Why Is Chatgpt So Slow
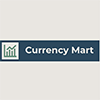
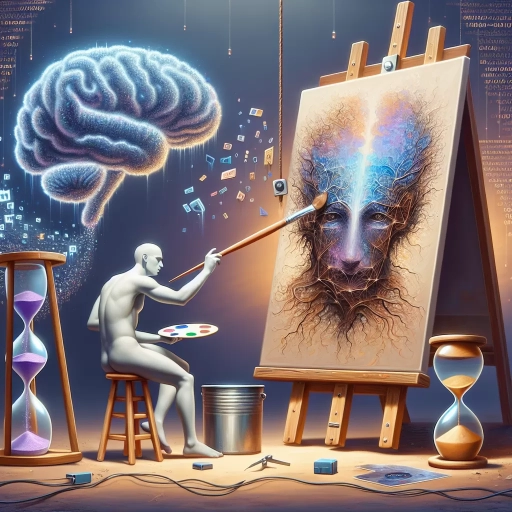
In the era of rapid technological advancements, the emergence of AI-powered chatbots like ChatGPT has revolutionized how we interact with machines. However, despite its groundbreaking capabilities, many users have noticed that ChatGPT can be slow in responding to queries. This lag is not merely a minor inconvenience but a symptom of deeper complexities. To understand why ChatGPT often struggles with speed, it is essential to delve into three critical factors: the inherent complexity of natural language processing, the limitations of infrastructure and resources, and the intricacies of user interaction and query complexity. Each of these elements plays a significant role in determining the performance of AI chatbots. Starting with the foundational issue, the complexity of natural language processing is a significant hurdle that AI models must overcome. This involves deciphering nuances, context, and ambiguities in human language, which is inherently more challenging than processing straightforward data. By examining this first layer of complexity, we can begin to unravel the multifaceted reasons behind ChatGPT's slower response times. --- **Complexity of Natural Language Processing** The core challenge in developing AI chatbots like ChatGPT lies in the intricate nature of natural language processing (NLP). Unlike structured data, human language is replete with ambiguities, idioms, and context-dependent expressions. For instance, words can have multiple meanings, and sentences can be interpreted in various ways depending on the context. This complexity necessitates sophisticated algorithms and extensive training datasets to ensure that the AI model can accurately understand and respond to user queries. The processing time increases as the model navigates through layers of linguistic analysis, semantic interpretation, and syntactic parsing. This foundational complexity sets the stage for understanding why ChatGPT might be slow, even before considering other factors. **Infrastructure and Resource Limitations** Beyond the inherent challenges of NLP, the infrastructure and resource constraints also significantly impact ChatGPT's performance. The computational power required to process complex queries in real-time is substantial. High-performance servers, advanced GPUs, and significant memory resources are necessary to handle the volume of user interactions efficiently. However, these resources are not always available or evenly distributed, leading to bottlenecks and delays. Additionally, network latency and data transmission speeds can further exacerbate response times. As demand for AI chatbots increases, the strain on existing infrastructure becomes more pronounced, contributing to slower responses. **User Interaction and Query Complexity** The nature of user interactions and the complexity of queries themselves also play a crucial role in determining ChatGPT's response speed. Users often pose open-ended questions or engage in multi-turn conversations, which require the AI to maintain context and adapt its responses accordingly. These dynamic interactions demand more processing power and time compared to simple, one-off queries. Moreover, queries that involve specialized knowledge or require extensive reasoning can slow down the system as it searches through vast amounts of data to provide accurate answers. The variability in user queries adds another layer of complexity, making it challenging for ChatGPT to consistently deliver rapid responses. By understanding these interrelated factors—complexity of NLP, infrastructure limitations, and user interaction dynamics—we can better appreciate why ChatGPT sometimes lags behind our expectations for speed. Each element contributes to the overall performance of the AI chatbot, highlighting the need for ongoing improvements in technology, infrastructure, and user interface design.
