Why Llmsmarcus Marcus
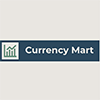
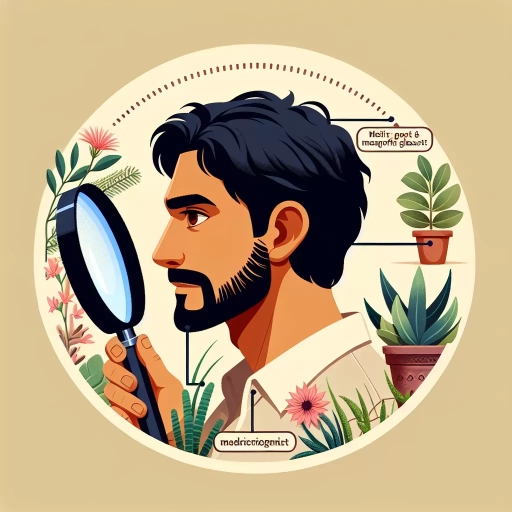
In the rapidly evolving landscape of artificial intelligence, Large Language Models (LLMs) have emerged as a transformative force, revolutionizing how we interact with technology and each other. These sophisticated models, capable of generating human-like text and understanding complex linguistic structures, are not just a novelty but a game-changer across various sectors. To fully appreciate the significance of LLMs, it is crucial to delve into their fundamental workings, which will be explored in the section "Understanding the Basics of LLMs." Beyond their technical underpinnings, LLMs are having a profound impact on multiple industries, from healthcare and education to finance and entertainment, as discussed in "The Impact of LLMs on Various Industries." Looking ahead, the future potential of these models is vast, but it also comes with significant challenges that need to be addressed, a topic that will be examined in "The Future Potential and Challenges of LLMs." By understanding these aspects, we can better navigate the exciting yet complex world of LLMs and their implications for our future. Let us begin by laying the groundwork with "Understanding the Basics of LLMs."
Understanding the Basics of LLMs
In the rapidly evolving landscape of artificial intelligence, Large Language Models (LLMs) have emerged as a cornerstone of modern technological advancements. These sophisticated models are capable of processing and generating human-like text, revolutionizing fields such as natural language processing, machine learning, and data analysis. To fully grasp the potential and implications of LLMs, it is essential to delve into their fundamental aspects. This article will provide a comprehensive overview by exploring three critical dimensions: **Definition and Functionality**, which will clarify what LLMs are and how they operate; **Historical Development**, tracing the evolution of these models from their inception to current state-of-the-art technologies; and **Key Components and Architecture**, detailing the intricate structures that enable their remarkable capabilities. By understanding these foundational elements, readers will gain a deeper insight into the mechanics and significance of LLMs, ultimately enhancing their appreciation for the transformative power of these models. This journey into the basics of LLMs will equip you with the knowledge necessary to navigate the complex and dynamic world of artificial intelligence, ensuring you are well-prepared to engage with the cutting-edge innovations that LLMs represent. Understanding the Basics of LLMs is crucial for anyone looking to stay ahead in this technological era.
