What Does Mtl Mean
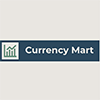
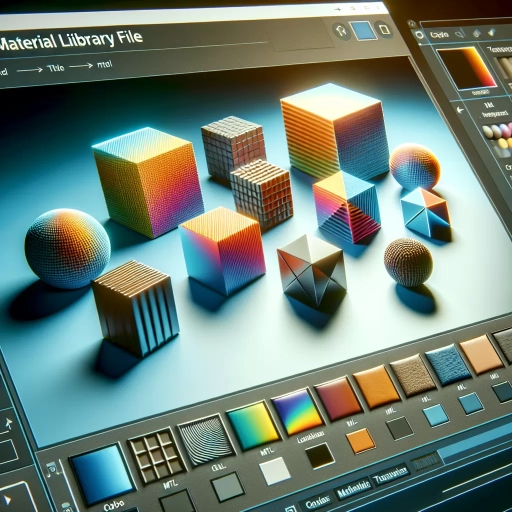
In today's rapidly evolving technological landscape, the term "MTL" has gained significant attention across various disciplines. MTL, or Machine Translation, is a critical component of artificial intelligence and natural language processing, enabling computers to translate text from one language to another without human intervention. This article delves into the multifaceted aspects of MTL, starting with **Understanding the Term MTL**, where we explore its definition, historical development, and current state. We then examine **Applications of MTL in Various Fields**, highlighting its use in industries such as education, healthcare, and global communication. Finally, we discuss **Benefits and Challenges of Implementing MTL**, analyzing its advantages in enhancing cross-cultural interactions and the hurdles it faces in achieving perfect translation accuracy. By grasping these elements, readers will gain a comprehensive understanding of MTL's role and impact. Let's begin by understanding the term MTL itself.
Understanding the Term MTL
Understanding the term "MTL" requires a multifaceted approach, as it encompasses various dimensions that shed light on its significance and evolution. To delve into the essence of MTL, it is crucial to explore its definition and origins, which provide the foundational understanding of what the term represents. Additionally, examining its common usage in different contexts reveals how MTL is applied across various fields, highlighting its versatility and relevance. Finally, tracing the historical development of the term offers insights into how MTL has evolved over time, influenced by technological advancements and societal needs. By examining these aspects, we can gain a comprehensive understanding of MTL. Let us begin by defining and exploring the origins of this term, which will serve as the cornerstone for our deeper analysis.
Definition and Origins
**Understanding the Term MTL** ### Definition and Origins The term "MTL" stands for "Machine Translation" or "Machine Translation Learning," depending on the context. In the realm of artificial intelligence and natural language processing, Machine Translation (MT) refers to the use of software to translate text or speech from one language to another. This technology leverages algorithms and statistical models to interpret and generate text in different languages, aiming to produce translations that are as accurate and natural-sounding as possible. The origins of Machine Translation date back to the 1950s, when the first attempts were made to automate translation processes using rule-based systems. These early systems relied on hand-coded rules and dictionaries to translate text, but they were limited in their ability to handle nuances and complexities of language. The advent of statistical machine translation in the 1990s marked a significant shift, as it introduced the use of large datasets and statistical models to improve translation accuracy. This approach allowed for more flexible and adaptive translation systems. In recent years, the rise of deep learning techniques has further revolutionized Machine Translation. Neural Machine Translation (NMT) models, which use neural networks to learn the patterns and structures of languages, have become the standard for high-quality translations. These models are trained on vast amounts of parallel text data and can capture contextual relationships and subtleties that were previously challenging to translate accurately. The term "MTL" can also refer to "Multi-Task Learning," a concept in machine learning where a single model is trained to perform multiple related tasks simultaneously. This approach is particularly relevant in the context of natural language processing, where models can be trained to handle various tasks such as translation, summarization, and sentiment analysis. By learning multiple tasks concurrently, these models can develop a deeper understanding of language and improve their overall performance across different tasks. In summary, "MTL" primarily denotes Machine Translation or Machine Translation Learning, with roots tracing back to the early days of computational linguistics. The evolution from rule-based systems to statistical models and now neural networks has significantly enhanced the capabilities of machine translation, making it an indispensable tool in global communication and information exchange. Understanding these definitions and origins is crucial for appreciating the advancements and potential applications of machine translation technology.