Complexity of Natural Language Processing
Natural Language Processing (NLP) is a multifaceted field that delves into the intricate dynamics of human language, aiming to enable machines to understand, interpret, and generate human-like text. At its core, NLP involves several key challenges that underscore its complexity. One of the primary hurdles is the development of **Deep Learning Models**, which are crucial for processing vast amounts of linguistic data but require sophisticated architectures to capture nuanced patterns. Another critical aspect is **Contextual Understanding**, as NLP systems must be able to grasp the subtleties of context to accurately interpret meaning. Additionally, **Linguistic Variability** poses a significant challenge, as languages are inherently diverse and constantly evolving, making it difficult for algorithms to generalize across different dialects and styles. These complexities collectively highlight the profound challenges inherent in NLP, making it a rich and demanding area of research. As we explore these facets in depth, it becomes clear that the true essence of NLP lies in navigating and overcoming these intricacies, ultimately revealing the **Complexity of Natural Language Processing**.
1. Deep Learning Models
Deep learning models are a cornerstone of modern natural language processing (NLP), but their complexity and computational demands are significant contributors to the latency experienced by users of advanced AI tools like ChatGPT. These models, particularly those based on transformer architectures such as GPT-3 and GPT-4, are designed to capture intricate patterns and relationships within vast amounts of text data. The architecture of these models involves multiple layers of self-attention mechanisms, which allow the model to weigh the importance of different words in a sentence relative to each other. This capability enables the model to understand context and generate coherent, contextually relevant responses. However, this sophistication comes at a cost. Each layer in a deep learning model requires substantial computational resources to process input data. For instance, the GPT-3 model contains over 175 billion parameters, making it one of the largest language models ever trained. Training such models involves massive datasets and extensive computational power, often necessitating distributed computing environments and significant energy consumption. When these models are deployed in real-time applications like chatbots, the computational overhead translates into slower response times. The sheer number of calculations required to generate a response means that even with high-performance hardware, there is an inherent delay between user input and the model's output. Moreover, the complexity of deep learning models extends beyond their architecture to their training processes. Fine-tuning these models on specific tasks or datasets further adds to their computational complexity. This fine-tuning process involves adjusting the model's parameters to better fit the nuances of a particular domain or task, which can be time-consuming and resource-intensive. Additionally, ensuring that these models generalize well across different contexts and avoid overfitting requires careful tuning of hyperparameters and regularization techniques, adding another layer of complexity. In summary, while deep learning models are instrumental in achieving state-of-the-art results in NLP tasks, their inherent complexity and computational requirements are key factors contributing to the latency observed in applications like ChatGPT. The trade-off between model performance and response time is a critical consideration for developers seeking to balance user experience with the sophistication of AI-driven interactions. As research continues to advance in this field, finding ways to optimize these models for faster inference without compromising their performance will be crucial for enhancing user satisfaction and real-time engagement.
2. Contextual Understanding
Contextual understanding is a pivotal aspect of Natural Language Processing (NLP) that significantly contributes to the complexity and challenges faced by advanced AI models like ChatGPT. At its core, contextual understanding involves the ability of a model to comprehend the nuances and subtleties of language within a specific context, taking into account the relationships between words, phrases, and sentences. This requires more than just processing individual words; it demands an intricate grasp of how these elements interact to convey meaning. In practice, achieving robust contextual understanding involves several layers of complexity. First, the model must be able to recognize and interpret various linguistic structures such as syntax, semantics, and pragmatics. Syntax deals with the rules governing how words are combined to form sentences, while semantics focuses on the meaning of these sentences. Pragmatics goes a step further by considering the context in which communication occurs, including non-verbal cues and implied meanings. For instance, understanding sarcasm or idioms requires not just recognizing the literal meaning of words but also grasping the underlying intent and cultural context. Moreover, contextual understanding is deeply intertwined with world knowledge and common sense. A model needs to have a broad base of knowledge about the world to make informed interpretations of text. This includes understanding temporal relationships, spatial awareness, and social norms. For example, when a user asks ChatGPT about a historical event, the model must draw upon its vast repository of knowledge to provide accurate and contextually relevant information. The computational demands of achieving such nuanced understanding are substantial. Advanced models like ChatGPT rely on large-scale neural networks trained on vast amounts of data to learn these complex patterns. However, even with significant computational resources, there are limitations to how well a model can capture the full richness of human language. Human communication often involves ambiguity, ambiguity resolution, and context-dependent expressions that can be challenging for AI systems to fully grasp. Additionally, the dynamic nature of language adds another layer of complexity. Language evolves over time with new words, expressions, and cultural references emerging continuously. This means that models must be constantly updated and fine-tuned to stay relevant and accurate. The slow response times of models like ChatGPT can be attributed in part to the extensive processing required to handle these complexities effectively. In summary, the quest for contextual understanding in NLP is a multifaceted challenge that underpins much of the complexity and slowness associated with advanced AI models like ChatGPT. By delving into the intricacies of linguistic structures, world knowledge, and dynamic language evolution, we gain a deeper appreciation for why these models require substantial computational resources and time to deliver accurate and contextually appropriate responses.
