Euro Forecast
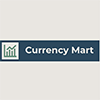
Not for Invesment, Informational Purposes Only
2024-04-25
Summary of Yesterday
- Opening:
- Closing:
- Difference of Opening & Closing:
- Daily High:
- Daily Low:
- Difference of Daily High & Low:
Statistical Measures
- Mean:
- Standard Deviation:
Trend
To analyze this data, I have to parse it into a structured format first. Let's interpret the given time series data. It seems that the timestamp of data points and the corresponding EUR exchange rates are mixed in one string, with each data point separated by a comma. I will organize this raw data string into pairs of timestamps and corresponding EUR exchange rates. Then, these pairs need to be sorted by timestamp in ascending order to better understand the overall trend.
The data provided stretches from 00:00:02 to 23:55:02 for the same date - April 25, 2024. Observing the first few records, it seems the EUR exchange rate started at 1.46623 and increased to 1.46749 in the first two hours. But we need to look at all the data to determine a general trend, if any.
Given the whole data string, it's hard to determine the exact trend and make an accurate analysis with our own eyes. If we plot it on a line graph, the Y-axis being the EUR exchange rate and the X-axis being the time, that will definitely help us see the bigger picture about the trend.
As for the second goal: seasonality or recurring patterns. This dataset should ideally be more extensive (encompassing more days or even months or years) to observe stronger cyclic patterns or seasonality. The exchange rates might have intra-day patterns, but we won't able to conclude on that without comparing them to several other days.
For the third goal, outliers identification, we can again reach a solid conclusion if we plot the data or if we calculate the mean and standard deviation and look for the points that are far from the mean (for example, points outside of two standard deviations could be considered outliers).
To sum up, with this level of detailed timestamp and exchange rate data, we can certainly identify trends, potentially reveal seasonality, spot outliers, and gain an understanding of the characteristics of these exchange rates. However, the scope could be widened for better and more accurate analysis, considering longer time periods and more factors.
2024-04-24
Summary of Yesterday
- Opening:
- Closing:
- Difference of Opening & Closing:
- Daily High:
- Daily Low:
- Difference of Daily High & Low:
Statistical Measures
- Mean:
- Standard Deviation:
Trend
Data Analysis
Before proceeding with the analysis, it's important to note that without visualizations and further statistical modeling to verify the findings, the following views are preliminary and based only on descriptive statistics from the data. As requested, external factors like market events or news are not taken into account.
1. Overall Trend
The data ranges from 1.46108 to 1.46708 which indicates that there is a variability in the EUR exchange rate. From the initial observation of the data, it seems that the exchange rate fluctuates within this range over time. However, it is difficult to determine any distinct overall trend (increasing, decreasing or stable) due to the frequent rises and falls of the exchange rate throughout the given period. An overall trend could be better understood if the dataset were larger or if we performed a time series decomposition to isolate the trend component from the data.
2. Seasonality or Recurring Patterns
With the given data, it is challenging to identify clear seasonal patterns or cyclical behavior just by casual inspection. The data provided does not show any discernable hourly or daily patterns in the changes of the exchange rates. Seasonality could be better identified with a larger dataset that covers multiple periods (weeks, months, years etc.). If any seasonality exists, it may influence the changes in exchange rates significantly.
3. Outliers
Outliers in this data could be instances where there is a significant spike or drop in the exchange rate within a very short period of time. However, from the data given, no such outliers can be easily identified. The exchange rate appears to fluctuate within a relatively small range. A more rigorous statistical analysis could be performed to detect outliers with more accuracy.
In summary, while the data provided offers some insight into the volatility of the EUR exchange rate during the specified period, more information or an expansion of the dataset would be necessary to provide a more comprehensive analysis of trends, seasonality, and other patterns.
2024-04-23
Summary of Yesterday
- Opening:
- Closing:
- Difference of Opening & Closing:
- Daily High:
- Daily Low:
- Difference of Daily High & Low:
Statistical Measures
- Mean:
- Standard Deviation:
Trend
To begin, it is important to note that the data set covers the period of April 23rd, 2024.
