Who Is A
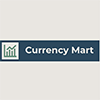
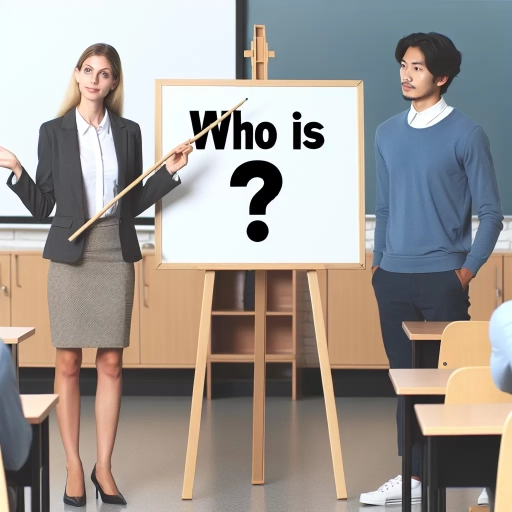
In today's data-driven world, the role of a data scientist has become increasingly pivotal. These professionals are at the forefront of extracting insights from vast amounts of data, driving business decisions, and innovating solutions across various industries. To fully appreciate the significance of data scientists, it is essential to delve into their multifaceted roles, educational backgrounds, and the evolving landscape of their field. Understanding the role of a data scientist involves recognizing their responsibilities in analyzing complex data sets, developing predictive models, and communicating findings effectively. Additionally, examining the educational and professional background of a data scientist reveals the rigorous academic preparation and continuous learning required to excel in this field. Looking ahead, the future of data science holds immense promise, with career prospects expanding rapidly as organizations seek to leverage data for competitive advantage. By exploring these aspects, we can gain a comprehensive understanding of what it means to be a data scientist and why their role is so critical in modern times. Let us begin by understanding the role of a data scientist.
Understanding the Role of a Data Scientist
In today's data-driven world, the role of a data scientist has become increasingly pivotal. These professionals are at the forefront of extracting insights from vast amounts of data, driving informed decision-making, and fostering innovation. To fully grasp the significance of their work, it is essential to delve into three key aspects: **Key Responsibilities and Tasks**, which outline the daily activities and challenges faced by data scientists; **Technical Skills and Tools**, highlighting the advanced competencies and technologies they employ; and **Impact on Business and Society**, exploring how their work influences organizational strategies and broader societal trends. By understanding these facets, we can appreciate the multifaceted nature of a data scientist's role and its profound implications. This article aims to provide a comprehensive overview of these critical dimensions, ultimately shedding light on **Understanding the Role of a Data Scientist**.
Key Responsibilities and Tasks
**Key Responsibilities and Tasks** Understanding the role of a data scientist involves delving into the multifaceted responsibilities and tasks that define this critical position. At the heart of their job, data scientists are tasked with extracting insights from complex data sets to inform business decisions, drive innovation, and solve real-world problems. One of their primary responsibilities is data collection and preprocessing, which involves gathering data from various sources, cleaning it to ensure accuracy, and transforming it into a usable format. This process often requires proficiency in programming languages such as Python or R, as well as familiarity with databases and data storage solutions. Data scientists must also possess strong analytical skills to interpret and model data effectively. This includes applying statistical techniques, machine learning algorithms, and data visualization tools to uncover patterns and trends within the data. For instance, they might use regression analysis to predict future outcomes or employ clustering algorithms to segment customer bases. Additionally, they are responsible for developing and deploying predictive models that can be integrated into operational systems, ensuring these models are scalable and perform well in real-time environments. Communication is another vital aspect of a data scientist's role. They must be able to present complex findings in a clear, concise manner to both technical and non-technical stakeholders. This involves creating compelling visualizations using tools like Tableau or Power BI and crafting narratives that highlight key insights and recommendations. Furthermore, data scientists often collaborate with cross-functional teams including product managers, engineers, and business leaders to align their work with organizational goals. Another key task is staying updated with the latest advancements in the field. This includes keeping abreast of new technologies, methodologies, and tools that can enhance their work. For example, they might explore emerging trends such as deep learning or natural language processing to identify potential applications within their organization. Continuous learning through attending conferences, participating in online forums, and engaging in professional development activities is essential for maintaining expertise. Finally, ethical considerations play a significant role in the responsibilities of a data scientist. They must ensure that their work adheres to ethical standards by handling sensitive information responsibly, avoiding biases in algorithms, and complying with regulatory requirements such as GDPR or HIPAA. By balancing technical acumen with ethical awareness and strong communication skills, data scientists can drive meaningful impact across various industries while upholding the highest standards of professionalism. In summary, the role of a data scientist is dynamic and multifaceted, requiring a blend of technical expertise, analytical prowess, effective communication skills, and ethical mindfulness to deliver value-driven insights that drive business success.