3. Linguistic Variability
Linguistic variability is a fundamental aspect of natural language processing (NLP) that significantly contributes to the complexity of tasks like those performed by ChatGPT. This variability manifests in several dimensions, each posing unique challenges for AI models. Firstly, **lexical variability** refers to the diverse ways words can be used and interpreted. Words often have multiple meanings (polysemy), and their meanings can shift based on context (contextual semantics). For instance, the word "bank" can refer to a financial institution or the side of a river, requiring the model to understand the surrounding text to disambiguate its meaning. Secondly, **syntactic variability** involves the different ways sentences can be structured. Language allows for a wide range of grammatical constructions, including variations in word order, clause structure, and use of passive voice versus active voice. This variability makes it difficult for models to predict and generate coherent sentences consistently. Thirdly, **pragmatic variability** deals with how language is used in context to convey meaning beyond the literal interpretation of words. This includes understanding idioms, metaphors, sarcasm, and other forms of figurative language that rely on shared cultural knowledge and situational awareness. For example, saying "It's raining cats and dogs" does not literally mean animals are falling from the sky but rather indicates heavy rain. Lastly, **dialectal and regional variability** adds another layer of complexity as different regions and communities use distinct dialects with unique vocabularies, pronunciations, and grammatical rules. These variations can make it challenging for models like ChatGPT to understand and respond accurately across diverse user bases. The cumulative effect of these variabilities is that NLP models must be incredibly robust and adaptable to handle the vast array of linguistic expressions they encounter. This requires extensive training on diverse datasets to capture the nuances of language use across different contexts and populations. However, even with large datasets, the inherent variability in language means that models will occasionally struggle to understand or generate text accurately. This struggle is one reason why ChatGPT might seem slow or less responsive at times; it is constantly processing and adapting to the complex landscape of human communication. In essence, linguistic variability underscores the intricate nature of natural language and highlights why developing sophisticated NLP models remains an ongoing challenge in the field of artificial intelligence.
Infrastructure and Resource Limitations
In today's digital age, the robustness and efficiency of infrastructure are crucial for supporting various technological applications, including those involving complex tasks like Natural Language Processing (NLP). However, several key limitations can hinder the performance and scalability of these systems. This article delves into three critical areas where infrastructure and resource constraints often emerge: server capacity and load, network latency, and data storage and retrieval. Understanding these limitations is essential for optimizing system performance and ensuring that applications can handle demanding tasks without compromising speed or accuracy. Server capacity and load issues can lead to bottlenecks that slow down processing times, while network latency can significantly delay data transmission and response times. Additionally, the challenges associated with data storage and retrieval can impact how quickly and efficiently information is accessed and processed. These infrastructure limitations have a direct impact on the complexity of NLP tasks, which require substantial computational resources and rapid data access to function effectively. By examining these constraints, we can better appreciate the intricacies involved in maintaining high-performance systems and the importance of addressing these challenges to support advanced applications like NLP.
