What Is A Control Variable
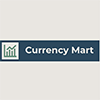
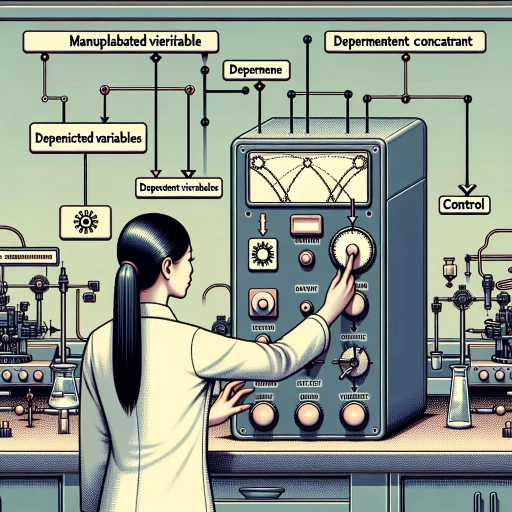
In the realm of scientific research, precision and accuracy are paramount, and one crucial element that ensures the integrity of experimental results is the control variable. A control variable is a factor that is held constant throughout an experiment to prevent it from influencing the outcome, thereby allowing researchers to isolate and measure the effect of the independent variable on the dependent variable. Understanding the concept of a control variable is fundamental to conducting rigorous and reliable research. This article delves into the essence of control variables, starting with a comprehensive explanation of what they are and their role in experimental design. We will then explore the process of identifying and selecting appropriate control variables, highlighting the importance of careful consideration in this step. Finally, we will discuss how control variables are implemented in research studies, illustrating their practical application. By grasping these key aspects, researchers can ensure that their studies yield meaningful and trustworthy data. Let's begin by understanding the concept of a control variable.
Understanding the Concept of a Control Variable
In the realm of scientific research and experimental design, understanding the concept of a control variable is crucial for ensuring the validity and reliability of results. A control variable is a factor that is kept constant to prevent it from influencing the outcome of an experiment, thereby allowing researchers to isolate the effect of the independent variable on the dependent variable. This article delves into the essence of control variables, starting with their **Definition and Purpose**, which highlights why these variables are indispensable in maintaining experimental integrity. We will also explore **Types of Control Variables**, examining how different kinds of control variables are used in various research contexts. Finally, we will discuss the **Importance in Experimental Design**, illustrating how controlling variables enhances the accuracy and generalizability of experimental findings. By grasping these key aspects, readers will gain a comprehensive understanding of the concept of a control variable and its pivotal role in scientific inquiry. Understanding the Concept of a Control Variable is essential for any researcher aiming to conduct rigorous and meaningful experiments.
Definition and Purpose
In the realm of scientific research and experimentation, understanding the concept of a control variable is crucial for ensuring the validity and reliability of results. A control variable, also known as a constant or independent variable, is a factor that is intentionally kept constant or unchanged throughout an experiment to prevent it from influencing the outcome. The definition and purpose of control variables are intertwined and fundamental to the scientific method. **Definition:** A control variable is any factor that could potentially affect the outcome of an experiment but is deliberately controlled or held constant to isolate the effect of the independent variable on the dependent variable. These variables can include environmental conditions such as temperature, humidity, or lighting, as well as other factors like the type of equipment used or the time of day when measurements are taken. **Purpose:** The primary purpose of identifying and controlling these variables is to ensure that any observed changes in the dependent variable are due solely to the manipulation of the independent variable rather than extraneous factors. By keeping control variables constant, researchers can establish cause-and-effect relationships with greater confidence. For instance, in a study examining how different fertilizers affect plant growth, factors such as soil type, water amount, and sunlight exposure would be controlled to ensure that any differences in plant growth are attributed solely to the fertilizer being tested. Moreover, controlling variables helps in reducing experimental error and increasing the precision of results. This is particularly important in fields like medicine, where small variations can have significant implications for patient outcomes. For example, in clinical trials evaluating new medications, controlling variables such as patient age, gender, and pre-existing conditions ensures that any observed effects are due to the medication itself rather than other factors. In summary, understanding and effectively managing control variables is essential for conducting rigorous scientific research. By defining what constitutes a control variable and meticulously controlling these factors during experiments, researchers can enhance the accuracy and reliability of their findings. This meticulous approach not only strengthens the conclusions drawn from experimental data but also contributes to advancing knowledge across various disciplines by ensuring that results are reproducible and trustworthy.
