Why Does My Data Not Work
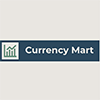
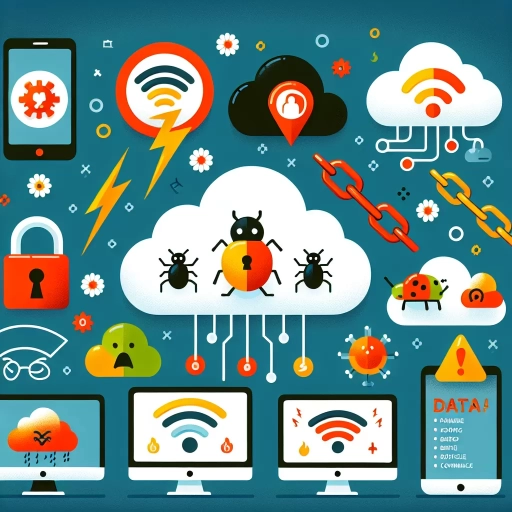
In today's data-driven world, the integrity and functionality of data are crucial for making informed decisions, driving business operations, and ensuring seamless user experiences. However, many individuals and organizations face the frustrating issue of data that simply does not work as intended. This problem can stem from various factors, each contributing to the overall dysfunction. To address this, it is essential to delve into three key areas: understanding data quality issues, technical factors affecting data functionality, and human and operational factors impacting data. By examining these aspects, we can gain a comprehensive understanding of why data may not be performing as expected. Starting with the foundational element, **Understanding Data Quality Issues** will provide insights into how inaccuracies, inconsistencies, and incompleteness can undermine the reliability of your data, setting the stage for a deeper exploration of the technical and human factors that further complicate this issue.
Understanding Data Quality Issues
In today's data-driven world, the integrity and reliability of data are paramount for making informed decisions and driving business success. However, data quality issues can significantly undermine these efforts. Understanding data quality issues is crucial for organizations to ensure their data sets are accurate, complete, and up-to-date. Three key areas where data quality often falters are data inconsistencies and errors, incomplete or missing data, and outdated data. Data inconsistencies and errors can arise from various sources, including human input mistakes or system malfunctions, leading to misleading insights. Incomplete or missing data can hinder comprehensive analysis, while outdated data fails to reflect current trends and realities. Addressing these issues is essential to maintain trust in data and derive meaningful conclusions. By delving into these specific challenges, we can better comprehend the broader landscape of data quality issues and develop strategies to mitigate them, ultimately enhancing the reliability and utility of our data. Understanding data quality issues is not just a technical necessity but a strategic imperative for any organization seeking to leverage data effectively.
Data Inconsistencies and Errors
Data inconsistencies and errors are critical issues that can significantly undermine the reliability and usability of data, making it challenging to derive accurate insights or make informed decisions. These problems arise from various sources, including human input errors, system glitches, data integration mishaps, and outdated information. For instance, typos or incorrect formatting during data entry can lead to inconsistencies in records, while system failures or bugs can result in missing or corrupted data. When different datasets are merged, discrepancies in formatting or coding schemes can also introduce errors. Furthermore, if data is not regularly updated, it may become outdated and no longer reflect current realities. Understanding these issues is crucial because they can have far-reaching consequences. Inconsistent data can lead to flawed analytics and reporting, which in turn can result in poor decision-making. For example, in healthcare, incorrect patient records could lead to inappropriate treatments, while in finance, erroneous transaction data could result in financial losses or compliance issues. Moreover, data inconsistencies can erode trust in the data itself and the systems that manage it, making stakeholders hesitant to rely on the information. To mitigate these problems, it is essential to implement robust data quality checks and validation processes. This includes using automated tools to detect and correct errors at the point of entry, as well as conducting regular audits to identify inconsistencies. Standardizing data formats and establishing clear guidelines for data entry can also help minimize errors. Additionally, leveraging advanced technologies such as machine learning and artificial intelligence can aid in identifying patterns and anomalies that may indicate data inconsistencies. Addressing data inconsistencies requires a proactive approach rather than a reactive one. By integrating data quality into the overall data management strategy from the outset, organizations can ensure that their data remains accurate, reliable, and consistent over time. This not only enhances the credibility of the data but also supports better decision-making processes across various domains. In summary, recognizing and addressing data inconsistencies and errors is vital for maintaining high-quality data that can be trusted and utilized effectively. By understanding these issues and implementing appropriate measures, organizations can safeguard their data integrity and derive meaningful insights from their datasets.
Incomplete or Missing Data
Incomplete or missing data is a pervasive issue that can significantly undermine the integrity and reliability of any dataset, thereby impacting the accuracy and validity of analyses, models, and decisions derived from it. This problem arises when some data points are either partially recorded or entirely absent, which can occur due to various reasons such as human error during data entry, technical failures in data collection systems, or even intentional omission. For instance, in a customer database, missing fields like email addresses or phone numbers can hinder effective communication and marketing efforts. Similarly, in healthcare datasets, incomplete medical histories can lead to inaccurate diagnoses and treatment plans. The consequences of incomplete or missing data are multifaceted. Firstly, it can introduce bias into statistical analyses and machine learning models, leading to skewed results that do not accurately reflect the true population characteristics. This bias can manifest in various forms, such as selection bias if certain groups are more likely to have missing data or information bias if the missing data is not randomly distributed. Secondly, missing data can reduce the power of statistical tests and the precision of estimates, making it more challenging to draw reliable conclusions. Furthermore, incomplete datasets may necessitate additional steps like imputation or interpolation, which themselves introduce assumptions and potential errors. To mitigate these issues, several strategies can be employed. One common approach is data imputation, where missing values are replaced with estimated values based on other available data points. Techniques such as mean imputation, regression imputation, and multiple imputation by chained equations (MICE) are widely used. Another strategy involves using robust statistical methods that are less sensitive to missing data, such as listwise deletion or pairwise deletion. Additionally, proactive measures during data collection can help minimize the occurrence of missing data; these include implementing robust validation checks in data entry systems and ensuring that data collection protocols are rigorous and well-documented. Understanding the nature and extent of missing data is crucial for developing effective mitigation strategies. This involves conducting thorough exploratory data analysis to identify patterns and distributions of missing values. Tools like heatmaps and summary statistics can help visualize and quantify the extent of missingness. Moreover, documenting the reasons behind missing data whenever possible can provide valuable context for subsequent analyses. In conclusion, addressing incomplete or missing data is essential for ensuring high-quality datasets that support reliable decision-making processes. By recognizing the sources and impacts of this issue and employing appropriate strategies for handling missing values, organizations can enhance the accuracy and reliability of their data-driven insights. This proactive approach not only improves data quality but also fosters trust in the analytical outcomes, ultimately contributing to better decision-making across various domains.