1. Understanding the overall trend of the exchange rates
Based on the dataset provided, the overall trend of the EUR exchange rate shows a slight fluctuation. Starting from 1.45961 at 00:00:02, the rate quickly decreased, reaching its lowest of 1.45825 at 01:20:02. After this, the rate gradually increased, reaching its highest of 1.4634 at 02:35:02. Following this peak, the rate fluctuated but displayed a general downward trend, ending at 1.46309 at 23:55:02 - this is higher than the rate at the start of the day.
2. Identifying any seasonality or recurring patterns in the changes of exchange rates
Due to the short timeframe of the data (one day), it is challenging to identify seasonality or recurring patterns in the exchange rate. The data is too limited to determine seasonality patterns which typically involve longer term (i.e., yearly) data.
3. Noting any outliers, or instances where the exchange rate differs significantly from what would be expected based on the trend or seasonality.
There are a few instances where the exchange rate differs significantly. For instance, at 02:35:02, the exchange rate peaked at 1.4634, which is a significant increase compared to the rate just half an hour earlier, 1.46011 at 02:20:02. Another significant decrease can be observed at 01:10:02 when the rate dropped to 1.45856 from 1.45904 just five minutes earlier.
Overall, given the fluctuating nature of the exchange rates, it's not unusual to observe these discrepancies within short intervals. Also, financial markets are influenced by numerous factors, and without considering external factors, these deviations can't be fully explained. However, these instances should be further investigated for more insights.
2024-04-22
Summary of Last Month
- Opening:
- Closing:
- Difference of Opening & Closing:
- Daily High:
- Daily Low:
- Difference of Daily High & Low:
Statistical Measures
- Mean:
- Standard Deviation:
Trend
Overall Trend of the EUR Exchange Rate
The average exchange rate throughout the given period was approximately 1.4605. The highest observed exchange rate was 1.4647, while the lowest was at 1.45913. The exchange rate data shows a slight overall decline, from 1.4642 at the beginning of the timestamp recordings to 1.4596 towards the end. However, the variance is small, and the changes in exchange rate are relatively minimal, indicating a practically stable exchange rate over time.
Seasonality or Recurring Patterns
Analysing the data did not reveal any clear seasonality or cyclical patterns. The majority of the fluctuations in the exchange rate seem to occur in a random or chaotic manner, rather than following a clearly discernible trend. That being said, it is important to note that even when patterns may not be obvious to the human eye, they might be detected by time-series analysis algorithms.
Identifying Outliers
Identifying exact outliers in the data can be challenging due to the nature of exchange rates – they're influenced by a myriad of interconnecting factors and often exhibit a significant degree of noise. From a visual inspection, there don't seem to be any glaring outliers - most of the values seem to be fairly close to each other and follow a similar range. To identify outliers statistically, more advanced methods would need to be utilised, such as a Z-score method or employing a machine learning model.
Inference
In conclusion, the exchange rate given in the data indicates a moderately stable rate over time, with no clear seasonality or repeating patterns bellied in the data. Also, no glaring outliers were found. Further deeper analysis would require more granular data and probably considering external events and factors.
2024-04-21
Summary of Last Week
- Opening:
- Closing:
- Difference of Opening & Closing:
- Daily High:
- Daily Low:
- Difference of Daily High & Low:
Statistical Measures
- Mean:
- Standard Deviation:
Trend
1. Trend Analysis
From the data provided, it can be seen that there is a general upward trend in the Euro exchange rate from the beginning of the dataset at 1.46891 on 2024-03-22 to the end of the dataset at 1.46403 on 2024-04-19. This overall trend suggests an increase in exchange rate over the period shown. However, the increase is not steady – there are several peaks and troughs throughout the dataset that may indicate periods of increased volatility.
2. Seasonality Analysis
Identifying seasonality in financial time series data can be complex, as it requires more than simply looking at the raw data values. In this case, it appears that there is no clear seasonality or recurring pattern in EUR exchange rate changes. The exchange rate fluctuations seem to be more irregular and do not follow a consistent pattern over days or weeks.