Types of Control Variables
In the realm of scientific research and experimentation, control variables play a crucial role in ensuring the integrity and reliability of the findings. These variables are factors that could potentially influence the outcome of an experiment but are controlled or held constant to isolate the effect of the independent variable on the dependent variable. There are several types of control variables, each serving a distinct purpose in maintaining experimental rigor. **Constant Control Variables** are those that remain unchanged throughout the experiment. For instance, in a study examining the effect of light on plant growth, the temperature and humidity levels might be kept constant to prevent any confounding effects. **Matched Control Variables** involve matching participants or samples in terms of relevant characteristics to ensure that any differences observed are due to the independent variable rather than pre-existing differences. This is often used in studies involving human subjects where factors like age, gender, or health status could influence outcomes. **Randomized Control Variables** involve randomly assigning participants to different groups to minimize any systematic differences between groups. This method helps to distribute potential confounding variables evenly across all groups, thereby reducing bias. **Statistical Control Variables** are used in data analysis to adjust for the effects of variables that could not be controlled during the experiment. Techniques such as regression analysis can be employed to statistically control for these variables, allowing researchers to isolate the effect of the independent variable. **Extraneous Control Variables** are factors that are not directly related to the research question but could still affect the outcome. These might include environmental factors like noise levels or time of day, which can be controlled through careful experimental design. **Instrumental Control Variables** pertain to the tools and equipment used in the experiment. Ensuring that all instruments are calibrated and functioning consistently helps to eliminate any variability introduced by the measurement tools themselves. Understanding these types of control variables is essential for researchers to design robust experiments that yield reliable and generalizable results. By meticulously controlling for various factors, researchers can enhance the validity of their studies, reduce errors, and draw more accurate conclusions about the relationships between variables. This meticulous approach not only strengthens the scientific method but also contributes to the advancement of knowledge across diverse fields by ensuring that findings are based on sound methodology rather than chance or uncontrolled influences.
Importance in Experimental Design
The importance of experimental design cannot be overstated, particularly when it comes to understanding and effectively utilizing control variables. Experimental design is the backbone of scientific research, providing a structured framework that ensures experiments are conducted in a way that minimizes bias and maximizes the reliability of results. At its core, experimental design involves carefully planning and executing experiments to isolate the effect of an independent variable on a dependent variable while controlling for other variables that could influence the outcome. A well-designed experiment allows researchers to draw causal inferences with confidence, which is crucial for advancing knowledge in any field. By systematically varying the independent variable and holding all other variables constant (including control variables), researchers can determine whether changes in the dependent variable are due to the manipulation of the independent variable or to extraneous factors. This precision is essential for validating hypotheses and theories. Control variables play a pivotal role in this process. These are variables that could potentially affect the outcome of an experiment but are not of primary interest. For instance, in a study examining the effect of fertilizer on plant growth, factors like soil type, temperature, and light exposure would be considered control variables. By controlling these variables—either by holding them constant or by randomizing their distribution across experimental groups—researchers can ensure that any observed effects are due solely to the independent variable (fertilizer) rather than to these extraneous factors. The importance of controlling these variables becomes evident when considering the potential for confounding variables to skew results. If left uncontrolled, these variables could lead to misleading conclusions about the relationship between the independent and dependent variables. For example, if soil type varies significantly between experimental groups, it might appear that fertilizer has a different effect than it actually does, simply because different soils respond differently to fertilization. Moreover, a robust experimental design enhances the generalizability and replicability of findings. When experiments are conducted under controlled conditions, other researchers can more easily replicate the study to verify or challenge the results. This process of replication is fundamental to scientific progress, as it allows for the validation and refinement of theories over time. In summary, the importance of experimental design lies in its ability to provide a rigorous framework for testing hypotheses while minimizing the impact of extraneous variables. By carefully controlling variables and ensuring that experiments are conducted systematically, researchers can draw reliable conclusions about causal relationships. This precision is what makes experimental design indispensable in scientific inquiry, enabling us to build a more accurate and comprehensive understanding of the world around us.
