What Is Mva
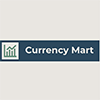
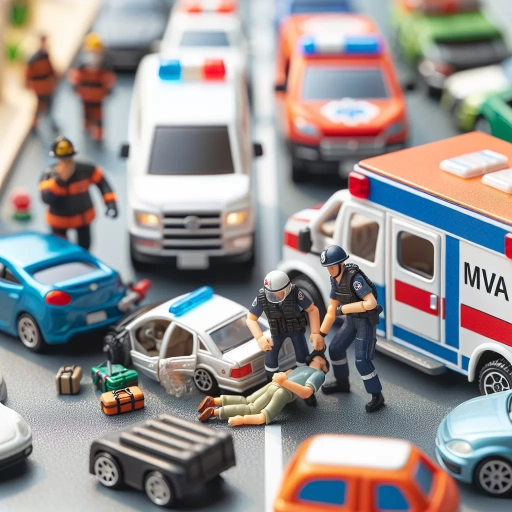
In the realm of data analysis and machine learning, the concept of Minimum Volume Analysis (MVA) has emerged as a powerful tool for extracting insights from complex datasets. MVA is a sophisticated technique that helps in identifying the most informative features and reducing data dimensionality, thereby enhancing the efficiency and accuracy of various analytical processes. This article delves into the multifaceted nature of MVA, starting with **Understanding the Basics of MVA**, where we explore the fundamental principles and definitions that underpin this methodology. We then delve into **Technical Aspects of MVA**, examining the algorithms and computational methods involved in its implementation. Finally, we discuss **Practical Uses and Benefits of MVA**, highlighting how this technique is applied in real-world scenarios to drive decision-making and improve outcomes. By grasping these core aspects, readers will gain a comprehensive understanding of MVA's role in modern data science. Let's begin by understanding the basics of MVA.
Understanding the Basics of MVA
Understanding the basics of Multivariate Analysis (MVA) is crucial for anyone looking to delve into the world of data science and statistical analysis. MVA is a powerful tool that allows researchers and analysts to examine multiple variables simultaneously, providing a comprehensive view of complex data sets. To fully grasp MVA, it is essential to explore three key aspects: its definition and history, the key components and terminology involved, and its common applications across various industries. Firstly, understanding the definition and history of MVA sets the foundation for appreciating its evolution and significance. This involves tracing back to its origins and key milestones that have shaped its current form. Secondly, familiarizing oneself with the key components and terminology is vital for effective application. This includes concepts such as principal component analysis, factor analysis, and cluster analysis, among others. Lastly, recognizing the common applications and industries where MVA is employed highlights its practical relevance and impact. From marketing and finance to healthcare and environmental science, MVA has become an indispensable tool for making informed decisions. By exploring these facets, one can gain a holistic understanding of MVA's role in modern data analysis. Let's begin by examining the definition and history of MVA, which will provide a solid groundwork for further exploration into its components and applications.
Definition and History of MVA
**Definition and History of MVA** Motor Vehicle Accidents (MVAs) are incidents involving vehicles that result in damage, injury, or death. The term encompasses a broad range of collisions, from minor fender benders to severe crashes involving multiple vehicles. Understanding the definition and history of MVAs is crucial for grasping the complexities and implications of these events. Historically, MVAs have been a significant concern since the advent of motorized transportation. The first recorded automobile accident occurred in 1896 when Henry Bliss, a pedestrian in New York City, was struck by an electric automobile. As the number of vehicles on the road increased, so did the frequency and severity of accidents. By the early 20th century, MVAs had become a major public health issue, prompting governments to implement safety regulations and infrastructure improvements. In the mid-20th century, the National Highway Traffic Safety Administration (NHTSA) was established in the United States to address the growing problem of traffic safety. NHTSA's efforts led to significant advancements in vehicle safety standards, including the mandatory installation of seat belts, airbags, and anti-lock braking systems (ABS). These measures have contributed to a decline in fatality rates per mile traveled over the decades. Despite these improvements, MVAs remain a leading cause of injury and death worldwide. According to the World Health Organization (WHO), approximately 1.35 million people die every year as a result of road traffic accidents. This staggering figure underscores the need for continued innovation in safety technologies and public awareness campaigns. The definition of an MVA extends beyond physical collisions; it also includes incidents where vehicles are involved but do not necessarily collide with other objects or vehicles. For example, rollover accidents or single-vehicle crashes where a driver loses control are also classified as MVAs. In recent years, advancements in technology have introduced new dimensions to MVA prevention and mitigation. The integration of advanced driver-assistance systems (ADAS) such as lane departure warning systems, automatic emergency braking, and blind-spot monitoring has shown promise in reducing accident rates. Additionally, the development of autonomous vehicles aims to further minimize human error—a primary cause of MVAs. Understanding the history and evolving nature of MVAs is essential for developing effective strategies to reduce their occurrence and impact. By recognizing the historical context and ongoing efforts to improve safety, individuals can better appreciate the importance of responsible driving practices and support initiatives aimed at enhancing road safety for all users. This foundational knowledge sets the stage for a deeper exploration into the basics of MVAs and their multifaceted implications on society.