Common Usage in Different Contexts
The term "MTL" can be understood through its various common usages in different contexts, which highlight its versatility and widespread application. In the realm of finance, MTL stands for "Multi-Tiered Licensing," a model used by software companies to offer different levels of access and features based on the user's needs and willingness to pay. This approach allows businesses to cater to a broad customer base, from individual users to large enterprises, by providing tiered pricing and feature sets. In technology, particularly in the field of blockchain and cryptocurrency, MTL refers to "Metal," a cryptocurrency designed to incentivize people to buy, sell, and trade goods and services using blockchain technology. Metal aims to make financial transactions more transparent, secure, and rewarding by offering a token that can be earned through everyday purchases. In the context of education and language learning, MTL can stand for "Mother Tongue Language," emphasizing the importance of learning one's native language as a foundational skill. This concept is crucial in multilingual societies where maintaining cultural heritage and linguistic diversity is valued. In healthcare, MTL might be an abbreviation for "Medical Treatment List" or "Medication Treatment List," which healthcare providers use to keep track of a patient's medications, dosages, and treatment plans. This ensures accurate and consistent care, especially for patients with multiple health conditions. In sports and fitness, MTL could refer to "Maximum Tolerable Load," a term used in strength training to describe the highest weight or resistance an athlete can handle during an exercise. Understanding this limit is essential for safe and effective training. Lastly, in urban planning and transportation, MTL might stand for "Mass Transit Line," denoting a public transportation route that connects various parts of a city or region. This term is often used in discussions about urban mobility and infrastructure development. Each of these contexts illustrates how the acronym MTL is adapted to serve specific purposes, reflecting its adaptability and relevance across diverse fields. Understanding these different usages provides a comprehensive view of how terminology can evolve and be applied in various domains.
Historical Development of the Term
The term "MTL" has its roots in various contexts, but one of the most significant historical developments is associated with the concept of "Machine Translation" (MT). The acronym MTL, in this context, stands for "Machine Translation Learning," a subset of artificial intelligence and natural language processing. Historically, the idea of machine translation dates back to the 1940s and 1950s when the first computational models were proposed. However, it wasn't until the advent of neural networks in the 1980s that MT began to gain traction. The real breakthrough came with the introduction of deep learning techniques in the early 21st century. This period saw the development of sequence-to-sequence models and attention mechanisms, which significantly improved the accuracy and fluency of machine-translated text. In the early 2010s, researchers began exploring ways to train these models more efficiently and effectively. This led to the development of techniques such as encoder-decoder architectures and the use of large-scale datasets. The term MTL started gaining prominence as researchers recognized the importance of multi-task learning in improving the performance of machine translation systems. By training models on multiple related tasks simultaneously, such as translation, summarization, and language modeling, researchers could leverage shared knowledge across tasks to enhance overall performance. The rise of transformer models, particularly with the introduction of BERT (Bidirectional Encoder Representations from Transformers) in 2018, further accelerated advancements in MTL. These models allowed for more sophisticated handling of contextual information and enabled better generalization across different languages and tasks. Today, MTL is a cornerstone in many state-of-the-art machine translation systems, enabling more accurate and contextually aware translations. In summary, the historical development of MTL is closely tied to the evolution of machine translation technology. From its early beginnings in computational linguistics to the current era of deep learning and transformer models, MTL has emerged as a critical component in enhancing the capabilities of artificial intelligence to understand and translate human language effectively. This evolution underscores the continuous pursuit of improving machine translation through innovative learning strategies and architectures.
Applications of MTL in Various Fields
The applications of Multi-Task Learning (MTL) are diverse and far-reaching, transforming various fields with its innovative approach to simultaneous task learning. In machine learning and AI, MTL enhances model performance by leveraging shared representations across tasks, leading to more robust and generalizable models. This technique is particularly beneficial in scenarios where data is scarce or when tasks are interrelated. Additionally, MTL has significant implications in education and language learning, where it can help develop more comprehensive learning systems that integrate multiple skills and subjects, thereby improving student outcomes. In the realm of business and marketing strategies, MTL can optimize decision-making processes by considering multiple objectives and constraints, resulting in more effective and balanced strategies. By exploring these applications, we can better understand the versatility and power of MTL. Let's delve deeper into how MTL is revolutionizing machine learning and AI, where its impact is most pronounced.
