What Is The Range In Math
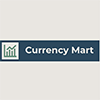
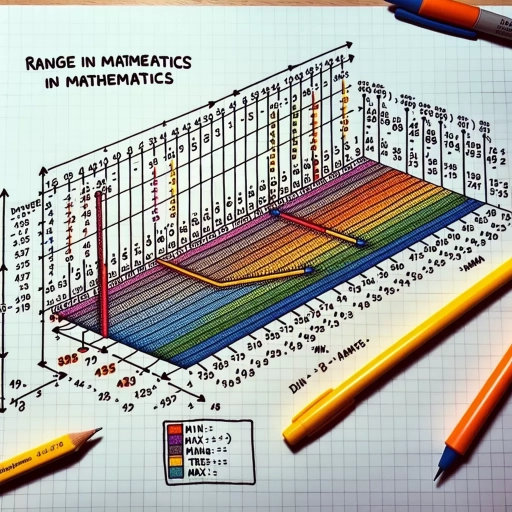
In the realm of mathematics, understanding statistical measures is crucial for analyzing and interpreting data. Among these measures, the range stands out as a fundamental concept that provides insights into the spread of data points. The range, simply defined as the difference between the highest and lowest values in a dataset, serves as a basic yet powerful tool for data analysis. This article delves into the multifaceted nature of range, starting with **Understanding the Concept of Range in Math**, where we explore its definition, significance, and how it differs from other statistical measures. We then move on to **Calculating Range in Various Data Sets**, where practical examples illustrate how to compute range across different types of data. Finally, we examine **Applications and Interpretations of Range**, highlighting its real-world uses and how it aids in making informed decisions. By grasping these aspects, readers will gain a comprehensive understanding of the range and its importance in mathematical analysis. Let's begin by **Understanding the Concept of Range in Math**.
Understanding the Concept of Range in Math
Understanding the concept of range in mathematics is a fundamental aspect that underpins various mathematical disciplines and statistical analyses. At its core, the range is a measure that quantifies the spread or dispersion of a dataset, providing valuable insights into the variability of data points. This article delves into the definition and basic principles of range, exploring how it is calculated and its significance in different mathematical contexts. We will also examine the various types of ranges that emerge in different mathematical frameworks, such as in algebra, geometry, and calculus, highlighting their unique characteristics and applications. Furthermore, we will discuss the importance of range in statistical analysis, where it plays a crucial role in understanding data distribution and making informed decisions. By grasping these concepts, readers will gain a comprehensive understanding of the range and its multifaceted role in mathematics. Transitioning seamlessly into the heart of this topic, we will explore these ideas in depth, starting with the definition and basic principles of range.
Definition and Basic Principles
**Definition and Basic Principles** Understanding the concept of range in mathematics begins with a clear definition and an appreciation of its fundamental principles. The range, in mathematical terms, refers to the set of all possible output values that a function can produce for the given input values. It is essentially the collection of all y-values that result from plugging in different x-values into a function. To grasp this concept fully, it's crucial to distinguish it from the domain, which is the set of all input values for which the function is defined. The basic principle underlying the range is rooted in the nature of functions themselves. A function is a relation between a set of inputs (domain) and a set of possible outputs (range). For instance, consider a simple linear function \( f(x) = 2x + 3 \). Here, if you input any real number \( x \), the output \( f(x) \) will also be a real number. However, not all functions have such straightforward ranges; some may be restricted due to the nature of their mathematical operations. For example, the square root function \( f(x) = \sqrt{x} \) has a range that consists only of non-negative real numbers because the square root of any negative number is not defined in the real number system. Similarly, trigonometric functions like sine and cosine have ranges limited to specific intervals, such as \([-1, 1]\). Another key principle is that the range can be affected by the type of function being considered. Polynomial functions, rational functions, exponential functions, and logarithmic functions each have unique characteristics that influence their ranges. For instance, polynomial functions of even degree with a positive leading coefficient have a range that includes all real numbers greater than or equal to the minimum value of the function. In summary, understanding the range involves recognizing it as the set of all possible output values generated by a function for its given input values. This concept is intertwined with the domain and is influenced by the specific type and characteristics of the function in question. By recognizing these principles, one can accurately determine and analyze the range for various mathematical functions, enhancing their overall comprehension of mathematical relationships and operations.
