What Does Gpt Stand For
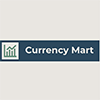
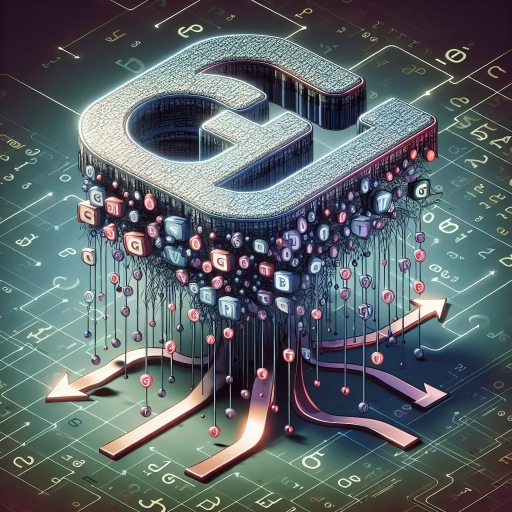
In the rapidly evolving landscape of artificial intelligence, one acronym has garnered significant attention: GPT. Standing for Generative Pre-trained Transformer, GPT represents a revolutionary leap in natural language processing (NLP) and machine learning. This technology, developed by OpenAI, has been transforming how we interact with computers and process information. To fully appreciate the impact of GPT, it is essential to delve into its core components. This article will explore the basics of GPT, explaining its fundamental principles and how it operates. We will also examine the technical aspects that make GPT so powerful, including its architecture and training methods. Finally, we will discuss the diverse applications and profound impact of GPT across various industries. By understanding these facets, readers will gain a comprehensive insight into what makes GPT a game-changer in AI. Let's begin by **Understanding the Basics of GPT**.
Understanding the Basics of GPT
In the rapidly evolving landscape of artificial intelligence, one technology has garnered significant attention and acclaim: Generative Pre-trained Transformers, commonly known as GPT. This cutting-edge AI model has revolutionized how we interact with machines, enabling them to generate human-like text and perform a wide range of tasks with unprecedented accuracy. To fully appreciate the capabilities of GPT, it is essential to delve into its fundamental aspects. This article will provide a comprehensive overview, starting with the **Definition and Full Form** of GPT, where we will explore what this acronym stands for and its core components. We will then delve into the **Historical Context and Development** of GPT, tracing its origins and key milestones in its evolution. Finally, we will examine the **Key Features and Capabilities** that make GPT so powerful, highlighting its ability to understand and generate coherent text. By understanding these basics, readers will gain a solid foundation for appreciating the transformative potential of GPT. In this article, we aim to demystify the complexities of GPT, making it accessible to a broad audience and providing a clear pathway to **Understanding the Basics of GPT**.
Definition and Full Form
Historical Context and Development
The development of GPT (Generative Pre-trained Transformer) is deeply rooted in the historical context of artificial intelligence and natural language processing. The journey began with the early days of AI, where researchers like Alan Turing and Marvin Minsky laid the groundwork for machine learning and neural networks. In the 1980s, the introduction of backpropagation by David Rumelhart, Geoffrey Hinton, and Ronald Williams revolutionized neural network training, paving the way for more complex models. The turn of the 21st century saw significant advancements with the rise of deep learning techniques. Researchers such as Yann LeCun, Yoshua Bengio, and Geoffrey Hinton further developed convolutional neural networks (CNNs) and recurrent neural networks (RNNs), which were instrumental in image recognition and sequence prediction tasks. However, these models had limitations when it came to handling long-range dependencies in text data. A pivotal moment came with the introduction of transformers by Vaswani et al. in 2017. This architecture replaced traditional RNNs and CNNs with self-attention mechanisms, allowing models to process sequences in parallel rather than sequentially. This innovation significantly improved performance on various natural language processing tasks. Building on this foundation, OpenAI developed GPT-1 in 2018, which was the first large-scale pre-trained language model using a transformer architecture. GPT-1 demonstrated remarkable capabilities in generating coherent text and performing well on downstream tasks with minimal fine-tuning. Subsequent versions—GPT-2 (2019) and GPT-3 (2020)—scaled up both in terms of model size and training data, leading to unprecedented levels of language understanding and generation. The historical context also includes ethical considerations and societal impacts. As GPT models became more sophisticated, concerns about bias, misinformation, and job displacement grew. This led to increased focus on responsible AI development, transparency in model training data, and ongoing research into mitigating these issues. In summary, the development of GPT is a culmination of decades of research in AI and NLP. From early neural networks to the transformative impact of transformers, each milestone has contributed to the creation of powerful language models like GPT. Understanding this historical context is crucial for appreciating the capabilities and limitations of GPT technology as it continues to evolve and shape our interactions with machines.