Identifying and Selecting Control Variables
In the realm of scientific research and data analysis, the meticulous selection of control variables is a cornerstone of ensuring the validity and reliability of study outcomes. Control variables are factors that could potentially influence the relationship between the independent and dependent variables, and their identification and selection are crucial for drawing accurate conclusions. This article delves into the essential aspects of identifying and selecting control variables, providing a comprehensive guide for researchers and analysts. We will explore the **Criteria for Selection**, outlining the key principles that guide the choice of control variables to ensure they are relevant and effective. Additionally, we will examine **Common Examples in Different Fields**, highlighting how control variables are applied across various disciplines such as medicine, psychology, and economics. Finally, we will discuss **Potential Pitfalls and Considerations**, warning against common mistakes and offering practical advice to avoid them. By understanding these critical components, readers will gain a deeper insight into the concept of control variables, ultimately enhancing their ability to design robust and meaningful studies. Transitioning seamlessly into **Understanding the Concept of a Control Variable**, this article aims to equip readers with the knowledge necessary to integrate control variables effectively into their research methodologies.
Criteria for Selection
When identifying and selecting control variables, it is crucial to adhere to specific criteria to ensure the integrity and reliability of your research. **Relevance** is the first key criterion; the control variable must be directly related to the research question or hypothesis. For instance, if you are studying the impact of exercise on blood pressure, age and gender could be relevant control variables because they can influence both exercise habits and blood pressure levels. **Measurability** is another critical factor; the control variable should be quantifiable or categorizable in a way that allows for precise measurement and analysis. This ensures that data collected is consistent and can be accurately analyzed. **Independence** from the independent variable is also essential. The control variable should not be influenced by the independent variable, as this could introduce confounding effects that skew the results. For example, in a study examining the effect of diet on weight loss, income level might be an independent control variable because it does not directly result from dietary changes but could affect outcomes. **Stability** over time is another important consideration. The control variable should remain relatively constant throughout the study period to avoid introducing unnecessary variability. In longitudinal studies, demographic factors like ethnicity or genetic predispositions are often stable and thus suitable as control variables. Additionally, **availability of data** is a practical criterion. The control variable should be something for which data can be readily obtained or measured without undue burden on participants or researchers. This ensures that the study remains feasible and efficient. Finally, **theoretical justification** is vital; there should be a sound theoretical basis for why a particular variable is chosen as a control. This involves understanding the underlying mechanisms and relationships between variables as supported by existing literature. By selecting control variables based on these criteria, researchers can enhance the validity of their findings, reduce confounding effects, and provide more accurate insights into the relationship between independent and dependent variables. This meticulous approach to selecting control variables is fundamental in maintaining the scientific rigor of any research study.
Common Examples in Different Fields
In various fields, control variables play a crucial role in ensuring the integrity and reliability of research findings. For instance, in **medicine**, when conducting clinical trials to test the efficacy of a new drug, researchers often control for variables such as age, gender, and pre-existing health conditions to isolate the effect of the drug. This helps in determining whether any observed outcomes are due to the drug itself rather than other factors. Similarly, in **psychology**, studies on cognitive development might control for socioeconomic status and family dynamics to understand how these variables influence cognitive outcomes independently of the primary variable being studied. In **economics**, researchers frequently control for macroeconomic indicators like inflation rates and unemployment levels when analyzing the impact of policy changes on economic growth. This ensures that any observed effects are not confounded by broader economic trends. **Environmental science** also relies heavily on control variables; for example, when studying the effects of climate change on ecosystems, scientists might control for variables such as soil type, altitude, and existing vegetation to isolate the impact of temperature changes. In **education**, studies on student performance often control for variables like prior academic achievement, parental education level, and access to resources to understand how specific interventions (such as new teaching methods) affect student outcomes. **Engineering** research may involve controlling for material properties and environmental conditions when testing the performance of new materials or technologies. Moreover, in **social sciences**, researchers studying social behaviors might control for demographic factors such as race, ethnicity, and income level to understand how these variables interact with the primary variable of interest. For example, a study on the impact of social media on mental health would need to control for factors like age group and usage patterns to draw accurate conclusions. By carefully identifying and selecting control variables across these diverse fields, researchers can enhance the validity of their findings and provide more precise insights into the relationships they are investigating. This meticulous approach helps in minimizing confounding effects and ensures that the results are reliable and generalizable to broader populations or contexts. Ultimately, the strategic use of control variables is essential for advancing knowledge and making informed decisions in various disciplines.