Types of Ranges in Different Mathematical Contexts
In various mathematical contexts, the concept of range manifests in different forms, each serving a unique purpose and offering distinct insights. In **algebra**, the range of a function is the set of all possible output values it can produce. For instance, if we have a function \( f(x) = x^2 \), its range is the set of all non-negative real numbers because \( x^2 \) is always non-negative. This understanding is crucial for analyzing the behavior and properties of functions. In **statistics**, the range is a measure of dispersion that quantifies the difference between the highest and lowest values in a dataset. It provides a simple yet effective way to gauge the spread of data points, helping statisticians understand how data varies. For example, if we have a dataset with values {3, 5, 7, 9}, the range would be \(9 - 3 = 6\), indicating that the data points span across six units. In **calculus**, particularly when dealing with **differential equations**, the range can refer to the interval over which a function or solution is defined. This is important because it helps in determining the domain of validity for solutions and ensures that any conclusions drawn are within the bounds of mathematical rigor. In **geometry**, especially in coordinate geometry, the range might be considered in terms of projections. For example, if we project points from a three-dimensional space onto a two-dimensional plane, the range could be understood as the set of all possible projected points on that plane. Moreover, in **set theory**, the range of a relation or function is essentially the codomain restricted to those elements that are actually mapped to by the relation or function. This distinction is vital for precise definitions and operations involving sets and relations. Lastly, in **probability theory**, when dealing with random variables, the range refers to all possible values that a random variable can take. This concept is fundamental for understanding probability distributions and calculating probabilities associated with different outcomes. Each of these contexts highlights how versatile and essential the concept of range is across various branches of mathematics. Understanding these different interpretations not only enriches one's mathematical toolkit but also fosters a deeper appreciation for the interconnectedness and diversity within mathematical disciplines. By recognizing how range operates in different mathematical frameworks, one can better navigate complex problems and derive meaningful insights from data and functions alike.
Importance in Statistical Analysis
Statistical analysis plays a pivotal role in understanding and interpreting data, and its importance cannot be overstated. When delving into mathematical concepts such as the range, statistical analysis provides the framework necessary to extract meaningful insights from datasets. The range, which is the difference between the highest and lowest values in a dataset, is just one of many statistical measures that help in describing data distribution. However, without robust statistical analysis, these measures remain isolated and lack context. Statistical analysis allows for the identification of trends, patterns, and correlations within data, enabling researchers and analysts to make informed decisions. It involves various techniques such as hypothesis testing, regression analysis, and confidence intervals, which collectively help in validating hypotheses and estimating population parameters from sample data. For instance, when analyzing the range of a dataset, statistical methods like outlier detection can highlight anomalies that might skew the range, providing a clearer picture of the data's central tendency. Moreover, statistical analysis enhances the reliability of conclusions drawn from data by accounting for variability and uncertainty. By applying statistical tests and models, analysts can determine whether observed differences or trends are statistically significant or merely due to chance. This is particularly crucial in fields like science, medicine, and social sciences where accurate interpretations of data can have profound implications. In addition to its analytical capabilities, statistical analysis facilitates communication of complex data insights in a clear and concise manner. Through visualizations such as histograms, box plots, and scatter plots, statistical tools help in presenting data in an accessible way that highlights key features like the range. This not only aids in understanding but also in sharing findings with stakeholders who may not have a deep statistical background. Ultimately, the integration of statistical analysis with concepts like the range in math ensures that data is not just described but also interpreted meaningfully. It bridges the gap between raw data and actionable knowledge, making it an indispensable tool in various disciplines. By leveraging statistical methods, researchers can uncover hidden patterns, validate theories, and drive decision-making processes with confidence—underscoring the paramount importance of statistical analysis in modern data-driven environments.
