What Is Logit Group
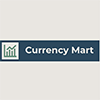
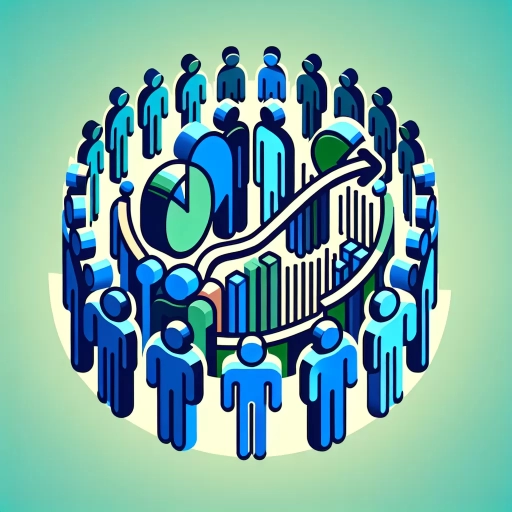
In the realm of data analysis and statistical modeling, the concept of a logit group has emerged as a powerful tool for understanding and predicting outcomes in various fields. A logit group, fundamentally, is a set of logistic regression models applied to different segments or groups within a dataset, allowing for more nuanced and accurate predictions. This article delves into the intricacies of logit groups, starting with an in-depth exploration of **Understanding the Concept of Logit Group**, where we will dissect the theoretical underpinnings and practical applications of this methodology. We will then examine **Applications and Uses of Logit Group**, highlighting how this approach is utilized in diverse domains such as marketing, healthcare, and finance. Finally, we will discuss **Benefits and Challenges of Implementing Logit Group**, shedding light on the advantages and potential pitfalls of integrating this technique into your analytical toolkit. By grasping these key aspects, readers will gain a comprehensive understanding of how logit groups can enhance predictive modeling and decision-making processes. Let us begin by **Understanding the Concept of Logit Group**.
Understanding the Concept of Logit Group
In the realm of statistical analysis and data modeling, the concept of Logit Group has emerged as a pivotal tool for understanding and predicting outcomes in various fields, including economics, sociology, and marketing. This article delves into the intricacies of Logit Group, providing a comprehensive overview that is both informative and engaging. To fully grasp the concept, it is essential to explore its **Definition and Origin**, which traces back to the early days of logistic regression and its evolution into group-level analysis. Additionally, understanding the **Key Characteristics and Features** of Logit Group is crucial for appreciating its applicability and strengths in real-world scenarios. Finally, examining the **Historical Context and Evolution** of Logit Group offers insights into how this methodology has adapted over time to meet the demands of increasingly complex data sets. By exploring these three facets, readers will gain a deeper understanding of the Logit Group concept and its significance in contemporary research and analysis. This article aims to provide a clear and detailed exploration, transitioning seamlessly into **Understanding the Concept of Logit Group**.
Definition and Origin
**Definition and Origin** The concept of a Logit Group, rooted in statistical analysis and data modeling, is a crucial component in understanding how categorical data can be effectively analyzed and interpreted. At its core, a Logit Group refers to a set of categories or outcomes that are analyzed together using logistic regression, a statistical method for predicting the outcome of a categorical dependent variable based on one or more predictor variables. This technique is particularly useful in fields such as marketing, social sciences, and healthcare where outcomes are often binary or multi-categorical. The origin of logistic regression, and by extension the Logit Group, dates back to the early 20th century. The term "logit" itself was coined by Joseph Berkson in 1944, derived from "logistic unit," reflecting the mathematical transformation involved in the model. Berkson's work built upon earlier contributions by mathematicians and statisticians who sought to model binary outcomes using probability theory. Over time, logistic regression evolved to accommodate more complex scenarios, including multi-categorical outcomes, leading to the development of multinomial logistic regression. In practice, a Logit Group is formed when researchers or analysts group multiple categories together to analyze their relationships with predictor variables. For instance, in market research, a Logit Group might consist of different product preferences (e.g., brand A, brand B, brand C) that are analyzed to understand how demographic factors influence consumer choice. By applying logistic regression to these groups, analysts can derive odds ratios and probabilities that help in predicting the likelihood of each outcome given certain conditions. The significance of understanding Logit Groups lies in their ability to provide nuanced insights into complex decision-making processes. By identifying patterns and relationships within these groups, researchers can make informed decisions that drive strategy and policy. For example, in healthcare, analyzing patient outcomes as part of a Logit Group can help in identifying risk factors associated with different health conditions, thereby enabling targeted interventions. In summary, the concept of a Logit Group is deeply intertwined with the principles of logistic regression and has its roots in early statistical modeling techniques. Understanding how to form and analyze these groups is essential for anyone looking to extract meaningful insights from categorical data, making it a foundational aspect of data-driven decision-making across various disciplines.