Key Features and Capabilities
**Key Features and Capabilities** Understanding the basics of GPT (Generative Pre-trained Transformer) involves delving into its key features and capabilities, which underpin its transformative impact on natural language processing. At its core, GPT is a type of deep learning model developed by OpenAI, designed to generate human-like text based on the input it receives. One of its most significant features is its ability to learn from vast amounts of data, allowing it to capture nuanced patterns and relationships within language. This pre-training phase, where the model is exposed to a massive corpus of text, equips GPT with a broad understanding of linguistic structures, idioms, and context-dependent expressions. A critical capability of GPT is its generative prowess. Unlike traditional language models that focus on specific tasks like translation or summarization, GPT can generate coherent and contextually relevant text across a wide range of topics and styles. This versatility makes it an invaluable tool for applications such as content creation, chatbots, and even creative writing. The model's architecture, based on the transformer framework, allows it to handle long-range dependencies in text more effectively than other models, ensuring that the generated text maintains coherence and logical flow. Another key feature is GPT's fine-tuning capability. While pre-training provides a robust foundation, fine-tuning allows the model to be adapted to specific tasks or domains. This process involves adjusting the model's parameters based on a smaller dataset relevant to the desired application, enabling GPT to perform exceptionally well in tasks such as sentiment analysis, question answering, and text classification. This adaptability has made GPT a go-to solution for various industries seeking to leverage advanced language processing capabilities. Moreover, GPT's ability to understand and respond to context is unparalleled. It can engage in conversations that feel natural and responsive, making it an excellent choice for customer service bots and virtual assistants. The model's capacity to learn from feedback also means it can improve over time, refining its responses based on user interactions. This continuous learning aspect ensures that GPT remains relevant and effective even as language usage evolves. In summary, GPT's key features—its extensive pre-training, generative capabilities, fine-tuning adaptability, contextual understanding, and continuous learning—make it a powerful tool in the realm of natural language processing. These capabilities collectively contribute to its versatility and effectiveness across various applications, solidifying its position as a leading technology in AI-driven language solutions. As understanding of GPT deepens, so does its potential to revolutionize how we interact with and generate text, opening up new avenues for innovation and efficiency in multiple sectors.
Technical Aspects of GPT
The advent of Generative Pre-trained Transformers (GPT) has revolutionized the landscape of natural language processing, enabling machines to generate human-like text with unprecedented accuracy and coherence. To fully appreciate the capabilities and limitations of GPT, it is crucial to delve into its technical aspects. This article will explore three pivotal components that underpin the functionality of GPT: **Architecture and Model Design**, which examines the structural framework and innovations that allow GPT to process and generate complex text; **Training Data and Algorithms**, which discusses the vast datasets and sophisticated algorithms used to train these models; and **Performance Metrics and Evaluation**, which analyzes how the performance of GPT is measured and evaluated. By understanding these technical facets, readers will gain a comprehensive insight into the inner workings of GPT, setting the stage for a deeper exploration of its applications and potential. This foundational knowledge is essential for **Understanding the Basics of GPT**, ensuring that readers can navigate the intricacies of this transformative technology with clarity and confidence.