Definition and Functionality
**Understanding the Basics of LLMs: Definition and Functionality** Large Language Models (LLMs) are sophisticated artificial intelligence systems designed to process, understand, and generate human-like language. At their core, LLMs are based on deep learning architectures, specifically transformer models, which enable them to capture complex patterns and relationships within vast amounts of text data. The definition of an LLM hinges on its ability to learn from extensive datasets and generalize this knowledge to perform a wide range of language-related tasks. Functionally, LLMs operate by leveraging massive computational resources to train on enormous datasets. This training involves optimizing the model's parameters to predict the next word in a sequence given the context of the preceding words. This predictive capability is what allows LLMs to generate coherent and contextually relevant text. The functionality extends beyond simple text generation; LLMs can be fine-tuned for various applications such as language translation, sentiment analysis, question answering, and even creative writing. One of the key functionalities of LLMs is their ability to understand and respond to natural language inputs. This is achieved through a process known as masked language modeling, where some words in the input text are randomly replaced with a special token, and the model is trained to predict these missing words. This approach enhances the model's comprehension and generation capabilities, making it adept at handling diverse linguistic tasks. Moreover, LLMs can be adapted for specific domains by fine-tuning them on domain-specific datasets. For instance, an LLM trained on medical texts can become proficient in generating medical summaries or answering clinical questions. This adaptability underscores the versatility of LLMs and their potential to revolutionize various industries by automating tasks that require advanced language understanding. In summary, the definition and functionality of LLMs are intertwined with their capacity for learning from extensive text data and applying this knowledge across a broad spectrum of language tasks. Their ability to generate coherent text, understand context, and adapt to different domains makes them powerful tools in the field of natural language processing. As technology continues to evolve, the role of LLMs in transforming how we interact with information and each other will only become more pronounced.
Historical Development
The historical development of Large Language Models (LLMs) is a fascinating narrative that underscores the rapid evolution of artificial intelligence and natural language processing. The journey began in the 1950s with the Dartmouth Summer Research Project on Artificial Intelligence, where the term "Artificial Intelligence" was coined, laying the groundwork for future advancements. In the 1960s, early AI programs like ELIZA, developed by Joseph Weizenbaum, could simulate human-like conversations, albeit in a very limited scope. However, these early experiments set the stage for more sophisticated language models. The 1980s saw significant strides with the introduction of statistical models for language processing. Researchers like Fred Jelinek and his team at IBM developed Hidden Markov Models (HMMs) that could recognize speech patterns, marking a crucial step towards understanding and generating human language. The 1990s brought about the rise of neural networks, particularly with the work of Yann LeCun, Yoshua Bengio, and Geoffrey Hinton, who laid the foundations for deep learning techniques that would later become pivotal in LLM development. In the early 2000s, the advent of Recurrent Neural Networks (RNNs) and Long Short-Term Memory (LSTM) networks enabled models to handle sequential data more effectively, leading to improved performance in tasks such as language translation and text generation. However, it was not until the introduction of transformer models in 2017 by Vaswani et al. that LLMs began to take their current form. The transformer architecture, with its self-attention mechanism, revolutionized the field by allowing models to process input sequences in parallel rather than sequentially, significantly enhancing efficiency and performance. Since then, LLMs have grown exponentially in size and capability. Models like BERT (Bidirectional Encoder Representations from Transformers), introduced by Google in 2018, demonstrated unprecedented success in various NLP tasks. This was followed by even larger models such as GPT-3 from OpenAI, which showcased remarkable abilities in generating coherent and contextually relevant text. Today, LLMs are at the forefront of AI research, with applications ranging from chatbots and virtual assistants to content generation and decision-making tools. Understanding the historical development of LLMs is crucial for appreciating their current capabilities and limitations. It highlights how continuous innovation and the integration of diverse technological advancements have led to the sophisticated language models we see today. This historical context also underscores the importance of ongoing research and development in pushing the boundaries of what is possible with AI, ultimately driving towards more intelligent, versatile, and beneficial applications of LLMs in various sectors.