Key Characteristics and Features
Understanding the concept of a Logit Group hinges on identifying its key characteristics and features, which are pivotal in grasping its functionality and application. A Logit Group, fundamentally, is a statistical method used in logistic regression analysis to categorize outcomes into distinct groups based on a set of predictor variables. One of the primary characteristics of a Logit Group is its ability to handle binary or multinomial outcomes, making it versatile for various types of data. This method leverages the logistic function to model the probability of an event occurring, thereby enabling researchers to predict the likelihood of an outcome falling into one category versus another. A crucial feature of Logit Groups is their reliance on odds ratios, which provide a measure of association between the predictor variables and the outcome. These ratios are essential for interpreting the results, as they indicate the change in odds of an event occurring with a one-unit change in the predictor variable, while controlling for other variables. Another significant aspect is the use of maximum likelihood estimation to derive the model parameters, ensuring that the model fits the data optimally. The interpretability of Logit Groups is enhanced by their ability to generate probability scores for each category, allowing for clear classification and prediction. This is particularly useful in fields such as marketing, where customer segmentation can be based on the probability of purchasing a product, or in healthcare, where patient outcomes can be predicted based on various risk factors. Additionally, Logit Groups can handle both continuous and categorical predictor variables, making them adaptable to diverse datasets. Moreover, the diagnostic tools associated with Logit Groups, such as the Hosmer-Lemeshow test and pseudo R-squared measures, provide insights into the model's goodness of fit and predictive power. These diagnostics are crucial for validating the model's accuracy and reliability. The flexibility of Logit Groups also extends to their ability to be extended into more complex models, such as generalized linear mixed models, which can account for random effects and clustering in data. In summary, the key characteristics and features of Logit Groups include their application in logistic regression, use of odds ratios for interpretation, reliance on maximum likelihood estimation, generation of probability scores, adaptability to various types of data, and robust diagnostic tools. These attributes collectively make Logit Groups a powerful tool for predictive modeling and classification tasks across multiple disciplines. By understanding these characteristics, researchers and analysts can effectively leverage Logit Groups to derive meaningful insights from their data.
Historical Context and Evolution
The concept of Logit Group, a statistical method used in data analysis, is deeply rooted in historical developments within the fields of mathematics, statistics, and social sciences. To fully understand the significance and application of Logit Group, it is essential to delve into its historical context and evolution. The origins of logistic regression, the foundational technique behind Logit Group, date back to the early 20th century. In the 1920s and 1930s, biostatisticians like Chester Ittner Bliss and David Finney began exploring the use of logistic functions to model binary outcomes in biological and agricultural studies. Bliss, in particular, is credited with developing the probit model, which laid the groundwork for later logistic regression models. The term "logit" itself was coined by Joseph Berkson in 1944, derived from the term "logistic unit." Berkson's work introduced the logit function as a way to transform probabilities into a linear form, making it easier to analyze binary data. This innovation marked a significant shift towards more sophisticated statistical modeling. In the mid-20th century, advances in computing technology enabled the widespread adoption of logistic regression. The development of algorithms and software packages facilitated the practical application of these models across various disciplines. By the 1970s and 1980s, logistic regression had become a staple in social sciences, particularly in fields such as economics, sociology, and political science. The evolution of Logit Group as a specific application of logistic regression can be traced to the need for more nuanced analysis within grouped data. In many research contexts, data is naturally aggregated into groups or clusters based on certain criteria. Traditional logistic regression models treat each observation independently, which can overlook important group-level effects. The Logit Group approach addresses this limitation by incorporating group-specific parameters, allowing researchers to capture both individual and group-level influences on binary outcomes. This methodological advancement has been particularly beneficial in fields like marketing, where customer behavior is often analyzed at both individual and segment levels. Similarly, in healthcare research, Logit Group models help in understanding disease prevalence and treatment outcomes across different patient groups. In conclusion, the historical context and evolution of Logit Group are intertwined with broader developments in statistical theory and computational capabilities. From its roots in early 20th-century biostatistics to its current applications across diverse fields, the Logit Group method represents a sophisticated tool for analyzing complex binary data within grouped structures. Understanding this historical trajectory not only enriches our appreciation of the technique but also highlights its versatility and relevance in contemporary research.