1. Server Capacity and Load
Server capacity and load are critical factors that significantly impact the performance and responsiveness of AI models like ChatGPT. When discussing infrastructure and resource limitations, it is essential to understand how server capacity influences the user experience. Server capacity refers to the maximum amount of computational resources, such as CPU power, memory, and bandwidth, that a server can handle at any given time. When these resources are fully utilized, the server is said to be at maximum load. In the context of ChatGPT, high server load can lead to slower response times and delayed interactions. This occurs because each user query requires significant computational power to process natural language inputs, generate responses, and manage context within conversations. If multiple users are querying the system simultaneously, it can quickly overwhelm the available server resources. As a result, users may experience delays or even timeouts as the system struggles to keep up with demand. Moreover, server capacity is not just about raw processing power but also includes other critical components such as storage, network connectivity, and database access. For instance, if a server's storage is nearing its limit or if network latency is high due to poor connectivity, these bottlenecks can further exacerbate load issues. Additionally, efficient resource allocation and load balancing strategies are crucial in managing server capacity effectively. Load balancing involves distributing incoming traffic across multiple servers to ensure no single server is overwhelmed while resource allocation ensures that each server has sufficient resources to handle its share of the workload. To mitigate these issues, organizations often employ various strategies such as scaling up (increasing the power of individual servers) or scaling out (adding more servers to distribute the load). However, these solutions come with their own set of challenges including increased costs for hardware and maintenance as well as complexities in managing distributed systems. Furthermore, optimizing server performance through software enhancements like better algorithms or caching mechanisms can also help in managing load more efficiently. In summary, server capacity and load are pivotal in determining the performance of AI-driven applications like ChatGPT. Understanding these limitations is crucial for optimizing infrastructure to ensure seamless user experiences even during peak usage times. By addressing these challenges through strategic resource management and technological advancements, organizations can better handle high loads and provide faster response times for their users.
2. Network Latency
Network latency is a critical factor contributing to the perceived slowness of AI models like ChatGPT, particularly when these models are accessed through cloud services. Latency refers to the delay between the time data is sent and the time it is received, which can significantly impact user experience. In the context of infrastructure and resource limitations, network latency arises from several key sources. Firstly, geographical distance between the user and the data center hosting the AI model can introduce substantial delays. Signals have to travel longer distances, which inherently increases the round-trip time (RTT) for data transmission. This issue is exacerbated in regions with limited or underdeveloped internet infrastructure, where slower network speeds and higher packet loss rates further degrade performance. Secondly, network congestion plays a significant role. When multiple users are accessing the same resources simultaneously, it can lead to bottlenecks in data transmission. This congestion not only slows down individual requests but also increases the overall latency experienced by users. Additionally, the complexity of modern AI models like ChatGPT, which require significant computational resources and data exchanges, compounds these issues. Each request involves multiple back-and-forth communications between the client and server, each contributing to the cumulative latency. Furthermore, the architecture of cloud services themselves can introduce latency. Load balancing, content delivery networks (CDNs), and other optimizations aimed at improving performance can sometimes add layers of complexity that inadvertently increase latency. For instance, if a CDN is not optimally configured or if load balancing algorithms are not efficient, they can introduce additional delays in routing requests to available servers. To mitigate these issues, developers and service providers employ various strategies such as edge computing, where AI models are deployed closer to users to reduce geographical latency; optimizing network protocols to minimize packet loss and retransmissions; and leveraging advanced caching mechanisms to reduce the number of requests that need to be processed by the central servers. However, these solutions are not foolproof and come with their own set of challenges and limitations, highlighting the ongoing struggle to balance performance with resource constraints in delivering fast and reliable AI services like ChatGPT. Ultimately, understanding and addressing network latency is crucial for enhancing user experience and ensuring that AI models can operate at their full potential despite infrastructure and resource limitations.