Calculating Range in Various Data Sets
Calculating the range in various data sets is a fundamental concept in statistics and data analysis, providing valuable insights into the spread of data. This article aims to guide readers through the process of determining range, ensuring clarity and accuracy. We will begin by outlining the **Step-by-Step Process for Finding Range**, breaking down the calculation into manageable steps to help readers grasp the methodology. Next, we will delve into **Examples with Different Types of Data**, illustrating how range is calculated across diverse datasets, from simple numerical sets to more complex data types. Additionally, we will highlight **Common Mistakes to Avoid**, addressing potential pitfalls that can lead to incorrect calculations. By understanding these key aspects, readers will gain a comprehensive grasp of range calculation, ultimately enhancing their ability to analyze and interpret data effectively. This foundational knowledge will transition seamlessly into **Understanding the Concept of Range in Math**, equipping readers with the tools necessary to apply this concept in various mathematical contexts.
Step-by-Step Process for Finding Range
To calculate the range in various data sets, you need to follow a systematic step-by-step process. This process ensures accuracy and clarity, making it easier to understand and interpret the data. Here’s how you can do it: 1. **Identify the Data Set**: Start by clearly identifying the set of numbers you are working with. This could be a list of exam scores, temperatures, or any other numerical data. 2. **Arrange the Data in Order**: Next, arrange all the numbers in the data set in ascending order. This means listing the smallest number first and the largest number last. For example, if your data set is {3, 6, 1, 8, 2}, arranging it in ascending order gives you {1, 2, 3, 6, 8}. 3. **Find the Maximum and Minimum Values**: Identify the largest and smallest numbers in your ordered list. In our example, the maximum value is 8 and the minimum value is 1. 4. **Calculate the Range**: The range is calculated by subtracting the minimum value from the maximum value. Using our example: Range = Maximum Value - Minimum Value = 8 - 1 = 7. 5. **Interpret the Result**: Finally, interpret what the range tells you about your data set. A larger range indicates greater variability or spread in the data, while a smaller range suggests that the data points are closer together. By following these steps consistently, you can accurately determine the range for any given set of numbers, providing valuable insights into how spread out or concentrated your data is. This method applies universally across different types of data sets, making it a fundamental tool in statistical analysis and data interpretation. Whether you're analyzing student test scores, stock prices, or weather patterns, calculating the range helps you understand the dispersion of your data and make informed decisions based on that understanding.
Examples with Different Types of Data
When calculating the range in various data sets, it is crucial to understand how different types of data can affect the outcome. Let's consider a few examples to illustrate this point. **Quantitative Data:** For numerical data, such as exam scores or temperatures, the range is straightforward to calculate. For instance, if we have a set of exam scores {85, 90, 78, 92, 88}, the range is found by subtracting the smallest value from the largest: \(92 - 78 = 14\). This simple calculation provides a clear measure of variability. **Categorical Data:** However, when dealing with categorical data (e.g., colors or types of fruit), the concept of range does not apply directly because these values are not numerical. Instead, we might use other measures like the number of distinct categories or frequency distributions to understand variability. **Ordinal Data:** Ordinal data, which has a natural order but no equal intervals between consecutive values (e.g., survey responses on a scale from "very dissatisfied" to "very satisfied"), presents another challenge. While we can rank these responses, calculating a meaningful range is not feasible in the same way as with quantitative data. Here, we might use alternative metrics like the number of categories or the proportion of responses in each category. **Mixed Data Sets:** In some cases, we encounter mixed data sets that combine different types of data. For example, a dataset might include both numerical values (ages) and categorical values (genders). In such scenarios, it's important to separate the data types and analyze them independently. For numerical components like age, we can calculate the range as usual; for categorical components like gender, we focus on other statistical measures. **Real-World Applications:** Understanding these differences is vital in real-world applications. In education, calculating the range of student scores helps teachers identify the spread of performance levels within a class. In climate science, analyzing temperature ranges over time can reveal patterns and trends that are crucial for understanding global warming. In summary, the type of data significantly influences how we approach calculating and interpreting the range. By recognizing these distinctions and applying appropriate methods for each data type, we can gain deeper insights into variability and make more informed decisions across various fields. This nuanced understanding ensures that our statistical analyses are accurate and meaningful, reflecting the true nature of our data sets.