Applications and Uses of Logit Group
In the realm of data-driven decision-making, the Logit Group stands as a pivotal force, offering a suite of applications that transform raw data into actionable insights. This article delves into the multifaceted uses of the Logit Group, highlighting its impact across various domains. We begin by examining **Market Research and Consumer Behavior Analysis**, where Logit Group tools enable businesses to understand consumer preferences and behaviors, thereby informing product development and marketing strategies. Next, we explore **Statistical Modeling and Data Analysis**, showcasing how the Logit Group's advanced statistical techniques help in predicting outcomes and identifying trends. Finally, we discuss **Business Strategy and Decision Making**, illustrating how these insights are integrated into strategic planning to drive business growth. By understanding these applications, we can appreciate the comprehensive value that the Logit Group brings to the table, ultimately leading us to a deeper **Understanding of the Concept of Logit Group**.
Market Research and Consumer Behavior Analysis
Market research and consumer behavior analysis are pivotal components in the strategic arsenal of any successful business, and they find robust support through the applications and uses of Logit Group. Logit Group, a leading provider of market research solutions, empowers businesses to delve deep into the psyche of their consumers, uncovering hidden patterns and preferences that drive purchasing decisions. By leveraging advanced statistical models and sophisticated data analytics, Logit Group enables companies to conduct comprehensive market research that goes beyond mere data collection; it provides actionable insights. Consumer behavior analysis, a core aspect of market research, involves studying the factors that influence how consumers make purchasing decisions. This includes understanding demographic characteristics, psychological factors, social influences, and cultural background. Logit Group's tools and methodologies allow businesses to segment their markets effectively, identifying high-value customer segments and tailoring marketing strategies to resonate with these groups. For instance, through conjoint analysis—a technique often facilitated by Logit Group—companies can determine how different product features impact consumer preferences, enabling them to design products that meet specific market needs. Moreover, Logit Group's expertise in choice modeling helps businesses predict how changes in product attributes or market conditions will affect consumer choices. This predictive capability is crucial for making informed decisions about product launches, pricing strategies, and marketing campaigns. By analyzing consumer behavior through the lens of choice modeling, companies can anticipate market trends and adjust their strategies accordingly to stay ahead of the competition. In addition to these analytical tools, Logit Group's market research solutions also incorporate qualitative methods such as focus groups and interviews. These qualitative approaches provide rich contextual data that complements quantitative findings, offering a more holistic understanding of consumer motivations and behaviors. This integrated approach ensures that businesses gain a nuanced view of their target audience, allowing them to craft compelling brand narratives and develop customer-centric strategies. The applications of Logit Group's market research and consumer behavior analysis extend across various industries. For example, in the retail sector, these insights can inform inventory management decisions by predicting demand for different products. In healthcare, they can help pharmaceutical companies understand patient preferences for new treatments. In technology, they can guide software development by identifying key features that users value most. In summary, Logit Group's market research and consumer behavior analysis capabilities are essential for businesses seeking to navigate complex markets effectively. By providing robust analytical tools and methodologies, Logit Group empowers companies to make data-driven decisions that align with consumer needs and preferences. This synergy between market research and consumer insights not only enhances business performance but also fosters long-term customer loyalty and satisfaction.