3. Data Storage and Retrieval
Data storage and retrieval play a crucial role in the performance and efficiency of AI models like ChatGPT, significantly impacting their speed and responsiveness. When discussing infrastructure and resource limitations, it is essential to delve into how data storage and retrieval mechanisms affect these systems. ChatGPT, powered by OpenAI's GPT-4 architecture, relies on vast amounts of data to generate responses. This data is stored in complex databases that must be accessed quickly to maintain user experience. However, several challenges arise here. Firstly, the sheer volume of data required for training and operation is enormous, necessitating robust storage solutions that can handle petabytes of information. Traditional storage systems often struggle with such scale, leading to bottlenecks in data retrieval times. Moreover, the architecture of these databases must be optimized for rapid access. This involves sophisticated indexing and caching mechanisms to ensure that the model can retrieve relevant information swiftly. Despite these optimizations, latency can still occur due to network congestion or the physical limitations of storage devices. For instance, even with high-speed SSDs (Solid-State Drives), accessing specific pieces of data from a vast dataset can take milliseconds, which may seem negligible but can add up significantly in real-time applications. Another critical aspect is the distribution of data across multiple servers or nodes in a cloud environment. While this allows for better scalability and redundancy, it introduces additional complexities such as data synchronization and consistency issues. Ensuring that all nodes have the most up-to-date information without compromising performance is a significant challenge. This distributed architecture also increases the likelihood of network latency, further slowing down the overall response time. Furthermore, security measures and compliance requirements add another layer of complexity to data storage and retrieval. Encrypting data at rest and in transit, along with implementing access controls and auditing mechanisms, are essential but can introduce additional overhead that slows down operations. In summary, the efficiency of ChatGPT is heavily influenced by its data storage and retrieval systems. The need for massive storage capacity, optimized database architectures, distributed systems management, and stringent security protocols all contribute to potential slowdowns. Addressing these challenges through innovative storage solutions, advanced caching techniques, and optimized network configurations is crucial for enhancing the speed and responsiveness of AI models like ChatGPT. By understanding these intricacies, developers can better design infrastructure that mitigates resource limitations and improves user experience.
User Interaction and Query Complexity
In the realm of user interaction, the complexity of queries poses a significant challenge for system designers and developers. As users increasingly rely on digital interfaces to fulfill their needs, the intricacies of their interactions have become a focal point for enhancing user experience. This article delves into three critical aspects that define the landscape of user interaction: the length and complexity of queries, user input variability, and real-time processing demands. Understanding these elements is crucial for developing systems that can effectively handle diverse and often complex user inputs. The length and complexity of queries highlight the need for sophisticated algorithms that can parse and interpret lengthy, nuanced requests. User input variability underscores the importance of flexibility in handling diverse types of inputs, from simple commands to elaborate queries. Finally, real-time processing demands emphasize the necessity for systems to respond swiftly and accurately, even under high loads. These factors collectively contribute to the broader challenge of natural language processing complexity, where the goal is to create systems that can seamlessly interpret and respond to human language in all its forms. By exploring these dimensions, we can better navigate the complexities inherent in user interaction and move closer to achieving seamless human-computer communication.
1. Length and Complexity of Queries
The length and complexity of queries significantly impact the performance and response time of AI models like ChatGPT. When users interact with these systems, the nature of their queries can vary widely, from simple, straightforward questions to intricate, multi-layered inquiries. Simple queries, such as asking for definitions or basic information, typically require minimal processing power and can be answered swiftly. However, as queries become more complex—encompassing multiple sub-questions, requiring contextual understanding, or involving nuanced reasoning—the computational demands increase substantially. Complex queries often necessitate deeper semantic analysis, broader knowledge retrieval, and more sophisticated reasoning mechanisms, all of which consume more time and resources. For instance, a user might ask a straightforward question like "What is the capital of France?" which can be answered almost instantaneously. In contrast, a more complex query such as "Can you explain the historical context behind the French Revolution and its impact on modern European politics?" requires the model to delve into extensive historical data, understand causal relationships, and synthesize information into a coherent narrative. This increased complexity not only prolongs the response time but also places greater strain on the underlying infrastructure, including servers and algorithms. Moreover, the length of queries also plays a crucial role. Longer queries often contain more context or additional details that need to be processed, which can slow down the response. For example, if a user provides a lengthy backstory or multiple examples to support their question, the model must parse this additional information to provide an accurate and relevant response. This added processing time can make the interaction feel slower to the user. Furthermore, the complexity of queries can sometimes lead to iterative interactions where users refine their questions based on initial responses. This back-and-forth process, while beneficial for achieving accurate results, further extends the overall interaction time. As AI models continue to evolve and improve, managing query complexity and length will remain a critical challenge in ensuring efficient and satisfying user interactions. In summary, the length and complexity of queries are key factors influencing the speed and efficiency of AI models like ChatGPT. Understanding these dynamics is essential for optimizing system performance and enhancing user experience, especially as these technologies become increasingly integral to our daily lives. By acknowledging and addressing these challenges, developers can work towards creating more responsive and effective AI systems that meet the diverse needs of users.