Common Mistakes to Avoid
When calculating the range in various data sets, it is crucial to avoid common mistakes that can lead to inaccurate results. One of the most prevalent errors is incorrect identification of the maximum and minimum values. This often occurs when data points are not thoroughly examined or when outliers are mistakenly included or excluded. For instance, if a dataset contains an anomaly that significantly deviates from other values, failing to recognize it as an outlier can skew the range calculation. Another mistake is neglecting to check for duplicate values or missing data, which can affect the true range of the dataset. Additionally, misunderstanding the context of the data can lead to misinterpretation. For example, in a dataset involving temperatures, failing to account for units (e.g., Celsius vs. Fahrenheit) can result in incorrect range calculations. Similarly, not considering the scale of measurement—whether it's discrete or continuous—can also lead to errors. It's essential to ensure that all data points are on the same scale and that any transformations or adjustments are consistently applied. Furthermore, computational errors during the calculation process are common pitfalls. These can arise from simple arithmetic mistakes or from using incorrect formulas. For instance, if the range is calculated as the difference between the largest and smallest values without ensuring these values are correctly identified, the result will be flawed. It's also important to double-check calculations, especially when dealing with large datasets where manual computation may be impractical. Another critical aspect is ensuring that the dataset is free from errors such as typos or incorrect entries. A single incorrect entry can significantly alter the range, leading to misleading conclusions. Therefore, it's advisable to validate data before proceeding with range calculations. Lastly, it's vital to consider the implications of rounding numbers during calculations. Rounding too aggressively can mask significant variations within the dataset, while not rounding at all might make the range appear more precise than it actually is. Balancing precision with practicality is key. By being vigilant about these potential pitfalls—accurately identifying maximum and minimum values, accounting for context and scale, avoiding computational errors, ensuring data integrity, and managing rounding appropriately—you can ensure that your range calculations are reliable and meaningful. This attention to detail is essential for drawing accurate conclusions from your data analysis and making informed decisions based on those findings.
Applications and Interpretations of Range
In the realm of statistical analysis, the concept of range is a fundamental metric that provides valuable insights into data sets. The range, defined as the difference between the highest and lowest values in a dataset, is a straightforward yet powerful tool for understanding data variability. This article delves into the applications and interpretations of range, exploring its practical uses in real-world scenarios where understanding data spread is crucial. We will compare range with other statistical measures to highlight its unique strengths and limitations, and discuss alternatives that may offer more nuanced insights in certain contexts. By examining these aspects, readers will gain a comprehensive understanding of how range operates within broader statistical frameworks. Transitioning seamlessly from these discussions, we will ultimately deepen our understanding of the concept of range in mathematics, ensuring a solid foundation for further exploration and application.
Range in Real-World Scenarios
In real-world scenarios, the concept of range plays a crucial role in various fields, offering valuable insights and practical applications. For instance, in **meteorology**, understanding the range of temperatures and precipitation levels is essential for weather forecasting. Meteorologists analyze historical data to determine the typical range of weather conditions for a given region, enabling them to predict future weather patterns more accurately. This information is vital for planning agricultural activities, managing water resources, and issuing timely warnings for extreme weather events. In **medicine**, the range of a patient's vital signs such as blood pressure, heart rate, and body temperature is closely monitored to ensure they fall within healthy limits. Healthcare professionals use these ranges to diagnose conditions and monitor the effectiveness of treatments. For example, knowing the normal range for blood glucose levels helps in managing diabetes, while understanding the range of blood pressure readings aids in diagnosing hypertension. In **engineering**, the range of a sensor or instrument is critical for ensuring accurate measurements. Engineers must consider the range of possible inputs when designing systems to ensure that they can handle all expected values without malfunctioning. For instance, in automotive engineering, the range of a vehicle's speed sensor must be calibrated to cover all possible speeds from zero to the vehicle's top speed. In **finance**, understanding the range of stock prices or market indices over time helps investors make informed decisions. Analysts use historical price ranges to identify trends and predict future market movements. This analysis is crucial for risk management and portfolio optimization. Moreover, in **quality control**, manufacturers use the range of product specifications to ensure consistency and quality. By monitoring the range of dimensions or performance metrics, manufacturers can identify any deviations from the standard and take corrective actions to maintain product quality. In **sports analytics**, the range of player performance metrics such as speed, distance covered, or scoring rates provides coaches with valuable data to optimize team strategies and player training programs. This data helps in identifying areas for improvement and predicting player performance under different conditions. Lastly, in **environmental science**, the range of environmental parameters like pH levels in water bodies or concentration levels of pollutants is vital for assessing ecosystem health. Scientists use these ranges to set standards for environmental protection and monitor compliance with regulations. In summary, the concept of range is ubiquitous across various disciplines, providing a framework for understanding variability and making informed decisions based on data. Whether it's predicting weather patterns, diagnosing medical conditions, designing engineering systems, analyzing financial markets, ensuring product quality, optimizing sports performance, or protecting the environment, the range offers a powerful tool for interpretation and application.
