Why Is Character Ai Not Working
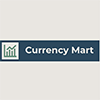
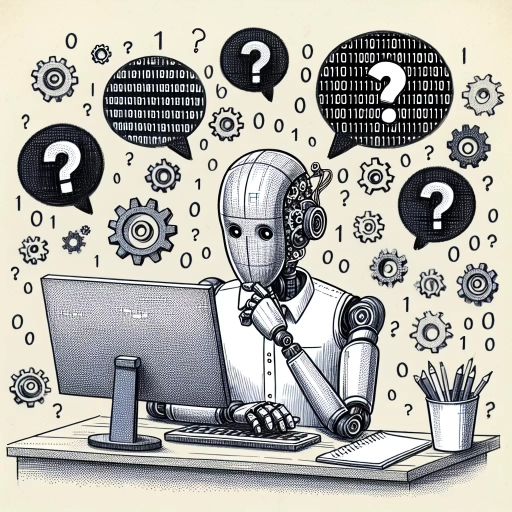
In the rapidly evolving landscape of artificial intelligence, Character AI has emerged as a promising tool for generating human-like text and engaging in conversational interactions. However, despite its potential, many users have encountered instances where Character AI fails to perform as expected. This article delves into the multifaceted reasons behind these failures, exploring three key areas: Technical Issues and System Failures, Data Quality and Training Limitations, and User Input and Interaction Challenges. By understanding these factors, we can better navigate the complexities of AI technology and identify potential solutions. Technical issues, such as server downtime or software glitches, often disrupt the seamless operation of Character AI. Additionally, the quality of the data used to train these models significantly impacts their performance, while user input and interaction dynamics can also hinder optimal functionality. This article will begin by examining the technical aspects that underpin these failures, starting with an in-depth look at Technical Issues and System Failures.
Technical Issues and System Failures
In today's interconnected world, where technology plays a pivotal role in nearly every aspect of our lives, technical issues and system failures can have far-reaching and debilitating consequences. These disruptions can arise from various sources, each with its own set of complexities and challenges. One common culprit is the lack of server capacity, which can lead to slow response times and complete system crashes when demand exceeds available resources. Additionally, software bugs and glitches can introduce unforeseen errors, compromising data integrity and user experience. Network connectivity problems also pose significant threats, as they can sever critical communication links and bring operations to a grinding halt. Understanding these root causes is essential for mitigating their impact and ensuring the reliability of our technological infrastructure. This article delves into these critical areas—lack of server capacity, software bugs and glitches, and network connectivity problems—to provide a comprehensive overview of technical issues and system failures.
Lack of Server Capacity
When exploring the reasons behind Character AI's downtime, one critical factor to consider is the lack of server capacity. This issue can significantly impact the performance and reliability of the platform, leading to frustrating experiences for users. Server capacity refers to the ability of a server to handle a certain volume of requests and data processing without compromising speed or functionality. When Character AI's servers are overwhelmed by an influx of user interactions, it can result in slow response times, errors, and even complete system failures. The consequences of insufficient server capacity are multifaceted. For instance, if the servers are not adequately scaled to meet demand, they may struggle to process complex AI-driven conversations efficiently. This can lead to delayed responses or incomplete interactions, which undermine the user experience and trust in the platform. Moreover, during peak usage times or when new features are introduced, the strain on servers can become particularly acute, exacerbating these issues. From a technical standpoint, addressing server capacity involves several strategies. One approach is horizontal scaling, where additional servers are added to distribute the load more evenly. Vertical scaling, which involves upgrading existing servers with more powerful hardware, is another option. However, both methods require careful planning and resource allocation to ensure seamless integration and optimal performance. Furthermore, proactive monitoring and predictive analytics play crucial roles in mitigating server capacity issues. By continuously tracking server performance metrics and anticipating potential bottlenecks, developers can take preemptive measures such as load balancing or temporary redirects to manage traffic more effectively. This proactive approach helps in maintaining a stable and responsive system even under high demand. In addition to these technical solutions, it's also important for developers to consider user behavior and usage patterns. Understanding peak usage times and common user interactions can help in optimizing server resources accordingly. For example, if certain features are more frequently used during specific hours of the day, servers can be pre-emptively scaled up to handle this increased load. In conclusion, the lack of server capacity is a significant contributor to technical issues and system failures in Character AI. By recognizing this challenge and implementing robust scaling strategies, proactive monitoring, and user-centric optimizations, developers can significantly enhance the reliability and performance of the platform. This not only improves user satisfaction but also ensures that Character AI remains a robust and dependable tool for those relying on its advanced AI capabilities.