Technical Skills and Tools
**Technical Skills and Tools** To excel as a data scientist, one must possess a robust arsenal of technical skills and tools. At the core of these skills is proficiency in programming languages, particularly Python and R, which are widely used for data manipulation, analysis, and visualization. Data scientists must also be adept at working with databases, including SQL and NoSQL systems, to extract and manage large datasets. Additionally, knowledge of big data technologies such as Hadoop, Spark, and cloud-based platforms like AWS or Azure is crucial for handling vast amounts of data. Machine learning and deep learning are fundamental components of a data scientist's toolkit. Familiarity with libraries like TensorFlow, PyTorch, and scikit-learn allows data scientists to build predictive models and neural networks that drive insights from complex data sets. Data visualization tools like Tableau, Power BI, or Matplotlib and Seaborn in Python are essential for communicating findings effectively to both technical and non-technical stakeholders. Statistical knowledge is another critical skill, enabling data scientists to understand the underlying principles of data analysis and make informed decisions. This includes understanding hypothesis testing, regression analysis, and time series analysis. Furthermore, data scientists need to be proficient in data preprocessing techniques such as cleaning, normalization, and feature engineering to ensure that the data is ready for analysis. In today's fast-paced environment, collaboration is key. Therefore, proficiency in version control systems like Git and experience with collaborative development platforms such as Jupyter Notebooks or Google Colab are highly valued. Moreover, understanding of agile methodologies and DevOps practices helps in integrating data science projects into broader organizational workflows. Lastly, staying updated with the latest advancements in the field is paramount. This involves continuous learning through courses, workshops, and participation in professional communities such as Kaggle or attending conferences like NIPS or IJCAI. By mastering these technical skills and tools, a data scientist can effectively extract insights from data, build robust models, and drive business decisions with confidence. This comprehensive skill set not only enhances their ability to solve complex problems but also positions them as invaluable assets within any organization seeking to leverage data-driven strategies for growth and innovation.
Impact on Business and Society
The impact of data science on business and society is profound and multifaceted. In the business realm, data scientists play a crucial role in driving decision-making processes by analyzing vast amounts of data to uncover insights that can lead to strategic advantages. For instance, predictive analytics can help companies forecast market trends, optimize supply chains, and personalize customer experiences, thereby enhancing operational efficiency and customer satisfaction. This data-driven approach also enables businesses to mitigate risks more effectively by identifying potential issues before they escalate. Moreover, data science fuels innovation by facilitating the development of new products and services tailored to specific market needs, which can significantly boost revenue and competitiveness. In society, the influence of data science extends beyond the corporate world. It has transformative potential in various sectors such as healthcare, education, and public policy. For example, in healthcare, data scientists can analyze patient data to develop personalized treatment plans, predict disease outbreaks, and improve patient outcomes. In education, data analytics can help tailor learning experiences to individual students' needs, improving academic performance and reducing dropout rates. In public policy, data science informs decision-making by providing evidence-based insights on issues like economic development, crime prevention, and environmental sustainability. Additionally, data science contributes to social good by enabling organizations to track the effectiveness of their initiatives and make data-driven improvements. The ethical implications of data science also warrant consideration. As data collection and analysis become more pervasive, concerns about privacy, bias, and transparency grow. Data scientists must navigate these ethical complexities to ensure that their work benefits society without compromising individual rights or perpetuating existing inequalities. By doing so, they can foster trust in data-driven decision-making processes and ensure that the benefits of data science are equitably distributed. Overall, the role of a data scientist is not just about analyzing numbers; it involves leveraging data to drive meaningful change across various domains. Their work has the potential to revolutionize how businesses operate and how societies function, making them indispensable in today's data-driven world. As technology continues to evolve, the impact of data science will only continue to grow, underscoring the importance of understanding the role that data scientists play in shaping our future.
The Educational and Professional Background of a Data Scientist
In the rapidly evolving landscape of data-driven decision-making, the role of a data scientist has become increasingly pivotal. To excel in this field, one must possess a robust educational and professional background that encompasses a blend of academic rigor, practical experience, and ongoing learning. This article delves into the essential components that define the career trajectory of a data scientist, starting with the **Academic Requirements and Degrees** necessary to lay a solid foundation in statistics, mathematics, and computer science. It then explores **Relevant Work Experience and Projects**, highlighting the importance of hands-on experience in real-world data analysis and problem-solving. Finally, it emphasizes **Continuous Learning and Professional Development**, underscoring the need for data scientists to stay abreast of emerging technologies and methodologies. By understanding these critical aspects, we can gain a comprehensive insight into the multifaceted role of a data scientist, ultimately shedding light on **Understanding the Role of a Data Scientist**.