Architecture and Model Design
In the realm of architecture and model design, particularly within the context of Technical Aspects of GPT (Generative Pre-trained Transformer), there exists a symbiotic relationship between the structural integrity of buildings and the intricate details of artificial intelligence models. Just as an architect meticulously designs every aspect of a building—from its foundation to its facade—ensuring both functionality and aesthetic appeal, the architects of AI models like GPT must carefully construct their frameworks. This involves layering complex neural networks, each with its own set of parameters and weights, to create a robust system capable of generating coherent and contextually relevant text. The foundational elements of GPT models are rooted in transformer architecture, which revolutionized the field by introducing self-attention mechanisms. These mechanisms allow the model to weigh the importance of different words in a sentence relative to each other, much like how an architect considers the interplay between various structural components to ensure stability and harmony. The transformer architecture is built upon encoder and decoder layers, each comprising multiple sub-layers such as multi-head self-attention and feed-forward neural networks. This layered approach mirrors the hierarchical design seen in architecture, where each component serves a specific purpose yet contributes to the overall functionality and beauty of the structure. Moreover, just as architects must balance form and function, AI model designers must strike a balance between model complexity and computational efficiency. Overly complex models can be computationally expensive and may suffer from issues like overfitting, while simpler models might lack the nuance required for sophisticated tasks. This balance is akin to an architect's challenge in designing a building that is both visually appealing and structurally sound. The training process for GPT models also parallels the iterative design process in architecture. Architects often create multiple prototypes and refine their designs based on feedback and performance metrics. Similarly, GPT models are trained on vast datasets and fine-tuned through iterative processes to optimize their performance on specific tasks. This iterative refinement ensures that the final product—whether it be a building or an AI model—is highly optimized for its intended purpose. In conclusion, the technical aspects of GPT models reflect many of the same principles that guide architectural design. From the foundational layers to the iterative refinement process, both disciplines require a deep understanding of structure, function, and aesthetics. By drawing parallels between these seemingly disparate fields, we can gain a deeper appreciation for the intricate craftsmanship involved in creating both physical structures and sophisticated AI models. This synergy underscores the importance of meticulous design in achieving excellence across various domains.
Training Data and Algorithms
Training data and algorithms are the cornerstone of advanced AI models like GPT (Generative Pre-trained Transformer). The quality and scope of the training data directly influence the performance, accuracy, and versatility of these models. High-quality training data must be diverse, comprehensive, and well-annotated to ensure that the model can learn a wide range of patterns and relationships. For instance, GPT models are trained on vast amounts of text data from various sources, including books, articles, and websites. This extensive dataset allows them to understand linguistic structures, context, and nuances of language. The algorithms used in training these models are equally crucial. GPT models leverage transformer architectures, which are particularly adept at handling sequential data like text. These architectures use self-attention mechanisms that enable the model to weigh the importance of different words in a sentence relative to each other, capturing complex dependencies and long-range relationships. This capability is pivotal for tasks such as language translation, text generation, and question answering. Moreover, the training process involves fine-tuning the model's parameters through techniques like masked language modeling and next sentence prediction. In masked language modeling, some words in the input text are randomly replaced with a special token, and the model predicts these missing words based on context. This approach helps the model develop a deep understanding of language semantics and syntax. The efficiency of these algorithms is further enhanced by distributed computing and parallel processing techniques, allowing large-scale models to be trained on massive datasets in reasonable timeframes. Additionally, advancements in hardware such as GPUs and TPUs have significantly accelerated the training process, making it feasible to train models with billions of parameters. In summary, the synergy between high-quality training data and sophisticated algorithms is what empowers GPT models to achieve remarkable performance in natural language processing tasks. The meticulous selection and preparation of training data combined with the innovative use of transformer architectures and advanced training techniques collectively contribute to the robustness and versatility of these AI systems. As research continues to evolve, we can expect even more refined models that leverage larger datasets and more sophisticated algorithms to push the boundaries of what is possible in AI-driven language understanding and generation.
