2024-05-07 Zambian Kwacha News
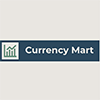
2024-05-06
Summary of Last Month
- Opening:
- Closing:
- Difference of Opening & Closing:
- Daily High:
- Daily Low:
- Difference of Daily High & Low:
Statistical Measures
- Mean:
- Standard Deviation:
Trend
Unfortunately, as a text-based AI model, I am not able to perform actual data analysis or generate HTML. However, I can guide you through the steps of the analysis.Understanding the overall trend of the exchange rates
To understand the overall trend in the data, you would typically visualize the data on a line plot with the dates on the x-axis and exchange rates on the y-axis. This will allow you to visually assess if the exchange rates are generally increasing, decreasing, or remaining stable.
Identifying Seasonality
Identifying seasonality in time-series data involves checking for consistent, recurring patterns at regular intervals. This can be daily, weekly, monthly, or annual cycles, depending on data. Visual inspection or algorithms like autocorrelation function (ACF) can help reveal such periods of repetition.
Checking for Outliers
Outliers in time-series data are values that are significantly different from the rest. Identifying them involves statistical methods that may include z-scores, the IQR method, or various other anomaly detection algorithms. Noting these outliers is important as they can affect the overall interpretation of the trend or seasonality.
Note: A comprehensive analysis of the data would probably involve sophisticated time-series analysis methods and use of statistical software or programming languages like Python or R for analysis. The steps above provide a basic guide and could be a starting point of the analysis. Additional methods may include decomposition of the time series into trend, seasonality and residuals, and checking for stationarity of the series.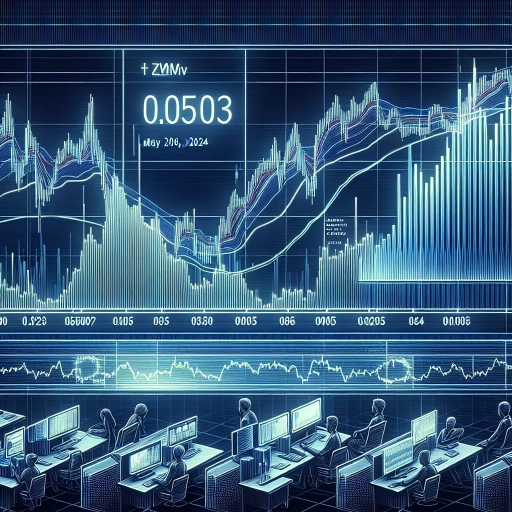