Academic Requirements and Degrees
### Academic Requirements and Degrees To embark on a career as a data scientist, one must navigate a rigorous academic landscape that emphasizes both theoretical foundations and practical skills. Typically, aspiring data scientists begin with a strong undergraduate degree in a quantitative field such as mathematics, statistics, computer science, or engineering. These programs provide a solid groundwork in linear algebra, calculus, probability theory, and programming languages like Python, R, or SQL. Many data scientists also pursue advanced degrees, with a Master's in Data Science or related fields being highly sought after. A Master's program delves deeper into specialized topics such as machine learning, data visualization, and big data analytics, while also offering hands-on experience through projects and internships. Ph.D. programs in Data Science or related disciplines are also available for those who wish to delve into research-oriented roles or academia. These programs focus on advanced research methodologies and the development of new algorithms and techniques. Regardless of the degree level, coursework often includes data mining, statistical modeling, and computational methods, ensuring that graduates are well-equipped to handle complex data sets and derive meaningful insights. In addition to formal education, many data scientists engage in continuous learning through certifications, online courses, and professional development workshops. Certifications like Certified Data Scientist (CDS) or Certified Analytics Professional (CAP) can enhance job prospects by demonstrating expertise in specific tools and methodologies. Furthermore, staying updated with industry trends and advancements is crucial due to the rapidly evolving nature of data science. This involves participating in conferences, reading scholarly articles, and contributing to open-source projects. The educational journey of a data scientist is not just about accumulating degrees but also about developing a versatile skill set that includes technical proficiency, analytical thinking, and communication skills. Data scientists must be able to interpret complex data insights and present them in a clear, actionable manner to both technical and non-technical stakeholders. This blend of academic rigor and practical application ensures that data scientists are equipped to tackle the diverse challenges of their field and drive innovation in various industries. Ultimately, the academic requirements for becoming a data scientist are designed to foster a deep understanding of data-driven decision-making processes and the ability to leverage data for strategic advantage.
Relevant Work Experience and Projects
In the realm of data science, relevant work experience and projects are pivotal in showcasing a professional's ability to apply theoretical knowledge in practical, real-world scenarios. For a data scientist, a robust portfolio of work experience and projects not only highlights technical skills but also demonstrates problem-solving capabilities, analytical thinking, and the ability to drive business value through data-driven insights. A data scientist with extensive experience in industry-leading companies has likely worked on diverse projects that span multiple domains, such as finance, healthcare, and technology. For instance, they might have led a team in developing predictive models to forecast stock market trends, leveraging machine learning algorithms and large datasets to provide actionable insights for investment strategies. In another project, they could have collaborated with healthcare professionals to analyze patient data and develop risk models for disease diagnosis, contributing significantly to improving patient outcomes. Moreover, involvement in open-source projects or contributions to data science communities further underscores a data scientist's commitment to innovation and continuous learning. Participating in hackathons or Kaggle competitions, for example, showcases their ability to work under pressure and innovate within tight deadlines. These experiences also highlight their proficiency in using various tools and technologies such as Python, R, SQL, and cloud platforms like AWS or Google Cloud. Additionally, experience in data visualization tools like Tableau or Power BI is crucial for communicating complex data insights effectively to both technical and non-technical stakeholders. A data scientist who has successfully implemented data visualization dashboards for executive teams or clients demonstrates their understanding of the importance of clear communication in driving business decisions. Projects that involve working with big data technologies such as Hadoop, Spark, or NoSQL databases also indicate a data scientist's ability to handle large-scale datasets efficiently. Experience with cloud-based services like Azure Databricks or Google BigQuery shows adaptability and the capacity to scale solutions according to organizational needs. Ultimately, a strong portfolio of relevant work experience and projects is essential for a data scientist looking to advance their career. It serves as tangible evidence of their expertise and readiness to tackle complex challenges in various industries. By highlighting these experiences within their educational and professional background, a data scientist can convincingly demonstrate their value as a skilled professional capable of driving impactful change through data-driven solutions.