Key Components and Architecture
Understanding the basics of Large Language Models (LLMs) hinges on grasping their key components and architecture. At the core of LLMs are **transformer models**, which revolutionized natural language processing by introducing self-attention mechanisms. This allows the model to weigh the importance of different words in a sentence relative to each other, enabling more nuanced and context-aware processing. The **encoder-decoder structure** is another crucial component. The encoder processes input text and generates a continuous representation, while the decoder uses this representation to generate output text. This setup is particularly effective for tasks like machine translation and text summarization. **Embeddings** play a vital role in converting raw text into numerical representations that the model can understand. These embeddings capture semantic relationships between words, allowing the model to generalize across different contexts. **Positional encoding** is also essential, as it helps the model understand the sequence and order of words in a sentence. The architecture of LLMs often includes multiple **layers of self-attention** and **feed-forward neural networks**. Each layer processes the input sequence in parallel, allowing for efficient computation and better handling of long-range dependencies. This multi-layered approach enables the model to capture complex patterns and relationships within the text. **Training data** is another key component, as LLMs are typically trained on vast amounts of text data. This training involves optimizing the model's parameters to predict the next word in a sequence, a process known as **masked language modeling**. The quality and diversity of the training data significantly impact the model's performance and ability to generalize. Finally, **fine-tuning** is a critical step in adapting pre-trained LLMs to specific tasks. By adjusting the model's parameters on task-specific data, fine-tuning allows LLMs to excel in various applications such as sentiment analysis, question answering, and text generation. In summary, the architecture of LLMs is built around transformer models, encoder-decoder structures, embeddings, positional encoding, and multi-layered processing. These components, combined with extensive training data and fine-tuning capabilities, make LLMs powerful tools for understanding and generating human language. Understanding these basics is essential for leveraging the full potential of LLMs in various applications.
The Impact of LLMs on Various Industries
The advent of Large Language Models (LLMs) has ushered in a transformative era across various industries, revolutionizing how businesses operate, interact with customers, and make informed decisions. These advanced AI technologies are not just tools but catalysts for innovation, driving significant changes in customer service, content creation, and data analysis. In the realm of customer service, LLMs are transforming the way companies engage with their clients, offering personalized and efficient support that enhances customer satisfaction. Meanwhile, in content creation, LLMs are revolutionizing the writing process by generating high-quality, engaging content at unprecedented speeds. Additionally, these models are enhancing data analysis and decision-making by providing insights that were previously unattainable, thereby empowering businesses to make more informed and strategic choices. As we delve into the impact of LLMs on these critical areas, it becomes clear that understanding their capabilities and limitations is essential. Therefore, this article will explore these transformative effects in detail, ultimately leading to a deeper understanding of the basics of LLMs and their potential to reshape the future of multiple industries.
Transforming Customer Service and Support
Transforming Customer Service and Support is a pivotal aspect of the broader impact of Large Language Models (LLMs) on various industries. The integration of LLMs into customer service frameworks has revolutionized the way businesses interact with their clients, enhancing both efficiency and satisfaction. At the forefront of this transformation are AI-powered chatbots and virtual assistants, which leverage LLMs to understand and respond to customer inquiries with unprecedented accuracy and speed. These AI-driven tools can handle a multitude of tasks, from answering frequently asked questions to resolving complex issues, thereby reducing the workload on human customer support agents and allowing them to focus on more critical and emotionally demanding cases. Moreover, LLMs enable personalized customer experiences through advanced sentiment analysis and context understanding. By analyzing customer interactions, these models can identify patterns and preferences, enabling businesses to tailor their responses and offers to individual needs. This personalization not only improves customer satisfaction but also fosters loyalty and trust. Additionally, LLMs facilitate multi-language support, breaking down language barriers and expanding a company's global reach without the need for extensive human translation resources. The predictive capabilities of LLMs also play a crucial role in proactive customer service. By analyzing historical data and real-time inputs, these models can anticipate potential issues and proactively offer solutions, often before the customer even realizes there is a problem. This proactive approach significantly reduces the number of complaints and escalations, leading to higher overall customer satisfaction. Another significant benefit of LLMs in customer service is their ability to provide 24/7 support without the constraints of human working hours. This round-the-clock availability ensures that customers receive immediate assistance regardless of their time zone or the time of day, enhancing their overall experience and reducing wait times. In conclusion, the impact of LLMs on customer service and support is multifaceted and transformative. By automating routine tasks, providing personalized interactions, facilitating multi-language support, enabling predictive maintenance, and offering continuous availability, LLMs are redefining the standards of customer service. As these technologies continue to evolve, they promise to further elevate the efficiency, effectiveness, and satisfaction of customer service operations across various industries.