MTL in Machine Learning and AI
**Applications of MTL in Various Fields** Multi-Task Learning (MTL) is a powerful paradigm in machine learning and AI that involves training a single model to perform multiple related tasks simultaneously. This approach leverages the shared representations and patterns across tasks to improve the overall performance and efficiency of the model. In various fields, MTL has proven to be highly effective due to its ability to generalize better and reduce overfitting. **Natural Language Processing (NLP):** In NLP, MTL is widely used for tasks such as sentiment analysis, named entity recognition, and machine translation. By training a model on multiple NLP tasks, it can learn a more robust and versatile language understanding. For instance, a model trained on both sentiment analysis and named entity recognition can better capture contextual nuances and improve its performance on each individual task. **Computer Vision:** In computer vision, MTL is applied to tasks like object detection, segmentation, and classification. Training a model to detect objects while also segmenting them can enhance its ability to understand the visual context. This is particularly useful in applications such as autonomous driving, where the model needs to perform multiple visual tasks accurately. **Healthcare:** In healthcare, MTL can be used to predict multiple clinical outcomes from patient data. For example, a model trained to predict both disease diagnosis and treatment outcomes can provide more comprehensive insights into patient care. This approach helps in leveraging the shared underlying patterns in medical data to improve predictive accuracy. **Finance:** In finance, MTL is used for tasks such as stock price prediction and risk analysis. By training a model on multiple financial metrics simultaneously, it can capture broader market trends and make more accurate predictions. This is crucial for portfolio management and risk assessment. **Robotics:** In robotics, MTL is applied to control and navigation tasks. A robot trained to perform multiple tasks like grasping, manipulation, and navigation can adapt more effectively to new environments. This enhances the robot's ability to generalize and perform well in various scenarios. Overall, MTL's versatility and ability to leverage shared knowledge across tasks make it a valuable tool in diverse fields, leading to more efficient and accurate models that can handle complex real-world problems.
MTL in Education and Language Learning
**MTL in Education and Language Learning** Machine Translation (MTL) has revolutionized the landscape of education and language learning by offering unprecedented opportunities for linguistic accessibility and comprehension. In educational settings, MTL tools facilitate the integration of diverse language groups, enabling students to engage with materials in their native languages. This enhances understanding and retention, particularly for non-native speakers who may struggle with complex texts in a second language. For instance, real-time translation software can translate educational content, such as textbooks, lectures, and assignments, making it easier for students to follow along and participate actively in class discussions. Moreover, MTL supports personalized learning experiences by providing immediate feedback on language usage. Language learning apps and platforms leverage MTL to offer interactive lessons where students can practice speaking, writing, and listening in a target language. These tools often include features like grammar correction, pronunciation feedback, and conversational practice with AI-powered chatbots. This not only accelerates the learning process but also makes it more engaging and accessible for learners of all levels. In addition, MTL bridges cultural gaps by facilitating communication between teachers and students from different linguistic backgrounds. For example, in multilingual classrooms, teachers can use translation tools to communicate effectively with students who may not speak the dominant language of instruction. This fosters a more inclusive learning environment where every student has an equal opportunity to participate and succeed. Furthermore, MTL aids in the development of language curricula by enabling educators to create and share resources across linguistic boundaries. Educational institutions can now easily adapt teaching materials to cater to diverse student populations, ensuring that all learners have access to high-quality educational content regardless of their native language. Overall, the integration of MTL in education and language learning has transformed the way we approach linguistic diversity, making education more inclusive, accessible, and effective for learners worldwide. By leveraging these technologies, educators can create a more equitable and engaging learning environment that supports the linguistic and academic growth of all students.