Continuous Learning and Professional Development
Continuous learning and professional development are paramount for a data scientist, given the rapidly evolving nature of the field. The landscape of data science is characterized by constant advancements in technologies, methodologies, and tools, making it imperative for professionals to stay updated and adapt quickly. This involves a commitment to ongoing education, whether through formal courses, workshops, conferences, or self-directed learning. Data scientists must be adept at acquiring new skills and knowledge in areas such as machine learning algorithms, deep learning techniques, natural language processing, and data visualization tools. Moreover, staying current with industry trends and breakthroughs is crucial; this can be achieved by engaging with professional networks, participating in online forums and communities like Kaggle or GitHub, and reading scholarly articles and industry publications. Professional development extends beyond technical skills; it also encompasses soft skills such as communication, collaboration, and problem-solving. Data scientists often work in interdisciplinary teams and must be able to translate complex technical insights into actionable recommendations for non-technical stakeholders. Therefore, developing strong presentation skills, learning to articulate complex ideas simply, and fostering a collaborative mindset are essential components of their professional growth. Additionally, certifications and specialized training programs can significantly enhance a data scientist's career trajectory. Certifications like Certified Data Scientist (CDS) or Certified Analytics Professional (CAP) validate expertise and demonstrate a commitment to professional standards. Participating in hackathons or data science competitions can also provide valuable experience and exposure to real-world challenges. Incorporating continuous learning into daily routines is key. This might involve setting aside dedicated time for reading research papers, experimenting with new tools, or contributing to open-source projects. Leveraging resources such as MOOCs (Massive Open Online Courses) from platforms like Coursera, edX, or Udacity can provide structured learning opportunities without the need for a traditional classroom setting. Ultimately, the pursuit of continuous learning and professional development not only ensures that data scientists remain relevant in their field but also positions them for leadership roles and innovative contributions. By embracing a culture of lifelong learning, data scientists can drive innovation, solve complex problems more effectively, and contribute meaningfully to their organizations' success. This mindset of continuous improvement is a hallmark of successful data scientists and a critical component of their educational and professional background.
The Future of Data Science and Career Prospects
In the rapidly evolving landscape of technology, data science stands at the forefront, driving innovation and transformation across various industries. The future of data science is poised to be even more dynamic, with emerging trends such as artificial intelligence, machine learning, and big data analytics set to redefine how we extract insights and make decisions. As these trends gain momentum, the job market is experiencing a surge in demand for skilled data scientists, offering unprecedented growth opportunities for those entering this field. However, this growth also brings forth challenges and ethical considerations that must be addressed to ensure responsible and ethical use of data. Understanding these facets is crucial for anyone looking to navigate the exciting yet complex world of data science. In this article, we will delve into emerging trends in data science, explore the job market demand and growth opportunities, and discuss the challenges and ethical considerations that come with this field. Ultimately, this will help us understand the role of a data scientist in today's and tomorrow's world.
Emerging Trends in Data Science
As we delve into the future of data science, it becomes evident that several emerging trends are set to redefine the landscape of this dynamic field. One of the most significant trends is the integration of **Artificial Intelligence (AI) and Machine Learning (ML)**. These technologies are not only enhancing predictive analytics but also automating complex data processing tasks, allowing data scientists to focus on higher-level strategic decisions. **Deep Learning**, a subset of ML, is particularly gaining traction due to its ability to handle large datasets and extract meaningful insights from unstructured data. Another trend that is gaining momentum is **Big Data Analytics**. With the exponential growth in data generation from various sources such as IoT devices, social media, and sensors, the need for scalable and efficient data processing solutions has never been more critical. **Cloud Computing** is playing a pivotal role here by providing scalable infrastructure that can handle massive datasets and support real-time analytics. This convergence of big data and cloud computing is enabling organizations to make data-driven decisions faster than ever before. **Natural Language Processing (NLP)** is another area that is witnessing rapid advancements. NLP algorithms are becoming more sophisticated, enabling better text analysis, sentiment analysis, and even generating human-like text. This has far-reaching implications for industries such as customer service, marketing, and healthcare where understanding and generating human language is crucial. The rise of **Explainable AI (XAI)** is also a significant trend. As AI models become more complex, there is an increasing need to understand how these models arrive at their decisions. XAI aims to provide transparency and interpretability to AI models, which is essential for building trust in AI-driven systems. Furthermore, **Ethical Data Science** is emerging as a critical aspect of the field. With data science influencing various aspects of life, ethical considerations such as data privacy, bias in algorithms, and responsible use of data are becoming paramount. Data scientists are now expected not only to be technically proficient but also ethically aware. Lastly, **Quantum Computing** holds immense potential for data science. Quantum computers can process certain types of data much faster than classical computers, which could revolutionize fields like cryptography, optimization problems, and simulations. While still in its early stages, quantum computing promises to unlock new possibilities in data analysis and processing. These emerging trends collectively point towards a future where data science will be more integrated, automated, and ethically conscious. For professionals in this field, understanding and adapting to these trends will be crucial for career growth and staying relevant in an ever-evolving industry. The future of data science is not just about technological advancements but also about leveraging these advancements responsibly and ethically to drive meaningful impact across various sectors.