Outdated Data
Outdated data is a pervasive issue that can significantly undermine the reliability and effectiveness of any dataset, leading to inaccurate insights and poor decision-making. When data becomes outdated, it fails to reflect current trends, changes in market conditions, or evolving user behaviors. This can happen due to various reasons such as infrequent updates, lack of real-time data collection mechanisms, or delays in data processing. For instance, in the context of customer relationship management (CRM), outdated contact information can lead to failed communication attempts and missed opportunities. Similarly, in financial analysis, using historical data that does not account for recent economic shifts can result in flawed forecasts and misguided investment strategies. The consequences of relying on outdated data are multifaceted. It can lead to misinformed strategic decisions, which in turn may result in financial losses or reputational damage. In healthcare, outdated patient records can compromise patient safety by failing to include recent medical history or changes in treatment plans. In marketing, outdated consumer preferences may cause campaigns to miss their target audience entirely, resulting in wasted resources and diminished return on investment. Moreover, outdated data can also perpetuate biases and inaccuracies that are embedded within the dataset. For example, if a dataset reflects demographic trends from several years ago, it may not capture the current diversity of a population, leading to skewed analyses and unfair outcomes. This is particularly problematic in fields like AI and machine learning where models trained on outdated data may perpetuate historical biases and discrimination. To mitigate these issues, it is crucial to implement robust data refresh mechanisms. This includes setting up regular update schedules, leveraging real-time data streams where possible, and ensuring that data sources are reliable and current. Additionally, organizations should invest in data validation processes to ensure that the information they are using is accurate and up-to-date. By prioritizing data freshness and integrity, organizations can enhance the quality of their datasets, thereby improving the accuracy of their insights and the effectiveness of their decision-making processes. In summary, outdated data poses significant risks across various sectors by providing a distorted view of reality. Recognizing these risks and taking proactive steps to maintain current and accurate datasets is essential for ensuring data quality and driving informed decision-making. By addressing this critical issue, organizations can avoid the pitfalls associated with outdated information and leverage their data assets more effectively to achieve their goals.
Technical Factors Affecting Data Functionality
In today's data-driven world, the functionality of data is crucial for making informed decisions and driving business success. However, several technical factors can significantly impact data functionality, leading to inefficiencies and inaccuracies. This article delves into three critical areas that affect data performance: compatibility problems with software or systems, network and connectivity issues, and hardware limitations or failures. Each of these factors can disrupt the seamless flow and utilization of data, compromising its integrity and usability. Understanding these technical challenges is essential for identifying and mitigating data quality issues. By exploring these key areas, we can better appreciate the complexities involved in maintaining high-quality data and take proactive steps to ensure that our data systems operate optimally. This insight will ultimately contribute to a deeper understanding of data quality issues, enabling organizations to implement robust solutions that enhance data reliability and functionality.
Compatibility Problems with Software or Systems
Compatibility problems with software or systems are a significant technical factor that can severely impact data functionality. When different software applications or systems are not designed to work seamlessly together, they can lead to a myriad of issues that hinder the effective use and analysis of data. For instance, if a database management system is incompatible with the analytics software, it may result in errors during data import, processing, or visualization. This incompatibility can stem from various sources such as differences in data formats, communication protocols, or even the operating systems on which these applications run. One common scenario is when legacy systems are integrated with modern software tools. Legacy systems often use outdated technologies and data formats that are not supported by newer applications, leading to compatibility issues. For example, an older database might store data in a proprietary format that is not recognized by contemporary analytics tools, necessitating cumbersome data conversion processes that can introduce errors and delays. Moreover, compatibility issues can arise due to differences in software versions. When different components of a system are updated at different times, it can create version mismatches that disrupt functionality. For instance, if a web application is updated to a new version but its backend services remain on an older version, this mismatch can cause communication failures between the frontend and backend layers. Additionally, platform-specific compatibility problems can occur when software is developed for one operating system but needs to be used on another. Applications optimized for Windows may not function correctly on macOS or Linux without significant modifications, which can complicate cross-platform data sharing and collaboration. To mitigate these compatibility problems, organizations often employ various strategies such as using standardized data formats like JSON or XML, implementing middleware solutions to bridge the gap between different systems, and conducting thorough testing before deploying new software or system updates. Furthermore, adopting cloud-based services that offer robust integration capabilities can help ensure smoother interactions between disparate applications. In conclusion, addressing compatibility issues is crucial for maintaining robust data functionality. By understanding the potential pitfalls and implementing appropriate solutions, organizations can ensure that their software and systems work harmoniously together to support efficient data processing and analysis. This proactive approach not only enhances operational efficiency but also minimizes downtime and errors associated with incompatibilities, ultimately leading to better decision-making and business outcomes.