Performance Metrics and Evaluation
**Performance Metrics and Evaluation** In the realm of AI, particularly within the context of GPT (Generative Pre-trained Transformer) models, performance metrics and evaluation play a crucial role in assessing the efficacy and reliability of these advanced language processing systems. These metrics serve as benchmarks to gauge how well a GPT model performs in various tasks such as text generation, translation, summarization, and conversational dialogue. **Key Metrics:** 1. **Perplexity:** This is a measure of how well a model predicts a sample of text. Lower perplexity scores indicate better performance. 2. **BLEU Score:** Commonly used for translation tasks, it measures the similarity between generated text and human-generated references. 3. **ROUGE Score:** Used for summarization tasks, it evaluates the overlap between generated summaries and reference summaries. 4. **F1 Score:** Often used in classification tasks to measure precision and recall. 5. **Human Evaluation:** Subjective evaluations by humans can provide insights into aspects like coherence, fluency, and relevance. **Evaluation Frameworks:** - **Automated Metrics:** Tools like BLEU and ROUGE provide quick, quantitative assessments but may not fully capture nuances in language. - **Human Evaluation:** While more time-consuming and subjective, human evaluators can assess aspects that automated metrics miss. - **Hybrid Approaches:** Combining automated metrics with human evaluation offers a balanced view of performance. **Challenges:** - **Contextual Understanding:** GPT models must be evaluated on their ability to understand context and generate coherent responses. - **Bias Detection:** Evaluating for biases in generated text is crucial to ensure fairness and ethical use. - **Adversarial Testing:** Testing models against adversarial examples helps in identifying vulnerabilities. **Best Practices:** - **Multi-Faceted Evaluation:** Using a combination of metrics to get a comprehensive view of performance. - **Continuous Monitoring:** Regularly updating and re-evaluating models as new data becomes available. - **Transparency:** Clearly documenting evaluation methods and results to facilitate reproducibility and trust. By leveraging these performance metrics and evaluation frameworks, developers can refine GPT models to achieve higher accuracy, relevance, and overall performance. This meticulous approach ensures that these AI systems not only meet but exceed expectations in real-world applications, making them indispensable tools in various industries such as customer service, content creation, and research.
Applications and Impact of GPT
The advent of Generative Pre-trained Transformers (GPT) has revolutionized the landscape of artificial intelligence, particularly in the realm of natural language processing. This transformative technology has not only enhanced our ability to generate coherent and contextually relevant text but also opened up a myriad of applications across various industries. In this article, we will delve into the multifaceted uses of GPT, exploring its impact on natural language processing, its far-reaching effects on diverse sectors, and the future potential it holds along with the ethical considerations that come with such powerful technology. We will examine how GPT is being utilized in natural language processing to achieve unprecedented levels of accuracy and efficiency. Additionally, we will discuss how different industries are leveraging GPT to innovate and streamline their operations. Finally, we will consider the future implications of GPT and the ethical dilemmas it presents. By understanding these aspects, readers will gain a comprehensive insight into the applications and impact of GPT, setting the stage for a deeper exploration into the basics of this groundbreaking technology.
Use Cases in Natural Language Processing
Natural Language Processing (NLP) has revolutionized the way we interact with technology, and its use cases are diverse and impactful. Within the broader context of Applications and Impact of GPT, NLP's role is particularly significant. GPT, or Generative Pre-trained Transformer, is a powerful AI model that leverages NLP to generate human-like text. Here are some key use cases that highlight the versatility and importance of NLP: 1. **Chatbots and Virtual Assistants**: NLP enables chatbots to understand and respond to user queries in a more natural and intuitive way. GPT models can be fine-tuned to create sophisticated chatbots that provide customer support, answer frequently asked questions, and even engage in conversational dialogue. 2. **Sentiment Analysis**: By analyzing text data, NLP can determine the sentiment or emotional tone behind user feedback, reviews, or social media posts. This is crucial for businesses looking to gauge customer satisfaction and make data-driven decisions. 3. **Language Translation**: NLP facilitates real-time language translation, breaking down communication barriers across the globe. GPT models can be trained on multilingual datasets to enhance translation accuracy and fluency. 4. **Content Generation**: GPT's ability to generate coherent and contextually relevant text makes it an invaluable tool for content creation. Whether it's generating articles, blog posts, or even entire books, NLP-driven models like GPT are redefining content production. 5. **Summarization and Extraction**: NLP can automatically summarize long documents or extract key information from large datasets, saving time and increasing efficiency in various industries such as law, medicine, and finance. 6. **Speech Recognition**: While often associated with speech-to-text systems, NLP also plays a critical role in improving speech recognition accuracy by understanding the nuances of spoken language. 7. **Healthcare Applications**: In healthcare, NLP is used to analyze medical records, diagnose conditions based on patient histories, and even predict patient outcomes. GPT models can help in generating personalized treatment plans and patient communication. 8. **Education**: NLP-powered tools can assist in grading assignments, providing feedback on essays, and even creating personalized learning plans tailored to individual students' needs. 9. **Marketing and Advertising**: By analyzing consumer behavior through text data, NLP helps marketers understand their target audience better and craft more effective marketing campaigns. 10. **Legal and Compliance**: In legal contexts, NLP aids in document review, contract analysis, and compliance monitoring by identifying key clauses and potential risks within large volumes of text. These use cases demonstrate how NLP, particularly when powered by advanced models like GPT, has the potential to transform various sectors by enhancing efficiency, accuracy, and user experience. As GPT continues to evolve, its applications will likely expand further into new domains, driving innovation and societal impact.