Potential Pitfalls and Considerations
When identifying and selecting control variables, researchers must navigate a series of potential pitfalls and considerations to ensure the integrity and validity of their studies. One of the primary concerns is the risk of confounding variables, which can masquerade as control variables but actually influence the outcome in unintended ways. For instance, if a study aims to examine the effect of diet on blood pressure but fails to control for physical activity levels, the results may be skewed because physical activity is a significant confounder. Therefore, thorough literature reviews and preliminary analyses are crucial to identify all relevant control variables. Another critical consideration is the issue of multicollinearity, where two or more control variables are highly correlated with each other. This can lead to unstable estimates and inflated standard errors, making it difficult to interpret the results accurately. Researchers should use statistical techniques such as variance inflation factor (VIF) to detect multicollinearity and adjust their models accordingly. Additionally, the selection of control variables must be grounded in theoretical reasoning and empirical evidence. Simply including a large number of variables without a clear rationale can lead to overfitting, where the model becomes too complex and fails to generalize well to other populations. This underscores the importance of a priori hypotheses and careful model specification. Moreover, ethical considerations play a significant role in selecting control variables. For example, in studies involving human subjects, it is essential to control for variables that could impact participant well-being or privacy. Ignoring these ethical dimensions can compromise the study's validity and lead to ethical violations. Data quality is another pivotal aspect; poor data quality in control variables can propagate errors throughout the analysis. Ensuring that data collection methods are robust and that data cleaning processes are rigorous can help mitigate this issue. Finally, the temporal relationship between the control variables and the outcome variable must be carefully considered. Controlling for variables that occur after the outcome variable can introduce reverse causality biases, leading to misleading conclusions. By carefully timing the measurement of control variables relative to the outcome variable, researchers can strengthen their causal inferences. In summary, identifying and selecting control variables is a nuanced process that requires meticulous attention to potential pitfalls such as confounding variables, multicollinearity, overfitting, ethical considerations, data quality issues, and temporal relationships. By addressing these challenges thoughtfully, researchers can enhance the reliability and generalizability of their findings.
Implementing Control Variables in Research Studies
Implementing control variables in research studies is a crucial step in ensuring the validity and reliability of experimental results. Control variables are factors that could potentially influence the outcome of an experiment, and managing them effectively is essential to isolate the effect of the independent variable. This article delves into the methodologies and best practices for controlling variables, providing a comprehensive guide for researchers. We will explore **Methods for Controlling Variables**, discussing various strategies to minimize the impact of extraneous factors. Additionally, we will examine **Tools and Techniques for Measurement**, highlighting the instruments and methods used to accurately quantify and control variables. Finally, we will outline **Best Practices for Effective Control**, offering practical advice on how to implement these strategies in real-world research settings. By understanding these key aspects, researchers can enhance the rigor of their studies, leading to more accurate and meaningful conclusions. This foundational knowledge is pivotal for **Understanding the Concept of a Control Variable**, a fundamental principle in scientific research.
Methods for Controlling Variables
When implementing control variables in research studies, several methods are employed to ensure the integrity and reliability of the findings. **Matching** is a common technique where participants are paired based on relevant characteristics to minimize differences between groups. For instance, in a study examining the impact of a new educational program, researchers might match students by age, socioeconomic status, and prior academic performance to create comparable groups. **Stratification** involves dividing the sample into subgroups based on key variables and then analyzing each subgroup separately. This method helps to isolate the effect of the independent variable by controlling for confounding variables within each stratum. **Regression analysis** is another powerful tool for controlling variables. By including control variables in a regression model, researchers can statistically adjust for their influence, allowing for a clearer understanding of the relationship between the independent and dependent variables. For example, in a study investigating the relationship between exercise and weight loss, researchers might include age, gender, and initial weight as control variables to account for their potential impact on the outcome. **Randomization** is a robust method for controlling variables, particularly in experimental designs. By randomly assigning participants to different groups, researchers can distribute both known and unknown confounding variables evenly across groups, thereby reducing bias. However, randomization alone may not always be sufficient; hence, **blocking** is used to ensure that certain variables are evenly distributed within each group. This is particularly useful in studies where randomization alone might not adequately control for significant confounders. **Instrumental variables** are used in situations where direct control over confounding variables is not feasible. An instrumental variable is a third variable that affects the independent variable but not the dependent variable, except through its effect on the independent variable. This method helps to isolate the causal effect of the independent variable by leveraging the instrumental variable to create a pseudo-experiment. Finally, **propensity score matching** is a sophisticated technique that combines elements of matching and stratification. It involves calculating the probability (propensity score) of a participant being in a particular group based on observed characteristics and then matching participants with similar propensity scores. This method is particularly useful in observational studies where randomization is not possible. Each of these methods has its own strengths and limitations, and the choice of method depends on the research design, data availability, and the nature of the variables involved. By carefully selecting and implementing these control strategies, researchers can enhance the validity of their findings and provide more accurate insights into the relationships under investigation.