Revolutionizing Content Creation and Writing
The advent of Large Language Models (LLMs) is revolutionizing content creation and writing across various industries, transforming the way we produce, consume, and interact with written content. These advanced AI models, such as those developed by OpenAI and Google, possess the capability to generate coherent, contextually relevant, and often engaging text. This technological leap has significant implications for industries ranging from media and publishing to marketing and education. In the media and publishing sector, LLMs are streamlining the content creation process by automating routine tasks such as data reporting, summarization, and even initial drafts of articles. Journalists can now focus on higher-level tasks like in-depth analysis and investigative reporting, enhancing the quality and depth of their work. For instance, AI-generated summaries can help readers quickly grasp key points from lengthy documents, while automated reporting tools can compile data-driven stories with unprecedented speed and accuracy. In marketing, LLMs are redefining how companies engage with their audiences. These models can generate personalized content at scale, from tailored email campaigns to social media posts that resonate with specific demographics. This personalization not only increases customer engagement but also enhances brand loyalty by making interactions feel more authentic and relevant. Additionally, LLMs can analyze vast amounts of customer feedback and sentiment data to provide actionable insights that guide marketing strategies. The educational sector is also benefiting from LLMs as they offer innovative tools for teaching and learning. AI-driven writing assistants can help students improve their writing skills by providing real-time feedback on grammar, syntax, and style. Moreover, LLMs can create customized educational materials that cater to individual learning needs, making education more accessible and effective. For educators, these models can assist in grading assignments and generating lesson plans, freeing up time for more hands-on teaching. Furthermore, LLMs are democratizing access to high-quality content creation. Small businesses and independent creators can now produce professional-grade content without the need for extensive resources or specialized teams. This democratization opens up new opportunities for entrepreneurship and creative expression, fostering a more diverse and vibrant digital landscape. However, it's important to note that while LLMs offer numerous benefits, they also raise ethical considerations. Issues such as authorship, copyright, and the potential for misinformation must be addressed as these technologies become more integrated into our workflows. Despite these challenges, the impact of LLMs on content creation and writing is undeniable, promising a future where AI and human collaboration elevate the quality, efficiency, and reach of written content across all sectors.
Enhancing Data Analysis and Decision-Making
Enhancing Data Analysis and Decision-Making is a pivotal aspect of how Large Language Models (LLMs) are transforming various industries. By leveraging advanced natural language processing capabilities, LLMs can significantly improve the efficiency and accuracy of data analysis, thereby bolstering decision-making processes. Here’s how this works: LLMs can rapidly process vast amounts of unstructured data, such as text documents, emails, and social media posts, to extract meaningful insights. This is particularly valuable in industries like finance, where financial reports and market analyses can be quickly summarized and analyzed to identify trends and potential risks. In healthcare, LLMs can help clinicians by synthesizing patient histories, medical literature, and clinical trial data to provide personalized treatment recommendations. Moreover, LLMs can automate routine data tasks, freeing up human analysts to focus on more complex and strategic work. For instance, in marketing, LLMs can analyze customer feedback from multiple sources to identify common themes and sentiment patterns, enabling companies to tailor their marketing strategies more effectively. The models can also generate reports and visualizations that are easy to understand, making it simpler for non-technical stakeholders to make informed decisions. Additionally, LLMs can predict future trends based on historical data, allowing businesses to anticipate market shifts and adjust their strategies accordingly. The integration of LLMs into data analysis workflows also enhances the speed at which insights are derived. Traditional data analysis often involves manual data cleaning, coding, and interpretation, which can be time-consuming. LLMs streamline this process by automatically identifying relevant data points, performing statistical analyses, and presenting findings in a clear and actionable manner. This accelerated decision-making cycle is crucial in competitive industries where timely responses to market changes can be the difference between success and failure. Furthermore, the interpretability of LLM-driven insights is improving with advancements in explainable AI. This means that decision-makers can not only rely on the recommendations provided by LLMs but also understand the underlying reasoning behind these suggestions. This transparency builds trust in AI-driven decision-making processes and ensures that decisions are well-informed and accountable. In summary, the impact of LLMs on enhancing data analysis and decision-making is profound. By automating routine tasks, providing deep insights from unstructured data, predicting future trends, and speeding up the decision-making process, LLMs are revolutionizing how industries operate. As these models continue to evolve, their role in driving strategic decisions will only become more pivotal, making them an indispensable tool for businesses aiming to stay ahead in their respective fields.