Key Components and Terminology
Understanding the basics of Motor Vehicle Accidents (MVA) involves grasping several key components and terminology that are crucial for analyzing and addressing these incidents. At the heart of MVA analysis are **causal factors**, which include human error, vehicle defects, and environmental conditions. **Human error** encompasses driver behavior such as speeding, reckless driving, and failure to obey traffic laws. **Vehicle defects** refer to mechanical failures or design flaws that can contribute to accidents. **Environmental conditions** include weather, road design, and other external factors that may impact driving safety. Another critical component is **crash dynamics**, which involves the study of how vehicles interact during a collision. This includes understanding concepts like **kinematics** (the motion of vehicles) and **kinetics** (the forces involved in the collision). **Injury mechanisms** are also vital, as they explain how injuries occur during an MVA, helping in the assessment of injury severity and potential long-term impacts. **Terminology** plays a significant role in MVA discussions. Terms such as **point of impact**, **angle of incidence**, and **crash severity** are essential for describing the specifics of an accident. The **point of impact** refers to the location where the collision occurred, while the **angle of incidence** describes the direction from which the vehicles collided. **Crash severity** is often measured using metrics like the **delta-V** (change in velocity), which helps in estimating the force of the impact. Additionally, understanding **occupant protection** is crucial. This includes the role of **airbags**, **seatbelts**, and other safety features designed to mitigate injury during an accident. The effectiveness of these safety measures can significantly influence the outcome of an MVA. In legal and insurance contexts, terms like **liability** and **fault** become important. Determining who is at fault in an MVA can have significant implications for compensation and legal proceedings. **Evidence collection** and **accident reconstruction** are also key components, as they involve gathering data from the scene and using it to recreate the sequence of events leading up to and during the accident. Finally, **data analysis** is a critical aspect of MVA understanding. This involves examining statistical trends and patterns to identify common causes of accidents and areas for improvement in road safety. By combining these components—causal factors, crash dynamics, injury mechanisms, terminology, occupant protection, legal considerations, evidence collection, and data analysis—one can gain a comprehensive understanding of MVAs and work towards reducing their occurrence and impact. This holistic approach is essential for developing effective strategies in road safety and accident prevention.
Common Applications and Industries
Understanding the basics of Multivariate Analysis (MVA) is crucial for grasping its diverse applications across various industries. MVA, which involves analyzing multiple variables simultaneously, is a powerful tool for uncovering complex relationships and patterns in data. In **business and finance**, MVA is used to predict stock prices, assess credit risk, and optimize portfolio performance. For instance, financial analysts employ techniques like Principal Component Analysis (PCA) to reduce data dimensions and identify key factors influencing market trends. Similarly, in **marketing**, MVA helps in segmenting customer bases by analyzing demographic, behavioral, and transactional data, enabling targeted marketing strategies. In the **healthcare sector**, MVA is instrumental in medical research and clinical trials. It aids in identifying risk factors for diseases, understanding the efficacy of treatments, and predicting patient outcomes. Techniques such as cluster analysis and discriminant analysis are used to classify patients into different risk categories or to predict the likelihood of disease progression. **Environmental science** also benefits from MVA, where it is used to monitor and predict environmental changes. For example, analyzing multiple variables like temperature, humidity, and pollution levels helps in understanding climate patterns and predicting future environmental impacts. The **manufacturing industry** leverages MVA for quality control and process optimization. By analyzing multiple variables related to production processes, manufacturers can identify factors contributing to defects or inefficiencies, thereby improving product quality and reducing costs. In **social sciences**, MVA is used to study social phenomena such as crime rates, educational outcomes, and economic development. Researchers use techniques like factor analysis to understand underlying social constructs and their interrelations. Moreover, **data science and machine learning** heavily rely on MVA for developing predictive models. Techniques such as canonical correlation analysis help in understanding the relationship between two sets of variables, which is crucial for building robust machine learning models. In **agriculture**, MVA is applied to optimize crop yields by analyzing factors like soil composition, weather patterns, and fertilizer usage. Overall, the versatility of MVA makes it an indispensable tool across a wide range of industries. By providing insights into complex data sets, MVA enables better decision-making, improved efficiency, and enhanced understanding of multifaceted problems. As data continues to grow in volume and complexity, the importance of MVA will only increase, making it a fundamental skill for professionals in various fields.