MTL in Business and Marketing Strategies
In the realm of business and marketing strategies, Machine Translation (MTL) has emerged as a transformative tool, enabling companies to expand their global reach and enhance customer engagement. By leveraging MTL, businesses can overcome language barriers, facilitating communication with diverse customer bases across different regions. For instance, multinational corporations can use MTL to translate marketing materials, product descriptions, and customer support content in real-time, ensuring consistency and accuracy. This not only improves the user experience but also helps in maintaining brand integrity by ensuring that the message is conveyed uniformly regardless of the language. MTL also plays a crucial role in market research and analysis. Companies can utilize machine translation to analyze customer feedback, reviews, and social media posts from various languages, providing valuable insights into consumer behavior and preferences. This data can be used to tailor marketing strategies more effectively, thereby increasing the likelihood of successful product launches and campaigns. Additionally, MTL can streamline the process of entering new markets by quickly translating legal documents, contracts, and regulatory compliance materials, reducing the time and cost associated with manual translation. From an operational standpoint, MTL enhances efficiency within organizations by automating routine translation tasks. This allows human translators to focus on more complex and nuanced content that requires cultural sensitivity and contextual understanding. Furthermore, integrating MTL into CRM systems and customer service platforms enables businesses to offer multilingual support, improving customer satisfaction and loyalty. In the digital age, where content is king, MTL helps businesses keep up with the demand for multilingual content. It facilitates the translation of blogs, articles, and social media posts in multiple languages, allowing companies to maintain a robust online presence that resonates with a global audience. This is particularly beneficial for e-commerce platforms looking to expand their customer base beyond geographical boundaries. Moreover, MTL supports data-driven decision-making by providing access to a broader spectrum of data from different languages. This comprehensive data set can be analyzed using advanced analytics tools to identify trends, patterns, and opportunities that might have been missed otherwise. By integrating MTL into their analytics frameworks, businesses can gain a more holistic view of their market dynamics. In conclusion, the application of MTL in business and marketing strategies is multifaceted and highly beneficial. It enhances global communication, improves market research capabilities, streamlines operations, supports multilingual customer service, and facilitates data-driven decision-making. As technology continues to evolve, the role of MTL will only become more pivotal in helping businesses navigate the complexities of a globalized market.
Benefits and Challenges of Implementing MTL
Implementing Multi-Task Learning (MTL) in machine learning models can be a transformative strategy, offering a myriad of benefits while also presenting several challenges. This article delves into the advantages, challenges, and real-world examples of MTL implementation. On one hand, MTL allows models to leverage shared representations and learn from multiple tasks simultaneously, enhancing overall performance and efficiency. This is explored in detail under the section "Advantages of Multi-Task Learning." However, the journey to successful MTL is not without its hurdles, as discussed in "Challenges in Implementing MTL Models," where issues such as task conflict and resource allocation are highlighted. Despite these challenges, numerous real-world applications have successfully harnessed the power of MTL, as illustrated in "Real-World Examples of Successful MTL Implementation." By understanding these facets, practitioners can better navigate the complexities and opportunities of MTL. Let's begin by examining the advantages that make MTL such a compelling approach in modern machine learning.
Advantages of Multi-Task Learning
**Advantages of Multi-Task Learning** Multi-task learning (MTL) offers several compelling advantages that make it a valuable approach in machine learning. One of the primary benefits is **improved generalization**. By training a model on multiple related tasks simultaneously, MTL encourages the model to learn more general and robust representations of the data. This shared knowledge across tasks can lead to better performance on each individual task compared to single-task learning, where models might overfit to specific task details. Another significant advantage is **reduced overfitting**. When a model is trained on a single task with limited data, it may memorize the training examples rather than learning meaningful patterns. MTL mitigates this by forcing the model to find commonalities and shared structures among different tasks, thereby reducing the risk of overfitting and improving model reliability. **Efficient use of resources** is another key benefit. Training multiple models for different tasks can be computationally expensive and resource-intensive. In contrast, MTL allows for the simultaneous training of a single model on multiple tasks, which can significantly reduce computational costs and memory requirements. This efficiency makes MTL particularly appealing in scenarios where resources are limited. Additionally, MTL can **enhance transfer learning** capabilities. The shared representations learned during multi-task training can be easily adapted to new tasks with minimal additional training data or fine-tuning. This adaptability is crucial in real-world applications where new tasks or datasets may become available over time. Moreover, MTL can **improve interpretability** by providing insights into how different tasks relate to each other. By analyzing the shared features and weights learned by the model across tasks, researchers can gain a deeper understanding of the underlying data structures and relationships that are not immediately apparent from single-task analyses. Finally, MTL can **accelerate the development cycle** for machine learning models. By leveraging the synergies between tasks, developers can more quickly identify effective architectures and hyperparameters that generalize well across multiple tasks, thereby speeding up the overall development process. In summary, multi-task learning offers a range of advantages including improved generalization, reduced overfitting, efficient use of resources, enhanced transfer learning capabilities, improved interpretability, and accelerated development cycles. These benefits make MTL an attractive strategy for many machine learning applications.