The Future Potential and Challenges of LLMs
The future of Large Language Models (LLMs) is poised to revolutionize various aspects of our lives, from enhancing productivity and innovation to transforming how we interact with technology. As these sophisticated AI systems continue to evolve, they promise significant advancements in AI technology, enabling more accurate and efficient processing of vast amounts of data. However, this progress is not without its challenges. Ethical considerations and bias mitigation will become increasingly critical as LLMs are integrated into more sensitive areas, such as healthcare and education, where fairness and transparency are paramount. Additionally, addressing security concerns and privacy issues will be essential to safeguard user data and prevent potential misuse. Understanding these future potential and challenges is crucial for harnessing the full benefits of LLMs while minimizing their risks. To fully appreciate these complexities, it is first important to understand the basics of LLMs, which will provide a foundational knowledge necessary for navigating the intricate landscape of their future applications.
Predicted Advancements in AI Technology
As we delve into the future potential and challenges of Large Language Models (LLMs), it is crucial to consider the predicted advancements in AI technology that will shape their evolution. Over the next decade, AI is expected to undergo significant transformations, driven by breakthroughs in deep learning, natural language processing, and cognitive architectures. One of the most anticipated advancements is the integration of multimodal learning capabilities, enabling LLMs to process and generate content across various media types such as text, images, audio, and video. This will enhance their ability to understand and interact with users in more intuitive and human-like ways, making them indispensable tools for applications ranging from customer service and content creation to healthcare and education. Another key area of advancement is the development of more transparent and explainable AI models. As LLMs become increasingly complex, there is a growing need for techniques that can provide insights into their decision-making processes. Explainable AI (XAI) will play a pivotal role in building trust and ensuring accountability in AI-driven systems, allowing developers and users to understand why certain outputs are generated. This transparency will be particularly important in high-stakes environments such as legal, financial, and medical fields where the reliability and fairness of AI outputs are paramount. Furthermore, advancements in edge AI and distributed computing will enable LLMs to operate more efficiently and securely. Edge AI allows for real-time processing at the point of data collection, reducing latency and enhancing privacy by minimizing the need for data transmission to centralized servers. This will be particularly beneficial for applications requiring immediate responses, such as autonomous vehicles and smart home devices. Additionally, the integration of cognitive architectures with LLMs is expected to enhance their reasoning and problem-solving capabilities. Cognitive architectures like SOAR and ACT-R will provide a framework for simulating human cognition, enabling LLMs to better understand context, make more informed decisions, and exhibit more human-like intelligence. Lastly, ethical considerations will drive significant advancements in AI governance and regulation. As LLMs become more pervasive, there will be a greater emphasis on ensuring they are developed and deployed responsibly. This includes addressing issues related to bias, privacy, and job displacement, as well as establishing clear guidelines for their use in various sectors. In summary, the future of LLMs is intertwined with broader advancements in AI technology. From multimodal learning and explainable AI to edge computing and cognitive architectures, these developments will not only enhance the capabilities of LLMs but also address critical challenges related to transparency, accountability, and ethical use. As we navigate this complex landscape, it is essential to balance the potential benefits of these technologies with the need for responsible innovation and governance.