2. User Input Variability
User input variability is a significant factor contributing to the complexity and slowness of AI models like ChatGPT. When users interact with these systems, they bring a wide range of linguistic styles, preferences, and contexts that can vastly differ from one another. This variability manifests in several ways: **lexical diversity**, where users employ different words and phrases to convey the same meaning; **syntactic variations**, such as differences in sentence structure and grammar; and **semantic nuances**, where subtle differences in meaning can significantly impact the interpretation of queries. For instance, a user might ask "What are the best ways to improve my writing skills?" while another might phrase it as "How can I become a better writer?" These questions, though semantically similar, require the AI to recognize and adapt to these variations in real-time. This adaptability is computationally intensive because it involves complex natural language processing (NLP) tasks such as tokenization, part-of-speech tagging, named entity recognition, and dependency parsing. Moreover, user input can be **context-dependent**, meaning that the same query might have different implications based on previous interactions or external knowledge. For example, if a user asks "What is the capital of France?" after discussing European history, the AI needs to understand this contextual relationship to provide accurate and relevant responses. This context-aware processing adds another layer of complexity, as the model must maintain a memory of past interactions and integrate this information seamlessly. Additionally, **ambiguity and uncertainty** in user queries further complicate the processing. Users may ask ambiguous questions like "What is the best restaurant in town?" without specifying criteria such as cuisine or budget. The AI must then either seek clarification or make educated guesses based on general knowledge or user preferences, both of which require additional computational resources. The cumulative effect of these factors—lexical diversity, syntactic variations, semantic nuances, context dependency, and ambiguity—significantly increases the computational load on AI models like ChatGPT. Each unique input requires the model to perform extensive processing to understand and respond accurately, which can lead to slower response times compared to more predictable and standardized inputs. This inherent variability in user interactions underscores why optimizing for real-world user input remains a challenging but crucial aspect of improving AI performance and efficiency.
3. Real-Time Processing Demands
Real-time processing demands are a critical factor contributing to the perceived slowness of ChatGPT. When users interact with ChatGPT, they expect immediate and seamless responses, akin to human-like conversation. However, the complexity of processing user queries in real-time imposes significant computational challenges. Each query involves multiple layers of processing: understanding the context, parsing the input, generating a coherent response, and ensuring it aligns with ethical guidelines and knowledge boundaries. This multi-step process requires substantial computational resources and sophisticated algorithms to handle the nuances of natural language. One of the primary reasons for the delay is the sheer volume of data that needs to be processed. ChatGPT relies on large language models trained on vast amounts of text data, which necessitates powerful servers and advanced hardware to manage these operations efficiently. The model must quickly retrieve relevant information from its vast knowledge base, perform complex calculations to generate contextually appropriate responses, and do so while maintaining a high level of accuracy and relevance. This real-time data retrieval and processing can be time-consuming, especially when dealing with intricate or open-ended questions that require deeper analysis. Moreover, real-time processing demands are exacerbated by the need for continuous learning and adaptation. As new information becomes available or user preferences evolve, the model must incorporate these changes without compromising performance. This dynamic nature of real-time processing means that ChatGPT cannot simply rely on precomputed responses but must dynamically generate them based on current inputs and contexts. The latency introduced by these dynamic computations can sometimes make the interaction feel slower than expected. Additionally, ensuring that responses are not only accurate but also safe and respectful adds another layer of complexity. Real-time processing must include checks for sensitive topics, adherence to ethical standards, and compliance with regulatory requirements. These additional checks, while crucial for maintaining trust and safety, further contribute to the processing time. In summary, the real-time processing demands of ChatGPT are multifaceted and demanding. They involve complex data retrieval, sophisticated algorithmic processing, continuous learning, and stringent safety checks—all of which require significant computational power and advanced infrastructure. These factors collectively contribute to the perceived slowness of ChatGPT interactions, highlighting the intricate balance between speed, accuracy, and safety in AI-driven user interfaces.