Technical Aspects of MVA
When delving into the technical aspects of Motion Video Analysis (MVA), it is crucial to understand the multifaceted nature of this field. MVA involves a comprehensive approach that encompasses several key components. First, **Principles of Motion Analysis** form the foundational framework, guiding how motion is captured, interpreted, and analyzed. This includes understanding kinematics, dynamics, and biomechanics to accurately assess movement patterns. Second, **Tools and Software Used in MVA** play a vital role in enhancing the precision and efficiency of the analysis process. Advanced software and hardware tools such as high-speed cameras, 3D motion capture systems, and specialized algorithms are essential for data collection and processing. Finally, **Data Collection and Processing Methods** are critical for ensuring the accuracy and reliability of the analysis. These methods involve systematic procedures for capturing data, filtering noise, and applying statistical models to derive meaningful insights. By grasping these technical aspects, one can appreciate the complexity and sophistication involved in MVA. To begin, let's explore the **Principles of Motion Analysis**, which serve as the cornerstone for all subsequent steps in the MVA process.
Principles of Motion Analysis
**Principles of Motion Analysis** Motion analysis is a critical component of Motion Video Analysis (MVA), serving as the foundational framework for understanding and interpreting the dynamic movements captured in video footage. At its core, motion analysis involves the systematic study of human or object movement to extract meaningful data. This process is grounded in several key principles that ensure accuracy, reliability, and applicability across various contexts. 1. **Kinematics and Kinetics**: The analysis begins with kinematics, which focuses on the description of motion without considering the forces that cause it. This includes measuring parameters such as displacement, velocity, acceleration, and time. Kinetics, on the other hand, delves into the forces and torques that produce motion, providing a deeper understanding of the underlying mechanics. 2. **Segmentation and Tracking**: Effective motion analysis requires precise segmentation of the moving entities from the background and tracking their movement over time. Advanced algorithms and machine learning techniques are often employed to achieve this, ensuring that each segment or object is accurately identified and followed throughout the video sequence. 3. **Coordinate Systems**: A well-defined coordinate system is essential for quantifying motion. This could be a two-dimensional (2D) or three-dimensional (3D) system, depending on the complexity of the analysis. The choice of coordinate system influences how data is collected and interpreted, making it crucial for maintaining consistency and accuracy. 4. **Temporal and Spatial Resolution**: The quality of motion analysis is heavily dependent on the temporal and spatial resolution of the video footage. Higher resolutions allow for more precise measurements and better tracking of movements, while lower resolutions may introduce errors or ambiguities. 5. **Data Validation**: To ensure the reliability of the analysis, it is imperative to validate the data against known standards or benchmarks. This involves cross-referencing with other data sources, using multiple observers or automated systems to verify findings, and accounting for potential biases or errors in measurement. 6. **Contextual Understanding**: Motion analysis is not just about quantifying movement but also about understanding the context in which it occurs. This includes considering environmental factors, the purpose of the movement, and any external influences that might affect the motion. 7. **Ethical Considerations**: Especially in forensic or surveillance applications, ethical considerations must be taken into account. This includes ensuring privacy rights are respected, obtaining necessary permissions, and adhering to legal standards for data collection and use. By adhering to these principles, motion analysis within MVA can provide robust insights into various phenomena, from human gait patterns in forensic investigations to object tracking in surveillance systems. These insights are invaluable for making informed decisions, enhancing safety protocols, and optimizing performance in diverse fields such as sports, healthcare, and security. Ultimately, the meticulous application of these principles underscores the importance of rigorous methodology in extracting meaningful information from video data.