Ethical Considerations and Bias Mitigation
As we delve into the future potential and challenges of Large Language Models (LLMs), it is imperative to address the ethical considerations and bias mitigation strategies that are crucial for their responsible development and deployment. Ethical considerations in LLMs revolve around issues such as data privacy, fairness, transparency, and accountability. These models are often trained on vast datasets that may contain personal information, raising significant concerns about data protection and user consent. Moreover, LLMs can perpetuate existing biases present in the training data, leading to discriminatory outcomes in applications like hiring, education, and healthcare. For instance, if a model is trained on biased historical data, it may generate responses that reflect and amplify these biases, potentially harming marginalized groups. To mitigate these biases, several strategies can be employed. First, ensuring diversity in the training datasets is essential; this involves actively seeking out diverse sources of data to reduce the risk of perpetuating existing societal biases. Second, implementing fairness metrics and regular audits can help identify and correct biases within the model's outputs. Techniques such as debiasing word embeddings and using adversarial training methods can also be effective in reducing bias. Additionally, transparency in model development is critical; developers should provide clear explanations of how the models work and what data they are trained on to foster trust and accountability. Another key aspect is human oversight and feedback mechanisms. Human evaluators can review model outputs to detect and correct biases, ensuring that the model aligns with ethical standards. Furthermore, involving diverse stakeholders in the development process can provide multiple perspectives on ethical issues, leading to more robust and fair models. Regulatory frameworks also play a vital role; policymakers must establish guidelines that mandate ethical AI development practices, including requirements for transparency, accountability, and fairness. In conclusion, while LLMs hold immense potential for transforming various sectors, their ethical implications cannot be overlooked. By prioritizing ethical considerations and actively working to mitigate biases through diverse datasets, fairness metrics, transparency, human oversight, and regulatory compliance, we can ensure that these powerful tools benefit society without causing harm. Addressing these challenges proactively will be essential for realizing the full potential of LLMs while maintaining public trust and ethical integrity.
Addressing Security Concerns and Privacy Issues
As we delve into the future potential and challenges of Large Language Models (LLMs), addressing security concerns and privacy issues becomes paramount. The sophisticated capabilities of LLMs, while revolutionary, also introduce significant vulnerabilities that must be mitigated to ensure their safe and ethical deployment. One of the primary security concerns is data leakage, where sensitive information inadvertently gets embedded into the model's outputs. This can occur through various means, such as memorization of training data or inference attacks that exploit patterns in the model's responses. To combat this, developers are implementing robust data anonymization techniques and differential privacy mechanisms to protect user data without compromising model performance. Another critical issue is the potential for malicious use, such as generating phishing emails, spreading misinformation, or even creating deepfakes. Here, the role of ethical AI governance comes into play. Establishing clear guidelines and regulations can help prevent the misuse of LLMs by ensuring that they are developed and deployed with built-in safeguards against harmful applications. Additionally, ongoing research in adversarial training and robustness testing aims to enhance the resilience of LLMs against attacks designed to manipulate or deceive them. Privacy issues are equally pressing, particularly given the vast amounts of personal data that LLMs process during training. Ensuring transparency in data collection and usage is crucial; this involves clear communication with users about what data is being collected, how it will be used, and providing them with control over their personal information. Furthermore, techniques like federated learning, which allow models to learn from decentralized data without requiring direct access to it, offer promising solutions for maintaining privacy while still leveraging the power of collective data. Incorporating human oversight and accountability into the development process is also essential. This includes regular audits to detect any biases or security flaws in the models and implementing mechanisms for users to report suspicious activities or inaccuracies. By fostering a collaborative environment between AI researchers, policymakers, and end-users, we can create a framework that balances the immense potential of LLMs with the need for stringent security and privacy measures. Ultimately, addressing these concerns is not just a technical challenge but also a societal one. It requires a multifaceted approach that involves technological innovation, ethical considerations, and regulatory frameworks. As we continue to push the boundaries of what LLMs can achieve, it is imperative that we do so with a keen eye on safeguarding both security and privacy to ensure that these powerful tools benefit society without compromising individual rights or safety. By doing so, we can unlock the full potential of LLMs while maintaining trust and confidence in their use.