Impact on Various Industries
The advent of GPT (Generative Pre-trained Transformer) has revolutionized various industries, transforming the way businesses operate, innovate, and interact with customers. In the **healthcare sector**, GPT is being leveraged to analyze vast amounts of medical data, helping in the early detection of diseases and personalized treatment plans. For instance, GPT models can process patient histories, medical literature, and genomic data to provide accurate diagnoses and suggest effective treatments. This not only enhances patient care but also streamlines clinical workflows. In **education**, GPT is redefining the learning experience by creating personalized educational content tailored to individual students' needs. It can generate adaptive learning materials, assist in grading assignments, and even provide real-time feedback to students. This technology also aids in automating administrative tasks, allowing educators to focus more on teaching and mentoring. The **financial industry** is also experiencing a significant impact from GPT. It is used for risk assessment, fraud detection, and portfolio management. GPT models can analyze market trends, predict stock prices, and identify potential risks more accurately than traditional methods. Additionally, these models can generate detailed financial reports and compliance documents, reducing the workload for financial analysts. In **customer service**, GPT-powered chatbots are revolutionizing how companies interact with their customers. These AI-driven chatbots can handle a wide range of queries, provide instant support, and offer personalized recommendations based on customer data. This enhances customer satisfaction while reducing the need for human intervention in routine inquiries. The **media and entertainment industry** is also benefiting from GPT technology. It is being used to generate content such as news articles, scripts for TV shows and movies, and even entire books. This not only speeds up content creation but also opens up new possibilities for creative storytelling. Furthermore, **software development** has seen a boost with GPT. Developers can use these models to generate code snippets, debug existing code, and even create entire applications from scratch. This accelerates the development process and reduces the likelihood of errors. Lastly, in **marketing**, GPT is helping businesses craft compelling content that resonates with their target audience. It can generate social media posts, blog articles, and email campaigns that are tailored to specific demographics. This improves engagement rates and helps companies build stronger brand connections. Overall, the impact of GPT across various industries is profound. It enhances efficiency, innovation, and customer satisfaction while opening up new avenues for growth and development. As this technology continues to evolve, its applications are likely to expand even further, transforming more sectors in the process.
Future Potential and Ethical Considerations
As we delve into the applications and impact of GPT (Generative Pre-trained Transformer), it is crucial to consider both its future potential and the ethical considerations that come with such advanced technology. The future potential of GPT is vast and multifaceted, promising to revolutionize various sectors including education, healthcare, customer service, and content creation. For instance, GPT can be used to generate personalized educational materials tailored to individual learning styles, enhance patient care by providing accurate medical information, and streamline customer interactions through intelligent chatbots. Moreover, its ability to produce coherent and contextually relevant text could transform the way we approach content creation, from automated news articles to creative writing. However, alongside these promising applications come significant ethical considerations. One of the primary concerns is data privacy and security. GPT models are trained on vast amounts of data, which raises questions about the sourcing and handling of this data. Ensuring that personal information is anonymized and protected is paramount to avoid potential misuse. Another ethical issue is bias and fairness; GPT models can inherit biases present in the training data, leading to discriminatory outcomes in real-world applications. This necessitates careful curation of training datasets and ongoing monitoring for bias. Additionally, the use of GPT raises questions about authorship and intellectual property. As these models generate content that is often indistinguishable from human-written text, it challenges traditional notions of creativity and ownership. There is a need for clear guidelines on how to attribute work generated by AI models and how to protect original creators from potential plagiarism or misuse. Furthermore, the potential for misinformation and disinformation is a critical ethical concern. GPT's ability to generate convincing text can be exploited to spread false information or propaganda, which could have serious societal implications. Therefore, it is essential to develop robust mechanisms for verifying the authenticity of AI-generated content and holding accountable those who misuse this technology. In conclusion, while GPT holds immense potential for transforming various aspects of our lives, it is imperative that we address the ethical considerations associated with its development and deployment. By doing so, we can ensure that this powerful technology benefits society while minimizing its risks and negative impacts. This balanced approach will be key to harnessing the full potential of GPT in a responsible and ethical manner.