Statistical Modeling and Data Analysis
Statistical modeling and data analysis are cornerstone components in the arsenal of any data-driven organization, and they play a pivotal role in the applications and uses of Logit Group. Logit Group, specializing in advanced statistical techniques, leverages these methodologies to uncover insights, predict outcomes, and inform strategic decisions. At its core, statistical modeling involves the development of mathematical representations to describe relationships between variables within a dataset. This process is crucial for understanding complex phenomena and making accurate predictions. In the context of Logit Group, statistical modeling is employed to analyze large datasets, often involving multiple variables and intricate relationships. For instance, in market research, Logit Group might use logistic regression models to predict consumer behavior based on demographic data, purchasing history, and other relevant factors. These models help businesses identify high-value customer segments, optimize marketing campaigns, and forecast sales trends. Data analysis, a complementary discipline, involves the systematic examination of data to draw meaningful conclusions. Logit Group's data analysts use a variety of techniques including hypothesis testing, confidence intervals, and regression analysis to extract actionable insights from raw data. This process is essential for validating the assumptions underlying statistical models and ensuring that the insights derived are reliable and applicable. One of the key applications of statistical modeling and data analysis by Logit Group is in risk assessment and management. By developing sophisticated models that account for various risk factors, Logit Group helps financial institutions and other organizations mitigate potential risks and make informed investment decisions. For example, credit scoring models developed by Logit Group can predict the likelihood of loan defaults based on historical credit data, thereby enabling lenders to make more informed lending decisions. Moreover, Logit Group's expertise in statistical modeling and data analysis extends to healthcare, where it aids in clinical trial design, patient outcome prediction, and disease surveillance. By analyzing large datasets of patient records and clinical trial data, Logit Group can identify patterns that may not be immediately apparent, leading to better treatment protocols and improved patient care. In summary, the integration of statistical modeling and data analysis is fundamental to the services offered by Logit Group. These methodologies enable the company to deliver high-value insights that drive business growth, improve decision-making processes, and solve complex problems across various industries. Whether it's predicting consumer behavior, assessing financial risk, or advancing healthcare outcomes, Logit Group's expertise in statistical modeling and data analysis stands as a testament to the power of data-driven decision-making.
Business Strategy and Decision Making
In the realm of business strategy and decision making, the integration of advanced analytics and data-driven insights is paramount for achieving competitive advantage. Logit Group, a leading provider of data analytics solutions, plays a pivotal role in this context by offering tools and methodologies that enhance strategic planning and informed decision-making processes. By leveraging Logit Group's capabilities, businesses can transform raw data into actionable intelligence, enabling them to navigate complex market landscapes with greater precision. At the core of effective business strategy lies the ability to make informed decisions quickly and accurately. Logit Group's solutions facilitate this by providing comprehensive data analysis, predictive modeling, and market research insights. For instance, through the use of logistic regression and other statistical techniques, businesses can predict customer behavior, identify market trends, and assess the likelihood of various outcomes. This predictive power allows companies to anticipate challenges and opportunities, thereby enabling proactive rather than reactive decision-making. Moreover, Logit Group's applications extend beyond predictive analytics to include optimization techniques that help businesses allocate resources efficiently. By analyzing vast datasets, companies can identify optimal pricing strategies, supply chain configurations, and marketing campaigns that maximize returns while minimizing costs. This holistic approach ensures that every aspect of the business is aligned with overarching strategic goals. Another critical aspect of business strategy is risk management. Logit Group's advanced analytics tools help companies quantify and mitigate risks by analyzing historical data and simulating future scenarios. This risk assessment capability is particularly valuable in volatile markets where unforeseen events can significantly impact business operations. By understanding potential risks and opportunities, businesses can develop robust contingency plans that safeguard their interests. Furthermore, the insights provided by Logit Group are not limited to internal operations; they also offer valuable external perspectives. Market segmentation analysis, for example, helps businesses understand their target audiences better, allowing for more tailored marketing strategies that resonate with specific customer segments. This targeted approach not only enhances brand loyalty but also drives revenue growth by ensuring that marketing efforts are focused on high-value customers. In conclusion, the applications and uses of Logit Group in business strategy and decision making are multifaceted and transformative. By harnessing the power of data analytics, predictive modeling, optimization techniques, risk management, and market research, businesses can craft and execute strategies that are both agile and resilient. As the business landscape continues to evolve, the role of Logit Group in enabling data-driven decision making will remain indispensable for companies seeking to stay ahead of the curve.