Job Market Demand and Growth Opportunities
The job market demand for data science professionals is experiencing unprecedented growth, driven by the increasing reliance on data-driven decision-making across various industries. As businesses continue to leverage big data and advanced analytics to gain competitive advantages, the demand for skilled data scientists, analysts, and engineers has skyrocketed. This trend is expected to persist well into the future, with the global data science market projected to expand significantly over the next decade. Several factors contribute to this robust demand. Firstly, technological advancements in artificial intelligence (AI), machine learning (ML), and cloud computing have made it more feasible for organizations to collect, process, and analyze vast amounts of data. This has opened up new avenues for insights that can drive innovation, improve operational efficiency, and enhance customer experiences. Secondly, industries such as healthcare, finance, retail, and manufacturing are increasingly adopting data science to solve complex problems and uncover hidden opportunities. Career prospects in data science are not only abundant but also diverse. Data scientists can find roles in research institutions, government agencies, startups, and large corporations. Specializations within the field include data engineering, business analytics, predictive modeling, and data visualization. Moreover, the rise of emerging technologies like IoT (Internet of Things) and blockchain further expands the scope of data science applications. The skills required to succeed in this field are multifaceted. Proficiency in programming languages such as Python and R is essential, along with expertise in statistical modeling and machine learning algorithms. Additionally, strong communication skills are crucial for translating complex data insights into actionable recommendations for stakeholders. As a result, educational programs and professional certifications in data science are becoming more popular as individuals seek to capitalize on these growth opportunities. In terms of geographical distribution, while traditional tech hubs like Silicon Valley and New York City remain hotspots for data science jobs, other regions are also seeing significant growth. Cities in Asia such as Singapore and Bangalore are emerging as major centers for data science talent due to their robust tech ecosystems and government support for innovation. Overall, the future of data science looks promising with substantial career prospects and continuous growth opportunities. As technology evolves and more industries adopt data-driven strategies, the demand for skilled professionals in this field will continue to rise. For those interested in pursuing a career in data science, now is an opportune time to invest in education and training to capitalize on this burgeoning market.
Challenges and Ethical Considerations
As data science continues to shape the future of various industries, it is imperative to address the challenges and ethical considerations that accompany its rapid advancement. One of the primary challenges is ensuring data privacy and security. With the increasing volume and complexity of data, protecting sensitive information from breaches and unauthorized access becomes a daunting task. This necessitates robust security protocols and stringent compliance with regulations such as GDPR and CCPA, which can be resource-intensive and costly. Moreover, the ethical dilemma of balancing individual privacy rights with the need for data-driven insights is a constant concern. For instance, while personalized recommendations can enhance user experiences, they often rely on extensive personal data collection, raising questions about consent and transparency. Another significant challenge is bias in data and algorithms. Machine learning models can perpetuate existing social biases if trained on biased datasets, leading to discriminatory outcomes in areas such as hiring, lending, and law enforcement. Addressing these biases requires careful data curation, diverse datasets, and regular audits to ensure fairness and equity. Additionally, the issue of explainability in AI models is crucial; as models become more complex, understanding their decision-making processes becomes increasingly difficult, which can hinder accountability and trust. The ethical considerations extend beyond technical issues to societal impacts. Data science has the potential to exacerbate existing inequalities if not managed responsibly. For example, predictive policing algorithms may disproportionately target marginalized communities, while AI-driven healthcare solutions could widen the gap in healthcare access if they are not designed with inclusivity in mind. Therefore, data scientists must adopt a human-centered approach, considering the broader social implications of their work and engaging in open dialogue with stakeholders to ensure that their innovations serve the greater good. Furthermore, the rapid evolution of data science raises questions about job displacement and the future of work. While data science creates new career opportunities, it also automates certain tasks, potentially displacing workers in traditional roles. This underscores the need for continuous education and retraining programs to help workers adapt to changing job landscapes. Ethical considerations here involve ensuring that technological advancements do not leave behind vulnerable populations and that benefits are shared equitably across society. In conclusion, as data science continues to drive innovation and growth, it is essential to confront the challenges and ethical considerations head-on. By prioritizing data privacy, addressing algorithmic bias, considering societal impacts, and fostering a culture of continuous learning, we can harness the full potential of data science while maintaining ethical standards that benefit all stakeholders. This balanced approach will be crucial in shaping a future where data science not only drives technological progress but also promotes social responsibility and equity.