Tools and Software Used in MVA
In the realm of Motion Vector Analysis (MVA), the choice of tools and software is crucial for accurate and efficient data processing. MVA, a technique used to analyze the motion of objects within video sequences, relies heavily on sophisticated algorithms and robust computational frameworks. At the forefront of MVA are advanced software tools such as OpenCV, a widely-used open-source library that provides extensive functionalities for image and video processing. OpenCV offers a comprehensive suite of algorithms for feature detection, tracking, and optical flow estimation, which are fundamental components of MVA. Another key tool is MATLAB, particularly its Computer Vision Toolbox, which offers a rich set of functions tailored for motion analysis. MATLAB's user-friendly interface and extensive documentation make it an ideal platform for researchers and engineers to develop and implement complex MVA algorithms. Additionally, libraries like PyTorch and TensorFlow, popular deep learning frameworks, are increasingly being utilized in MVA to leverage the power of neural networks for more accurate motion detection and tracking. Specialized software such as Adobe After Effects and Blackmagic Design Fusion also play significant roles in certain aspects of MVA. These tools are particularly useful for post-processing tasks where precise control over motion vectors is required, often in applications like visual effects and video editing. Furthermore, commercial solutions like Avid Media Composer and Sony Vegas Pro offer integrated motion analysis features that can be invaluable in professional video production environments. For more specialized tasks, such as 3D motion analysis, software like Vicon Nexus and Qualysis Track Manager are indispensable. These systems provide high-precision tracking capabilities using marker-based or markerless tracking methods, which are essential in fields like biomechanics and sports analytics. The integration of these tools with other data acquisition systems ensures comprehensive data sets that can be analyzed using advanced statistical and machine learning techniques. In addition to these software tools, hardware components such as high-speed cameras and motion capture systems are critical for capturing high-quality data. High-speed cameras from manufacturers like Phantom and Basler provide the necessary frame rates to capture detailed motion patterns, while motion capture systems from companies like Xsens and OptiTrack offer precise 3D tracking capabilities. The synergy between these tools and software enables researchers and practitioners to conduct thorough and accurate MVA. By leveraging the strengths of each tool—whether it's the computational power of deep learning frameworks or the precision of specialized motion capture systems—users can extract meaningful insights from video data, driving advancements in various fields from sports science to surveillance technology. Ultimately, the careful selection and integration of these tools are pivotal in ensuring the technical aspects of MVA are executed with precision and reliability.
Data Collection and Processing Methods
Data collection and processing are pivotal components of the technical aspects of Multivariate Analysis (MVA), a statistical technique used to analyze multiple variables simultaneously. The quality and reliability of MVA outcomes heavily depend on the methodologies employed in data collection and processing. **Data Collection Methods:** Data collection involves gathering relevant information from various sources. In MVA, this can include surveys, experiments, observational studies, and existing databases. For instance, in market research, data might be collected through customer surveys to understand purchasing behaviors across different demographic groups. In scientific research, experiments could be designed to collect data on multiple variables affecting a particular phenomenon. Ensuring that the data collection process is systematic and unbiased is crucial; this often involves careful sampling techniques such as random sampling or stratified sampling to ensure representativeness. **Data Processing Methods:** Once data is collected, it must be processed to prepare it for analysis. This involves several steps including cleaning, transformation, and normalization. Data cleaning involves identifying and correcting errors or inconsistencies in the dataset, such as missing values or outliers. Transformation may be necessary to ensure that all variables are on the same scale or to meet the assumptions of certain statistical tests; for example, logarithmic transformation can stabilize variance. Normalization is often used to scale variables so that they contribute equally to the analysis, preventing variables with large ranges from dominating the results. **Technological Tools:** Modern data processing relies heavily on advanced technological tools. Software packages like R, Python libraries such as Pandas and NumPy, and specialized statistical software like SPSS or SAS are commonly used for data manipulation and analysis. These tools offer robust functionalities for handling large datasets efficiently and performing complex statistical operations with ease. Additionally, machine learning algorithms integrated into these tools can help in identifying patterns and relationships within the data that might not be apparent through traditional methods. **Quality Assurance:** Quality assurance is a critical aspect of both data collection and processing. This includes validating the accuracy of collected data against known benchmarks or using data validation rules to check for inconsistencies. During processing, it is essential to monitor for any changes that could introduce bias or errors into the dataset. Regular checks for missing values, outliers, and data distribution are necessary to ensure that the processed data accurately reflects the real-world scenario being analyzed. **Ethical Considerations:** Ethical considerations are also paramount in data collection and processing. Ensuring privacy and confidentiality of respondents' information is mandatory, especially when dealing with sensitive data such as personal health records or financial information. Compliance with regulations like GDPR (General Data Protection Regulation) in Europe or HIPAA (Health Insurance Portability and Accountability Act) in the United States is essential to avoid legal repercussions and maintain public trust. In summary, effective data collection and processing are foundational to conducting robust MVA. By employing rigorous methodologies in collecting high-quality data and using advanced technological tools for efficient processing, researchers can ensure that their analyses are reliable and meaningful. Attention to detail in every step—from ensuring representativeness during collection to maintaining ethical standards—enhances the validity of MVA results, thereby supporting informed decision-making across various fields.