Benefits and Challenges of Implementing Logit Group
The implementation of Logit Group, a sophisticated analytical tool, presents a dual-edged sword for organizations seeking to enhance their predictive capabilities. On one hand, Logit Group offers significant advantages in predictive analytics and forecasting, enabling businesses to make informed decisions by accurately modeling complex data sets. This can lead to improved operational efficiency, better resource allocation, and enhanced strategic planning. However, the journey to leveraging these benefits is not without its challenges. One of the primary hurdles is the complexity involved in data collection and interpretation, which can be time-consuming and require specialized expertise. Additionally, effective implementation of Logit Group necessitates adherence to best practices that ensure data quality, model validation, and continuous monitoring. Understanding these facets is crucial for organizations aiming to maximize the potential of Logit Group. As we delve into the intricacies of this powerful tool, it becomes evident that grasping its full potential requires a comprehensive understanding of its benefits, challenges, and optimal implementation strategies. This article will explore these aspects in detail, ultimately leading to a deeper understanding of the concept of Logit Group.
Advantages in Predictive Analytics and Forecasting
Predictive analytics and forecasting are pivotal components in the arsenal of modern businesses, offering a myriad of advantages that can significantly enhance decision-making processes. At the heart of these benefits lies the ability to anticipate future trends and outcomes with a high degree of accuracy. By leveraging historical data, statistical models, and machine learning algorithms, predictive analytics enables organizations to identify patterns and correlations that might otherwise remain hidden. This foresight allows companies to proactively manage risks, capitalize on opportunities, and optimize operations. For instance, in supply chain management, predictive analytics can forecast demand fluctuations, enabling businesses to adjust inventory levels and logistics accordingly, thereby reducing costs and improving customer satisfaction. Moreover, predictive analytics fosters a culture of data-driven decision-making. By providing actionable insights, it empowers executives to make informed choices rather than relying on intuition or historical trends alone. In marketing, predictive models can segment customer bases more effectively, allowing for targeted campaigns that yield higher conversion rates. Similarly, in finance, predictive analytics can help in credit risk assessment, fraud detection, and portfolio optimization, leading to more robust financial health. Another significant advantage is the enhancement of operational efficiency. Predictive maintenance, for example, can predict when equipment is likely to fail, allowing for timely interventions that prevent costly downtime. This not only saves resources but also ensures continuous production and service delivery. Additionally, predictive analytics can improve resource allocation by forecasting resource needs accurately, thereby optimizing resource utilization across various departments. However, the integration of predictive analytics and forecasting into an organization's framework, such as within a Logit Group setup, also presents certain challenges. One of the primary hurdles is the requirement for high-quality data; inaccurate or incomplete data can lead to flawed predictions. Furthermore, the complexity of some predictive models necessitates specialized skills and expertise, which can be a barrier for smaller organizations or those without a robust analytics team. Despite these challenges, the advantages of predictive analytics far outweigh the costs, making it an indispensable tool for any forward-thinking business aiming to stay ahead in a competitive landscape. In the context of implementing a Logit Group, which often involves complex logistical and operational considerations, predictive analytics can be particularly beneficial. It can help in optimizing routes for delivery fleets, managing inventory levels across multiple locations, and predicting demand spikes or dips. By integrating predictive analytics into their operations, Logit Groups can streamline their processes, reduce operational costs, and enhance overall efficiency. This synergy between predictive analytics and logistical operations underscores the critical role that data-driven insights play in modern business strategies.