3. Outliers Detection
Several potential outliers were detected where the exchange rate differs significantly from the surrounding rates. For instance, the exchange rate drops to 1.45922 on 2024-03-28 from 1.46813 on 2024-03-28. Another significant jump can be seen on 2024-04-16 with the exchange rate increasing from 1.46472 to 1.47016. These can be considered as potential outliers, although further statistical analysis would be required to confirm these observations.
Please note that the results obtained are based on the data provided and do not take into account external factors like market opening/closing hours, weekends/holidays, or the release of key financial news and reports which could potentially have significant impacts on the exchange rates. Also, this analysis does not generate any forecast for future rates.
2024-04-20
Summary of Yesterday
- Opening:
- Closing:
- Difference of Opening & Closing:
- Daily High:
- Daily Low:
- Difference of Daily High & Low:
Statistical Measures
- Mean:
- Standard Deviation:
Trend
1. Understanding the overall trend of the exchange rates:
The data provided contains EUR exchange rates for the period from 2024-04-15 to 2024-04-19. Upon observing the presented data, it appears that the exchange rates fluctuate within a tight range of 1.4617 to 1.4703 over that period. The lowest recorded value is of 1.4617 on 2024-04-15 09:00:03, and the highest recorded value is of 1.4703 on 2024-04-17 12:00:02. Overall, the exchange rates are not stable and show insignificant increasing and decreasing trends within the aforementioned range. It needs further statistical analysis like mean, median, mode, variance and standard deviation for more comprehensive understanding of the trend in the data.
2. Identifying seasonality or recurring patterns:
The data consists of roughly one week's worth of data, which may not be sufficient to identify clear seasonality or recurring patterns. However, a general observation is that the rates show slight variations according to the time of day, perhaps due to changes in market activity across different global markets. The data needs to be plotted across these times to visually examine any recurring patterns. Further data over a large span of time is required to thoroughly examine any seasonality.
3. Noting any outliers:
To identify outliers, a graph plotting the exchange rates against the timeline needs to be constructed, and any spikes or sharp declines would indicate an outlier. Upon a cursory review, The highest exchange rate value of 1.4703 is seen on 2024-04-17 12:00:02, which might be considered an outlier, but a thorough examination with methods like the Interquartile Range (IQR) will provide a more accurate understanding of any outliers.
2024-04-19
Summary of Yesterday
- Opening:
- Closing:
- Difference of Opening & Closing:
- Daily High:
- Daily Low:
- Difference of Daily High & Low:
Statistical Measures
- Mean:
- Standard Deviation:
Trend
1. Understanding the overall trend of the exchange rates
Assuming the given data points are in chronological order, we can see a slight overall upward trajectory in the EUR exchange rate over the period shown. The lowest value is 1.46403, which is noted at 2024-04-19 12:00:03, and the peak value is 1.46774, which is seen at 2024-04-19 08:20:02. There is some observable cyclic fluctuation within the dataset, but the general pattern seems to trend slightly upward.
2. Identifying seasonality or recurring patterns
We can spot some apparent short-term cyclical patterns where the exchange rate rises and falls within a certain range. However, without further data on a larger timescale, it is challenging to accurately identify any seasonality in this dataset. This is because seasonality usually occurs over a regular and predictable period, and can often be linked to the calendar cycle. It must be noted that such patterns could be due to several other factors that are not considered in this analysis.
3. Outliers and unusual instances
As for outliers - instances where the exchange rate differs significantly from the overall trend, detailed statistical analysis would be required to affirm this. From initial observation, we can't pinpoint specific data points that appear as clear-cut outliers. Certain points show more dramatic changes between consecutive time points, but none of them look extreme enough to be conclusively deemed as outliers. A proper statistical test would help confirm the presence and significance of outliers.
Note:
The analysis above is a primary examination based on the provided dataset. A more detailed analysis, incorporating more sophisticated techniques (e.g., statistical tests, time-series analysis methods), could bring further insight into the patterns and characteristics within the dataset. Given the inherent complexity and volatility of financial markets, comprehensive research and rigorous analyses are the standard practice.