting results in a clear and accessible manner. By focusing on these three areas—enhancing objectivity and reliability, addressing potential biases and errors, and interpreting and communicating results effectively—researchers can ensure that their quantitative observations contribute significantly to the advancement of knowledge. Let us begin by examining how enhancing objectivity and reliability sets the foundation for robust quantitative research.
Enhancing Objectivity and Reliability
Enhancing objectivity and reliability is crucial in quantitative observation, as it ensures that the data collected is accurate, unbiased, and consistent. To achieve this, researchers must employ several strategies. First, **standardized protocols** should be used to ensure that all observations are conducted in the same manner, reducing variability and personal biases. This involves clearly defining the variables to be measured and the methods for data collection. **Training observers** is another key step; observers should be educated on the observation techniques and criteria to minimize subjective interpretations. **Inter-rater reliability** checks, where multiple observers independently record data on the same subjects, help identify and correct discrepancies. Additionally, **pilot studies** can be conducted to test the observation tools and procedures, allowing for refinements before the main study. **Automated data collection tools**, such as sensors or cameras, can also be used to reduce human error and increase precision. Furthermore, **data validation** processes should be implemented to verify the accuracy of the collected data. By combining these methods, researchers can significantly enhance the objectivity and reliability of their quantitative observations, thereby increasing the validity and generalizability of their findings. This is particularly important because reliable data forms the foundation of any scientific inquiry, enabling robust conclusions and informed decision-making. In the context of quantitative observation, maintaining high standards of objectivity and reliability addresses many of the challenges associated with this method, such as ensuring consistency across different observers and settings, which ultimately contributes to the overall quality and impact of the research.
Addressing Potential Biases and Errors
Addressing potential biases and errors is a critical component of ensuring the reliability and validity of quantitative observations. Biases can arise from various sources, including the observer's own preconceptions, the selection of participants, and the design of the observation protocol. To mitigate these biases, researchers must employ rigorous methodologies. For instance, using multiple observers and inter-rater reliability checks can help identify and correct for individual biases. Additionally, random sampling techniques should be utilized to ensure that the observed sample is representative of the population being studied. Another key strategy is to use objective measurement tools and standardized protocols to minimize subjective interpretations. This could involve using automated data collection systems or well-defined criteria for coding and categorizing observed behaviors. Furthermore, pilot studies can be conducted to test the observation instruments and procedures, allowing for necessary adjustments before the main study. Errors in quantitative observation can also stem from data collection and analysis processes. To address this, researchers should implement robust data validation procedures to detect and correct errors in real-time. Regular training sessions for observers can also enhance their accuracy and consistency. Moreover, employing statistical methods such as reliability analysis and sensitivity analysis can help identify potential errors and assess the robustness of the findings. Incorporating triangulation methods—where multiple data sources or methods are used to validate findings—can further enhance the credibility of quantitative observations. This approach helps to cross-validate results and provides a more comprehensive understanding of the phenomenon being observed. Ultimately, addressing potential biases and errors requires a systematic and transparent approach. By documenting all steps of the observation process, including any challenges encountered and solutions implemented, researchers can ensure that their findings are trustworthy and generalizable. This meticulous attention to detail not only strengthens the validity of quantitative observations but also contributes to the advancement of knowledge in various fields by providing reliable data that can be built upon by future studies.
Interpreting and Communicating Results Effectively
Interpreting and communicating results effectively is a crucial step in the process of quantitative observation, as it ensures that the data collected is translated into meaningful insights that can inform decision-making and drive action. Effective interpretation involves several key steps: first, ensuring that the data is accurately analyzed using appropriate statistical methods to uncover patterns, trends, and correlations. This analysis must be grounded in a clear understanding of the research question or hypothesis being tested. Once the data has been analyzed, it is essential to contextualize the findings within the broader framework of existing literature and knowledge in the field. This helps to validate the results and provide a deeper understanding of their significance. Clear communication of these results is equally important. Researchers should present their findings in a manner that is transparent, concise, and accessible to their audience. This often involves using visual aids such as graphs, charts, and tables to illustrate complex data points in an intuitive way. The narrative accompanying these visuals should be free of jargon and technical terms that might confuse non-experts, while still maintaining the integrity of the scientific rigor involved in the study. Moreover, effective communication requires an awareness of the potential biases and limitations inherent in the data collection and analysis process. Acknowledging these limitations can enhance the credibility of the research by demonstrating a commitment to objectivity and transparency. Finally, communicating results in a way that highlights their practical implications can make them more relevant and actionable for stakeholders. For instance, in a business context, interpreting sales data to identify trends can help inform marketing strategies; in healthcare, analyzing patient outcomes can guide treatment protocols. In summary, interpreting and communicating results effectively is pivotal for maximizing the value of quantitative observations. By ensuring accurate analysis, contextualizing findings, presenting data clearly, acknowledging limitations, and highlighting practical implications, researchers can turn raw data into actionable insights that drive meaningful change. This process not only enhances the utility of quantitative observations but also builds trust among stakeholders by demonstrating a commitment to rigorous methodology and clear communication.