Software Bugs and Glitches
Software bugs and glitches are fundamental contributors to technical issues and system failures, particularly in complex AI systems like Character AI. These errors can arise from various sources, including coding mistakes, compatibility problems, and unforeseen interactions between different components of the software. A bug is typically a defect or flaw in the code that causes the program to behave in an unintended way, often resulting in crashes, data corruption, or incorrect outputs. Glitches, on the other hand, are temporary or intermittent problems that may not be as severe but can still disrupt user experience. The complexity of AI systems exacerbates the challenge of identifying and resolving these issues. For instance, Character AI relies on sophisticated algorithms and machine learning models that process vast amounts of data. Even minor errors in these algorithms can lead to significant malfunctions. Moreover, the dynamic nature of AI systems means that bugs and glitches can emerge as new data is introduced or as the system evolves over time. Debugging these issues is a meticulous process that requires thorough testing and analysis. Developers must use various tools and techniques to isolate the source of the problem, which can be time-consuming and resource-intensive. In some cases, bugs may be so deeply embedded that they require a complete overhaul of certain sections of the code. User feedback plays a crucial role in identifying bugs and glitches. When users report anomalies or errors, it provides developers with valuable insights into where the system might be failing. This feedback loop is essential for continuous improvement and ensuring that the AI system operates as intended. Furthermore, preventive measures such as rigorous testing protocols, code reviews, and adherence to best practices in software development can significantly reduce the likelihood of bugs and glitches. Automated testing tools can also help detect potential issues before they reach production environments. In summary, software bugs and glitches are inherent risks in the development and operation of AI systems like Character AI. Understanding their causes, implementing robust debugging processes, and leveraging user feedback are critical steps in mitigating these risks and ensuring reliable system performance. By addressing these technical issues proactively, developers can enhance user experience and maintain the integrity of complex AI systems.
Network Connectivity Problems
Network connectivity problems are a common and frustrating issue that can significantly impact the functionality of various systems, including AI-driven platforms like Character AI. These problems can arise from a multitude of sources, making diagnosis and resolution challenging. At the core, network connectivity issues often stem from either hardware or software malfunctions. On the hardware side, faulty network adapters, routers, or modems can disrupt data transmission. For instance, a malfunctioning Ethernet cable or a router with outdated firmware can prevent devices from establishing a stable connection. Similarly, physical obstructions or interference from other devices can weaken wireless signals, leading to dropped connections and slow data transfer rates. Software-related issues are equally prevalent. Misconfigured network settings, outdated drivers, or conflicts between different network protocols can all contribute to connectivity problems. Firewalls and antivirus software, while essential for security, can sometimes block necessary traffic if not properly configured. Moreover, DNS resolution issues or problems with the operating system's network stack can prevent devices from resolving hostnames or establishing connections. In the context of Character AI, network connectivity issues can manifest in various ways. Users may experience delays or failures in loading the AI interface, slow response times to queries, or complete disconnections during interactions. These issues can be particularly problematic if the AI is being used for critical tasks such as customer service or data analysis. To mitigate these problems, it is crucial to follow a systematic approach to troubleshooting. This includes checking the physical connections and ensuring that all hardware components are functioning correctly. Next, verifying the network settings and updating drivers and firmware as necessary can help resolve software-related issues. Utilizing network diagnostic tools to identify bottlenecks and performing basic troubleshooting steps like restarting routers and modems can also be effective. Additionally, ensuring that the network environment is optimized for low latency and high bandwidth can improve the overall performance of AI systems. This might involve upgrading network infrastructure or implementing quality of service (QoS) policies to prioritize traffic critical to the AI's operation. In conclusion, network connectivity problems are a significant technical challenge that can impact the reliability and performance of AI systems like Character AI. By understanding the potential causes and taking proactive steps to maintain robust network infrastructure, users can minimize downtime and ensure seamless interactions with these advanced technologies. Regular maintenance, thorough troubleshooting, and optimization of network settings are key strategies in preventing and resolving these issues effectively.