Tools and Techniques for Measurement
When implementing control variables in research studies, the precision and reliability of measurement tools and techniques are paramount. Control variables are factors that could influence the outcome of a study but are not of primary interest to the researcher. To effectively control for these variables, researchers must employ robust measurement methods that ensure accuracy and consistency. One key tool is the use of standardized instruments such as surveys, questionnaires, and psychometric tests. These tools are designed to measure specific constructs with high reliability and validity, allowing researchers to capture nuanced variations in control variables. Another critical technique is the use of observational methods, including both structured and unstructured observations. Structured observations involve predefined criteria and protocols to ensure consistency across different observers, while unstructured observations allow for more flexibility in capturing unexpected phenomena. Additionally, physiological measurements like heart rate, blood pressure, and brain activity can provide objective data that complement self-reported measures. Techniques such as content analysis and coding schemes are also essential for quantifying qualitative data. Content analysis involves systematically analyzing text, images, or videos to identify patterns and themes related to control variables. Coding schemes help in categorizing data into meaningful categories, which can then be quantified for statistical analysis. Furthermore, statistical techniques play a crucial role in controlling for variables. Methods like regression analysis and propensity score matching help adjust for the effects of control variables on the outcome variable. These statistical tools enable researchers to isolate the effect of the independent variable while accounting for potential confounding factors. In experimental designs, randomization is a powerful technique for distributing participants across different conditions in a way that minimizes differences between groups on control variables. This ensures that any observed differences in outcomes are more likely due to the independent variable rather than pre-existing differences. Lastly, technology has significantly enhanced measurement capabilities with tools like mobile apps, wearable devices, and online platforms. These tools can collect real-time data on various aspects of human behavior and physiological responses, providing rich datasets that can be analyzed to control for a wide range of variables. In summary, the effective implementation of control variables in research studies hinges on the meticulous selection and application of measurement tools and techniques. By combining standardized instruments, observational methods, physiological measurements, content analysis, statistical adjustments, randomization, and technological innovations, researchers can ensure that their findings are robust and free from confounding influences. This rigorous approach not only enhances the validity of research but also contributes to the advancement of knowledge in various fields by providing clear insights into the relationships between variables.
Best Practices for Effective Control
When implementing control variables in research studies, adhering to best practices is crucial for ensuring the integrity and reliability of the findings. Effective control involves meticulously identifying, measuring, and managing variables that could influence the outcome of the study. First, researchers must conduct a thorough literature review to identify potential confounding variables relevant to their research question. This step helps in developing a comprehensive list of control variables that need to be accounted for. Next, it is essential to use robust statistical methods to control for these variables. Techniques such as matching, stratification, and regression analysis can be employed to adjust for differences between groups. For instance, propensity score matching can help balance the distribution of covariates between treatment and control groups, thereby reducing bias. Additionally, using multiple regression models allows researchers to isolate the effect of the independent variable while controlling for other factors. Another critical best practice is ensuring data quality through rigorous data collection and validation processes. This includes using reliable and validated measurement tools, training data collectors, and implementing data cleaning protocols to minimize errors. Furthermore, transparency in reporting is vital; researchers should clearly document all control variables considered, the methods used to control them, and any limitations encountered during the process. Moreover, ethical considerations must be taken into account when controlling variables. Informed consent from participants is necessary, especially if the study involves sensitive or personal information. Ensuring participant confidentiality and adhering to ethical guidelines set by regulatory bodies such as Institutional Review Boards (IRBs) is paramount. Finally, ongoing evaluation and adjustment are key components of effective control. Researchers should continuously monitor their study design and data collection process to identify any emerging issues or biases. This may involve conducting pilot studies or interim analyses to assess whether the control strategies are effective and make necessary adjustments before proceeding with the full study. By following these best practices—thoroughly identifying control variables, using robust statistical methods, ensuring data quality, maintaining ethical standards, and continuously evaluating the study design—researchers can enhance the validity and generalizability of their findings. This meticulous approach not only strengthens the research but also contributes to the advancement of knowledge in various fields by providing reliable and actionable insights.