Challenges in Implementing MTL Models
Implementing Multi-Task Learning (MTL) models presents several challenges that can hinder their effectiveness and efficiency. One of the primary challenges is **task interference**, where the performance on one task may degrade due to the influence of other tasks. This can occur if the tasks are not sufficiently related or if the model is not designed to handle such interactions effectively. Another significant challenge is **task imbalance**, where some tasks may have significantly more data or importance than others, leading to biased learning and suboptimal performance across tasks. **Optimization difficulties** also arise in MTL models. Since multiple tasks are being learned simultaneously, the optimization process becomes more complex, and finding a common set of hyperparameters that work well for all tasks can be daunting. Additionally, **overfitting and underfitting** are more pronounced in MTL settings due to the increased complexity of the model and the need to balance learning across multiple objectives. **Data quality and availability** are critical factors. MTL models require high-quality data for all tasks, which can be challenging to obtain, especially if some tasks have limited or noisy data. Furthermore, **computational resources** can be a bottleneck; training MTL models often requires more computational power and memory compared to single-task models. **Model architecture design** is another challenge. Designing an architecture that can effectively share knowledge across tasks while maintaining task-specific features is a delicate balance. This often involves careful selection of shared layers, task-specific layers, and appropriate regularization techniques to prevent overfitting. Lastly, **evaluation metrics** pose a challenge. Since MTL models are trained on multiple tasks, evaluating their performance requires careful consideration of how to balance and combine metrics from different tasks. This can be particularly tricky when tasks have different performance metrics or when some tasks are more critical than others. Addressing these challenges requires careful planning, robust model design, and thorough experimentation to ensure that the benefits of MTL are fully realized. Despite these hurdles, the potential advantages of MTL in improving generalization and efficiency make it a valuable approach in many machine learning applications.
Real-World Examples of Successful MTL Implementation
In the realm of machine learning, Multi-Task Learning (MTL) has proven to be a powerful approach by leveraging shared representations across multiple tasks to improve performance. Several real-world examples highlight the successful implementation of MTL: 1. **Natural Language Processing (NLP):** Google's BERT model is a prime example of MTL in NLP. By training on a combination of tasks such as masked language modeling and next sentence prediction, BERT achieves state-of-the-art results in various downstream NLP tasks like sentiment analysis, question answering, and text classification. 2. **Computer Vision:** In the field of computer vision, MTL has been applied to tasks such as object detection, segmentation, and classification. For instance, the YOLO (You Only Look Once) model uses MTL to simultaneously detect objects and predict their bounding boxes, leading to faster and more accurate object detection. 3. **Speech Recognition:** Microsoft's speech recognition system employs MTL to improve the accuracy of speech-to-text models. By training on multiple tasks including phoneme recognition, word recognition, and language modeling, the system achieves better performance in noisy environments and with diverse accents. 4. **Healthcare:** In healthcare analytics, MTL is used to predict multiple clinical outcomes from patient data. For example, a model might predict both disease diagnosis and patient risk scores simultaneously, enhancing the overall predictive power and clinical decision-making. 5. **Autonomous Driving:** Companies like Waymo use MTL in their autonomous driving systems to handle multiple tasks such as lane detection, traffic light recognition, and pedestrian detection. This approach ensures that the system can handle complex driving scenarios more effectively. 6. **Recommendation Systems:** Online platforms like Netflix and Amazon use MTL to improve their recommendation algorithms. By training models on multiple tasks such as user behavior prediction, item categorization, and rating estimation, these platforms can provide more personalized recommendations. These examples illustrate how MTL can be effectively implemented across various domains to enhance model performance, reduce training time, and improve generalization capabilities. However, it is crucial to balance the benefits of MTL with its challenges, such as task interference and the need for careful task selection and weighting strategies.