Comparing Range with Other Statistical Measures
When evaluating data, the range is often compared to other statistical measures to gain a more comprehensive understanding of the dataset. Unlike the mean and median, which provide central tendency, the range focuses on the spread or dispersion of data. It is calculated as the difference between the highest and lowest values in a dataset, offering a straightforward measure of variability. However, its simplicity can also be a limitation; it is highly sensitive to outliers, which can skew the range significantly. In contrast, other measures like the interquartile range (IQR) and standard deviation provide more robust insights into data dispersion. The IQR, which is the difference between the third quartile (Q3) and the first quartile (Q1), is less affected by outliers and thus offers a more stable measure of variability. The standard deviation, on the other hand, quantifies the amount of variation or dispersion of a set of data values. While it is more complex to calculate than the range, it provides a more nuanced view of how data points deviate from the mean. Another key comparison is with variance, which is the square of the standard deviation. Variance gives an idea of how spread out the data is from its mean value but does not provide an immediate visual or intuitive sense of dispersion like the range does. For practical applications, understanding these different measures together is crucial. For instance, in quality control, knowing both the range and standard deviation can help identify whether variations in production are due to random fluctuations or systematic issues. Moreover, when interpreting data in real-world scenarios such as finance or social sciences, combining these statistical measures can lead to more accurate conclusions. For example, in stock market analysis, a high range might indicate volatility but does not tell us about the distribution of prices within that range. Here, combining it with standard deviation or IQR can provide a clearer picture of market stability. In summary, while the range offers a quick snapshot of data spread, it should be considered alongside other statistical measures for a complete analysis. Each measure has its strengths and weaknesses: the range for simplicity and immediate insight into extreme values; IQR for robustness against outliers; standard deviation for detailed variability; and variance for understanding deviations from the mean. By leveraging these tools together, analysts can derive richer interpretations and make more informed decisions across various fields.
Limitations and Alternatives to Range
When delving into the concept of range in mathematics, it is crucial to acknowledge its limitations and explore alternative measures that can provide a more comprehensive understanding of data sets. The range, defined as the difference between the highest and lowest values in a dataset, offers a simple yet limited insight into data dispersion. One significant limitation of range is its sensitivity to outliers; a single extreme value can drastically alter the range, making it an unreliable measure for datasets with anomalous entries. Additionally, range does not account for the distribution of data points within the dataset, providing no information about how data is spread out between the minimum and maximum values. To overcome these limitations, several alternative measures of dispersion are employed. One such alternative is the **interquartile range (IQR)**, which calculates the difference between the third quartile (Q3) and the first quartile (Q1). Unlike the range, IQR is more robust against outliers because it focuses on the middle 50% of the data. Another alternative is **variance**, which measures the average of the squared differences from the mean. Variance provides a more detailed view of data spread but can be influenced by extreme values. **Standard deviation**, the square root of variance, is another widely used measure that offers a better understanding of how data points deviate from the mean. For datasets that require a more nuanced analysis, **percentiles** can be used to understand the distribution of data at different levels. For instance, the 10th and 90th percentiles can give insights into the spread of data without being heavily influenced by outliers. Furthermore, graphical representations like **box plots** and **histograms** can visually depict data distribution, making it easier to identify patterns and anomalies that might not be apparent through numerical measures alone. In practical applications, these alternatives to range are essential for making informed decisions. For example, in finance, understanding the standard deviation of stock prices helps investors gauge risk more accurately than relying solely on the range. In engineering, using IQR to analyze sensor readings can help identify potential issues without being skewed by occasional erroneous readings. By recognizing the limitations of range and leveraging these alternative measures, analysts can gain a deeper understanding of their data and make more accurate interpretations in various fields. This holistic approach ensures that data analysis is comprehensive and reliable, leading to better decision-making processes across different disciplines.