Challenges in Data Collection and Interpretation
Implementing a Logit Group, a sophisticated analytical tool for data-driven decision-making, comes with several benefits but also poses significant challenges, particularly in the realms of data collection and interpretation. One of the primary challenges is ensuring the quality and integrity of the data. Logit models rely heavily on accurate and comprehensive datasets to produce reliable results. However, collecting such data can be arduous due to issues like missing values, outliers, and inconsistencies. For instance, in market research, respondents may provide incomplete or biased answers, which can skew the analysis. Additionally, integrating data from various sources often involves dealing with different formats and standards, making harmonization a time-consuming task. Another challenge lies in the complexity of interpreting the results. Logit models generate coefficients and odds ratios that require a deep understanding of statistical analysis to interpret correctly. Misinterpretation can lead to incorrect conclusions and misguided decisions. Furthermore, the models assume certain conditions about the data distribution and relationships, which may not always hold true in real-world scenarios. This necessitates rigorous validation and sensitivity analysis to ensure that the findings are robust. Moreover, ethical considerations play a crucial role in data collection. Ensuring privacy and compliance with regulations such as GDPR or HIPAA is essential but can add layers of complexity to the process. Balancing the need for detailed data with the need to protect individual privacy is a delicate task that requires careful planning and execution. Technological limitations also present a hurdle. Advanced computational resources are often required to handle large datasets efficiently, and not all organizations have access to such infrastructure. Moreover, the rapid evolution of technology means that tools and methods can become outdated quickly, necessitating continuous updates and training for analysts. Lastly, there is the issue of stakeholder buy-in and communication. Logit models can produce nuanced insights that may be difficult to communicate effectively to non-technical stakeholders. This can lead to resistance or misunderstanding of the results, hindering the implementation of recommendations derived from the analysis. In summary, while Logit Groups offer powerful analytical capabilities, they demand meticulous attention to data quality, statistical expertise for interpretation, adherence to ethical standards, robust technological infrastructure, and effective communication strategies. Addressing these challenges is crucial for leveraging the full potential of Logit Groups in decision-making processes.
Best Practices for Effective Implementation
Effective implementation of Logit Group, a comprehensive logistics and supply chain management solution, hinges on several best practices that ensure seamless integration, optimal performance, and sustained benefits. **Clear Communication** is paramount; it involves aligning all stakeholders on the project's goals, timelines, and expectations. This transparency fosters a collaborative environment where team members are aware of their roles and responsibilities, reducing misunderstandings and misalignments. **Thorough Planning** is another critical aspect; it entails conducting a detailed needs assessment to identify specific pain points within the current logistics operations. This step helps in tailoring the implementation to address unique challenges and leverage existing strengths. **Training and Support** are essential components for successful implementation. Providing comprehensive training programs for staff ensures that they are proficient in using the new system, minimizing the learning curve and reducing errors. Ongoing support from both internal IT teams and external vendors is crucial for addressing any issues promptly, maintaining system stability, and ensuring continuous improvement. **Phased Rollout** can also mitigate risks associated with large-scale changes. By implementing Logit Group in stages, organizations can test the system in controlled environments before full deployment, allowing for iterative refinements based on real-world feedback. **Data Integration** is a key best practice; it involves ensuring that all relevant data sources are seamlessly integrated into the Logit Group platform. This includes historical data, real-time tracking information, and other critical metrics that drive decision-making. Effective data integration enhances the accuracy of analytics, improves forecasting capabilities, and supports more informed strategic decisions. **Continuous Monitoring and Evaluation** are vital for long-term success. Regularly assessing the performance of the system against predefined KPIs helps in identifying areas for improvement and measuring the overall impact of the implementation. Additionally, **Change Management** should be a focal point. Implementing a new system often requires significant cultural and operational changes within an organization. Effective change management involves engaging with employees at all levels to address concerns, build buy-in, and foster a culture of adaptability. This approach ensures that the transition to Logit Group is smooth and sustainable. By adhering to these best practices, organizations can maximize the benefits of implementing Logit Group while minimizing potential challenges, ultimately leading to enhanced operational efficiency, improved customer satisfaction, and increased competitiveness in the market.