Practical Uses and Benefits of MVA
The practical uses and benefits of Motion Vector Analysis (MVA) are multifaceted and impactful across various domains. This advanced technology has the potential to significantly enhance performance in sports and fitness, revolutionize medical and rehabilitation practices, and improve industrial and workplace safety. By analyzing the movement patterns of individuals, MVA can provide detailed insights that help athletes optimize their techniques, reduce injury risks, and achieve peak performance. In medical settings, MVA aids in the precise assessment and treatment of patients, facilitating more effective rehabilitation programs. Additionally, in industrial environments, MVA can identify potential safety hazards and help implement preventive measures to protect workers. These applications underscore the versatility and value of MVA in enhancing human performance and safety. As we delve into the specifics, let's first explore how MVA is transforming the world of sports and fitness by enhancing performance and reducing injury risks.
Enhancing Performance in Sports and Fitness
Enhancing performance in sports and fitness is a multifaceted endeavor that can significantly benefit from the integration of Multivariate Analysis (MVA). MVA, a statistical technique that examines multiple variables simultaneously, offers a robust framework for optimizing athletic performance and fitness outcomes. By leveraging MVA, athletes, coaches, and fitness professionals can gain deeper insights into the complex interplay of factors influencing performance. One of the primary practical uses of MVA in sports and fitness is the identification of key performance indicators (KPIs). Traditional methods often focus on isolated metrics such as speed, strength, or endurance. However, MVA allows for the analysis of multiple variables together, revealing how different factors interact and impact overall performance. For instance, in team sports like soccer or basketball, MVA can help coaches understand how variables such as player positioning, passing accuracy, and defensive strategies collectively influence game outcomes. This holistic approach enables more informed decision-making and targeted training programs. Another significant benefit of MVA is its ability to predict future performance based on historical data. By analyzing past performances and various contributing factors, MVA models can forecast potential outcomes under different scenarios. This predictive capability is particularly valuable for athletes preparing for major competitions or undergoing rehabilitation from injuries. For example, in track and field events, MVA can predict an athlete's likely performance times based on their training data, weather conditions, and other environmental factors. Moreover, MVA facilitates personalized training programs by accounting for individual differences among athletes. Unlike one-size-fits-all approaches, MVA allows coaches to tailor training regimens to each athlete's unique strengths, weaknesses, and physiological characteristics. In fitness settings, personal trainers can use MVA to create customized workout plans that optimize client progress while minimizing the risk of injury. This personalized approach not only enhances performance but also boosts motivation and adherence to training programs. In addition to these benefits, MVA aids in injury prevention and recovery. By analyzing data on training intensity, recovery time, and biomechanical factors, MVA can identify early warning signs of potential injuries. This proactive approach enables coaches and trainers to implement preventive measures such as modified training schedules or additional recovery protocols. During the recovery phase following an injury, MVA can help monitor progress and adjust rehabilitation plans accordingly, ensuring a safe and effective return to full performance. Finally, the use of MVA in sports and fitness extends beyond individual athletes to team dynamics and organizational efficiency. At the team level, MVA can analyze communication patterns, player chemistry, and strategic alignments to enhance team cohesion and effectiveness. For sports organizations, MVA can optimize resource allocation by evaluating the impact of various investments—such as coaching staff, facilities, and equipment—on overall team performance. In conclusion, integrating Multivariate Analysis into sports and fitness practices offers a powerful tool for enhancing performance across multiple dimensions. From identifying key performance indicators and predicting future outcomes to personalizing training programs and preventing injuries, MVA provides a comprehensive framework for achieving peak performance. As the field continues to evolve with advancements in data collection and analytics, the practical uses and benefits of MVA will only become more pronounced, making it an indispensable asset for anyone serious about optimizing their athletic or fitness journey.