Data Quality and Training Limitations
In the era of data-driven decision-making, the quality of data is paramount. However, several limitations can compromise the integrity and effectiveness of data, leading to significant technical issues and system failures. This article delves into three critical areas where data quality and training limitations can have profound impacts: inadequate training data, biased or inconsistent data sets, and insufficient model updates. Each of these factors can undermine the accuracy and reliability of data-driven systems, ultimately affecting their performance and trustworthiness. Inadequate training data can result in models that are not adequately prepared to handle real-world scenarios, while biased or inconsistent data sets can introduce errors and unfair outcomes. Furthermore, insufficient model updates can render models obsolete and ineffective over time. Understanding these limitations is crucial for mitigating technical issues and preventing system failures, ensuring that data-driven systems operate at their best. By exploring these challenges in depth, we aim to provide insights that can help improve data quality and training practices, thereby enhancing the overall robustness of data-driven technologies.
Inadequate Training Data
Inadequate training data is a critical limitation that can significantly impede the performance and reliability of AI models, including those used in character AI systems. When the dataset used to train an AI model is insufficient, it can lead to a myriad of issues such as poor accuracy, biased outcomes, and an inability to generalize well to new or unseen data. For instance, if a character AI is trained on a dataset that lacks diversity in terms of language styles, cultural contexts, or demographic representation, it may struggle to understand and respond appropriately to users from diverse backgrounds. This can result in responses that are either irrelevant or offensive, which can erode user trust and satisfaction. Moreover, inadequate training data can exacerbate the problem of overfitting, where the model becomes overly specialized in recognizing patterns in the training data but fails to perform well on new, unseen data. This is particularly problematic for character AI systems that need to adapt to a wide range of user inputs and scenarios. Without comprehensive and high-quality training data, these systems may not be able to learn the nuances of human communication effectively, leading to subpar interactions. Another significant issue with inadequate training data is the potential for bias. If the training dataset reflects existing societal biases or lacks representation from certain groups, the AI model will likely perpetuate these biases in its responses. This can have serious ethical implications and may lead to discriminatory outcomes. For example, if a character AI is trained on data that predominantly reflects male perspectives, it may not be able to understand or respond appropriately to female users, thereby reinforcing gender biases. To mitigate these issues, it is essential to ensure that the training data is robust, diverse, and well-curated. This involves collecting data from a wide range of sources, ensuring that it represents various demographics and contexts, and continuously updating the dataset to reflect changing trends and user behaviors. Additionally, employing techniques such as data augmentation and transfer learning can help in leveraging existing datasets more effectively. By focusing on improving the quality and breadth of training data, developers can significantly enhance the performance and reliability of character AI systems, ultimately leading to better user experiences and more trustworthy interactions.
Biased or Inconsistent Data Sets
**Biased or Inconsistent Data Sets** One of the critical challenges in ensuring the effectiveness of AI models, such as those powering Character AI, is the quality and consistency of the data sets used for training. Biased or inconsistent data can significantly undermine the performance and reliability of these models. When data sets are biased, they reflect skewed perspectives or demographics, leading to AI outputs that perpetuate these biases. For instance, if a language model is trained on text data that predominantly represents a particular gender or ethnic group, it may struggle to understand and respond appropriately to inputs from other groups. This not only limits the model's utility but also raises ethical concerns about fairness and inclusivity. Inconsistencies in data sets can arise from various sources, including errors in data collection, variations in formatting, and discrepancies in labeling. For example, if some data points are labeled incorrectly or inconsistently, the model may learn to recognize patterns that are not accurate, resulting in poor generalization to new, unseen data. Furthermore, inconsistent data can lead to overfitting or underfitting, where the model either becomes too specialized to the training data or fails to capture meaningful patterns altogether. The impact of biased or inconsistent data is particularly pronounced in AI applications that require high levels of accuracy and fairness, such as healthcare, finance, and education. In these domains, flawed data can lead to decisions that are not only suboptimal but also potentially harmful. For instance, a medical diagnosis AI trained on biased data might misdiagnose conditions more frequently for certain patient groups, leading to adverse health outcomes. To mitigate these issues, it is essential to implement rigorous data quality checks and validation processes. This includes ensuring diversity and representativeness in the data sets, using multiple sources to cross-validate information, and employing techniques such as data normalization and preprocessing to standardize the data. Additionally, ongoing monitoring and feedback mechanisms can help identify and correct biases or inconsistencies as they arise. By prioritizing data quality and addressing these challenges proactively, developers can build more robust and reliable AI models that deliver consistent performance across diverse scenarios. Ultimately, the success of AI systems like Character AI hinges on the integrity and reliability of their underlying data sets.