Medical and Rehabilitation Applications
Medical and rehabilitation applications of Motor Vehicle Accidents (MVA) data are multifaceted and crucial, offering significant benefits in the healthcare and rehabilitation sectors. One of the primary uses is in the development of personalized treatment plans. By analyzing MVA data, healthcare providers can gain insights into the types and severity of injuries commonly sustained in such accidents, allowing them to tailor rehabilitation programs to meet the specific needs of patients. For instance, understanding the prevalence of spinal cord injuries or traumatic brain injuries from MVAs helps in allocating resources and expertise effectively, ensuring that patients receive the most appropriate care. Moreover, MVA data informs the design of rehabilitation facilities and equipment. Knowing the common injury patterns helps in equipping rehabilitation centers with necessary tools and technologies, such as advanced prosthetics, physical therapy equipment, and cognitive rehabilitation software. This ensures that patients have access to the best possible resources for their recovery. Additionally, MVA data can be used to train healthcare professionals in managing trauma cases more effectively. Simulation training programs based on real-world MVA scenarios enhance the preparedness of medical staff, leading to better patient outcomes. The integration of MVA data into public health initiatives is another significant application. By identifying high-risk areas and common causes of MVAs, public health campaigns can be targeted more effectively to reduce the incidence of accidents. This includes initiatives such as improving road safety infrastructure, promoting safe driving practices, and enhancing emergency response systems. Furthermore, MVA data can be used to evaluate the effectiveness of existing safety measures and policies, providing valuable feedback for policymakers to implement more stringent regulations or interventions. In terms of research, MVA data is invaluable for studying the long-term effects of injuries sustained in motor vehicle accidents. Longitudinal studies using this data can provide insights into the chronic health conditions that may arise from such injuries, helping researchers to develop new treatments and interventions. This research also aids in the development of evidence-based guidelines for managing complex injuries, which can improve patient care standards globally. From an economic perspective, analyzing MVA data helps in assessing the financial burden of motor vehicle accidents on healthcare systems. This information is critical for budgeting and resource allocation within healthcare institutions and insurance companies. It also supports the development of cost-effective treatment protocols that balance quality of care with economic feasibility. In conclusion, the medical and rehabilitation applications of MVA data are vast and impactful. By leveraging this data, healthcare providers can enhance patient care, improve rehabilitation outcomes, inform public health initiatives, support research efforts, and optimize resource allocation. These applications underscore the importance of collecting and analyzing MVA data to drive improvements in medical practice and public safety.
Industrial and Workplace Safety Improvements
Industrial and workplace safety improvements are paramount in today's fast-paced and technologically advanced work environments. The integration of advanced technologies, such as Machine Vision Analytics (MVA), has revolutionized the way safety protocols are implemented and monitored. MVA, with its ability to analyze visual data in real-time, offers a robust solution for enhancing workplace safety. Here’s how it contributes to practical improvements: 1. **Real-Time Monitoring**: MVA systems can continuously monitor workspaces, detecting potential hazards before they become incidents. For instance, in manufacturing plants, MVA can identify if workers are wearing required personal protective equipment (PPE) or if machinery is being operated safely. This real-time feedback allows for immediate intervention, significantly reducing the risk of accidents. 2. **Predictive Maintenance**: By analyzing visual data from machinery and equipment, MVA can predict when maintenance is needed, preventing unexpected breakdowns that could lead to accidents. This proactive approach ensures that equipment is always in optimal working condition, thereby reducing downtime and enhancing overall safety. 3. **Compliance Enforcement**: Ensuring compliance with safety regulations is a critical aspect of workplace safety. MVA can monitor adherence to safety protocols, such as proper lifting techniques, correct use of tools, and adherence to safety signage. This helps in maintaining a culture of safety within the organization. 4. **Training and Feedback**: MVA can provide valuable insights for training programs by analyzing worker behavior and identifying areas where additional training may be necessary. This data-driven approach to training enhances worker competence and reduces the likelihood of safety breaches. 5. **Incident Analysis**: In the event of an incident, MVA can provide detailed visual records that help in conducting thorough investigations. This aids in identifying root causes and implementing corrective measures to prevent future occurrences. 6. **Enhanced Worker Well-being**: Beyond physical safety, MVA can also monitor worker well-being by detecting signs of fatigue or stress through facial recognition and body language analysis. This allows for timely interventions to ensure workers' mental health and overall well-being. 7. **Cost Savings**: Implementing MVA not only improves safety but also reduces costs associated with accidents, downtime, and compliance violations. By preventing accidents and ensuring machinery operates efficiently, organizations can save significant resources. In summary, the integration of MVA into industrial settings offers a multifaceted approach to improving workplace safety. From real-time monitoring and predictive maintenance to compliance enforcement and incident analysis, MVA provides a comprehensive toolkit for creating a safer, more efficient work environment. Its practical uses and benefits make it an indispensable asset for any organization committed to enhancing workplace safety and overall operational excellence.